Development and validation of a prognostic model related to pyroptosis-related genes for esophageal squamous cell carcinoma using bioinformatics analysis
Introduction
Esophageal cancer (EC) is the 7th most prevalent malignant neoplasm and 6th leading cause of cancer-related death worldwide (1). Pathological types of EC are classified as esophageal squamous cell carcinoma (ESCC) and esophageal adenocarcinoma (EAC), of which ESCC accounts for 90% of all cases of EC and mostly occurs in the Eastern Asian and Eastern and Southern African regions (2,3). The etiology of ESCC is incompletely understood, and may include genetic factors (e.g., mutation), environmental factors (e.g., alcohol and tobacco), viral factors [e.g., papillomavirus (HPV) infection], or the coexistence of these factors (4). When the esophageal mucosa is exposed to repeated damage from mechanical injury or carcinogens, abnormal epithelium cell proliferation occurs, and the abnormal cell will eventually develop into invasive cancer. Owing to the large population and high incidence of ESCC, the number of cases in China makes up more than half of those worldwide (5). Although patients with ESCC are treated according to current standard guidelines, some with locally advanced ESCC have a unsatisfactory overall survival (OS) (6), with the global 5-year OS rate around 15–25% (1,7). Current treatment options for ESCC generally depend on its stage (8-10), with endoscopic resection for mucosal lesions versus esophagectomy for submucosal lesions the primary treatment option for early disease. Extensive studies have recorded that neoadjuvant therapy combined surgery is beneficial in improving the prognosis of locally advanced ESCC (11). Over the past years, technology advancements have comprehensively characterized the genomic landscape in ESCC, and targeted therapy has attracted increased attention (12). To the best of our knowledge, pembrolizumab, ramucirumab, and trastuzumab have been approved by the U.S. Food and Drug Administration (FDA) for the treatment of the advanced EC (13). Despite this, a minority of targeted therapies have prognostic and therapeutic implications for patients with ESCC, and the development of biomarkers and therapeutic targets is required.
Pyroptosis is triggered by caspase-1/4/5/11 and is one type of lytic programmed cell death (14). Differences between pyroptosis and apoptosis in morphology were observed by Zychlinsky et al. in 1992 (15), and Cookson et al. created the term “pyroptosis” after observing macrophages infected with bacteria in 2001 (16). Pyroptosis can cause cell swelling, plasma membrane lysis, and chromatin fragmentation, which lead to osmotic swelling and cell death (17). Moreover, it can cause the release of intracellular pro-inflammatory factors, including IL-18 and IL-1β, into the extracellular space (18). Pyroptosis shows several similar features to apoptosis, including DNA damage, nuclear pyknosis, and TUNEL-positive staining, but with several unique characteristics (19,20). The mechanism of pyroptosis can be summarized into the following three pathways: the canonical inflammasome pathway, the non-canonical inflammasome pathway, and the caspase-3-dependent pyroptotic pathway (21,22). Triggered caspase 1/4/5/11/3 lead to gasdermin D (GSDMD) cleavage into the gasdermin-C domain and gasdermin-D domain, and the gasdermin-D domain then combines with the acidic phospholipids of the membrane to form oligomeric death-inducing pores, called gasdermin pores (23-26). This type of pore in membrane leads to cell swelling, rupture of the membrane, and eventual cell death (27). Pyroptosis is considered a general innate immune response (18). It can defend against intracellular infection, lead to the death of intracellular bacteria through pore-induced intracellular traps (28,29), and eliminate the pathogen protective niche (27). Pyroptosis is also significantly linked to the tumorigenesis and development of various cancers. The NLR family pyrin domain containing 3 (NLRP3), one pyroptosis-related molecule, was significantly down-regulated in hepatocellular carcinoma (HCC) tissue and associated with prognosis of HCC patients (30). According to a published report, the inhibition of caspase-1 interfered with the anticancer activity of euxanthone in HCC cells (31). Dihlmann et al. have demonstrated that a low expression of the interferon-inducible protein AIM2 was found in colorectal cancer (CRC) cells, and the mortality of patients with low AIM2 expression levels was higher than patients with normal levels (32). A study has shown docosahexaenoic acid (DHA) promoted the activation of caspase-1 and gasdermin D in breast cancer cells, suggesting it induces pyroptosis, and the anti-cancer effect of DHA in breast cancer was associated with it (33).
Pyroptosis-related molecules have also been confirmed as potential biomarkers in various kinds of human malignant neoplasms, including cervical cancer, ovarian carcinoma (OC), non-small cell lung cancer (NSCLC), and gastric cancer (GC) (34-37). Ye et al. developed a prognostic model related to pyroptosis for OC for the first time, which demonstrated good OS prediction (38), and while a LUAD prognostic model constructed using five pyroptosis-related genes (PRGs) was able to predict the OS of LUAD patients with moderate to high accuracy (39). To date, several prognostic biomarkers and associated prognostic model have been suggested in ESCC. A prognostic model based on six N6-methyladenosine (m6A) RNA methylation regulator (METTL3, WTAP, IGF2BP3, YTHDF1, HNRNPA2B1 and HNRNPC) was able to predict the OS of ESCC patients (40). Yao et al. developed a prognostic model of ESCC associated with 10 M2 macrophage genes, which also had good predictive power for OS (41). However, there were several limitations for these studies. These prognostic models need to be further validated in several large cohorts and multi-center clinical trials. In addition, further experiments are needed to elucidate the potential mechanisms of these associated genes in the progression of ESCC.
To the best of our knowledge, no prognostic model related to ESCC has been developed. Herein, we performed a bioinformatics analysis to identify the four prognostic PRGs in ESCC. The riskscore based on the PRGs was constructed and validated in a validation set, and the prognostic model related to the riskscore was also constructed and validated. Finally, we explored the potential role of the riskscore in guiding the immunotherapy of ESCC patients. Our data provided a novel prognostic model that can be used to stratify the risk hazards of patients with ESCC, and to offer theoretical evidence for individualized therapy of ESCC patients. We present the following article in accordance with the TRIPOD reporting checklist (available at https://jtd.amegroups.com/article/view/10.21037/jtd-22-948/rc).
Methods
Research design
All analyses performed are shown in Figure 1. Firstly, we selected expression profiles data of PRGs and performed differential analysis to obtain differential PRGs. Gene Ontology (GO)-terms Semantic Similarity Measures (GSSM), GO, Kyoto Encyclopedia of Genes and Genomes (KEGG) analyses and protein-protein interaction (PPI) network construction were performed based on differential PRGs. Next, we obtained the training set and the validation set after randomly grouping the ESCC cases. In the training set, we found four prognostic PRGs by cox regression analysis and constructed the riskscore based on the four prognostic PRGs via Least Absolute Shrinkage and Selection Operator (LASSO) cox regression analysis. Subsequently, the riskscore was validated in the validation set, and the nomogram was established based on the riskscore and other independent prognostic factors. Lastly, the correlation between riskscore and the response to immunotherapy was explored via Tumor Immune Dysfunction and Exclusion (TIDE) algorithm. The study was conducted in accordance with the Declaration of Helsinki (as revised in 2013).
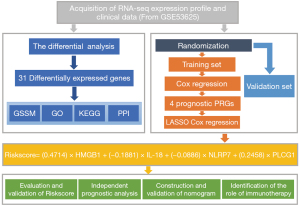
Acquisition and processing of gene expression profile and clinical data
Expression profiles of mRNAs of 179 ESCC patients and matched clinical information were downloaded from the NCBI Gene Expression Omnibus (GEO) database (GSE53625) for differential expression and survival analysis. The clinical data, including eleven clinical variables, is shown in website: https://cdn.amegroups.cn/static/public/jtd-22-948-01.xlsx. The baseline characteristics of patients with ESCC in the GSE53625 was generated using the “tableone” R package, and the RNA-seq dataset was normalized via log2 conversion before further exploration.
Identification of differentially expressed PRGs in ESCC
The PRGs, including fifty-two genes, were obtained from previous studies and the Molecular Signatures Database (MSigDB database, https://www.gsea-msigdb.org/gsea/msigdb/index.jsp), and are listed in Table S1 (27,42-46). The PRGs-expression profiling data were extracted from the GSE53625 dataset, which is shown in website: https://cdn.amegroups.cn/static/public/jtd-22-948-02.xlsx. The “limma” and “reshape2” R packages were used to identify differentially expressed PRGs in ESCC tissues in comparison to the normal tissues, and the PPI network was established by STRING database (https://string-db.org/). The correlation network between the differentially expressed genes (DEGs) was built using the “igraph” R package and was visualized by the “reshape2” R package.
Functional enrichment analysis
GO analysis and KEGG analysis were used to explore the potential biological functions or pathways of DEGs. GO analysis of DEGs, including cellular component (CC) biological process (BP), and molecular function (MF) categories, was performed by the “clusterProfiler”, “org.Hs.eg.db” and “enrichplot” R packages. KEGG analysis of DEGs was conducted using the same R package, and the top 30 pathways or biological function were visualized using the “ggplot2” package.
GSSM analysis
According to a previous study, GSSM analysis by “GOSemSim” R package is a quantitative way to calculate the similarities between gene groups, including semantic similarity of GO terms (47). Therefore, the semantic similarity of GO terms between DEGs was measured using this package. The score of GO semantic similarity can be considered as functional similarity between genes.
Construction of the PRG prognostic model
The outcome of interest in this study was OS of ESCC. To construct an ESCC prognostic model related to PRGs, the GSE53625 dataset was split randomly into a training set and validation set via the “caret” R package, and the group allocation was performed in a 1:1 ratio (Table S2). Cox regression analysis was used to identify the prognostic PRGs in ESCC, and results were visualized by forest plots plotted via the “forestplot” R package. Survival analysis was performed via the “survival” R package, and Kaplan-Meier survival curves were established using the “survminer” R package. Log-rank tests were performed to obtain the P value of the Kaplan-Meier survival curves. LASSO-Cox regression analysis was then performed to construct a riskscore based on the prognostic PRGs, with the riskscore = (0.4714) × HMGB1 + (−0.1881) × IL-18 + (−0.0886) × NLRP7 + (0.2458) × PLCG1. The all patients with ESCC in the GSE53625 dataset were distributed into a high-risk group and low-risk group by the median of the riskscore, and the OS prediction ability of the riskscore was assessed via the Kaplan-Meier curves and the risk curves. When we identified riskscore as an independent prognostic factor, nine other clinical variables were included for analysis, including age, gender, tobacco use, alcohol use, tumor location, grade, tumor node metastasis (TNM) stage, anastomotic leak, pneumonia. To construct the prognostic model, all independent prognostic factors, including the riskscore, were used to construct the nomogram using a multivariable regression model, and for assessing the accuracy of the nomogram, the C-index, Receiver operating characteristic (ROC) curves, and Calibration curves were used. The model has no predictive power when the C-index is equal to 0.5, low accuracy when the C-index is 0.51–0.70, moderate accuracy when the C-index is 0.71–0.90, and high accuracy when the C-index is greater than 0.90 (48). The C-index of the nomogram and that of the TNM stage were compared to evaluate the accuracy of the nomogram further. All results of training set were verified by the validation set or all set.
Exploration of the role of the riskscore in ESCC immunotherapy
The TIDE algorithm (http://tide.dfci.harvard.edu), developed by Jiang et al., is based on integration and modelling of data from 189 human cancer research studies and can be used to evaluate the clinical response to immunotherapy (49). In the present study, the TIDE algorithm was utilized to assess the efficacy of immunotherapy in each ESCC patient. Subsequently, the Wilcoxon test was applied to evaluate the relationship between the riskscore and the efficacy of immunotherapy. A P value of less than 0.05 indicated the difference was significant.
Immune infiltration analysis
The activity or infiltration levels of 19 immunocytes or immune-related function was calculated by the single-sample gene set enrichment analysis (ssGSEA), which was run in the “GSEABase” and “GSVA” R packages (50). The correlation between the riskscore and the infiltration levels of the different immune cells or functions was analyzed via the Wilcoxon test. All results above were considered as significance if the P value were less than 0.05.
Statistical analysis
The R software (version 4.1.0) and various R packages were used for conducting the data analysis and visualizing the data. Statistical significance for all analyses was defined by P<0.05.
Results
Identification of the differentially expressed genes in ESCC
The transcriptome dataset of ESCC was obtained from the GEO databse (GSE53625), which consists of 179 ESCC samples and 179 normal samples. As shown in Figure 2A, the RNA-level expression of 52 PRGs in ESCC tissue and normal tissue were identified. We identified the differentially expressed PRGs via the differential expression analysis between ESCC samples and normal samples, and the results show there were 31 DEGs in ESCC (FDR <0.001) (Figure 2B). Of these, 16 DEGs were up-regulated in ESCC tissues, and the remainder were down-regulated.
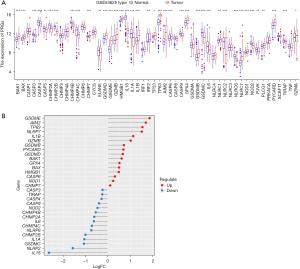
Functional enrichment analysis and the exploration of semantic similarity
To predict the CC, BP, and MF, GO enrichment analysis was performed, and the results showed the pyroptosis DEGs were enriched in positive regulation of cytokine production, interleukin-1 beta production, pyroptosis, inflammasome complex, inflammasome complex, late endosome, phospholipid binding, and cytokine receptor binding (Figure 3A). In addition, KEGG analysis suggested DEGs were primarily involved in the NOD−like receptor signaling pathway and necroptosis (Figure 3B).
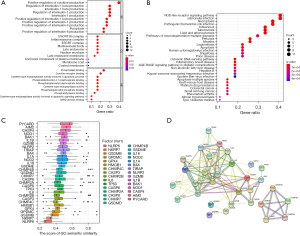
To explore the semantic similarity of GO terms among the differentially expressed PRGs, the “GOSemSim” R package was used. The results suggested PYCARD, AIM2, CASP4, NOD1, and BAK1 had a higher semantic similarity of GO terms, which meant these genes had more interactions with other genes on the pathway and were more likely to be hub genes (Figure 3C). PPI network results of the DEGs are shown in Figure 3D, and the co-expression network of the DEGs is clearly documented in Figure S1.
Construction of a riskscore based on prognostic PRGs
The GSE53625 dataset was split randomly into a training set and validation set, and the characteristics of patients with ESCC in the two sets is shown in Table 1. To determine the PRGs with an impact on the prognosis of ESCC, Cox regression analysis was applied in the training set, and the result showed the expression levels of the four PRGs respectively had significant relations to the OS of ESCC patients (Figure 4A). Kaplan-Meier survival curves suggested the expression of HMGB1 (Figure 4B, P=0.013) and PLCG1 (Figure 4C, P=0.00069) were associated with poorer prognosis, and the expression of IL-18 (Figure 4D P=0.045) and NLRP7 (Figure 4E, P=0.0045) were associated with a better prognosis. The riskscore based on the four prognostic PRGs mentioned above was constructed through the LASSO-Cox regression analysis (Figure 5A,5B), with the riskscore = (0.4714) × HMGB1 + (−0.1881) × IL-18 + (−0.0886) × NLRP7 + (0.2458) × PLCG1. The training set was classified into a low-risk group and a high-risk group according to the median of the riskscore, and the survival analysis of the training set indicated the OS of the former was worse than that of the latter (Figure 5C, P<0.001). Similarly, the Kaplan-Meier survival curves of the validation set showed the low-risk group had a better OS (Figure 5D, P=0.03). In addition, patients from the training set were ordered (from left to right) according to the increasing riskscore, and the results showed the mortality risk increased and survival time decreased with the increase (Figure 5E). In the validation set, the trends of the distribution of survival states and survival time of patients were consistent with the trends in the training set, and the increase of deaths and decrease of survival time were significantly associated with the increased riskscore (Figure 5F). The results of principal component analysis (PCA) indicated ESCC patients could be significantly distinguished into high-risk and low-risk groups by the riskscore based upon the four prognostic PRGs (Figure 5G,5H).
Table 1
Characteristics | All set (N=179) | Training set (N=90) | Validation set (N=89) |
---|---|---|---|
Status, n (%) | |||
Alive | 73 (40.8) | 34 (37.8) | 39 (43.8) |
Death | 106 (59.2) | 56 (62.2) | 50 (56.2) |
Age (years), n (%) | |||
≤65 | 130 (72.6) | 69 (76.7) | 61 (68.5) |
>65 | 49 (27.4) | 21 (23.3) | 28 (31.5) |
Gender, n (%) | |||
Female | 33 (18.4) | 15 (16.7) | 18 (20.2) |
Male | 146 (81.6) | 75 (83.3) | 71 (79.8) |
Tumor location, n (%) | |||
Upper | 20 (11.2) | 13 (14.4) | 7 (7.9) |
Middle | 97 (54.2) | 43 (47.8) | 54 (60.7) |
Lower | 62 (34.6) | 34 (37.8) | 28 (31.5) |
Grade, n (%) | |||
I | 32 (17.9) | 16 (17.8) | 16 (18.0) |
II | 98 (54.7) | 52 (57.8) | 46 (51.7) |
III | 49 (27.4) | 22 (24.4) | 27 (30.3) |
TNM stage, n (%) | |||
I | 10 (5.6) | 5 (5.6) | 5 (5.6) |
II | 77 (43.0) | 41 (45.6) | 36 (40.4) |
III | 92 (51.4) | 44 (48.9) | 48 (53.9) |
T, n (%) | |||
T1 | 12 (6.7) | 7 (7.8) | 5 (5.6) |
T2 | 27 (15.1) | 14 (15.6) | 13 (14.6) |
T3 | 110 (61.5) | 56 (62.2) | 54 (60.7) |
T4 | 30 (16.8) | 13 (14.4) | 17 (19.1) |
N, n (%) | |||
N0 | 83 (46.4) | 42 (46.7) | 41 (46.1) |
N1 | 62 (34.6) | 34 (37.8) | 28 (31.5) |
N2 | 22 (12.3) | 8 (8.9) | 14 (15.7) |
N3 | 12 (6.7) | 6 (6.7) | 6 (6.7) |
Anastomotic leak, n (%) | |||
Yes | 12 (6.7) | 3 (3.3) | 9 (10.1) |
No | 167 (93.3) | 87 (96.7) | 80 (89.9) |
Pneumonia, n (%) | |||
Yes | 15 (8.4) | 4 (4.4) | 11 (12.4) |
No | 164 (91.6) | 86 (95.6) | 78 (87.6) |
Tobacco use, n (%) | |||
Yes | 114 (63.7) | 60 (66.7) | 54 (60.7) |
No | 65 (36.3) | 30 (33.3) | 35 (39.3) |
Alcohol use, n (%) | |||
Yes | 106 (59.2) | 54 (60.0) | 52 (58.4) |
No | 73 (40.8) | 36 (40.0) | 37 (41.6) |
ESCC, esophageal squamous cell carcinoma; TNM, tumor node metastasis.
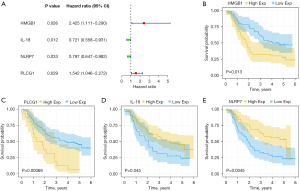
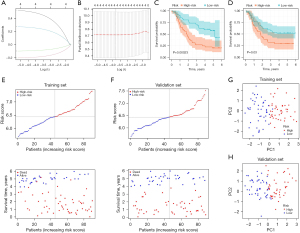
Construction of a prognostic model related to the prognostic PRGs
We extracted total relevant clinical information from the GEO database (GSE53625). Subsequently, univariate (Figure 6A) and multivariate (Figure 6B) Cox regression analysis were applied in the training set, and the results showed the riskscore (P<0.05), TNM stage (P<0.05), and grade (P<0.05) were independent prognostic factors. At the same time, the riskscore, TNM stage, and grade in the training set were used to construct the nomogram for OS prediction (Figure 6C). The time-dependent ROC curves indicated the nomogram based on the three independent prognostic factors had excellent accuracy for the prognostic prediction of ESCC, as the Area under the ROC Curve (AUC) were 0.715, 0.820, and 0.728 for 1-, 3-, and 5-year (Figure 6D). With the aim of assessing the Calibration of the nomogram, Calibration curves predicting 1-, 3-, and 5-year OS were generated, and the results showed the actual OS rates were close to the nomogram-predicted OS rates (Figure 6E), while the C-index for the nomogram (0.705) was better than that for the TNM stage (0.620) (Figure 6F). In the all set (Figure 7A,7B) and the validation set (Figure 7C,7D), the independent prognostic factors were also identified, and the results showed the riskscore (P<0.05), TNM stage (P<0.05), and age (P<0.05) were independent prognostic factors. The nomogram based on independent prognostic factors was also constructed in the all set and is shown in Figure S2A. The AUSs of the nomogram for predicting 1-, 3-, and 5-year OS in the all set were 0.701, 0.752, and 0.675, respectively (Figure 7E), and the Calibration curves for this nomogram also indicated the nomogram had a good prediction ability for OS rates (Figure 7F). In terms of the C-index, the nomogram (C-index =0.676) was also higher than the TNM stage (C-index =0.596) (Figure 7G). The nomogram of the validation set was also constructed (Figure S2B) and the time-dependent ROC curves analysis evaluated its accuracy. The nomogram of the validation set was verified by the ROC curves (Figure 7H), Calibration curves (Figure 7I), and C-index (Figure 7J) and the results demonstrated the ability to predict the OS of ESCC patients was better than the TNM stage. In conclusion, the data above demonstrated the prognostic model related to PRGs had a satisfactory performance for predicting OS of ESCC.
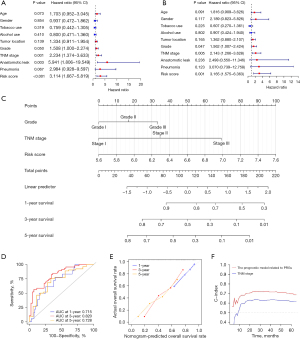
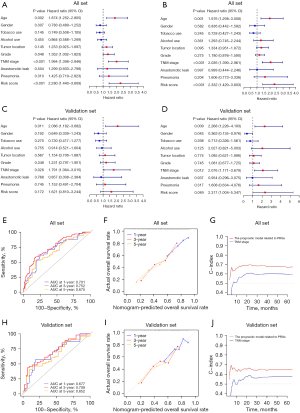
Role of the riskscore based on the PRGs in tumor immunotherapy
Pyroptosis is closely correlated with immunity, and the riskscore is based on the PRGs. Hence, we speculated the riskscore might be associated with tumor immunotherapy, and the relationships between the expression of immunotherapy-related mRNAs (PD-L1, CD23, CTLA4, IDO1, IFNG, IL-2, LAG3) and the riskscore were explored. The findings showed PD-L1 (Figure 8A, P=0.000046) and IL-2 (Figure 8B, P=0.0019) expression levels in the low-risk group were higher than in the high-risk group, and the high-risk group had a higher expression level of CD23 (Figure 8C, P=0.0023). In addition, the TIDE score was calculated using the TIDE web tool and patients were divided into two groups by its median. Patients with low TIDE scores showed better OS (Figure 8D, P=0.021). As described for Figure 8E, the high-risk group had a higher TIDE score, which meant that the effect of immunotherapy was significantly related with the riskscore, and the low-risk group had a better efficacy of immunotherapy (P=0.00034). In addition, patients in the high-risk group were more likely to experience immune evasion (Figure S3, P<0.001). Furthermore, the relationship between the riskscore and immune cells and function were analyzed using the ssGSEA R package, and the findings suggested significant differences were found in 10 kinds of immune cells, including Regulatory T Cells (Treg), tumor-infiltrating lymphocyte (TIL), T helper 2 cells (Th2 cells), T helper 1 cells (Th1 cells), T helper cells, Neutrophils, Neutrophils, Mast cells, Macrophages, Dendritic cells (DCs), and B lymphocytes (B cells), between the high-risk group and low-risk group (Figure 8F, P<0.05). Moreover, the scores of the immune functions in the low-risk group, including Antigen-presenting cell (APC)_co_inhibition, CC chemokine receptor (CCR), Parainflammation, and Type_II_interferons (IFN)_reponse, were higher than those in the high-risk group. Therefore, those immune functions played a greater role in low-risk group.
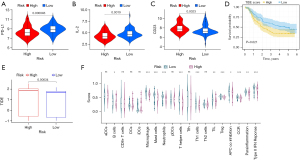
Discussion
Pyroptosis is a form of programmed cell death different from apoptosis, and is regulated by inflammasome and involved in tumorigenesis and progression of various malignancies (51,52). Accumulating evidence suggests pyroptosis has a dual effect on tumor growth and progression. It can promote tumor progression by releasing inflammatory cytokines and contributing to inflammation (53), and has the ability to inhibit tumorigenesis and tumor development caused by triggering tumor cell death (54). It has previously been suggested that a high expression level of Gasdermin B has a significant relation with the lower survival and increased metastasis in patients with breast cancer (55). NALP1 inflammasome has been proven to be a prognostic marker related to pyroptosis (56). However, to date there are no reports on prognostic signatures related to pyroptosis in ESCC, which was the objective of this study.
The first objective of this study was to determine the differentially expressed genes among PRGs in ESCC, and the results showed over half of all PRGs were DEGs in ESCC, indicating there was a relationship between ESCC and pyroptosis (Figure 2B, FDR <0.001). In accordance with our results, previous study have shown GSDME was up-regulated in ESCC tissues and a high expression level meant a better 5-year survival in patients with the disease (57). Additionally, it has been previously suggested that IL-18 expression of ESCC tissues was down-regulated and the deficiency of IL-18 enhanced the progression of ESCC in vivo and in vitro, which also was consistent with our findings (58,59). IL-18 was also one of the factors used to construct the riskscore related to pyroptosis.
The current study found DEGs most enriched in the positive regulation of cytokine production, interleukin-1 beta production, pyroptosis, inflammasome complex, late endosome, phospholipid binding, cytokine receptor binding, response to molecule of bacterial origin, and interleukin-8 production (Figure 3A,3B). A previous study revealed IL-1 beta could promote the migration and invasion of ESCC cells, which was in accord with our results about functional enrichment (60). This study also found the five genes in the DEGs, including PYCARD, AIM2, CASP4, NOD1, and BAK1, had a higher semantic similarity of GO terms (Figure 3C). A literature review concluded PYCARD had dual role in cancers (61) by inhibiting tumors by mediating tumor cell death on the one hand, and promoting tumors by triggering the release of inflammatory cytokines and changing the tumor microenvironment on the other. This mechanism is similar to the dual effect mechanism on tumors related to pyroptosis. Moreover, PYCARD expression was usually silenced by methylation, and its methylation degree of PYCARD correlated with the depth of ESCC invasion (61). It has been previously confirmed that AIM2 expression was up-related in human cutaneous squamous cell carcinoma (cSCC) and its degree became higher with the grade of cSCC increasing (62). Similar histological features are seen in sSCC and ESCC, and previous work has shown this suggests a similar pathogenesis (63). Based on the results of these results and our data, we hypothesized AIM2 might play a role in ESCC.
In this study, we observed a significant relationship between the prognosis of ESCC and the four PRGs; PLCG1, HMGB1, IL-18, and NLRP7 (Figure 4B-4E), and the riskscore was constructed based on these. Kaplan-Meier survival curves and the risk curves of the training set showed the riskscore could predict OS of ESCC patients with great accuracy (Figure 5C,5E). The prognostic role of the riskscore was confirmed in the validation set, the related results of validation set being similar to those of the training set (Figure 5D,5F). Prior study has revealed a signature based on extracellular matrix (ECM) which could also be used to predict the OS of patients with ESCC (64). Micro(mi)RNAs are non-coding RNAs and are significantly associated with malignant initiation and progression (65). A riskscore based on five prognostic miRNA was constructed in ESCC, and the high-risk group also had a worse survival (66). The present study is the first to establish a riskscore based on pyroptosis. We found it had a meaningful effect on the prognosis of ESCC patients and was an independent prognostic factor (Figure 6A,6B, P<0.001). The riskscore and the other independent prognostic factors (TNM stage and grade) were used to construct the prognostic model related to pyroptosis in the training set. (Figure 6C), and the nomogram constructed in the training set could accurately and consistently predict the 1-, 3-, and 5-year OS (Figure 6D,6E). Furthermore, the C-index of the prognostic model we constructed was significantly higher than that of the TNM stage (Figure 6F), and these results were validated in the validation set and all set. Our data also showed the riskscore was an independent prognostic factor in the validation set and all set (Figure 7A-7D). The nomogram related to the riskscore obtained better OS prediction than the TNM stage, evidenced by the C-index of the nomograms being significantly higher than those of the TNM stage (Figure 7G,7J). These findings clearly indicate our prognostic model related to PRGs had a better OS prediction ability because of the prognostic prediction value of the riskscore.
Pyroptosis is mediated by the gasdermin family of genes and is accompanied by immune and inflammatory responses (42). Caspase-1 has an important effect on innate immunity by triggering the release of the pro-inflammatory cytokines (IL-β and IL-18) and activating pyroptosis (18). Previous study has found a deficiency of IL-18 contributes to the impairment of anti-tumor immune response and the immune escape in ESCC (60). The results of functional enrichment also showed significant enrichment for the immune response (Figure 3A,3B), suggesting the riskscore based on PRGs had some relation to immunotherapy in ESCC. We found the expressions of PD-L1 (Figure 8A), IL-2 (Figure 8B), and CD23 (Figure 8C) were significantly associated with the riskscore. PD-1 is mainly expressed on activated T cells and is a significant immune checkpoint (67). It often combines with PD-L1, triggering tumor immune escape (68), and many studies have suggested that PD-L1 could be used to predict the efficacy for PD1/PD-L1 targeted therapy in a variety of tumor types (69). In 2019, an immune checkpoint inhibitor was approved by the FDA for use in the treatment of advanced ESCC patients with positive PD-L1 expression (70). In the present study, PD-L1 expression level in the low-risk group was higher than the high-risk group (Figure 7A) and the high-risk group had a higher TIDE score, which meant immunotherapy showed better efficacy in the low-risk group (Figure 8E). These results indicate the riskscore based on PRGs plays a significant role in predicting the effect of immunotherapy.
In conclusion, we identified a riskscore based on four PRGs (PLCG1, HMGB1, IL-18, and NLRP7), which is an independent prognostic factor in ESCC. The prognostic model related to the riskscore and other independent factors was constructed, and the data demonstrated the prognostic model had a better OS prediction ability than the TNM stage. The riskscore could be taken as a reliable factor to guide the immunotherapy of ESCC. This study has several limitations. Firstly, the lack of an external cohort to validate the prediction accuracy of the prediction model is a major limitation of this study. Secondly, the number of cases included was only 179, which is small compared to other studies. Lastly, the present study is the only investigation into the prognostic model related to PRGs. Therefore, further studies should focus on in vitro and in vivo experiments to confirm our findings.
Acknowledgments
Funding: This research was funded by the National Natural Science Foundation of China (grant number: 82070499), Natural Science Foundation of Fujian Province (grant number: 2020J02055), the Startup Fund for Scientific Research of Fujian Medical University (grant number: 2020QH1087), and the Special financial subsidy project of Fujian Province (2020B020).
Footnote
Reporting Checklist: The authors have completed the TRIPOD reporting checklist. Available at https://jtd.amegroups.com/article/view/10.21037/jtd-22-948/rc
Conflicts of Interest: All authors have completed the ICMJE uniform disclosure form (available at https://jtd.amegroups.com/article/view/10.21037/jtd-22-948/coif). The authors have no conflicts of interest to declare.
Ethical Statement: The authors are accountable for all aspects of the work in ensuring that questions related to the accuracy or integrity of any part of the work are appropriately investigated and resolved. The study was conducted in accordance with the Declaration of Helsinki (as revised in 2013).
Open Access Statement: This is an Open Access article distributed in accordance with the Creative Commons Attribution-NonCommercial-NoDerivs 4.0 International License (CC BY-NC-ND 4.0), which permits the non-commercial replication and distribution of the article with the strict proviso that no changes or edits are made and the original work is properly cited (including links to both the formal publication through the relevant DOI and the license). See: https://creativecommons.org/licenses/by-nc-nd/4.0/.
References
- Bray F, Ferlay J, Soerjomataram I, et al. Global cancer statistics 2018: GLOBOCAN estimates of incidence and mortality worldwide for 36 cancers in 185 countries. CA Cancer J Clin 2018;68:394-424. [Crossref] [PubMed]
- Abnet CC, Arnold M, Wei WQ. Epidemiology of Esophageal Squamous Cell Carcinoma. Gastroenterology 2018;154:360-73. [Crossref] [PubMed]
- Lagergren J, Smyth E, Cunningham D, et al. Oesophageal cancer. Lancet 2017;390:2383-96. [Crossref] [PubMed]
- Ohashi S, Miyamoto S, Kikuchi O, et al. Recent Advances From Basic and Clinical Studies of Esophageal Squamous Cell Carcinoma. Gastroenterology 2015;149:1700-15. [Crossref] [PubMed]
- Du W, Gao A, Herman JG, et al. Methylation of NRN1 is a novel synthetic lethal marker of PI3K-Akt-mTOR and ATR inhibitors in esophageal cancer. Cancer Sci 2021;112:2870-83. [Crossref] [PubMed]
- Leng XF, Daiko H, Han YT, et al. Optimal preoperative neoadjuvant therapy for resectable locally advanced esophageal squamous cell carcinoma. Ann N Y Acad Sci 2020;1482:213-24. [Crossref] [PubMed]
- Pennathur A, Gibson MK, Jobe BA, et al. Oesophageal carcinoma. Lancet 2013;381:400-12. [Crossref] [PubMed]
- Kitagawa Y, Uno T, Oyama T, et al. Esophageal cancer practice guidelines 2017 edited by the Japan esophageal society: part 2. Esophagus 2019;16:25-43.
- Lordick F, Mariette C, Haustermans K, et al. Oesophageal cancer: ESMO Clinical Practice Guidelines for diagnosis, treatment and follow-up. Ann Oncol 2016;27:v50-7. [Crossref] [PubMed]
- Rice TW, Patil DT, Blackstone EH. 8th edition AJCC/UICC staging of cancers of the esophagus and esophagogastric junction: application to clinical practice. Ann Cardiothorac Surg 2017;6:119-30.
- Yang H, Liu H, Chen Y, et al. Neoadjuvant Chemoradiotherapy Followed by Surgery Versus Surgery Alone for Locally Advanced Squamous Cell Carcinoma of the Esophagus (NEOCRTEC5010): A Phase III Multicenter, Randomized, Open-Label Clinical Trial. J Clin Oncol 2018;36:2796-803. [Crossref] [PubMed]
- Fatehi Hassanabad A, Chehade R, Breadner D, et al. Esophageal carcinoma: Towards targeted therapies. Cell Oncol (Dordr) 2020;43:195-209. [Crossref] [PubMed]
- Yang YM, Hong P, Xu WW, et al. Advances in targeted therapy for esophageal cancer. Signal Transduct Target Ther 2020;5:229. [Crossref] [PubMed]
- Fang Y, Tian S, Pan Y, et al. Pyroptosis: A new frontier in cancer. Biomed Pharmacother 2020;121:109595. [Crossref] [PubMed]
- Zychlinsky A, Prevost MC, Sansonetti PJ. Shigella flexneri induces apoptosis in infected macrophages. Nature 1992;358:167-9. [Crossref] [PubMed]
- Cookson BT, Brennan MA. Pro-inflammatory programmed cell death. Trends Microbiol 2001;9:113-4. [Crossref] [PubMed]
- Tsuchiya K, Nakajima S, Hosojima S, et al. Caspase-1 initiates apoptosis in the absence of gasdermin D. Nat Commun 2019;10:2091. [Crossref] [PubMed]
- Jorgensen I, Miao EA. Pyroptotic cell death defends against intracellular pathogens. Immunol Rev 2015;265:130-42. [Crossref] [PubMed]
- Mariathasan S, Weiss DS, Dixit VM, et al. Innate immunity against Francisella tularensis is dependent on the ASC/caspase-1 axis. J Exp Med 2005;202:1043-9. [Crossref] [PubMed]
- Bergsbaken T, Cookson BT. Macrophage activation redirects yersinia-infected host cell death from apoptosis to caspase-1-dependent pyroptosis. PLoS Pathog 2007;3:e161. [Crossref] [PubMed]
- Shojaie L, Iorga A, Dara L. Cell Death in Liver Diseases: A Review. Int J Mol Sci 2020;21:9682. [Crossref] [PubMed]
- Schnappauf O, Chae JJ, Kastner DL, et al. The Pyrin Inflammasome in Health and Disease. Front Immunol 2019;10:1745. [Crossref] [PubMed]
- Kayagaki N, Stowe IB, Lee BL, et al. Caspase-11 cleaves gasdermin D for non-canonical inflammasome signalling. Nature 2015;526:666-71. [Crossref] [PubMed]
- Shi J, Zhao Y, Wang K, et al. Cleavage of GSDMD by inflammatory caspases determines pyroptotic cell death. Nature 2015;526:660-5. [Crossref] [PubMed]
- Frank D, Vince JE. Pyroptosis versus necroptosis: similarities, differences, and crosstalk. Cell Death Differ 2019;26:99-114. [Crossref] [PubMed]
- Shi J, Gao W, Shao F. Pyroptosis: Gasdermin-Mediated Programmed Necrotic Cell Death. Trends Biochem Sci 2017;42:245-54. [Crossref] [PubMed]
- Kovacs SB, Miao EA. Gasdermins: Effectors of Pyroptosis. Trends Cell Biol 2017;27:673-84. [Crossref] [PubMed]
- Miao EA, Leaf IA, Treuting PM, et al. Caspase-1-induced pyroptosis is an innate immune effector mechanism against intracellular bacteria. Nat Immunol 2010;11:1136-42. [Crossref] [PubMed]
- Jorgensen I, Zhang Y, Krantz BA, et al. Pyroptosis triggers pore-induced intracellular traps (PITs) that capture bacteria and lead to their clearance by efferocytosis. J Exp Med 2016;213:2113-28. [Crossref] [PubMed]
- Wei Q, Mu K, Li T, et al. Deregulation of the NLRP3 inflammasome in hepatic parenchymal cells during liver cancer progression. Lab Invest 2014;94:52-62. [Crossref] [PubMed]
- Chu Q, Jiang Y, Zhang W, et al. Pyroptosis is involved in the pathogenesis of human hepatocellular carcinoma. Oncotarget 2016;7:84658-65. [Crossref] [PubMed]
- Dihlmann S, Tao S, Echterdiek F, et al. Lack of Absent in Melanoma 2 (AIM2) expression in tumor cells is closely associated with poor survival in colorectal cancer patients. Int J Cancer 2014;135:2387-96. [Crossref] [PubMed]
- Pizato N, Luzete BC, Kiffer LFMV, et al. Omega-3 docosahexaenoic acid induces pyroptosis cell death in triple-negative breast cancer cells. Sci Rep 2018;8:1952. [Crossref] [PubMed]
- So D, Shin HW, Kim J, et al. Cervical cancer is addicted to SIRT1 disarming the AIM2 antiviral defense. Oncogene 2018;37:5191-204. [Crossref] [PubMed]
- Saeki N, Usui T, Aoyagi K, et al. Distinctive expression and function of four GSDM family genes (GSDMA-D) in normal and malignant upper gastrointestinal epithelium. Genes Chromosomes Cancer 2009;48:261-71. [Crossref] [PubMed]
- Teng JF, Mei QB, Zhou XG, et al. Polyphyllin VI Induces Caspase-1-Mediated Pyroptosis via the Induction of ROS/NF-κB/NLRP3/GSDMD Signal Axis in Non-Small Cell Lung Cancer. Cancers (Basel) 2020;12:193. [Crossref]
- Jiang M, Wang W, Zhang J, et al. Protective Effects and Possible Mechanisms of Actions of Bushen Cuyun Recipe on Diminished Ovarian Reserve Induced by Cyclophosphamide in Rats. Front Pharmacol 2020;11:546. [Crossref] [PubMed]
- Ye Y, Dai Q, Qi H. A novel defined pyroptosis-related gene signature for predicting the prognosis of ovarian cancer. Cell Death Discov 2021;7:71. [Crossref] [PubMed]
- Lin W, Chen Y, Wu B, et al. Identification of the pyroptosis-related prognostic gene signature and the associated regulation axis in lung adenocarcinoma. Cell Death Discov 2021;7:161. [Crossref] [PubMed]
- Guo W, Tan F, Huai Q, et al. Comprehensive Analysis of PD-L1 Expression, Immune Infiltrates, and m6A RNA Methylation Regulators in Esophageal Squamous Cell Carcinoma. Front Immunol 2021;12:669750. [Crossref] [PubMed]
- Yao J, Duan L, Huang X, et al. Development and Validation of a Prognostic Gene Signature Correlated With M2 Macrophage Infiltration in Esophageal Squamous Cell Carcinoma. Front Oncol 2021;11:769727. [Crossref] [PubMed]
- Xia X, Wang X, Cheng Z, et al. The role of pyroptosis in cancer: pro-cancer or pro-"host"? Cell Death Dis 2019;10:650. [Crossref] [PubMed]
- Yu P, Zhang X, Liu N, et al. Pyroptosis: mechanisms and diseases. Signal Transduct Target Ther 2021;6:128. [Crossref] [PubMed]
- Karki R, Kanneganti TD. Diverging inflammasome signals in tumorigenesis and potential targeting. Nat Rev Cancer 2019;19:197-214. [Crossref] [PubMed]
- Wang B, Yin Q. AIM2 inflammasome activation and regulation: A structural perspective. J Struct Biol 2017;200:279-82. [Crossref] [PubMed]
- Latz E, Xiao TS, Stutz A. Activation and regulation of the inflammasomes. Nat Rev Immunol 2013;13:397-411. [Crossref] [PubMed]
- Yu G, Li F, Qin Y, et al. GOSemSim: an R package for measuring semantic similarity among GO terms and gene products. Bioinformatics 2010;26:976-8. [Crossref] [PubMed]
- Chen J, Wang A, Ji J, et al. An Innovative Prognostic Model Based on Four Genes in Asian Patient with Gastric Cancer. Cancer Res Treat 2021;53:148-61. [Crossref] [PubMed]
- Jiang P, Gu S, Pan D, et al. Signatures of T cell dysfunction and exclusion predict cancer immunotherapy response. Nat Med 2018;24:1550-8. [Crossref] [PubMed]
- Hänzelmann S, Castelo R, Guinney J. GSVA: gene set variation analysis for microarray and RNA-seq data. BMC Bioinformatics 2013;14:7. [Crossref] [PubMed]
- Yu J, Li S, Qi J, et al. Cleavage of GSDME by caspase-3 determines lobaplatin-induced pyroptosis in colon cancer cells. Cell Death Dis 2019;10:193. [Crossref] [PubMed]
- Li Z, Xu H, Liu X, et al. GDF11 inhibits cardiomyocyte pyroptosis and exerts cardioprotection in acute myocardial infarction mice by upregulation of transcription factor HOXA3. Cell Death Dis 2020;11:917. [Crossref] [PubMed]
- Thi HTH, Hong S. Inflammasome as a Therapeutic Target for Cancer Prevention and Treatment. J Cancer Prev 2017;22:62-73. [Crossref] [PubMed]
- Nagarajan K, Soundarapandian K, Thorne RF, et al. Activation of Pyroptotic Cell Death Pathways in Cancer: An Alternative Therapeutic Approach. Transl Oncol 2019;12:925-31. [Crossref] [PubMed]
- Hergueta-Redondo M, Sarrió D, Molina-Crespo Á, et al. Gasdermin-B promotes invasion and metastasis in breast cancer cells. PLoS One 2014;9:e90099. [Crossref] [PubMed]
- Chen C, Wang B, Sun J, et al. DAC can restore expression of NALP1 to suppress tumor growth in colon cancer. Cell Death Dis 2015;6:e1602. [Crossref] [PubMed]
- Wu M, Wang Y, Yang D, et al. A PLK1 kinase inhibitor enhances the chemosensitivity of cisplatin by inducing pyroptosis in oesophageal squamous cell carcinoma. EBioMedicine 2019;41:244-55. [Crossref] [PubMed]
- Li J, Qiu G, Fang B, et al. Deficiency of IL-18 Aggravates Esophageal Carcinoma Through Inhibiting IFN-γ Production by CD8+T Cells and NK Cells. Inflammation 2018;41:667-76. [Crossref] [PubMed]
- Xu X, Song C, Chen Z, et al. Downregulation of HuR Inhibits the Progression of Esophageal Cancer through Interleukin-18. Cancer Res Treat 2018;50:71-87. [Crossref] [PubMed]
- Zhou J, Zheng S, Liu T, et al. IL-1β from M2 macrophages promotes migration and invasion of ESCC cells enhancing epithelial-mesenchymal transition and activating NF-κB signaling pathway. J Cell Biochem 2018;119:7040-52. [Crossref] [PubMed]
- Protti MP, De Monte L. Dual Role of Inflammasome Adaptor ASC in Cancer. Front Cell Dev Biol 2020;8:40. [Crossref] [PubMed]
- Lugrin J, Martinon F. The AIM2 inflammasome: Sensor of pathogens and cellular perturbations. Immunol Rev 2018;281:99-114. [Crossref] [PubMed]
- Bhardwaj P, Madan K, Thareja S, et al. Comparative redox status in alcoholic liver disease and nonalcoholic fatty liver disease. Hepatol Int 2008;2:202-8. [Crossref] [PubMed]
- Zhang H, Shi Q, Yang Z, et al. An Extracellular Matrix-Based Signature Associated With Immune Microenvironment Predicts the Prognosis and Therapeutic Responses of Patients With Oesophageal Squamous Cell Carcinoma. Front Mol Biosci 2021;8:598427. [Crossref] [PubMed]
- Konno M, Koseki J, Asai A, et al. Distinct methylation levels of mature microRNAs in gastrointestinal cancers. Nat Commun 2019;10:3888. [Crossref] [PubMed]
- Yu J, Zhu M, Lv M, et al. Characterization of a five-microRNA signature as a prognostic biomarker for esophageal squamous cell carcinoma. Sci Rep 2019;9:19847. [Crossref] [PubMed]
- Yang R, Sun L, Li CF, et al. Galectin-9 interacts with PD-1 and TIM-3 to regulate T cell death and is a target for cancer immunotherapy. Nat Commun 2021;12:832. [Crossref] [PubMed]
- Ren D, Hua Y, Yu B, et al. Predictive biomarkers and mechanisms underlying resistance to PD1/PD-L1 blockade cancer immunotherapy. Mol Cancer 2020;19:19. [Crossref] [PubMed]
- Siu LL, Even C, Mesía R, et al. Safety and Efficacy of Durvalumab With or Without Tremelimumab in Patients With PD-L1-Low/Negative Recurrent or Metastatic HNSCC: The Phase 2 CONDOR Randomized Clinical Trial. JAMA Oncol 2019;5:195-203. [Crossref] [PubMed]
- Food, Administration D. FDA approves pembrolizumab for advanced esophageal squamous cell cancer. Available online: https://www.fda.gov/drugs/resources-information-approved-drugs/fda-approves-pembrolizumab-advanced-esophageal-squamous-cell-cancer.
(English Language Editor: B. Draper)