High-risk early-stage lung adenocarcinoma patients are identified by an immune-related circadian clock gene signature
Introduction
The rotation of the earth causes the day-night cycle and deeply influences the circadian rhythm of large mammals. Like many other biological processes, circadian rhythm is also regulated by a series of genes which include several core clock genes and numerous clock-controlled genes (CCGs) (1). The homeostasis of human body inevitably depends on the periodical oscillation of the clock genes. Once disorders have occurred in the circadian rhythm of body, diseases will appear. So far, many diseases have been found to be associated with chronic circadian disruption, such as cardiovascular and cerebrovascular diseases, metabolic diseases, and cancers (2). For example, in mouse lung adenocarcinoma (LUAD) models, loss of the core clock gene, aryl hydrocarbon receptor nuclear translocator like (Arntl, also termed Bmal1), caused an increased tumor burden and a decreased overall survival (OS), suggesting that genetic disruption of core genes of circadian rhythm increased tumorigenesis (3). Lack of the core clock gene Cryptochrome Circadian Regulator 1 (Cry1) and/or Cry2 also resulted in an increased risk of tumorigenesis and radiation-induced tumor growth in mice (4).
As an important component of tumor microenvironment (TME), tumor-infiltrating lymphocytes (TILs) play critical roles in tumorigenesis. Focusing on the circadian rhythm of immune cells within the TME, accumulative studies revealed that molecular clocks, at least partially controlled the metastatic tumor burden. For example, in a breast cancer model, lung metastatic tumor burden in the chronic jet-lagged rats was higher, because of the circadian rhythm disruption in the natural killer (NK) cells (5). In a mouse melanoma model, different injection time of cancer cells demonstrated different lung metastatic burden, while deletion of Bmal1 in neutrophils eliminated the rhythms (6). Although the specific mechanisms of immune rhythms in the TME remain obscure, the immune clock appears to be involved in the development of lung cancer.
In this study, we aimed to construct and validate a circadian clock gene signature (CGS) using gene expression profiles of core clock genes and CCGs from multiple public datasets. This CGS could accurately identify high-risk early-stage LUAD patients among different sequencing platforms including microarray data and RNA-sequencing (RNA-seq) data. To explore the association between CGS and immune infiltration, we performed three different algorithms (including ImmuCellAI, TIMER, and quanTIseq) to calculate the abundance of different tumor-infiltrating immune cells. Finally, we collected LUAD tissues from a local hospital and performed immunohistochemical analyses to validate the differential infiltration of CD4+ T cells in the high- and low-CGS groups. We present the following article in accordance with the TRIPOD reporting checklist (available at https://jtd.amegroups.com/article/view/10.21037/jtd-22-570/rc).
Methods
Data collection
The study was conducted in accordance with the Declaration of Helsinki (as revised in 2013). This study was approved by the Ethics Committees of Tongji Medical College, Huazhong University of Science and Technology (No. UHCT-IEC-SOP-016-03-01). Transcriptome sequencing data and clinical data of early-stage LUAD patients were downloaded from public LUAD datasets, including the Gene Expression Omnibus (GEO) (https://www.ncbi.nlm.nih.gov/geo/) and the Genomic Data Commons Data Portal (https://portal.gdc.cancer.gov/) (7-17). Early-stage LUAD tissues which were used for immunohistochemical analyses were obtained from Union Hospital (Wuhan, China) with the consent of patients Only patients who received no chemotherapy or other immunotherapy were included. Microarray datasets from GEO (GSE68465, GSE50081, GSE42127, GSE41271, GSE37745, GSE31547, GSE31210, GSE30219, GSE26939, GSE14814, GSE13213, GSE11969) were performed with intra-array normalization and inter-array normalization to remove batch effect. All early-stage LUAD cases from GEO database were merged into one dataset. The merged dataset was randomly divided into the training and testing datasets. The early-stage LUAD dataset from The Cancer Genome Atlas (TCGA) was used as an independent validation dataset.
Construction and validation of the CGS
The CGS was constructed according to a previously described method (18). The CGS is comprised of the core clock genes and CCGs which were downloaded from the CircaDB dataset (http://circadb.hogeneschlab.org/human) (19). Only 260 core clock genes and CCGs were detected among all platforms, including Agilent, Affymetrix, and Illumina platforms. Considering the different circadian rhythm of clock genes, one of the core clock genes or one of the CCGs was paired to one of the core clock genes to estimate the relative expression of two clock genes. Finally, the clock gene pair was constructed by one core clock gene and one CCG or two core clock genes (the two core clock genes must be different). Univariate Cox regression analyses of OS were performed to screen clock gene pairs that were significantly related to prognosis of patients with early-stage LUAD in the training dataset. The least absolute shrinkage and selection operator (LASSO)-penalized Cox regression model was utilized to construct the CGS from the clock gene pairs in the training dataset. The optimal model penalty parameter λ was determined by tenfold cross-validation following the previously recommended criteria (20). The time-dependent receiver operating characteristic (ROC) curve analyses were conducted to evaluate the optimal cutoff value and the predictive power of the CGS. The patients were stratified into high- and low-risk groups according to the cutoff of the CGS. The same model was performed in the testing and validation datasets to validate the predictive ability of the CGS. Multivariate Cox regression analyses were performed to validate the CGS as an independent prognostic signature for early-stage LUAD in the training, testing, and validation datasets.
Exploration of the association between the clock gene signature (CGS) and the immune infiltration in early-stage LUAD
Disruption of circadian rhythm partially contributed to the immunosuppression in the tumor microenvironment by attenuating immune infiltration. To compare the abundance of different tumor-infiltrating immune cells in high- and low-CGS groups, ImmuCellAI (http://bioinfo.life.hust.edu.cn/ImmuCellAI#!/) that was based on the ssGSEA algorithm was utilized to analyze the infiltration of 24 immune cell types including 18 T-cell subsets, B cells, dendritic cells, macrophages, monocytes, natural killer cells, and neutrophils (21). To validate the accuracy of ImmuCellAI, TIMER database (http://timer.cistrome.org/) was utilized to calculate the abundance of 6 immune cells including CD4+ T cells, CD8+ T cells, B cells, neutrophils, macrophages, and dendritic cells (22). Moreover, quanTIseq method (http://icbi.at/quantiseq) was also applied to estimate the infiltration of 10 immune cells including non-regulatory CD4+ T cells, regulatory T cells, CD8+ T cells, B cells, M1 macrophages, M2 macrophages, monocytes, neutrophils, NK cells, and myeloid dendritic cells (23).
Immunohistochemical (IHC) analyses
The detailed IHC analysis was conducted according to a previous study (24). All early-stage LUAD tissues used in this study were placed in liquid nitrogen after surgery. The LUAD tissues were fixed in 4% paraformaldehyde and then embedded in paraffin. The paraffin blocks that contained LUAD tissues were cut into sections and processed for antigen activity restoration and endogenous peroxidase activity quenching. The anti-CD4 antibody (ab133616, Abcam, Cambridge, MA, USA) was used for immunostaining. The estimation of the infiltration of CD4+ T cells in the high- and low-CGS groups was performed by 2 independent pathologists. Briefly, the CD4 staining score was estimated by the intensity score [0 (no response), 1 (weak response), 2 (mild response), 3 (strong response)] and the proportional score [1 (0–25%), 2 (26–50%), 3 (51–75%), 4 (76–100%)]. The final CD4 score was calculated by multiplying the intensity score and the proportional score. A score of 0–4 indicated low infiltration of CD4+ T cells, and a score of 5–12 indicated high infiltration of CD4+ T cells.
Statistical analyses
Continuous variables were summarized using median and interquartile range and compared using Wilcoxon test. Categorical variables were compared using chi-square test. The LASSO-penalized Cox proportional hazards regression model was utilized to minimize the risk of overfitting with the glmnet R package. The time-dependent ROC curve was used to determine the optimal cutoff and the areas under the curve (AUCs). The Kaplan-Meier curves and log-rank test were applied to compare OS of patients in high- and low-risk groups with the survival R package and the survminer R package. Univariate and multivariate Cox regression analyses were also conducted with the survival R package and the survminer R package. The restricted mean survival (RMS) curves and the concordance index (C-index) were utilized to compare two models with the survRM2 R package and the survcomp R package. All statistical analyses were conducted with the R software. Two-side P values which were lower than 0.05 were considered statistically significant.
Results
Clinical data
Detailed data of patients are demonstrated in Table 1. In total, 1,820 early-stage LUAD patients from the GEO and TCGA datasets were enrolled. A flow chart demonstrated the complete analysis process (Figure 1). In brief, 12 early-stage LUAD datasets from the GEO dataset were merged into one dataset. Then, the merged dataset was randomly divided into a training dataset and a testing dataset that consisted of 1,084 and 354 early-stage LUAD patients, respectively. The statistical difference between the training and testing datasets was also calculated (Table 1).
Table 1
Characteristics | Training dataset, n=1,084 | Testing dataset, n=354 | P value* | Validation dataset, n=382 |
---|---|---|---|---|
Median age [interquartile range] | 63 [56–70] | 63 [57–71] | 0.538 | 67 [59–72] |
Gender | ||||
Female | 551 | 165 | 0.168 | 205 (53.7) |
Male | 533 | 189 | – | 177 (46.3) |
Stage | ||||
I | 853 | 249 | 0.001 | 262 (68.6) |
II | 231 | 105 | – | 120 (31.4) |
Median overall survival in days | 1,706 | 1,575 | 0.030 | 701.5 |
Number of death (%) | 402 (37.1) | 150 (42.4) | 0.076 | 118 (30.9) |
Data is expressed as interquartile range and number or number (percentage). *, the difference between training and testing cohorts was calculated.
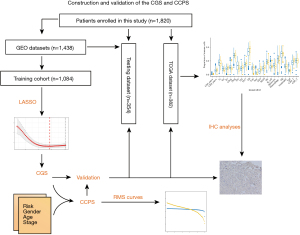
Construction of the CGS
Among the core clock genes and CCGs from CircaDB, only 260 genes were detected in all datasets. The 260-gene list consisted of 255 CCGs and 5 core clock genes, including aryl hydrocarbon receptor nuclear translocator like (ARNTL), clock circadian regulator (CLOCK), period circadian regulator 2 (PER2), cryptochrome circadian regulator 1 (CRY1), and cryptochrome circadian regulator 2 (CRY2). Therefore, a total of 1,285 clock gene pairs that contained at least one of core clock genes were constructed. Among them, only 258 clock gene pairs that were significantly associated with OS were included in the next LASSO regression model. Finally, 37 clock gene pairs that consisted of 35 circadian clock genes were included in the construction of the CGS (Figure 2A,2B). The pan-cancer analysis of 35 circadian clock genes was demonstrated in the supplementary materials (Figure S1). Among clock genes that appeared in at least 1 model, the 37 clock gene pairs were included. Wilcoxon rank-sum test showed significantly higher frequencies for the 37 clock gene pairs compared with the background distribution of frequencies for all clock gene pairs. We then constructed the CGS using the 37 clock gene pairs based on Cox proportional hazards regression model. Detailed information on the 37 clock gene pairs was demonstrated in Table 2. The optimal cutoff for CGS was 1.08 based on the time-dependent ROC analyses. In the training dataset, the AUCs at 1, 3, and 5 years were 0.724, 0.729, and 0.724, respectively (Figure 2C). The Kaplan-Meier curves were used to describe the survival probability of high- and low-risk groups based on the CGS. The log-rank test was used to compare the survival difference between two groups (P<0.001) and the univariate Cox regression model was used to calculate the hazard ratio [HR =3.06; 95% confidence interval (CI): 2.47–3.78] (Figure 2D).
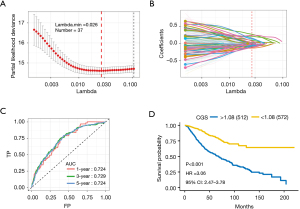
Table 2
Gene 1 | Full name | Gene 2 | Full name | Clock gene pair |
---|---|---|---|---|
ARNTL | Aryl hydrocarbon receptor nuclear translocator like | RNF38 | Ring finger protein 38 | ARNTL-RNF38 |
ARNTL | Aryl hydrocarbon receptor nuclear translocator like | MFAP4 | Microfibril associated protein 4 | ARNTL-MFAP4 |
ARNTL | Aryl hydrocarbon receptor nuclear translocator like | CHD9 | Chromodomain helicase DNA binding protein 9 | ARNTL-CHD9 |
ARNTL | Aryl hydrocarbon receptor nuclear translocator like | ITPR1 | Inositol 1,4,5-trisphosphate receptor type 1 | ARNTL-ITPR1 |
ARNTL | Aryl hydrocarbon receptor nuclear translocator like | RNASE4 | Ribonuclease A family member 4 | ARNTL-RNASE4 |
ARNTL | Aryl hydrocarbon receptor nuclear translocator like | GFOD1 | Glucose-fructose oxidoreductase domain containing 1 | ARNTL-GFOD1 |
ARNTL | Aryl hydrocarbon receptor nuclear translocator like | EPB41L1 | Erythrocyte membrane protein band 4.1 like 1 | ARNTL-EPB41L1 |
ARNTL | Aryl hydrocarbon receptor nuclear translocator like | ETV1 | ETS variant transcription factor 1 | ARNTL-ETV1 |
ARNTL | Aryl hydrocarbon receptor nuclear translocator like | COL4A5 | Collagen type IV alpha 5 chain | ARNTL-COL4A5 |
ARNTL | Aryl hydrocarbon receptor nuclear translocator like | CCR1 | C-C motif chemokine receptor 1 | ARNTL-CCR1 |
CLOCK | Clock circadian regulator | CCR1 | C-C motif chemokine receptor 1 | CLOCK-CCR1 |
CLOCK | Clock circadian regulator | FHL1 | Four and a half LIM domains 1 | CLOCK-FHL1 |
CLOCK | Clock circadian regulator | CDKN1B | Cyclin dependent kinase inhibitor 1B | CLOCK-CDKN1B |
PER2 | Period circadian regulator 2 | RNF38 | Ring finger protein 38 | PER2-RNF38 |
PER2 | Period circadian regulator 2 | RNF103 | Ring finger protein 103 | PER2-RNF103 |
PER2 | Period circadian regulator 2 | SEC61G | SEC61 translocon subunit gamma | PER2-SEC61G |
PER2 | Period circadian regulator 2 | PIK3R1 | Phosphoinositide-3-kinase regulatory subunit 1 | PER2-PIK3R1 |
CRY1 | Cryptochrome circadian regulator 1 | RNF38 | Ring finger protein 38 | CRY1-RNF38 |
CRY1 | Cryptochrome circadian regulator 1 | CBX7 | Chromobox 7 | CRY1-CBX7 |
CRY1 | Cryptochrome circadian regulator 1 | SENP2 | SUMO specific peptidase 2 | CRY1-SENP2 |
CRY1 | Cryptochrome circadian regulator 1 | PSMC4 | Proteasome 26S subunit, ATPase 4 | CRY1-PSMC4 |
CRY1 | Cryptochrome circadian regulator 1 | PCYOX1 | Prenylcysteine oxidase 1 | CRY1-PCYOX1 |
CRY1 | Cryptochrome circadian regulator 1 | ITPR1 | Inositol 1,4,5-trisphosphate receptor type 1 | CRY1-ITPR1 |
CRY1 | Cryptochrome circadian regulator 1 | PLEKHA5 | Pleckstrin homology domain containing A5 | CRY1-PLEKHA5 |
CRY1 | Cryptochrome circadian regulator 1 | FHL1 | Four and a half LIM domains 1 | CRY1-FHL1 |
CRY1 | Cryptochrome circadian regulator 1 | RPS6KA5 | Ribosomal protein S6 kinase A5 | CRY1-RPS6KA5 |
CRY1 | Cryptochrome circadian regulator 1 | PIK3R1 | Phosphoinositide-3-kinase regulatory subunit 1 | CRY1-PIK3R1 |
CRY1 | Cryptochrome circadian regulator 1 | RORA | RAR related orphan receptor A | CRY1-RORA |
CRY1 | Cryptochrome circadian regulator 1 | NFIL3 | Nuclear factor, interleukin 3 regulated | CRY1-NFIL3 |
CRY2 | Cryptochrome circadian regulator 2 | LIFR | LIF receptor subunit alpha | CRY2-LIFR |
CRY2 | Cryptochrome circadian regulator 2 | ZBTB16 | Zinc finger and BTB domain containing 16 | CRY2-ZBTB16 |
CRY2 | Cryptochrome circadian regulator 2 | CBX7 | Chromobox 7 | CRY2-CBX7 |
CRY2 | Cryptochrome circadian regulator 2 | NPM3 | Nucleophosmin/nucleoplasmin 3 | CRY2-NPM3 |
CRY2 | Cryptochrome circadian regulator 2 | MGMT | O-6-methylguanine-DNA methyltransferase | CRY2-MGMT |
CRY2 | Cryptochrome circadian regulator 2 | SF3A2 | Splicing factor 3a subunit 2 | CRY2-SF3A2 |
CRY2 | Cryptochrome circadian regulator 2 | PPFIBP2 | PPFIA binding protein 2 | CRY2-PPFIBP2 |
CRY2 | Cryptochrome circadian regulator 2 | TIMELESS | Timeless circadian regulator | CRY2-TIMELESS |
CGS, clock gene signature.
Validation of the CGS in the testing and validation datasets
To validate the robust prognostic ability of the CGS, we performed the same model in the testing dataset and the independent TCGA validation dataset. The calculated CGS was divided into high- and low-risk according to the same cutoff used in the training dataset (1.08). In the testing dataset, early-stage LUAD patients were significantly stratified into two different prognostic groups according to the Kaplan-Meier curves and log-rank test (HR =2.44; 95% CI: 1.74–3.43; P<0.001) (Figure 3A). In the TCGA validation dataset, the CGS remained robust predictive ability in differentiating patients with different prognosis (HR =2.09, 95% CI: 1.09–4.00; P=0.023) (Figure 3B). Results above demonstrated the high prognostic accuracy of the CGS across different platforms including microarray and RNA-seq platforms.
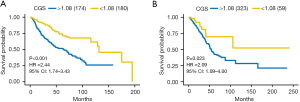
Association between the CGS and immune infiltration in early-stage LUAD
Tumor-infiltrating lymphocytes (TILs) play critical roles in the tumor microenvironment (TME). Disorders of circadian rhythms in TILs are, at least partially, associated with immunosuppression in the TME. Considering that the CGS is comprised of core clock genes and multiple CCGs, we reason that the CGS is related to TILs in early-stage LUAD. To explore the association between the CGS and TILs, we performed multiple methods, including ImmuCellAI (21), Timer (22), and quanTIseq (23), to analyze the immune infiltration among the high- and low-CGS groups in the TCGA LUAD dataset. ImmuCellAI, Timer, and quanTIseq are robust methods to analyze the proportions of multiple immune cells in solid tumors. Immune infiltration analysis demonstrated that tumor-infiltrating CD4+ T cells are significantly higher in the low-CGS group than in the high-CGS group according to ImmuCellAI (P<0.0001), Timer (P<0.05), and quanTIseq (P<0.05) (Figure 4A). Therefore, high infiltration of CD4+ T cells may explain the positive relationship between low CGS and better OS in patients with early-stage LUAD. To validate the high infiltration of CD4+ T cells, we collected several early-stage LUAD tissues from Union Hospital (Wuhan, China) with the consent of the patients and stratified these patients into high- and low-CGS groups based on quantitative real-time polymerase chain reaction (qRT-PCR) data. Detailed data of primers were shown in supplementary materials (Table S1). Then, we performed IHC to analyze the infiltration of CD4+ T cells of LUAD tissues. Results demonstrated that the proportion of tumor-infiltrating CD4+ T cells was higher in the low-CGS group than in the high-CGS group which was consistent with the bioinformatic analyses (Figure 4B). Detailed qRT-PCR data of each patient were provided in the supplementary materials (Figure S2).
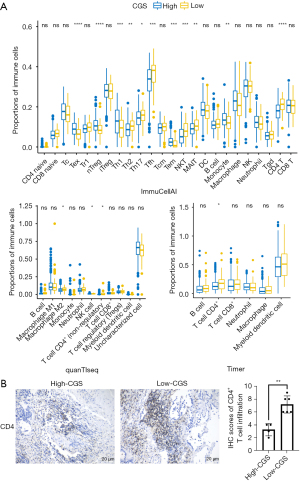
Validation of the CGS as an independent prognostic signature for early-stage LUAD
To validate the CGS as an independent prognostic signature, we performed univariate and multivariate Cox regression analysis with the CGS and other clinical characteristics, including age, gender, and stage. Only variables that were significantly associated with OS in the univariate analysis were included in the following multivariate analysis. Multivariate Cox proportional hazards regression analyses demonstrated that the CGS was an independent prognostic factor for early-stage LUAD in the training (HR =2.73; 95% CI: 2.20–3.38; P<0.001), testing (HR =2.21; 95% CI: 1.57–3.12; P<0.001), and validation (HR =2.34; 95% CI: 1.22–4.48; P=0.011) datasets (Table 3). Because the CGS, age, and stage were independent factors that were associated with OS in early-stage LUAD, these variables were integrated to construct a new score which was referred to as clock clinical prognostic score (CCPS). The CCPS was constructed based on the Cox proportional hazards regression (CCPS =1.03758701 × CGS + 0.03355251 × age + 0.69431531 × stage). The time-dependent ROC curve was used to determine the optimal cutoff value of the CCPS (Figure 5A). The RMS curves of the CGS and CCPS demonstrated that the CCPS performed better in predicting OS of patients with early-stage LUAD in the training dataset (C-index =0.69, 95% CI: 0.66–0.72, vs. C-index =0.72, 95% CI: 0.69–0.74; P=0.003) (Figure 5B). After excluding nine missing values, the same model was utilized to estimate the CCPS in the validation dataset. The Kaplan-Meier curves and log-rank test showed that the CCPS could accurately identify early-stage LUAD patients at high risk in the validation dataset (HR =2.08; 95% CI: 1.35–3.20; P<0.001) (Figure 5C). The RMS curves showed that the CCPS still remained higher accuracy in predicting OS in the validation dataset (C-index =0.55, 95% CI: 0.50–0.61, vs. C-index =0.64, 95% CI: 0.57–0.70; P=0.008) (Figure 5D).
Table 3
Datasets | Variables | Univariate | Multivariate | |||||
---|---|---|---|---|---|---|---|---|
HR | 95% CI | P value | HR | 95% CI | P value | |||
Training dataset | CGS | 3.06 | 2.47–3.78 | <0.001 | 2.73 | 2.20–3.38 | <0.001 | |
Gender | 1.60 | 1.31–1.96 | <0.001 | 1.39 | 1.13–1.69 | 0.001 | ||
Age | 1.04 | 1.03–1.05 | <0.001 | 1.03 | 1.02–1.04 | <0.001 | ||
Stage | 2.34 | 1.90–2.89 | <0.001 | 2.01 | 1.63–2.48 | <0.001 | ||
Testing dataset | CGS | 2.44 | 1.74–3.43 | <0.001 | 2.21 | 1.57–3.12 | <0.001 | |
Gender | 1.14 | 0.83–1.58 | 0.420 | – | – | – | ||
Age | 1.03 | 1.01–1.05 | <0.001 | 1.03 | 1.01–1.05 | 0.002 | ||
Stage | 2.11 | 1.51–2.93 | <0.001 | 2.00 | 1.43–2.79 | <0.001 | ||
Validation dataset | CGS | 2.09 | 1.09–4.00 | 0.023 | 2.34 | 1.22–4.48 | 0.011 | |
Gender | 1.07 | 0.74–1.54 | 0.718 | – | – | – | ||
Age | 1.02 | 1.00–1.04 | <0.001 | 1.02 | 1.00–1.05 | 0.016 | ||
Stage | 2.32 | 1.61–3.34 | <0.001 | 2.22 | 1.54–3.22 | <0.001 |
Age, stage, grade was coded as continuous variable. Stage was coded as I=1, II=2. The risk factors of gender and CGS are male and high CGS, respectively. CGS, clock gene signature; TCGA, The Cancer Genome Atlas; HR, hazard ratio; CI, confidence interval.
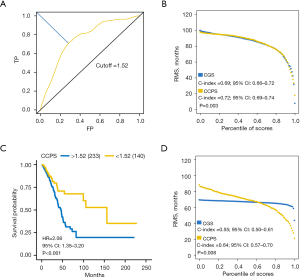
Discussion
Considering that some of patients with early-stage LUAD are in danger of recurrence, novel biomarkers are urgently needed to identify patients at high risk. Numerous gene signatures that were based on transcriptome sequencing data had been constructed for the prognosis evaluation and responses evaluation of LUAD (25,26). In addition to being constructed by key gene mutation profiles (27), prognostic signatures for LUAD could also be developed by a series of interrelated genes including cell-cycle-related genes (28), ferroptosis-related genes (29), immune-related genes (30), and glycolysis-related genes (31). Robust prognostic biomarkers were also constructed from miRNA alteration and DNA methylation data (32-34). However, few studies focused on the circadian CGSs for LUAD.
In this study, we aimed to construct a signature that was based on the key circadian clock genes. Given the periodical oscillation of molecular clock in human lung, we combined core clock genes and CCGs to construct multiple clock gene pairs. Each clock gene pair contained at least one core clock gene. To develop and validate a robust prognostic signature, multiple public datasets from GEO and TCGA databases were combined. LASSO-penalized Cox proportional hazards regression model was utilized to select optimal clock gene pairs. Finally, the CGS was constructed using the relative expression levels of two clock genes in each of the clock gene pairs. Considering the important roles of circadian clock in regulating pulmonary immune responses, we reasoned that there was a strong correlation between the CGS and immune infiltration of early-stage LUAD. Therefore, three bioinformatic methods, including ImmuCellAI, Timer, and quanTIseq, were utilized to evaluate the abundance of different tumor-infiltrating immune cells. Bioinformatic analyses demonstrated that tumor-infiltrating CD4+ T cells were significantly higher in the low-CGS group than in the high-CGS group. To validate the high proportions of CD4+ T cells in the low-CGS group, we collected early-stage LUAD tissues from Union Hospital (Wuhan, China) and performed qRT-PCR to identify early-stage LUAD tissues in different groups. Then, we performed IHC on the paraffin sections of early-stage LUAD tissues. IHC analyses demonstrated that the proportion of tumor-infiltrating CD4+ T cells was higher in the low-CGS group which was in coincidence with bioinformatic results. Recently, studies have shown that CD4+ T cells also function as direct tumor killers based on a HER2-targeting trispecific antibody therapy (35). This may explain the strong association between the higher abundance of CD4+ T cells and the better prognosis of patients in the low-CGS group.
The CGS was constructed using 35 unique circadian clock genes, including 5 core clock genes (ARNTL, CLOCK, PER2, CRY1, CRY2) and 30 CCGs (Table 2). The heterodimer of CLOCK and BMAL1 (also termed ARNTL) serves as a transcription factor to control the circadian expression of approximately 15% of genes by binding their E-Box sequences (36). Bioinformatic analyses showed that the expression of CRY2 was significantly different between normal tissues and cancer tissues in the TCGA LUAD dataset (37). The Kaplan-Meier curve and log-rank test showed that high levels of CRY2 and ARNTL were positively associated with prolonged OS in patients with LUAD (37). Pan-cancer analyses showed that the expression of negative core clock genes (PER1, PER2, CRY1, and CRY2) was widely downregulated, while the expression of positive core clock genes (ARNTL and CLOCK) rarely changed (38). The circadian clock disruption partially contributed to the immunosuppression and T cell exhaustion in the TME (38). Accumulating evidence had shown that circadian rhythm disruption promoted tumor proliferation by reconstructing immune infiltration in the TME (39). For example, circadian clock disruption remodeled the daily patterns of tumor-infiltrating M1 and M2 macrophages to favor tumor growth in a mouse melanoma model (40). Furthermore, circulating factors in the serum of patients with cancer could interrupt peripheral circadian rhythm in some degree. In one study by Chang et al., serum from patients with untreated LUAD was found to lengthen the daily oscillation of ARNTL of lung cancer cell lines, while heat inactivation of the serum eliminated the impact (41). In summary, circadian rhythm disruption played an important role in favoring lung cancer proliferation by remodeling immune infiltration in the TME, which partially explained the robust ability of prognosis evaluation and strong immune correlation of the CGS.
In conclusion, the CGS is a promising prognostic biomarker for early-stage LUAD. The positive correlation between low CGS and high infiltration of CD4+ T cells reflected the reconstruction of immune infiltration in early-stage LUAD microenvironment caused by chronic circadian rhythm disruption. In addition, further studies are urgently needed for the efficient estimation of prognosis prediction of the CGS.
Acknowledgments
We would like to thank Shi-Peng Guo for the bioinformatic analyses. We would like to thank Ying Yin for the experimental suggestions. The medical writing of this manuscript was improved with the help of Editage (www.editage.cn).
Funding: This work was supported by grants from the National Natural Science Foundation of China (No. 81973990, No. 81900096, and No. 81770090).
Footnote
Reporting Checklist: The authors have completed the TRIPOD reporting checklist. Available at https://jtd.amegroups.com/article/view/10.21037/jtd-22-570/rc
Conflicts of Interest: All authors have completed the ICMJE uniform disclosure form (available at https://jtd.amegroups.com/article/view/10.21037/jtd-22-570/coif). The authors have no conflicts of interest to declare.
Ethical Statement: The authors are accountable for all aspects of the work in ensuring that questions related to the accuracy or integrity of any part of the work are appropriately investigated and resolved. The study was conducted in accordance with the Declaration of Helsinki (as revised in 2013). This study was approved by the Ethics Committees of Tongji Medical College, Huazhong University of Science and Technology (UHCT-IEC-SOP-016-03-01). Early-stage LUAD tissues which were used for immunohistochemical analyses were obtained from Union Hospital (Wuhan, China) with the consent of patients.
Open Access Statement: This is an Open Access article distributed in accordance with the Creative Commons Attribution-NonCommercial-NoDerivs 4.0 International License (CC BY-NC-ND 4.0), which permits the non-commercial replication and distribution of the article with the strict proviso that no changes or edits are made and the original work is properly cited (including links to both the formal publication through the relevant DOI and the license). See: https://creativecommons.org/licenses/by-nc-nd/4.0/.
References
- Patke A, Young MW, Axelrod S. Molecular mechanisms and physiological importance of circadian rhythms. Nat Rev Mol Cell Biol 2020;21:67-84. [Crossref] [PubMed]
- Masri S, Sassone-Corsi P. The emerging link between cancer, metabolism, and circadian rhythms. Nat Med 2018;24:1795-803. [Crossref] [PubMed]
- Papagiannakopoulos T, Bauer MR, Davidson SM, et al. Circadian Rhythm Disruption Promotes Lung Tumorigenesis. Cell Metab 2016;24:324-31. [Crossref] [PubMed]
- Shafi AA, Knudsen KE. Cancer and the Circadian Clock. Cancer Res 2019;79:3806-14. [Crossref] [PubMed]
- Logan RW, Zhang C, Murugan S, et al. Chronic shift-lag alters the circadian clock of NK cells and promotes lung cancer growth in rats. J Immunol 2012;188:2583-91. [Crossref] [PubMed]
- Casanova-Acebes M, Nicolás-Ávila JA, Li JL, et al. Neutrophils instruct homeostatic and pathological states in naive tissues. J Exp Med 2018;215:2778-95. [Crossref] [PubMed]
- Beer DG, Kardia SL, Huang CC, et al. Gene-expression profiles predict survival of patients with lung adenocarcinoma. Nat Med 2002;8:816-24. [Crossref] [PubMed]
- Rousseaux S, Debernardi A, Jacquiau B, et al. Ectopic activation of germline and placental genes identifies aggressive metastasis-prone lung cancers. Sci Transl Med 2013;5:186ra66. [Crossref] [PubMed]
- Okayama H, Kohno T, Ishii Y, et al. Identification of genes upregulated in ALK-positive and EGFR/KRAS/ALK-negative lung adenocarcinomas. Cancer Res 2012;72:100-11. [Crossref] [PubMed]
- Sato M, Larsen JE, Lee W, et al. Human lung epithelial cells progressed to malignancy through specific oncogenic manipulations. Mol Cancer Res 2013;11:638-50. [Crossref] [PubMed]
- Der SD, Sykes J, Pintilie M, et al. Validation of a histology-independent prognostic gene signature for early-stage, non-small-cell lung cancer including stage IA patients. J Thorac Oncol 2014;9:59-64. [Crossref] [PubMed]
- Tomida S, Takeuchi T, Shimada Y, et al. Relapse-related molecular signature in lung adenocarcinomas identifies patients with dismal prognosis. J Clin Oncol 2009;27:2793-9. [Crossref] [PubMed]
- Wilkerson MD, Yin X, Walter V, et al. Differential pathogenesis of lung adenocarcinoma subtypes involving sequence mutations, copy number, chromosomal instability, and methylation. PLoS One 2012;7:e36530. [Crossref] [PubMed]
- Bhattacharjee A, Richards WG, Staunton J, et al. Classification of human lung carcinomas by mRNA expression profiling reveals distinct adenocarcinoma subclasses. Proc Natl Acad Sci U S A 2001;98:13790-5. [Crossref] [PubMed]
- Takeuchi T, Tomida S, Yatabe Y, et al. Expression profile-defined classification of lung adenocarcinoma shows close relationship with underlying major genetic changes and clinicopathologic behaviors. J Clin Oncol 2006;24:1679-88. [Crossref] [PubMed]
- Tang H, Xiao G, Behrens C, et al. A 12-gene set predicts survival benefits from adjuvant chemotherapy in non-small cell lung cancer patients. Clin Cancer Res 2013;19:1577-86. [Crossref] [PubMed]
- Kuner R, Muley T, Meister M, et al. Global gene expression analysis reveals specific patterns of cell junctions in non-small cell lung cancer subtypes. Lung Cancer 2009;63:32-8. [Crossref] [PubMed]
- Li B, Cui Y, Diehn M, et al. Development and Validation of an Individualized Immune Prognostic Signature in Early-Stage Nonsquamous Non-Small Cell Lung Cancer. JAMA Oncol 2017;3:1529-37. [Crossref] [PubMed]
- Pizarro A, Hayer K, Lahens NF, et al. CircaDB: a database of mammalian circadian gene expression profiles. Nucleic Acids Res 2013;41:D1009-13. [Crossref] [PubMed]
- Tu Z, Wu L, Wang P, et al. N6-Methylandenosine-Related lncRNAs Are Potential Biomarkers for Predicting the Overall Survival of Lower-Grade Glioma Patients. Front Cell Dev Biol 2020;8:642. [Crossref] [PubMed]
- Miao YR, Zhang Q, Lei Q, et al. ImmuCellAI: A Unique Method for Comprehensive T-Cell Subsets Abundance Prediction and its Application in Cancer Immunotherapy. Adv Sci (Weinh) 2020;7:1902880. [Crossref] [PubMed]
- Li T, Fan J, Wang B, et al. TIMER: A Web Server for Comprehensive Analysis of Tumor-Infiltrating Immune Cells. Cancer Res 2017;77:e108-10. [Crossref] [PubMed]
- Finotello F, Mayer C, Plattner C, et al. Molecular and pharmacological modulators of the tumor immune contexture revealed by deconvolution of RNA-seq data. Genome Med 2019;11:34. [Crossref] [PubMed]
- Wei S, Shao J, Wang J, et al. EHD2 inhibits the invasive ability of lung adenocarcinoma and improves the prognosis of patients. J Thorac Dis 2022;14:2652-64. [Crossref] [PubMed]
- Guo NL, Dowlati A, Raese RA, et al. A Predictive 7-Gene Assay and Prognostic Protein Biomarkers for Non-small Cell Lung Cancer. EBioMedicine 2018;32:102-10. [Crossref] [PubMed]
- Song Y, Yan S, Fan W, et al. Identification and Validation of the Immune Subtypes of Lung Adenocarcinoma: Implications for Immunotherapy. Front Cell Dev Biol 2020;8:550. [Crossref] [PubMed]
- Sun H, Liu SY, Zhou JY, et al. Specific TP53 subtype as biomarker for immune checkpoint inhibitors in lung adenocarcinoma. EBioMedicine 2020;60:102990. [Crossref] [PubMed]
- Jiang W, Xu J, Liao Z, et al. Prognostic Signature for Lung Adenocarcinoma Patients Based on Cell-Cycle-Related Genes. Front Cell Dev Biol 2021;9:655950. [Crossref] [PubMed]
- Zhang A, Yang J, Ma C, et al. Development and Validation of a Robust Ferroptosis-Related Prognostic Signature in Lung Adenocarcinoma. Front Cell Dev Biol 2021;9:616271. [Crossref] [PubMed]
- Song Q, Shang J, Yang Z, et al. Identification of an immune signature predicting prognosis risk of patients in lung adenocarcinoma. J Transl Med 2019;17:70. [Crossref] [PubMed]
- Zhang L, Zhang Z, Yu Z. Identification of a novel glycolysis-related gene signature for predicting metastasis and survival in patients with lung adenocarcinoma. J Transl Med 2019;17:423. [Crossref] [PubMed]
- Modhukur V, Iljasenko T, Metsalu T, et al. MethSurv: a web tool to perform multivariable survival analysis using DNA methylation data. Epigenomics 2018;10:277-88. [Crossref] [PubMed]
- Anuraga G, Wang WJ, Phan NN, et al. Potential Prognostic Biomarkers of NIMA (Never in Mitosis, Gene A)-Related Kinase (NEK) Family Members in Breast Cancer. J Pers Med 2021;11:1089. [Crossref] [PubMed]
- Liu F, Wu H. Identification of Prognostic Biomarkers and Molecular Targets Among JAK Family in Breast Cancer. J Inflamm Res 2021;14:97-114. [Crossref] [PubMed]
- Seung E, Xing Z, Wu L, et al. A trispecific antibody targeting HER2 and T cells inhibits breast cancer growth via CD4 cells. Nature 2022;603:328-34. [Crossref] [PubMed]
- Koike N, Yoo SH, Huang HC, et al. Transcriptional architecture and chromatin landscape of the core circadian clock in mammals. Science 2012;338:349-54. [Crossref] [PubMed]
- Qiu M, Chen YB, Jin S, et al. Research on circadian clock genes in non-small-cell lung carcinoma. Chronobiol Int 2019;36:739-50. [Crossref] [PubMed]
- Wu Y, Tao B, Zhang T, et al. Pan-Cancer Analysis Reveals Disrupted Circadian Clock Associates With T Cell Exhaustion. Front Immunol 2019;10:2451. [Crossref] [PubMed]
- Wang Z, Su G, Dai Z, et al. Circadian clock genes promote glioma progression by affecting tumour immune infiltration and tumour cell proliferation. Cell Prolif 2021;54:e12988. [Crossref] [PubMed]
- Aiello I, Fedele MLM, Román F, et al. Circadian disruption promotes tumor-immune microenvironment remodeling favoring tumor cell proliferation. Sci Adv 2020;6:eaaz4530. [Crossref] [PubMed]
- Chang Y, Zhao C, Ding H, et al. Serum factor(s) from lung adenocarcinoma patients regulates the molecular clock expression. J Cancer Res Clin Oncol 2021;147:493-8. [Crossref] [PubMed]