Analysis of susceptibility genes and myocardial infarction risk correlation of ischemic cardiomyopathy based on bioinformatics
Introduction
Ischemic cardiomyopathy (ICM) is an important cause of heart failure (HF). According to the National Institutes of Health, ICM is caused by an interaction of genetic and environmental factors (1). To date, a number of studies have explored ICM from different aspects (2-4). Long non-coding RNA (lncRNA) are a class of non-coding RNA whose length is more than 200 nt, and are an important part of all non-coding transcripts. The lncRNAs are involved in many important biological processes and are also associated with the occurrence of many diseases (5). Recent studies (6-8) have shown that lncRNA plays an important role in cardiovascular diseases, including ICM. The lncRNA can play a competitive endogenous role. Competing endogenous RNAs (Hub genes) play a role in regulating messenger RNA (mRNA) expression by competing with mRNA microRNAs (miRNAs). In this study, samples were screened from the Gene Expression Omnibus (GEO) (9). Through bioinformatics methods, related genes of ICM pathogenesis were analyzed and a differentially expressed lncRNA-related Hub gene regulatory network was constructed to explore potential targets for ICM treatment.
Ischemic cardiomyopathy (ICM) is the most common type of heart disease. It is a pathological condition resulting from reduced blood circulation and supply to the heart, reduced oxygen supply to the heart, and dysregulated energy metabolism of cardiomyocytes. In severe cases, ICM may lead to massive death of cardiomyocytes. ICM can cause acute myocardial infarction (AMI), fatal arrhythmias, angina pectoris, or some chronic sequelae, and can lead to HF and sudden death. ICM is a type of end-stage coronary atherosclerosis disease. Long-term oxygen supply and oxygen demand imbalance leads to cardiomyocyte apoptosis, necrosis, cardiac fibrosis, scar tissue formation, leading to poor prognosis. For a long time, because cardiomyocyte necrosis is an irreversible pathological process, ICM lacks effective treatment means. Although heart transplantation can replace the damaged heart, it is not widely carried out due to the limitation of donor and immune rejection. In the past few years, research has focused on elucidating the molecular mechanisms of the relevant protein-coding genes and miRNAs. As the technology developed, an lncRNA containing more than 200 nucleotides was discovered. This newly discovered RNA proved to play an important role in a wide range of biological processes.
Also known as ischemic heart disease (IHD), ICM is a common cause of HF, which places a heavy burden on families and communities. The pathogenesis of ICM is still not fully understood. Several studies (10,11) have shown that inflammation, apoptosis, myocardial fibrosis, and other pathological changes play an important role in the occurrence and development of ICM. We present the following article in accordance with the STREGA reporting checklist (available at https://jtd.amegroups.com/article/view/10.21037/jtd-22-1060/rc).
Methods
Data acquisition
Expression profile data were obtained from the National Center for Biotechnology Information (NCBI) GEO (http://www.ncbi.nlm.nih.gov/geo/), using the data set serial number for GSE42955 (species: Homo sapiens). Some 12 ICM samples and 5 “Normal heart” samples were screened. All samples were detected by GPL6244 (Hugene-1_0-ST) Affymetrix Human GeneChip 1.0 ST Array [transcript (gene) version] platform (Thermo Fisher Scientific, Waltham, MA, USA). The study was conducted in accordance with the Declaration of Helsinki (as revised in 2013).
Data preprocessing and lncRNA reannotation
The GEO database was used to download gene expression profile data in CEL format, and the robust multiarray average (RMA) algorithm of the R software package (The R Foundation for Statistical Computing, Vienna, Austria) was used for background correction, standardization, and summary. The chip platform was used for re-annotation of the annotation file. Finally, the probe set of lncRNA and mRNA was obtained. The unmatched probe was removed by matching probe number and mRNA/lncRNA (gene symbol) one by one. For different probes mapped to the same gene, the mean values of different probes were taken as the final expression value of the particular mRNA/lncRNA.
Differential mRNA and differential lncRNA screening
The Limma package of R language was used to analyze mRNA and lncRNA differences between ICM samples and normal samples using the classical Bayesian method. All mRNA/lncRNA were tested and corresponding P values were obtained. The mRNA/lncRNA with P<0.05 were regarded as differential mRNA/lncRNA.
Functional enrichment and pathway analysis of differentially expressed mRNA
The enrichment analysis tools used included the Database for Annotation, Visualization, and Integrated Discovery (DAVID; https://david-d.ncifcrf.gov/) biological processes, the Gene Ontology database (GO; http://geneontology.org/), and the Kyoto Encyclopedia of Genes and Genomes (KEGG; https://www.kegg.jp/). The threshold for significance was set at P<0.05 and an enrichment number of at least 3 was considered a significant accumulation.
Protein-protein interaction (PPI) network construction and module analysis
All differentially expressed genes (DEGs) were input into STRING (https://string-db.org/) online platform, combined score >0.4 was set, and then the calculated results were imported into Cytoscape-v 3.9.1 to construct PPI network. These interactions include physical and functional associations, with data mainly derived from computational prediction, high-throughput experiments, automated text mining, and co-expression networks. The line between two points in the PPI network diagram represents a direct relationship between two genes, and the more protein points connected by a protein, the more important the role of this protein in the whole network. MCODE plug-in was used to analyze the most closely connected gene clusters in PPI network, and CytoHubba plug-in was used to screen out the top 10 genes with the highest connectivity using MCC, Degree and MNC algorithms.
Prediction of co-expression relationship between lncRNA and mRNA
Pearson’s correlation coefficient of each differential mRNA and lncRNA was calculated by one-by-one matched mRNA and lncRNA data, and correlation testing was conducted to screen the relationship pairs between mRNA and lncRNA with R>0.6 and P<0.05. These mRNAs and lncRNA had a synergistic expression relationship.
LncRNA function prediction
For each predicted target factor of lncRNA, the r-package clusterProfiler was used for GO biological process and KEGG pathway enrichment analysis, and the function of lncRNA was indirectly predicted through enrichment analysis results. The Benjamini-Hochberg method was used to correct P values, and P<0.05 and an enrichment number of at least 5 were considered as significant enrichment results.
MiRNA prediction and Hub gene network construction
MiRWalk 2.0 (http://mirwalk.umm.uni-heidelberg.de/) was used for the differential co-expressed mRNAs, and the miRNA prediction results, miRDB (http://www.mirdb.org/), miRMap (https://mirmap.ezlab.org/), RNA22 (https://cm.jefferson.edu/rna22/), and TargetScan (https://www.targetscan.org/vert_80/) were integrated. It was considered that the miRNAs appearing in the prediction results of the 6 databases were the miRNAs regulating target genes. For lncRNAs co-expressed with differential mRNAs, 2 tools were used to predict targeted miRNAs: miRNA-lncRNA pairs with Score ≥140 and Energy ≤−20 were screened by using miRanDa (V3.3a; https://bioweb.pasteur.fr/packages/pack@miRanda@3.3a/) software. The online database starBase (V2.0; https://starbase.sysu.edu.cn/starbase2/) was used to predict the miRNA-lncRNA relationship pair, and the intersection of the 2 prediction results was taken as the final miRNA-lncRNA relationship pair. By integrating differential mRNA-miRNA and differential lncRNA-miRNA relationship pairs, first, the miRNA-lncRNA mRNA correlation regulated by the same miRNA was screened, and the co-expression relationship between the combined mRNA and lncRNA (correlation coefficient >0.6). The miRNA-lncRNA-mRNA relationship pairs were further screened, and Cytoscape software (https://cytoscape.org/) was used to construct the network, namely Hub gene network. We believe that co-expressed lncRNAs and mRNA regulated by the same miRNA in the Hub gene network are Hub genes of each other.
Statistical analysis
The software SPSS 23.0 (IBM Corp., Armonk, NY, USA) was used; χ2 test was used for inter-group comparison; mean ± standard deviation was used for measurement data; independent sample t-test was used for inter-group comparison.
Results
Basic information statistics of DEGs and WGCNA analysis
A total of 18,392 mRNA and 1,384 lncRNA were annotated by chip platform annotation files. The Limma package was used to analyze mRNA and lncRNA differences between ICM samples and normal samples, respectively. Based on the differential mRNA and differential lncRNA obtained by screening, bidirectional hierarchical clustering heat maps were made respectively. As shown in Figure 1, differential mRNA and differential lncRNA could completely separate the samples according to pre-grouping, indicating that the changes of these mRNAs and lncRNAs were related to the occurrence of ICM. By analyzing WGCNA PPI network connection in most closely gene cluster, using CytoHubba plug-ins using MCC, Degree and newsun focus algorithms selected link Degree of the top 10 genes, then joint centiscape plug-in degree, betweenness, eigenvector, closeness four parameters and so-called objective P value and log2[fold change (FC)] value were comprehensively analyzed to screen the core genes (Figure 1).
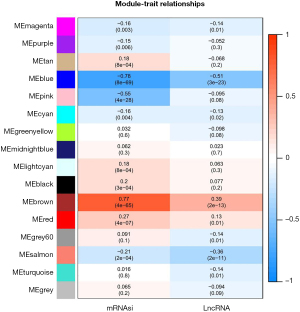
Functional enrichment and pathway analysis of differential mRNA
The GO functional enrichment analysis and KEGG pathway enrichment analysis were performed for up-regulated or down-regulated mRNAs obtained by difference analysis. Up-regulated mRNA was significantly enriched in the GO biological process and KEGG pathway, and down-regulated mRNA was significantly enriched in the GO biological process and KEGG pathway. As shown in Figure 2, upregulated mRNA was significantly enriched in endoplasmic reticulum stress (protein folding and unfolded protein reactions), extracellular matrix tissues, collagen catabolism, and other biological processes, as well as metabolic pathways, oxidative phosphorylation, extracellular matrix receptor interactions, and other pathways, in order of enrichment number. Down-regulated mRNAs were significantly enriched in biological processes such as inflammatory response and immune response, as well as immune response signaling pathways (Figure 2).
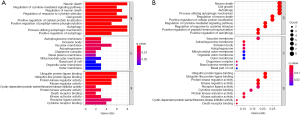
Prediction of co-expression relationship between lncRNA and mRNA
A total of 3,876 synergistically expressed mRNA-lncRNA pairs were screened, including 1,614 mRNAs and 48 lncRNAs. Table 1 displays the top 7 pairs of correlation coefficients from large to small.
Table 1
Gene symbol | Full name | Function |
---|---|---|
CEP55 | Centrosomal protein 55 | Plays a role in mitotic exit and cytokinesis, recruits PDCD6IP and TSG101 to midbody during cytokinesis |
NMU | Neuromedin U | Stimulates muscle contractions of specific regions of the gastrointestinal tract. In humans, NMU stimulates contractions of the ileum and urinary bladder |
CAV1 | Caveolin 1 | Form a stable heterooligomeric complex with CAV2 that targets to rafts and drives caveolae formation. Mediates the recruitment of CAVIN proteins (CAVIN1/2/3/4) to the caveolae |
SEMA6A | Semaphorin 6A | Cell surface receptor for PLXNA2 that plays an important role in cell-cell signaling |
Transcriptional repressor involved in developmental processes | ||
TBX3 | T-box transcription factor 3 | Probably plays a role in limb pattern formation. Acts as a negative regulator of PML function in cellular senescence |
Incorporated into fibronectin-containing matrix fibers | ||
FBLN1 | Fibulin 1 | May play a role in cell adhesion and migration along protein fibers within the ECM |
SYNM | Synemin | Type-VI IF which anchors the extracellular matrix to the cytoskeleton via F-actin |
Tryptase is the major neutral protease present in mast cells and is secreted upon the coupled activation-degranulation response of this cell type. May play a role in innate immunity |
mRNA, messenger RNA; lncRNA, long non-coding RNA; PML, promyelocytic leukemia protein; ECM, extracellular matrix; IF, intermediate filament.
LncRNA function prediction
According to the predicted mRNA-lncRNA synergistic pair, mRNA was used as the target gene of the corresponding lncRNA, and GO biological process and KEGG pathway enrichment analysis were conducted to predict the function of the corresponding lncRNA. The GO biological process and KEGG pathway enrichment results of each lncRNA ranked among the top 5 items (ranked by P value).
MiRNA prediction and Hub gene network construction
The Hub gene network is shown in Figure 3, which contains 34 groups of miRNA lncRNA-mRNA regulated correlates, indirectly inhibiting the expression of multiple mRNAs, and thus indirectly regulating the up-regulation of multiple mRNAs (Figure 3).
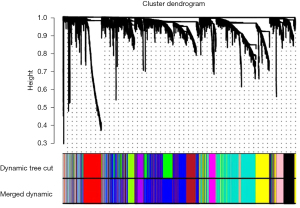
Principal component analysis (PCA)
PCA analysis was performed on these complex transcriptome data to make gene datasets with variables more clearly and unambiguously represented in three-dimensional (3D) space. Panel A shows all the key gene sets related to disease, panel B shows part of the gene sets related to immune infiltration, and panel C shows the key gene sets with prognostic risk assessment value after overlapping screening of the former two (Figure 4).
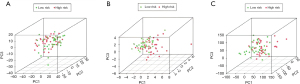
Discussion
As type of end-stage coronary atherosclerosis, in ICM, long-term imbalance between oxygen supply and demand leads to myocardial cell apoptosis and necrosis, cardiac fibrosis, and scar tissue formation, resulting in poor prognosis. For a prolonged period, ICM has lacked effective treatment because the necrosis of myocardial cells is an irreversible pathological process (12-15). Although heart transplantation can replace the damaged heart, it is not widely carried out due to the limitations of donor availability and immune rejection (16). In the past few years, research has focused on elucidating the molecular mechanisms of related protein-coding genes and miRNAs (17-19). With the development of technology, a lncRNA containing more than 200 nucleotides was discovered. This newly discovered RNA was shown to play an important role in a wide range of biological processes (20). The lncRNAs play an important regulatory role in the occurrence and development of diseases from cancer to cardiovascular diseases (21). Mounting studies (22-25) have shown that lncRNAs can target miRNAs to play their function as Hub genes, that is, lncRNAs can affect the expression of protein-coding genes by competitively binding to miRNAs, thus reducing the degradation of protein-coding genes.
The condition of ICM is a serious disease that harms human health (26). It is of great clinical significance to study the pathogenesis and new treatment of this disease (27). This study included a GSE79962 chip dataset downloaded from the GEO database. In addition to a complement system, KEGG enrichment analysis in this study also found that DEGs were enriched in the advanced glycation end (AGE)-receptor for AGE (RAGE) signaling pathway. AGE products are a group of heterogeneous proteins produced by the non-enzymatic reaction of reducing sugars with the amino groups of proteins, fats, and amino acids, with four specific receptors (RAGE) (28). Activation of the AGE-RAGE signaling pathway can promote the generation of inflammatory mediators, reactive oxygen species, and activate nuclear factor-κB (NF-κB) (29). The level of RAGE in serum correlated with the severity and prognosis of heart failure. Therefore, regulation of the AGE-RAGE signaling pathway may be an important method for the treatment of ICM. In this study, it was shown that calcitriol can reduce the expression of the key gene FCGR3A. Previous studies (30-32) have shown that calcitriol can reduce the expression level of RAGE in cardiomyocytes, reduce the accumulation of AGE in the aortic wall of diabetic rats, reduce the expression level of AGE-induced interleukin 6 (IL-6), and inhibit the activity of NF-κB.
In this study, through bioinformatics, differential expression of mRNA of ICM functional annotation (GO and KEGG) pathway analysis, it was shown that there was significant enrichment in fibrosis and endoplasmic reticulum stress pathways, and that these pathways prompt the pathogenesis of ICM (33). In addition, we also constructed differentially expressed lncRNA-related Hub gene networks in ICM. The lncRNAs are extensively involved in the regulation of various pathophysiological processes; H19 is an important member of this family and is closely related to ICM. Some studies (34,35) have found that H19 was present in the left ventricular tissue biopsy of patients with heart failure, and the content of H19 was more abundant in the vascular wall. It has also been detected in the heart tissue of a mouse cardiac hypertrophy model; its expression is up-regulated in the pathological state, and can inhibit cardiac hypertrophy.
This study was mainly based on bioinformatics analysis without experimental verification. Due to the complexity of the organism and the limitation of each database, the interpretation of some data may be insufficient. Through bioinformatics methods, we found that the onset of ICM was closely related to endoplasmic reticulum stress and the occurrence of fibrosis, and the related Hub gene regulatory network is of certain value for the diagnosis, treatment, and prognosis evaluation of ICM, which is conducive to further research on the occurrence and development mechanisms of ICM.
Acknowledgments
Funding: None.
Footnote
Reporting Checklist: The authors have completed the STREGA reporting checklist. Available at https://jtd.amegroups.com/article/view/10.21037/jtd-22-1060/rc
Conflicts of Interest: All authors have completed the ICMJE uniform disclosure form (available at https://jtd.amegroups.com/article/view/10.21037/jtd-22-1060/coif). The authors have no conflicts of interest to declare.
Ethical Statement: The authors are accountable for all aspects of the work in ensuring that questions related to the accuracy or integrity of any part of the work are appropriately investigated and resolved. The study was conducted in accordance with the Declaration of Helsinki (as revised in 2013).
Open Access Statement: This is an Open Access article distributed in accordance with the Creative Commons Attribution-NonCommercial-NoDerivs 4.0 International License (CC BY-NC-ND 4.0), which permits the non-commercial replication and distribution of the article with the strict proviso that no changes or edits are made and the original work is properly cited (including links to both the formal publication through the relevant DOI and the license). See: https://creativecommons.org/licenses/by-nc-nd/4.0/.
References
- Amoni M, Dries E, Ingelaere S, et al. Ventricular Arrhythmias in Ischemic Cardiomyopathy-New Avenues for Mechanism-Guided Treatment. Cells 2021;10:2629. [Crossref] [PubMed]
- Bansal SS, Ismahil MA, Goel M, et al. Dysfunctional and Proinflammatory Regulatory T-Lymphocytes Are Essential for Adverse Cardiac Remodeling in Ischemic Cardiomyopathy. Circulation 2019;139:206-21. [Crossref] [PubMed]
- Divoky L, Maran A, Ramu B. Gender Differences in Ischemic Cardiomyopathy. Curr Atheroscler Rep 2018;20:50. [Crossref] [PubMed]
- Lu YW, Wang DZ. Non-coding RNA in Ischemic and Non-ischemic Cardiomyopathy. Curr Cardiol Rep 2018;20:115. [Crossref] [PubMed]
- Howlett JG, Stebbins A, Petrie MC, et al. CABG Improves Outcomes in Patients With Ischemic Cardiomyopathy: 10-Year Follow-Up of the STICH Trial. JACC Heart Fail 2019;7:878-87. [Crossref] [PubMed]
- Richardson TD, Kanagasundram AN, Stevenson WG. Epicardial Ablation of Ventricular Tachycardia in Ischemic Cardiomyopathy. Card Electrophysiol Clin 2020;12:313-9. [Crossref] [PubMed]
- Neidhardt S, Garbade J, Emrich F, et al. Ischemic Cardiomyopathy Affects the Thioredoxin System in the Human Myocardium. J Card Fail 2019;25:204-12. [Crossref] [PubMed]
- Wu L, Zheng Y, Ruan X, et al. Long-chain noncoding ribonucleic acids affect the survival and prognosis of patients with esophageal adenocarcinoma through the autophagy pathway: construction of a prognostic model. Anticancer Drugs 2022;33:e590-603. [Crossref] [PubMed]
- Goldstein JA, Dixon SR. Mechanically supported PCI for ischemic cardiomyopathy reawakening of hibernating myocardium. Catheter Cardiovasc Interv 2020;96:771-2. [Crossref] [PubMed]
- Razeghian-Jahromi I, Matta AG, Canitrot R, et al. Surfing the clinical trials of mesenchymal stem cell therapy in ischemic cardiomyopathy. Stem Cell Res Ther 2021;12:361. [Crossref] [PubMed]
- Shipulin VM, Pryakhin AS, Andreev SL, et al. Results of reconstructive interventions in patients with ischemic cardiomyopathy. Asian Cardiovasc Thorac Ann 2021;29:508-17. [Crossref] [PubMed]
- Keil L, Chevalier C, Kirchhof P, et al. CMR-Based Risk Stratification of Sudden Cardiac Death and Use of Implantable Cardioverter-Defibrillator in Non-Ischemic Cardiomyopathy. Int J Mol Sci 2021;22:7115. [Crossref] [PubMed]
- Beanlands RSB, Wiefels C. PET Flow Quantification: An Arbitrator in Patients With Ischemic Cardiomyopathy?: The Jury Is Still Out. JACC Cardiovasc Imaging 2021;14:465-7. [Crossref] [PubMed]
- Wang X, Costello BT, Papapostolou S, et al. Differentiating Nonischemic Dilated Cardiomyopathy With Incidental Infarction From Ischemic Cardiomyopathy by Geometric Indices Derived From Cardiovascular Magnetic Resonance. J Thorac Imaging 2021;36:248-53. [Crossref] [PubMed]
- Al-Khatib SM, Stevenson WG. Management of Ventricular Arrhythmias and Sudden Cardiac Death Risk Related to Ischemic and Nonischemic Cardiomyopathy. JAMA Cardiol 2018;3:541-2. [Crossref] [PubMed]
- Patel H, Prejean S, Iskandrian AE, et al. Myocardial perfusion imaging in non-ischemic cardiomyopathy. J Nucl Cardiol 2019;26:1028-32. [Crossref] [PubMed]
- Chen C, Tian J, He Z, et al. Identified Three Interferon Induced Proteins as Novel Biomarkers of Human Ischemic Cardiomyopathy. Int J Mol Sci 2021;22:13116. [Crossref] [PubMed]
- Peet C, Ivetic A, Bromage DI, et al. Cardiac monocytes and macrophages after myocardial infarction. Cardiovasc Res 2020;116:1101-12. [Crossref] [PubMed]
- Aydin S, Ugur K, Aydin S, et al. Biomarkers in acute myocardial infarction: current perspectives. Vasc Health Risk Manag 2019;15:1-10. [Crossref] [PubMed]
- Berezin AE, Berezin AA. Adverse Cardiac Remodelling after Acute Myocardial Infarction: Old and New Biomarkers. Dis Markers 2020;2020:1215802. [Crossref] [PubMed]
- Dzubur A, Gacic E, Mekic M. Comparison of Patients with Acute Myocardial Infarction According to Age. Med Arch 2019;73:23-7. [Crossref] [PubMed]
- Zhang Y, Cao H, Jiang P, et al. Cardiac rehabilitation in acute myocardial infarction patients after percutaneous coronary intervention: A community-based study. Medicine (Baltimore) 2018;97:e9785. [Crossref] [PubMed]
- Occhipinti G, Bucciarelli-Ducci C, Capodanno D. Diagnostic pathways in myocardial infarction with non-obstructive coronary artery disease (MINOCA). Eur Heart J Acute Cardiovasc Care 2021;10:813-22. [Crossref] [PubMed]
- Wu L, Zhong Y, Yu X, et al. Selective poly adenylation predicts the efficacy of immunotherapy in patients with lung adenocarcinoma by multiple omics research. Anticancer Drugs 2022; Epub ahead of print. [Crossref] [PubMed]
- Arora S, Stouffer GA, Kucharska-Newton AM, et al. Twenty Year Trends and Sex Differences in Young Adults Hospitalized With Acute Myocardial Infarction. Circulation 2019;139:1047-56. [Crossref] [PubMed]
- Liu K, Chen S, Lu R. Identification of important genes related to ferroptosis and hypoxia in acute myocardial infarction based on WGCNA. Bioengineered 2021;12:7950-63. [Crossref] [PubMed]
- Andell P, James S, Östlund O, et al. Oxygen therapy in suspected acute myocardial infarction and concurrent normoxemic chronic obstructive pulmonary disease: a prespecified subgroup analysis from the DETO2X-AMI trial. Eur Heart J Acute Cardiovasc Care 2020;9:984-92. [Crossref] [PubMed]
- Sun Q, Luo M, Gao Z, et al. TUG1 knockdown suppresses cardiac fibrosis after myocardial infarction. Mamm Genome 2021;32:435-42. [Crossref] [PubMed]
- Kwok CS, Bennett S, Azam Z, et al. Misdiagnosis of Acute Myocardial Infarction: A Systematic Review of the Literature. Crit Pathw Cardiol 2021;20:155-62. [PubMed]
- Xie Y, Wang Y, Zhao L, et al. Identification of potential biomarkers and immune cell infiltration in acute myocardial infarction (AMI) using bioinformatics strategy. Bioengineered 2021;12:2890-905. [Crossref] [PubMed]
- Soldati S, Di Martino M, Castagno D, et al. In-hospital myocardial infarction and adherence to evidence-based drug therapies: a real-world evaluation. BMJ Open 2021;11:e042878. [Crossref] [PubMed]
- Wu L, Zheng Y, Liu J, et al. Comprehensive evaluation of the efficacy and safety of LPV/r drugs in the treatment of SARS and MERS to provide potential treatment options for COVID-19. Aging (Albany NY) 2021;13:10833-52. [Crossref] [PubMed]
- Josiassen J, Møller JE, Holmvang L, et al. Interventional treatment of acute myocardial infarction-related cardiogenic shock. Curr Opin Crit Care 2021;27:433-9. [Crossref] [PubMed]
- Cao J, Liu Z, Liu J, et al. Bioinformatics Analysis and Identification of Genes and Pathways in Ischemic Cardiomyopathy. Int J Gen Med 2021;14:5927-37. [Crossref] [PubMed]
- Enezate T, Gifft K, Chen C, et al. Percutaneous Versus Surgical Revascularization for Acute Myocardial Infarction. Cardiovasc Revasc Med 2021;31:50-4. [Crossref] [PubMed]
(English Language Editor: J. Jones)