A bioinformatics-based immune-related prognostic index for lung adenocarcinoma that predicts patient response to immunotherapy and common treatments
Introduction
Lung cancer is one of the leading causes of cancer deaths worldwide, with 2.35 million new cases and 1.31 million deaths in the United States in 2021 alone (1). This disease is histologically divided into 2 categories, small cell lung cancer (SCLC) and non-small cell lung cancer (NSCLC). Within NSCLC, the major histological subtype, lung adenocarcinoma (LUAD) accounts for 40% of cases (2). Treating lung cancer involves a combination of surgical resection, chemotherapy, radiotherapy, and immunotherapy (3). Chemotherapy and molecular-targeted therapy are 2 common treatment strategies used for LUAD, while first-line treatment for patients with advanced LUAD is platinum-based combination chemotherapy (4). Immune checkpoint blockade (ICB) therapy is now an alternative option to treat LUAD (5). However, multiple factors, including the tumor microenvironment (TME), influence ICB therapy. The cellular heterogeneity of cancer cells and the TME contribute to disease progression and treatment resistance (6).
ICB therapy is an emerging anticancer weapon targeting T-cell regulatory pathways to enhance antitumor immune responses. Rather than activating the immune system to attack specific targets on tumor cells, this therapy aims to remove inhibitory pathways that block effective antitumor T-cell responses (7-9). ICB therapy has been shown to produce clinical responses in patients with a variety of tumor types (10). More specifically, anti-programmed death-ligand 1 (PD-L1) antibodies cause tumor regression in patients with different tumors, including NSCLC. Although ICB therapy shows significant clinical success, its efficacy varies widely among patients, especially NSCLC patients (11-15). However, existing biomarkers such as PD-L1 expression, TMB, etc. only reflect a small part of the tumor’s response to immunotherapy from a single perspective such as ligand expression and gene mutation, do not fully reflect the interaction of different factors in the complex TME, and are limited in prediction.
In this study, from the perspective of immunity, we hope to establish an indicator: immune-related gene prognostic index (IRGPI) that can better predict therapeutic efficacy than existing biomarkers through immune-related genes. At the same time, the different immune functions of genes in the model also more broadly reflect the important role of the immune system in tumor progression. Interestingly, in our analysis, the molecular and immunological characteristics of different IRGPI score-related subgroups were also quite different, which endows IRGPI with the ability to distinguish disease characteristics of LUAD patients. Our results suggested that IRGPI is an excellent prognostic index and has considerable advantages in predicting benefits of various treatments and distinguishing disease features for LUAD patients. We present the following article in accordance with the TRIPOD reporting checklist (available at https://jtd.amegroups.com/article/view/10.21037/jtd-22-494/rc).
Methods
Gene expression datasets and study design
RNA-sequencing data for 594 LUAD cases (59 normal tissues and 535 LUAD samples) and clinical and simple nucleotide variation (varscan2) data for 486 samples were downloaded from The Cancer Genome Atlas (TCGA) database (https://portal.gdc.cancer.gov/). Microarray data for 442 LUAD samples and survival data [Gene Expression Omnibus (GEO): GSE72094] were downloaded from the GEO database (https://www.ncbi.nlm.nih.gov/geo/). A list of immune genes was derived from the InnateDB (https://www.innatedb.com/) and the Immunology Database and Analysis Portal System (ImmPort) (https://www.immport.org/shared/home) databases. LUAD tumor samples downloaded from TCGA were divided into 6 immune subtypes based on 5 immune expression characteristics as previously described (16).
In this study, we first screened immune genes through differential expression analysis and weighted gene co-expression network analysis (WGCNA) analysis to obtain immune-related hub genes associated with LUAD. Using univariate and multivariate cox analyses, we built and tested a prognostic model and explored the molecular and immune characteristics of the two subgroups of the model. Finally, we tested the capability of the model to predict the patient’s response to ICB therapy through PD-L1 expression, Tumor Immune Dysfunction and Exclusion (TIDE), and other analyses. We also examined the sensitivity of different subgroups of the model to common treatments through Genomics of Drug Sensitivity in Cancer (GDSC) analysis. The study was conducted in accordance with the Declaration of Helsinki (as revised in 2013).
Immune-related hub genes
Using the “limma” package in R (17), we performed differential expression analysis of RNA-seq FPKM (fragments per kilobase of exon per million mapped fragments) in LUAD samples downloaded from TCGA to obtain differentially expressed genes [DEGs; absolute values of log fold change (FC) >1 and false discovery rate (FDR) adjusted P<0.05]. After comparing the DEGs and the list of immune-related genes, the “clusterProfiler” package in R (18) was used to perform Gene Ontology (GO) analysis and Kyoto Encyclopedia of Genes and Genomes (KEGG) analysis on differentially expressed immune-related genes.
WGCNA was performed for the analyzed genes to obtain co-expression modules using the “WGCNA” package in R (19). First, the Person correlation coefficient for any 2 genes was calculated and a similarity matrix was established. The optimal soft threshold power, β=4, was then obtained using unary linear regression, and the similarity matrix was transformed into an adjacency matrix based on the optimal soft threshold power before transforming it into a topological overlap matrix (TOM) to measure the correlation between 2 genes. After obtaining TOM, we clustered genes with 1-Tom set as the distance. Finally, by setting the minimum number of genes in each module to 25 and merging the threshold function of 0.25, we obtained clustering results. Three nongray modules (the blue, brown, and turquoise modules) were obtained, and the correlation of co-expression modules and traits (for both normal and tumor samples) were generated. We concluded that the absolute value for the turquoise module was the largest, and we constructed the network based on this.
A total of 50 immune-related hub genes significantly correlated (P<0.05) with the overall survival (OS) of LUAD patients, as shown by univariate Cox regression analysis of the 263 genes in the turquoise module. The “Survminer” package in R was used to calculate the optimal cut-off value for the expression of each gene. Based on the cut-off value, patients were divided into a high expression group and a low expression group. P values were calculated using the Kaplan-Meier (K-M) method and survival curves were plotted. The “Maftools” package in R (20) was then used to analyze 50 hub gene-related mutation information obtained for the LUAD patients.
Construction of IRGPI
To further screen genes that had a significant impact on patient prognosis, we conducted multivariate Cox regression analysis on immune-related hub genes and set the direction as ‘both’ to screen variables to obtain IRGPI. IRGPI, a Cox proportional-hazards model, was obtained by multiplying the expression values of specific genes by their coefficients. Based on the median value of IRGPI scores in the TCGA cohort, the cut-off point between IRGPI-high and IRGPI-low subgroups was obtained. The K-M method was used to perform survival analysis (log-rank test) for the TCGA and GEO cohorts to validate IRGPI values in predicting LUAD patient prognosis. Eventually, univariate and multivariate Cox regression analyses were performed for both IRGPI and common clinical factors, with the goal of further evaluating the independent prognostic ability of IRGPI.
Molecular and immune characteristics in 2 IRGPI subgroups
To obtain the differentially expressed signal pathways for the 2 IRGPI subgroups, we used the “limma” package in R to conduct differential expression analysis of the subgroups based on TCGA expression data to obtain their DEGs. Subsequently, the “clusterProfiler” package in R was used to generate gene set enriched analysis (GSEA) based on GO and KEGG gene sets downloaded from the MsigDB database (http://www.gsea-msigdb.org/). We then used the “Maftools” package in R to analyze the simple nucleotide variation data of LUAD patients and to obtain genetic mutation information for the 2 subgroups. Difference analysis was performed for PD-L1 expression and tumor mutation burden (TMB) for both subgroups using the “limma” package in R.
The CIBERSORT online tool (https://cibersort.stanford.edu/) was used to study immune cell infiltration of 22 unique immune cells using 1,000 iterations in each sample. With this, the TME landscape of IRGPI was obtained. We then compared immune cell infiltration with clinicopathological factors for both subgroups. Subsequently, we used the “limma” package in R to analyze differences in the proportions of the 22 different immune cells in the IRGPI subgroups and plotted K-M survival curves for immune cells showing significantly different proportions to determine which cells affected prognosis. To further explore the impact of immune-related function pathways on the prognosis of the subgroups, a single sample GSEA (ssGSEA) was performed using the “GSVA” package in R (21). Differential analysis was performed using the “limma” package in R and K-M survival curves were plotted for immune-related function pathways showing significant differences between the 2 subgroups.
To understand the association between immune and clinical grouping methods, we analyzed the distribution of immune subtypes and clinical stages for the different IRGPI subgroups using a chi-square test.
Prognostic ability, immune therapy response, and drug susceptibility of IRGPI
To evaluate the predicted efficacy of immunotherapy in the different IRGPI subgroups, we downloaded the TIDE score online calculator (http://tide.dfci.harvard.edu/). The differences in TIDE, microsatellite instability (MSI), T-cell dysfunction, and T-cell exclusion scores of the 2 groups were analyzed using the “limma” package in R. To confirm the reliability of IRGPI as a prognostic biomarker, the “timeROC” package in R was used to perform time-dependent receiver operating characteristic (ROC) curve analysis for IRGPI, TIDE, and tumor inflammation signature (TIS). The areas under the curve (AUC) for each of these variables were generated and multiple time comparisons were performed.
Finally, we compared the half-maximal inhibitory concentration (IC50) of chemotherapy and targeted drugs for the treatment of LUAD for the 2 IRGPI subgroups using the GDSC online tool (https://www.cancerRxgene.org) to obtain drug sensitivity information.
Statistical analysis
R software (version 4.1.1) (http://www.r-project.org/) and its corresponding R packages were used for all statistical data analysis and to generate graphs. Using the “clusterProfiler” package in R, we completed GO, KEGG, and GSEA analyses. Time-dependent ROC curve plotting and AUC calculations were performed using the “timeROC” package in R.
The chi-square test was used to determine whether differences in other groupings of IRGPI were significant between the 2 groups. A log-rank test was used to draw and compare K-M survival curves for univariate survival analysis. The Cox regression model was used to identify associated factors of survival outcomes. The AUC value of ROC curve greater than 0.7 is generally considered to be an excellent predictive model. P values less than 0.05 were considered statistically significant.
Results
Immune-related hub genes in LUAD
A graphical representation of the study design is shown in Figure 1. After differential expression analysis, a total of 8,109 DEGs were obtained. There were 1,864 genes found to be downregulated and 6,245 genes found to be upregulated in the tumor samples (Figure 2A). Intersection of the DEGs with the immune genes downloaded from InnateDB and ImmPort (website: https://cdn.amegroups.cn/static/public/jtd-22-494-01.pdf) yielded 681 immune-related DEGs, with 258 found to be downregulated genes and 423 found to be upregulated genes in tumor samples (Figure 2B). Functional enrichment analysis of immune-related DEGs showed that 681 immune-related DEGs were significantly correlated with 1,536 GO items and 56 KEGG pathways (website: https://cdn.amegroups.cn/static/public/jtd-22-494-02.pdf, Table S1). The top 8 GO items and KEGG pathways are displayed in Figure 2C,2D. Immune-related DEGs were enriched in multiple immune responses. Various cell types were found to mediate immunity and complement activation based on GO terms. KEGG pathways analysis showed many were enriched for cytokine signaling, chemokine signaling, and complement pathways. Thus, it was concluded that the screened genes were associated with immunity.
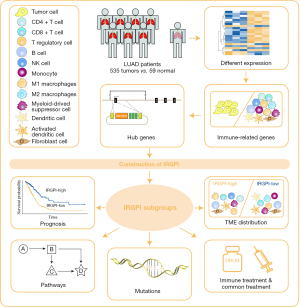
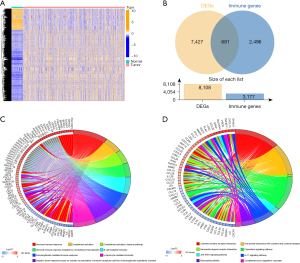
To obtain immune-related hub genes, WGCNA analysis was performed for immune-related DEGs. We first detected outliers in clustering and cut 2 samples by setting the upper limit to 20,000 (Figure 3A). The optimal soft-thresholding power was 4, based on the scale-free network (Figure 3B). Based on the optimal soft-thresholding and the average linkage hierarchical clustering power, 3 nongray modules containing a total of 588 genes were generated (Figure 3C,3D). Based on the absolute values and P values, the turquoise module, which was most closely related to LUAD, was selected for further analysis. The threshold weight was set to greater than 0.2, and there were 263 genes and 368 edges observable in the turquoise module (Figure 3E). Univariate cox regression analysis was performed on 263 genes in the turquoise module and 50 immune-related hub genes were identified that were closely related to the OS of LUAD patients (Figure 4A). Subsequently, to verify the prognostic correlation of hub genes, K-M survival analysis was performed and survival curves for the 16 genes with the highest and lowest prognostic risks are presented in Figure S1.
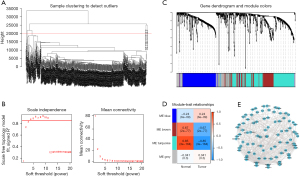
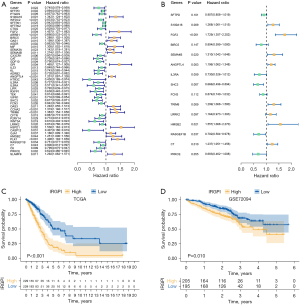
We then analyzed mutations present in the 50 immune-related hub genes. As shown in Figure S2, most genes contained missense mutations, and some had nonsense mutations, multiple hits, or frameshift deletions. The mutation rates of SEMA4B, C7, and LRRK2 were greater than 4%.
The IRGPI formula and prognostic value for IRGPI subgroups
We performed multivariate Cox regression analysis on 50 immune-related hub genes to identify independent prognostic genes and obtained 15 genes (SFTPD, S100A16, FGF2, BIRC5, SEMA4B, ANGPTL4, IL3RA, SHC3, FCN3, TRIM6, LRRK2, HMGB2, RASGEF1B, C7, and PRKCE) significantly associated with prognosis (Figure 4B). Next, we used a Cox proportional-hazards model to construct IRGPI based on these genes. The formula of IRGPI was as follows: IRGPI = SFTPD expression × (-0.10) + S100A16 expression × 0.24 + FGF2 expression × 0.55 + BIRC5 expression × (-0.22) + SEMA4B expression × 0.27 + ANGPTL4 expression × 0.18 + IL3RA expression × (-0.31) + SHC3 expression × (-0.41) + FCN3 expression × (-0.14) + TRIM6 expression × 0.26 + LRRK2 expression × 0.15 + HMGB2 expression × 0.44 + RASGEF1B expression × (-0.35) + C7 expression × 0.21 + PRKCE expression × 0.38.
To further confirm that IRGPI could be used as an independent prognostic factor, univariate and multivariate cox regression analyses were performed on IRGPI and other common clinical factors (Table S2). Univariate cox regression analysis showed that IRGPI, tumor (T) stage, metastasis (M) stage, and lymph node (N) stage significantly correlated with the prognosis of LUAD. Multivariate Cox regression analysis confirmed these data. We concluded that IRGPI was an independent prognostic factor after adjusting for other clinicopathological factors.
The median value of IRGPI in the TCGA cohort was used as the cut-off point to divide patients into high and low IRGPI score subgroups. In the TCGA cohort, the OS of the IRGPI-high subgroup was lower than the IRGPI-low subgroup (P<0.001, log-rank test, Figure 4C). The GEO cohort (GSE72094) showed a similar prognosis as the TCGA cohort (P=0.010, log-rank test; Figure 4D), confirming the ability of IRGPI to predict OS of LUAD patients.
Molecular characteristics of the IRGPI subgroups
First, GSEA analysis was performed to identify the enriched pathways in the 2 IRGPI subgroups (Table S3). The gene sets of the IRGPI-low subgroup were highly enriched in immune-related pathways (Figure 5A), indicating a better immune environment. However, gene sets of the IRGPI-high subgroup were abundantly enriched in the cell cycle pathway and pathways in cancer (Figure 5B), showing a high correlation with the development of cancer.
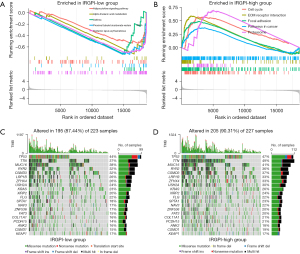
We then analyzed mutation data for the 2 subgroups to explore the characteristics from a molecular perspective. We first identified 20 genes with the largest number of mutations, we then analyzed mutation data of the 2 subgroups and displayed the mutation landscapes for the 20 genes (Figure 5C,5D). We found that missense mutations were the most common in both groups, followed by multiple hits and nonsense mutations. The mutation rates of TP53, TTN, MUC16, RYR2, CSMD3, LRP1B, ZFHX4, USH2A, KRAS, XIRP2, and FLGA in the 2 subgroups were all greater than 20%. The mutations of TTN (P=0.010) and PCDH15 (P<0.048) genes in the IRGPI-high subgroup were significantly higher than in the IRGPI-low subgroup.
Next, we analyzed the relationship between IRGPI score and PD-L1 expression level and TMB to explore the estimated benefits of ICB therapy. The expression of PD-L1 and TMB in the IRGPI-high subgroup was significantly greater than in the IRGPI-low subgroup (P=0.0036, P=0.0043; Figure S3A,S3B), suggesting that ICB therapy may result in a better response in IRGPI-high patients.
Immune characteristics of the 2 IRGPI subgroups
We obtained the TME landscape of LUAD after simulating the infiltration of 22 immune cells and grouped them into 2 IRGPI subgroups (Figure 6A). A comparison of 22 distinct immune cells and common clinical-pathological factors is shown in Figure 6B. We then used the “limma” package in R to compare the proportion of immune cells in the 2 IRGPI subgroups. The results showed that the abundance of activated memory cluster of differentiation 4 (CD4) T cells, resting natural killer (NK) cells, and macrophages M1 was higher in the IRGPI-high subgroup, while monocytes, macrophages M2, resting dendritic cells, and resting mast cells were widely distributed in the IRGPI-low subgroup (Figure 6C). For survival analysis of immune cells with significantly different distributions (Figure S4), we observed that the high expression of activated memory CD4 T cells, resting NK cells, and macrophages M1 resulted in poor prognosis (both P<0.05). In fact, these were all present at a higher abundance in the IRGPI-high subgroup. A higher level of resting dendritic cells predicted a better prognosis (P=0.048), and these were abundant in the IRGPI-low subgroup.
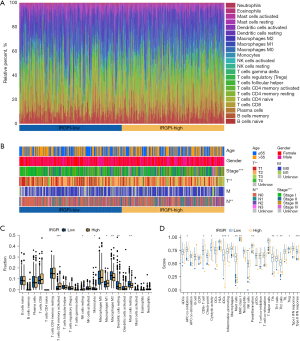
We then defined immune-related function pathway characteristics of the 2 subgroups based on the gene signature. We found that the IRGPI-high subgroup showed greater functions related to antigen presenting cell (APC) co-inhibition, CD8+ T cells, cytolytic activity, inflammation, major histocompatibility complex (MHC) class I molecules, para-inflammation, T follicular helper (Tfh) cells, T helper 1 (Th1) cells, and Th2 cells. The IRGPI-high group showed fewer functions related to immature dendritic cells (iDCs), mast cells, and type-II interferon (IFN) response (Figure 6D). In subsequent survival analyses (Figure S5), we found that CD8+ T cells and Treg enriched in the IRGPI-high subgroup predicted poor prognosis. Higher iDCs, mast cells, and type-II IFN response were associated with better prognosis in the IRGPI-low subgroup. These results also suggested that the prognostic value of IRGPI was related to differential enrichment of immune-related function pathways.
Relationship between IRGPI, immune subtypes, and clinical stage
Tumor samples from the TCGA were divided into 6 immune subtypes according to immune characteristics: C1 (wound healing), C2 (IFN-γ dominant), C3 (inflammatory), C4 (lymphocyte depleted), C5 (immunologically quiet), and C6 [transforming growth factor (TGF)-β dominant] (16). As shown in Figure 7A, there was a significant difference in the distribution of immune subtypes between the 2 subgroups (P=0.001). The proportion of the C1 subtype was greater in the IRGPI-high subgroup, and the proportion of the C3 subtype was greater in the IRGPI-low subgroup.
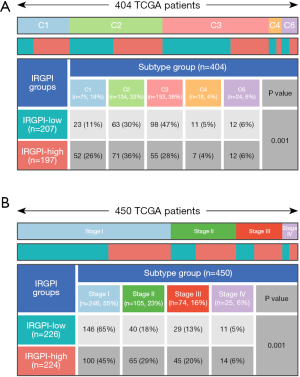
As for clinical stages, there was a significant difference in the distribution between the 2 subgroups (P=0.001; Figure 7B). The proportions of stage II and stage III in the IRGPI-high subgroup were high. In contrast, the proportion of stage I was high in the IRGPI-low subgroup. As a result, the IRGPI-high subgroup showed higher clinical stage, representing severe disease state and poor prognosis.
The relationship between IRGPI and ICB therapy or common treatments
TIDE was based on comprehensive analysis of the tumor expression spectrum to predict the efficacy of ICB therapy and was more accurate than PD-L1 expression and TMB (22). As shown in Figure 8A, we found that the T-cell dysfunction score of the IRGPI-high subgroup was higher than the IRGPI-low subgroup (P<0.001), suggesting that T cell-mediated immune processes may have been impaired in IRGPI-high patients (23,24). Benefits of ICB therapy showed no difference in the 2 subgroups since they had similar TIDE scores.
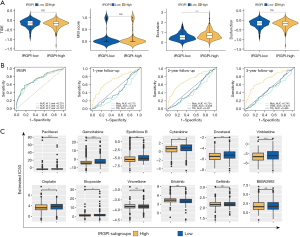
As shown in Figure 8B, we found that the AUCs of 1-, 2- and 3-year follow-up were all higher than 0.70 in the time-dependent ROC curve of IRGPI. This suggested that IRGPI was an important prognostic indicator. When comparing TIDE and TIS, the AUC of IRGPI was higher than TIDE and TIS at all follow-up times, showing that IRGPI had a better prognostic value than traditional biomarkers.
IRGPI had the ability to guide personalized treatment of LUAD based on drug susceptibility data for multiple treatments in the 2 subgroups through GDSC (Figure 8C). We found that the IC50 of 8 chemotherapeutic agents (paclitaxel, gemcitabine, epothilone B, cytarabine, docetaxel, vinblastine, cisplatin, and etoposide) in the IRGPI-high subgroup were significantly lower than in the IRGPI-low subgroup (both P<0.05), indicating that the response and efficacy of IRGPI-high patients to chemotherapy may be better. The IC50 values of the chemotherapy drug Vinorelbine and 3 molecular-targeted therapy drugs (erlotinib, gefitinib, and BIBW2992) showed no significant differences between the 2 subgroups (P>0.05).
Discussion
ICB therapy is very effective in the treatment of LUAD and various other cancers (11,25,26). However, the efficacy of ICB therapy is variable in different tumors and patients (11-15). Determining which patients will respond well to ICB therapy is necessary. Due to complex variations in the TME, it is difficult to identify a single factor biomarker that can predict response to multiple treatments. Yin et al., Shen et al., and Chen et al. developed prognostic biomarkers for head and neck squamous cell carcinoma, ovarian cancer, and clear cell renal cell carcinoma based on immune-related genes (27-29). However, there are no relevant studies looking into biomarkers for LUAD. Therefore, it is necessary to develop a biomarker based on the expression of multiple immune-related genes to guide LUAD treatment strategies (30).
WGCNA is an analysis method that can find co-expressed immune-related genes, allowing us to explore potential biomarkers and focus on hub genes. We obtained 50 immune-related hub genes significantly associated with OS through WGCNA and survival analysis and constructed IRGPI based on 15 independent prognostic genes. In LUAD patients, IRGPI demonstrated a good association with prognosis. For example, IRGPI-high patients had poor prognosis, while IRGPI-low patients showed better prognosis. The results were confirmed using TCGA and GEO cohorts, concluding that IRGPI is a valuable immune-related biomarker for LUAD that can be used to predict prognosis and guide treatment decisions.
IRGPI is based on 15 genes (SFTPD, S100A16, FGF2, BIRC5, SEMA4B, ANGPTL4, IL3RA, SHC3, FCN3, TRIM6, LRRK2, HMGB2, RASGEF1B, C7, and PRKCE). Pulmonary surfactant-associated protein (SP-D) encoded by surfactant protein D (SFTPD) shows dual effects of suppressing or enhancing inflammation through binding different receptors (31). SP-D can inhibit the proliferation, invasion, and migration of LUAD cells by interacting with epidermal growth factor receptor (EGFR) (32). Fibroblast growth factor-2 (FGF-2), a member of the FGF family, promotes proliferation, reparation, and migration in a variety of cells (33). The S100 protein family has shown a correlation with various signaling pathways in cancer cells, and proteomics have reported that S100A16 significantly correlated with poor prognosis of LUAD patients (34). Semaphoring 4B (SEMA4B) belongs to the semaphorin protein family, which regulates cell migration, angiogenesis, and immune response. According to the report, SEMA4B may have promoted the expression of matrix metalloproteinase-9 (MMP9) by activating the phosphatidylinositol 3-kinase (PI3K) signaling pathway. An increase in metastasis promotes NSCLC (35). Baculoviral inhibitor of apoptosis repeat-containing 5 (BIRC5, also as known as survivin), an inhibitor of apoptosis-related proteins, is expressed in the growth 2 (G2)/mitosis (M) checkpoint phase. Overexpression of BIRC5 in cancer is conducive to its aberrant progression (36). Alpha subunit of interleukin 3 receptor (IL3RA) is a cell membrane protein produced by activated T lymphocytes and plays a role in immunity and hematopoiesis. RasGEF1, a member of the guanine-nucleotide exchange factor (GEF) family, is induced in macrophages by stimulation of Toll-like receptor (TLR). Repression of RasGEF1B reduces intercellular adhesion molecule 1 (ICAM-1) expression. This plays a role in the immune response by promoting the binding of leukocytes to endotheliocytes and their subsequent migration into tissues (37). Both ficolin-3 (encoded by FCN) and complement component 7 (C7) are involved in the complement system and play key roles in immune-inflammatory responses. In summary, IRGPI fully reflects the progression of LUAD proliferation, invasion, metastasis, angiogenesis, and multiple other tumor development processes. It is widely associated with various aspects of the immune response. As a multifactor and multiperspective biomarker, IRGPI is a better prognostic indicator than traditional biomarkers that can only narrowly reflect a single perspective of the tumor.
Somatic mutations are related to tumor cell sensitivity and anti-chemotherapy mechanisms. These mutations play a major role in predicting an antitumor drug response (38). The mutant gene showing the greatest difference in the 2 groups was TTN. More specifically, the IRGPI-high subgroup showed a greater TTN mutation rate than the IRGPI-low subgroup. One study (39) found that long noncoding RNA (lncRNA) titan-antisense RNA1 (TTN-AS1) transcribed in TTN antisense chain is upregulated in LUAD, and TTN-AS1 binds to microRNA (miR)-142-5p as a competing endogenous RNA (ceRNA) to indirectly upregulate cyclin-dependent kinase 5 (CDK5) expression. Knockdown of TTN-AS1 significantly reduced the proliferation, invasion, and migration of LUAD cells This research suggested that TTN mutations may have been associated with the relatively poor prognosis of the IRGPI-high subgroup and could be a potential target for the treatment of IRGPI-high patients.
TME plays an important role in the ICB therapy response as well as LUAD patient prognosis. Our study revealed that there were significant differences in the infiltration of some immune cells between the 2 subgroups. These differences determined the heterogeneity of the TME and affected patient outcomes. Previous studies have found that both T-cells and NK cells have antitumor effects and are positively correlated with prognosis in cases of high-level infiltration (40,41). The main subtype of macrophages in most tumors is M2, which usually promotes proliferation and invasion that leads to poor prognosis. In contrast, the degree of infiltration of M1 macrophages positively correlates with prognosis (42). Results from our study supported these conclusions, including the proportion of various immune cells and also the location and activity of immune cells affecting immune response and ultimately prognosis. The exploration of deep TME characteristics of the 2 IRGPI subgroups is needed in future studies.
There were significant differences in the C1 and C3 immune subtypes between the 2 groups. The C1 subtype highly expresses angiogenic genes and contains a high tumor proliferation rate. The C3 subtype is characterized by abundant Th17 cells and Th1 cell infiltration, shows mild to moderate tumor proliferation rates, and contains low levels of somatic copy number variation and aneuploidy. In a previous study (16), the C3 subtype was linked to a better prognosis compared to the C1 subtype. This led to the conclusion that prognosis may be better in the IRGPI-low compared to the IRGPI-high subgroup. There were also significant differences observed in the distribution of clinical stages for the 2 subgroups. A higher clinical stage suggested that patients in the IRGPI-high subgroup were more likely to have poor prognosis. The results of these analyses are consistent with the prognostic results of IRGPI and are helpful for physicians to predict the immune type characteristics and disease progression of LUAD patients.
PD-L1 and TMB are 2 classic biomarkers commonly used in clinical practice, with both having a positive correlation with the response of ICB-treated patients (43). In our study, the expression levels of PD-L1 and TMB were significantly higher in the IRGPI-high subgroup, suggesting that patients in the IRGPI-high subgroup would better benefit from ICB therapy. In comparing IRGPI with TIDE and TIS, IRGPI was shown to be an excellent prognostic indicator with better predictability. IRGPI overcame the one-sided reflection of traditional biomarkers for prognosis, making it a promising biomarker for clinical application.
GDSC links complex genomes with drug sensitivity and facilitates the discovery of new biomarkers related to drug response (44). GDSC analysis showed that the IRGPI-high group would have a better response to chemotherapy and higher benefits in response to ICB therapy. The application of ICB therapy in combination with chemotherapy should be considered to improve the poor prognosis of IRGPI-high patients. The combined use of multiple therapies showed better effects in enhancing drug response and delaying drug resistance.
In future clinical application of IRGPI, a large amount of information for LUAD patients, such as reliable prediction of prognosis, TME landscape, estimation of clinical stage before cancer-related checkup, and benefits of multiple therapies can be predicted. This will help to determine the optimal treatment strategy. We are more inclined to recommend a combinational strategy including ICB therapy and chemotherapy for patients with high IRGPI scores. Meanwhile, our study needs further real-world research validation, which is the direction of our follow-up research.
Conclusions
In conclusion, we developed a biomarker with excellent prospects for clinical practice to help guide immunotherapy. IRGPI surpassed the limitations of traditional biomarkers and reduced bias of prognostic models through the use of immune-related hub DEGs, demonstrating powerful prognosis predictability. IRGPI can predict LUAD patient response to ICB therapy and traditional treatments, increasing the value of this biomarker in allowing for individualized treatment regimens.
Acknowledgments
We thank the CIBERSORT and TIDE websites for providing online tools for immune infiltration analysis and immunotherapy predictive efficacy analysis.
Funding: None.
Footnote
Reporting Checklist: The authors have completed the TRIPOD reporting checklist. Available at https://jtd.amegroups.com/article/view/10.21037/jtd-22-494/rc
Conflicts of Interest: All authors have completed the ICMJE uniform disclosure form (available at https://jtd.amegroups.com/article/view/10.21037/jtd-22-494/coif). The authors have no conflicts of interest to declare.
Ethical Statement: The authors are accountable for all aspects of the work in ensuring that questions related to the accuracy or integrity of any part of the work are appropriately investigated and resolved. The study was conducted in accordance with the Declaration of Helsinki (as revised in 2013).
Open Access Statement: This is an Open Access article distributed in accordance with the Creative Commons Attribution-NonCommercial-NoDerivs 4.0 International License (CC BY-NC-ND 4.0), which permits the non-commercial replication and distribution of the article with the strict proviso that no changes or edits are made and the original work is properly cited (including links to both the formal publication through the relevant DOI and the license). See: https://creativecommons.org/licenses/by-nc-nd/4.0/.
References
- Siegel RL, Miller KD, Fuchs HE, et al. Cancer Statistics, 2021. CA Cancer J Clin 2021;71:7-33. [Crossref] [PubMed]
- Hua X, Zhao W, Pesatori AC, et al. Genetic and epigenetic intratumor heterogeneity impacts prognosis of lung adenocarcinoma. Nat Commun 2020;11:2459. [Crossref] [PubMed]
- Miller M, Hanna N. Advances in systemic therapy for non-small cell lung cancer. BMJ 2021;375: [Crossref] [PubMed]
- Girard N, Perol M, Simon G, et al. Treatment strategies for unresectable locally advanced non-small cell lung cancer in the real-life ESME cohort. Lung Cancer 2021;162:119-27. [Crossref] [PubMed]
- Yu HA, Suzawa K, Jordan E, et al. Concurrent Alterations in EGFR-Mutant Lung Cancers Associated with Resistance to EGFR Kinase Inhibitors and Characterization of MTOR as a Mediator of Resistance. Clin Cancer Res 2018;24:3108-18. [Crossref] [PubMed]
- Marusyk A, Janiszewska M, Polyak K. Intratumor Heterogeneity: The Rosetta Stone of Therapy Resistance. Cancer Cell 2020;37:471-84. [Crossref] [PubMed]
- Pardoll DM. The blockade of immune checkpoints in cancer immunotherapy. Nat Rev Cancer 2012;12:252-64. [Crossref] [PubMed]
- Mellman I, Coukos G, Dranoff G. Cancer immunotherapy comes of age. Nature 2011;480:480-9. [Crossref] [PubMed]
- Sharma P, Allison JP. The future of immune checkpoint therapy. Science 2015;348:56-61. [Crossref] [PubMed]
- Morad G, Helmink BA, Sharma P, et al. Hallmarks of response, resistance, and toxicity to immune checkpoint blockade. Cell 2021;184:5309-37. [Crossref] [PubMed]
- Gettinger SN, Horn L, Gandhi L, et al. Overall Survival and Long-Term Safety of Nivolumab (Anti-Programmed Death 1 Antibody, BMS-936558, ONO-4538) in Patients With Previously Treated Advanced Non-Small-Cell Lung Cancer. J Clin Oncol 2015;33:2004-12. [Crossref] [PubMed]
- Herbst RS, Baas P, Kim DW, et al. Pembrolizumab versus docetaxel for previously treated, PD-L1-positive, advanced non-small-cell lung cancer (KEYNOTE-010): a randomised controlled trial. Lancet 2016;387:1540-50. [Crossref] [PubMed]
- Rizvi NA, Mazières J, Planchard D, et al. Activity and safety of nivolumab, an anti-PD-1 immune checkpoint inhibitor, for patients with advanced, refractory squamous non-small-cell lung cancer (CheckMate 063): a phase 2, single-arm trial. Lancet Oncol 2015;16:257-65. [Crossref] [PubMed]
- Garon EB, Rizvi NA, Hui R, et al. Pembrolizumab for the treatment of non-small-cell lung cancer. N Engl J Med 2015;372:2018-28. [Crossref] [PubMed]
- Borghaei H, Paz-Ares L, Horn L, et al. Nivolumab versus Docetaxel in Advanced Nonsquamous Non-Small-Cell Lung Cancer. N Engl J Med 2015;373:1627-39. [Crossref] [PubMed]
- Thorsson V, Gibbs DL, Brown SD, et al. The Immune Landscape of Cancer. Immunity 2018;48:812-830.e14. [Crossref] [PubMed]
- Smyth GK, Ritchie M, Thorne N, et al. limma: Linear Models for Microarray Data. 2010. Available online:
10.1007/0-387-29362-0_23 10.1007/0-387-29362-0_23 - Yu G, Wang LG, Han Y, et al. clusterProfiler: an R package for comparing biological themes among gene clusters. OMICS 2012;16:284-7. [Crossref] [PubMed]
- Langfelder P, Horvath S. WGCNA: an R package for weighted correlation network analysis. BMC Bioinformatics 2008;9:559. [Crossref] [PubMed]
- Mayakonda A, Lin DC, Assenov Y, et al. Maftools: efficient and comprehensive analysis of somatic variants in cancer. Genome Res 2018;28:1747-56. [Crossref] [PubMed]
- Hänzelmann S, Castelo R, Guinney J. GSVA: gene set variation analysis for microarray and RNA-seq data. BMC Bioinformatics 2013;14:7. [Crossref] [PubMed]
- Jiang P, Gu S, Pan D, et al. Signatures of T cell dysfunction and exclusion predict cancer immunotherapy response. Nat Med 2018;24:1550-8. [Crossref] [PubMed]
- Sharma P, Wagner K, Wolchok JD, et al. Novel cancer immunotherapy agents with survival benefit: recent successes and next steps. Nat Rev Cancer 2011;11:805-12. [Crossref] [PubMed]
- Schreiber RD, Old LJ, Smyth MJ. Cancer immunoediting: integrating immunity's roles in cancer suppression and promotion. Science 2011;331:1565-70. [Crossref] [PubMed]
- Brahmer JR, Tykodi SS, Chow LQ, et al. Safety and activity of anti-PD-L1 antibody in patients with advanced cancer. N Engl J Med 2012;366:2455-65. [Crossref] [PubMed]
- Robert C, Thomas L, Bondarenko I, et al. Ipilimumab plus dacarbazine for previously untreated metastatic melanoma. N Engl J Med 2011;364:2517-26. [Crossref] [PubMed]
- Yin X, Wang Z, Wang J, et al. Development of a novel gene signature to predict prognosis and response to PD-1 blockade in clear cell renal cell carcinoma. Oncoimmunology 2021;10:1933332. [Crossref] [PubMed]
- Shen S, Wang G, Zhang R, et al. Development and validation of an immune gene-set based Prognostic signature in ovarian cancer. EBioMedicine 2019;40:318-26. [Crossref] [PubMed]
- Chen Y, Li ZY, Zhou GQ, et al. An Immune-Related Gene Prognostic Index for Head and Neck Squamous Cell Carcinoma. Clin Cancer Res 2021;27:330-41. [Crossref] [PubMed]
- Frederick DT, Piris A, Cogdill AP, et al. BRAF inhibition is associated with enhanced melanoma antigen expression and a more favorable tumor microenvironment in patients with metastatic melanoma. Clin Cancer Res 2013;19:1225-31. [Crossref] [PubMed]
- Gardai SJ, Xiao YQ, Dickinson M, et al. By binding SIRPalpha or calreticulin/CD91, lung collectins act as dual function surveillance molecules to suppress or enhance inflammation. Cell 2003;115:13-23. [Crossref] [PubMed]
- Hasegawa Y, Takahashi M, Ariki S, et al. Surfactant protein D suppresses lung cancer progression by downregulation of epidermal growth factor signaling. Oncogene 2015;34:838-45. [Crossref] [PubMed]
- Bikfalvi A, Klein S, Pintucci G, et al. Biological roles of fibroblast growth factor-2. Endocr Rev 1997;18:26-45. [PubMed]
- Kobayashi M, Nagashio R, Saito K, et al. Prognostic significance of S100A16 subcellular localization in lung adenocarcinoma. Hum Pathol 2018;74:148-55. [Crossref] [PubMed]
- Jian H, Zhao Y, Liu B, et al. SEMA4b inhibits MMP9 to prevent metastasis of non-small cell lung cancer. Tumour Biol 2014;35:11051-6. [Crossref] [PubMed]
- Ambrosini G, Adida C, Altieri DC. A novel anti-apoptosis gene, survivin, expressed in cancer and lymphoma. Nat Med 1997;3:917-21. [Crossref] [PubMed]
- Ng WL, Marinov GK, Liau ES, et al. Inducible RasGEF1B circular RNA is a positive regulator of ICAM-1 in the TLR4/LPS pathway. RNA Biol 2016;13:861-71. [Crossref] [PubMed]
- Kim N, He N, Kim C, et al. Systematic analysis of genotype-specific drug responses in cancer. Int J Cancer 2012;131:2456-64. [Crossref] [PubMed]
- Jia Y, Duan Y, Liu T, et al. LncRNA TTN-AS1 promotes migration, invasion, and epithelial mesenchymal transition of lung adenocarcinoma via sponging miR-142-5p to regulate CDK5. Cell Death Dis 2019;10:573. [Crossref] [PubMed]
- Zhao S, Li C, Ye Y, et al. Expression of Chemerin Correlates With a Favorable Prognosis in Patients With Non-Small Cell Lung Cancer. Laboratory Medicine 2011;42:553-7. [Crossref]
- Huang SM, Wu SP, Liao RQ, et al. P2.03b-043 Peripheral Blood CD45RA+ CCR7+ Naive T Cells Were Correlated with Prognosis in Non-Small Cell Lung Cancer Patients. J Thorac Oncol 2017;12:S961-2. [Crossref]
- Josephs DH, Bax HJ, Karagiannis SN. Tumour-associated macrophage polarisation and re-education with immunotherapy. Front Biosci (Elite Ed) 2015;7:293-308. [PubMed]
- Topalian SL, Hodi FS, Brahmer JR, et al. Safety, activity, and immune correlates of anti-PD-1 antibody in cancer. N Engl J Med 2012;366:2443-54. [Crossref] [PubMed]
- Yang W, Soares J, Greninger P, et al. Genomics of Drug Sensitivity in Cancer (GDSC): a resource for therapeutic biomarker discovery in cancer cells. Nucleic Acids Res 2013;41:D955-61. [Crossref] [PubMed]
(English Language Editor: A. Muylwyk)