Characteristics of circadian rhythm-related genes and establishment of a prognostic scoring system for lung adenocarcinoma with experimental verification: a bioinformatics analysis
Introduction
In recent years, circadian rhythm (CR)-related genes (CRRGs) have become a hot topic in cancer research. Many studies have shown that CRRGs regulate cell proliferation, malignant tumor cell apoptosis, and neuroendocrine and immune function. The CRRGs are expressed in many behaviors and physiological processes, including tumor occurrence and development (1). Disruption to the CR plays a key role in tumorigenesis and promotes the establishment of cancer features. Moreover, tumorigenesis impairs the CR directly (2). In recent years, researchers have given increasing attention to the effects of the CR in the human body. For example, its role in tumorigenesis, cancer characteristics, treatment options, and how CRRGs work are becoming interesting topics for future research (3,4). There are strong links between cancer and CR disorders. For example, transcription of core CRRGs affects the efficacy of treatment and the prognosis of a variety of cancers (5-7). However, the mechanisms regulating the effects of CRRGs on clinical prognosis remain unclear.
A previous study showed that disruption of the CR can promote the development of lung tumors (8). Lung cancer is the leading cause of cancer death, accounting for 18.4% of all cancer deaths, and has the highest incidence of all types of cancer worldwide (11.6%) (9). Non-small cell lung cancer (NSCLC) is the most common type of lung cancer, accounting for approximately 85% of cases. Lung adenocarcinoma (LUAD) is the most common type of NSCLC, and its incidence is increasing annually. Therefore, it is necessary to identify key molecules and to establish an effective prediction model with good stability that can be used to implement precise treatment and improve the prognosis of patients with LUAD.
In this study, we investigated the relationship between LUAD prognosis and CRRGs and established a CR scoring system (CRscore) to predict the prognosis of patients with LUAD and guide treatment selection. We present the following article in accordance with the TRIPOD reporting checklist (available at https://jtd.amegroups.com/article/view/10.21037/jtd-22-1112/rc).
Methods
Data source and pre-processing
The data and clinical information of patients with LUAD and somatic mutation data were obtained from The Cancer Genome Atlas (TCGA). The download gene expression profiles met the following conditions: (I) the disease type were “adenomas and adenocarcinomas”; (II) the primary site was “bronchus and lung”; (III) the program was “TCGA”; (IV) the project ID was “TCGA-LUAD”; (V) the data category was “transcriptome profiling”; (VI) the data type was “Gene Expression Quantification”; (VII) the workflow type was“HTSeq-FPKM”. In addition, another set of files (GSE37745) was downloaded from the Gene Expression Omnibus (GEO) database to ensure the adequacy of the sample size. Subsequently, in UCSC Xena (http://xena.ucsc), we downloaded the copy number data of LUAD (10). Using the limma package in R (The R Foundation for Statistical Computing, Vienna, Austria), the standard human gene expression matrix of each independent sample was converted from the TCGA gene expression profile, and transcripts per million (TPM) data were converted from fragments per kilobase million (FPKM) data (11). The standard human gene names were converted from two GEO files in Perl, as was the clinical information obtained from the GEO. The batch effects of the data were corrected using the sva package of R (12). We combined the statistical data of TCGA-LUAD and GSE37745 as the combined queue. We used R (version 4.1.1) and Strawberry Perl (version 5.32.1; https://strawberryperl.com/) to process the data. The study was conducted in accordance with the Declaration of Helsinki (as revised in 2013).
Differential expression of CRRGs
This study examined 10 genes (AANAT, NPAS2, ARNTL, CRY1, PER3, CLOCK, CRY2, CSNK1E, NR1D2, and BHLHE40). First, the copy numbers of the CRRGs were extracted from TCGA-LUAD using Perl software, and histograms were intuitively constructed using R software. The R Circos package was used to map the change in 10 CRRGs on 23 pairs of chromosomes to investigate the relationships between CRRG copy number and chromosome. The differential expression of these CRRGs in TCGA-LUAD was compared using the Wilcoxon rank-sum test in the limma package, which provides a comprehensive solution for microarray and RNA-Seq differential analyses (13). A waterfall plot was created using the maftools package of R to determine the mutation rate of CRRGs in patients with LUAD. A boxplot was constructed using the ggpubr package of R, and a heatmap was drawn using the pheatmap package. A P value of <0.05 was considered statistically significant.
CR regulator analysis
CR clustering
To determine the value of CRRGs, we used an unsupervised cluster analysis to organize the amalgamated dataset according to the expression of CRRGs. The samples were clustered using the ConsensusClusterPlus package according to the expression of CRRGs. All samples were divided into K=[2–9] groups, and the most suitable CR regulator cluster was obtained according to three conditions after the cycle, including a close connection within types and an unclose connection between types, the number of samples in each cluster was not short, and no significant increase in the cumulative distribution curve area. According to the correlations between the CRRG clusters and the survival status, the survminer package was used to determine the cut-off points of each subgroup of data, and all possible cut-off points were tested to identify the maximum rank statistics. Based on the log-rank statistics, patients were divided into high, medium, and low expression groups. The Kaplan-Meier method and the survminer package were used to generate survival curves for the predictive analysis. The log-rank test was used to identify significant differences. A P value of <0.05 was considered statistically significant.
Single-sample gene set enrichment analysis (GSEA) and gene set variation analysis (GSVA)
GSVA is a non-parametric, unsupervised method for estimating path changes and changes in the activity of biological processes in samples in an experimental dataset (14). Based on differences in CRRGs, we revealed the biological pathways between different CRRG clusters. For the GSEA, we download “C2.CP.KEGG.7.5.1.symbols”. The scores of different paths in each sample were calculated using the GSA package of R, and the path differences were analyzed using the limma package. A P value of <0.05 indicated differential expression of pathways in pathway regulation (15,16). Heatmaps were drawn using the pheatmap package in R. We used the single-sample GSEA and the gene enrichment score to inspect the relative abundance of immune cell infiltration to obtain the immune score (17). We obtained the gene sets of every type of tumor microenvironment (TME)-infiltrating immune cell from a previous study (18), including activated dendritic cells, CD8+ T cells, regulatory T cells, and macrophages. The pertinence of CRRG clusters and immune scores was explored using the limma package, and the ggpubr package was used to draw the box graph. A P value of <0.05 was considered statistically significant.
Differential analysis
To identify differentially expressed genes (DEGs) related to CR, we used the limma and VennDiagram packages to identify DEGs between CRRG clusters. The DEGs with an adjusted P<0.001 were reserved (19). Gene Ontology (GO) and Kyoto Encyclopedia of Genes and Genomes (KEGG) analyses were used to examine the pathways and functions of DEGs. We used P values and Q values of <0.05 to identify the potential pathways and biological functions of these DEGs. According to the number of enriched CRRGs, we chose the top 30 KEGG pathways and GO pathways.
CRRG clusters
To identify the CRRGs there were associated with prognosis (P<0.05), univariate Cox regression analysis was used to analyze the DEGs using the survminer package. The ConsensusClusterPlus package was used to cluster the samples according to the expression of prognostic CRRGs to determine the CRRG clusters that should be further analyzed. First, we performed a survival analysis to evaluate the prognostic value of the CRRG clusters using the survminer package. Then, the patients were divided into three groups: A, B, and C. The Kaplan-Meier method was used to draw the survival curves of the three groups, and the difference between the three groups was significant (P<0.001) according to the log-rank test. After collecting the clinical data (LUAD stage, age, gender, and alive/deceased status), the pheatmap package of R was used to draw a heatmap of the correlations between clinical features and CRRG clusters. The boxplot was drawn using the ggpubr package.
CR score
To quantify the expression of CRRGs in patients with LUAD, we constructed a CR scoring system (CRscore) based on the CRRGs associated with prognosis. Then, we used the principal component analysis (PCA) to construct the CRscore. The PCA can effectually identify the most significant portions and structures in the data, eliminate redundancy and noise, reduce the dimension of primitive intricate data, and uncover the simple structure hidden behind the convoluted data.
We used the following calculation to construct the CRscore, mainly using the PCA:
where i represents the expression of prognostic DEGs.
Correlations between the CR score and patients’ clinical characteristics
We divided the patients into a high CR score group and a low CR score group for further analysis using the CRscore. First, the survival analysis was used to assess the prognostic value of the CRRG clusters using the same method as described above. Then, we analyzed the relationships between the CRscore and patients’ clinical features, including age, gender, survival status, and LUAD stage, to identify the relationship between the CR score and survival in the context of the different individual clinical characteristics using the univariate and multivariate Cox regression analyses. Patients with the same clinical characteristics were analyzed respectively to exclude the influence of clinical characteristics on the conclusion. Then, the correlations between CR clusters, gene clusters, CR grouping, and clinical data (LUAD stage, age, gender, alive/deceased status) were measured using the ggalluvial package for Mulberry plots. In addition, differences in the CR score were calculated for the different clinical characteristics (stage, age, and gender). The plyr and ggpubr packages were used to construct percentage plots and box-line plots, respectively. The CR score and CR stage were compared using the limma package. The log-rank test was used to identify statistically significant differences, and the Kaplan–Meier method was used to analyze each clinical feature based on the CR score.
Correlations between the CR score and the tumor mutation burden (TMB)
The TMB is the sum of somatic gene-coding errors, gene insertions, deletion errors, and base substitutions per million bases. First, using Perl software, the TMB was calculated for each sample. A correlation diagram and boxplot of the relationship between the TMB and CR grouping was constructed using the ggpubr package of R. Then, the survminer package was used to perform the survival analysis. According to the TMB, all samples were divided into two groups: the low expression group and the high expression group. We also used the Kaplan-Meier method to plot the survival curves based on the TMB and the TMB combined with the CR score. A P value of <0.05 was considered statistically significant with the log-rank test.
Analysis of immune checkpoint genes
First, the corrplot package was applied to contrast the correlation between the immune score and the CR score. Then, the samples (by CR group) were crossed with the clinical information samples (survival status), and the data were combined using R software. Immunotherapy score files were acquired from The Cancer Immunome Atlas (TCIA) website (https://tcia.at/home). A violin plot was created to observe the relationship between immune checkpoint genes and groups with high and low CR scores using the ggpubr package. We analyzed the relationships between the CR score and the expression of common immune checkpoints (PD-L1, PD-L2, PD-1, CTLA4) using the limma package.
Prognostic treatment of LUAD based on the CR score
The immunophenotypic scores (IPSs) of TCGA-LUAD patients were obtained from the TCIA database (20). The difference in the IPS between the high CR score group and the low CR score group was analyzed to comprehend the immunogenicity of the two groups of patients. We used the pRRophetic package of R to predict the half-maximum inhibitory concentration of five chemotherapeutic agents for the treatment of LUAD, including cisplatin, gemcitabine, paclitaxel, vinorelbine, and methotrexate (21). These five kinds of chemotherapy drugs in LUAD patients with the sensitivity of the forecast are based on the cancer drug sensitivity genomics (GDSC; https://www.cancerrxgene.org/).
Statistical analysis
The Kruskal-Wallis test was used to compare three or more groups. Using the Surv-Cutpoint function in the survminer package of R, the patients were divided into two groups: the high CR score group and the low CR score group. Spearman’s and distance correlation analyses were used to calculate the correlation coefficients between the TME-infiltrated immune cells and CRRG expression. We used Pearson’s correlation to calculate the correlation coefficients between CRRGs. A P value of <0.001 was considered statistically significant. The univariate Cox regression analysis was used to calculate the hazards ratios of the CRRGs and DEGs. The cor.mtest function was used to calculate the relationship between the CR score and immune cell infiltration, and the corrplot package of R was used to visualize this relationship. Spearman’s correlation analysis was used to obtain the correlation coefficient between the TMB and the CR score. The R Circos package was used to show the change in copy number on 23 chromosomes based on the CRRGs identified from TCGA-LUAD (22). The maftools package was used to construct a waterfall plot to show the mutation status of TCGA-LUAD. All data were analyzed using R (version 4.1.1) or Perl (version 5.32.1) software (23). The R packages used in this study and their functions are available from the Bioconductor website (https://www.bioconductor.org/).
Clinical validation of CRRGs
Quantitative reverse transcription polymerase chain reaction (PCR)
We extracted total RNA from freshly isolated tissues utilizing TRIZOL reagent (#15596026; Thermo Fischer Scientific, Waltham, MA, USA). Complementary DNA was synthesized from whole RNA using random primers. The PCR primer sequences of NPAS2 were designed as follows: forward: 5'-CGTGTTGGAAAAGGTCATCGG-3'; reverse: 5'-TCCAGTCTTGCTGAATGTCAC-3'. Reverse transcription was performed at 42 ℃ for 30 minutes, followed by 85 ℃ for 5 minutes. The PCR conditions included initial denaturation for 10 minutes at 95 ℃, followed by 40 cycles of 95 ℃ for 20 seconds, 55 ℃ for 30 seconds, 72 ℃ for 30 seconds, 95 ℃ for 1 minute, and 55 ℃ for 30 seconds. The messenger RNA (mRNA) of NPAS2 was quantified by quantitative reverse transcription (qRT)-PCR with SYBR Premix ExTaq (Applied Takara Bio, Shiga, Japan; Baori Medical Biotechnology, Beijing, China) and normalized to glyceraldehyde 3-phosphate dehydrogenase (GAPDH) as the reference gene.
Western blot
Total protein was extracted from tissue, and the protein concentration was determined using the bicinchoninic acid assay (#23225). Then, 20 µg protein was loaded into each well and separated by polyacrylamide gel electrophoresis (PAGE). The protein was then transferred to a polyvinylidene fluoride (PVDF) membrane using the wet transfer method and blocked with 5% skimmed milk at room temperature for 2 hours. Rabbit anti-human NPAS2 (1:2,000, PHR3777) and rabbit anti-human GAPDH were incubated overnight at 4 ℃. After washing, horseradish peroxidase (HRP)-conjugated goat anti-rabbit secondary antibody (1:10,000, #7076) was incubated at 37 ℃ for 2 hours. The membrane was prepared with enhanced chemiluminescence reagent. The average band strength was measured using Image J software (National Institutes of Health, Bethesda, MD, USA). The gray value of the target protein was divided by the gray value of GAPDH to calculate the relative protein expression of the target. All antibodies were purchased from Abmart (Shanghai, China).
Results
Epigenetic analysis of CR in LUAD samples
A total of 10 CRRGs were examined in this study. As shown in the waterfall figure, 33 of 561 (5.88%) samples had mutations (Figure 1A). The mutation rates of PER3, CRY2, NPAS2, CRY1, CLOCK, ARNTL, and CSNK1E were 1%, and the mutation rates of BHLHE40, NR1D2, and AANAT were 0%. The copy number variation (CNV) analysis showed a significant increase in the copy numbers of AANAT, NPAS2, ARNTL, CRY1, PER3, CLOCK, and CRY2, and there was a marked decrease in the copy numbers of CSNK1E, NR1D2, and BHLHE40 (Figure 1B). The circle diagram shows the chromosomal locations with CNVs in CRRGs (Figure 1C). The LUAD tissues and healthy tissues can be identified by CNVs in chromosomes. To identify the relationship between regulatory factors and epigenetics, we analyzed the expression of CRRGs (Figure 1D,1E). The genes NR1D2, AANAT, CRY1, NPAS2, CSNK1E, PER3, and CRY2 were significantly differentially expressed between LUAD tissues and healthy tissues (P<0.05) Changes in the expression of CRRGs may vary with copy number. The CRRGs demonstrated specific epigenetic changes in tumor tissues and adjacent non-cancerous tissues. Therefore, seven CRRGs with obvious differences in expression (NR1D2, AANAT, CRY1, NPAS2, CSNK1E, PER3, and CRY2) were studied further.
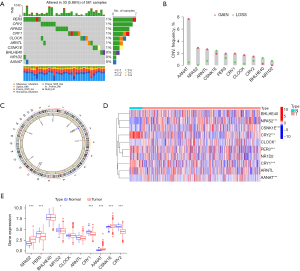
Unsupervised clustering based on CR
We introduced a new cohort (merged cohort), which consisted of TCGA-LUAD data and GEO data (GSE37745). Unsupervised clustering was used to separate the tumor samples according to the expression of the seven CRRGs mentioned above. According to the cumulative distribution function value, the optimal quantitative cluster was determined as 3 (K=3). Thus, the tumor samples were divided into three groups (Figure 2A): CRcluster A, CRcluster B, and CRcluster C. There were significant differences among the three clusters according to the results of the PCA (Figure 2B). Specifically, CRcluster C had the greatest survival advantage (Figure 2C). The heatmap shows differences in CRRG expression among the different clusters, and the expression of CRRGs was highest in CRcluster B (Figure 2D).
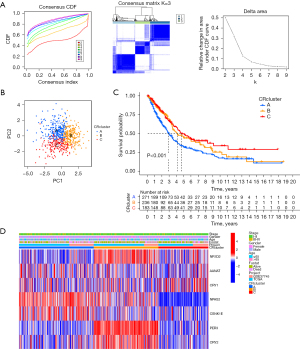
Differences in immune cell infiltration and function between the CR clusters
To explore the potential biological functions of the three CRRG clusters, the GSVA analysis was performed (Figure 3A-3C). CRcluster A and CRcluster B were mainly related to CR in mammals, such as transforming growth factor-beta (TGF-beta), the cellular response to TGF-beta, and regulation of the TGF-beta receptor. CRcluster C was related to protein expression, such as the mitogen-activated protein kinase (MAPK) signaling pathway and neurodegeneration. There were significant differences in immune cell infiltration among the three clusters based on the results of the GSEA analysis (Figure 4A). We found that CRcluster C had a mass of infiltrating immune cells, including CD4+ T cells and CD8+ T cells, which are involved in specific immunity. CRcluster A included dendritic cells, macrophages, and monocytes, which participate in the non-specific immune response. CRcluster B included eosinophils, immature dendritic cells, myeloid-derived suppressor cells, macrophages, mast cells, and plasmacytoid dendritic cells (P<0.05). Patients in CRcluster C revealed a favorable survival status (Figure 2C). In combination with the results of the GSVA analysis, we predicted that immune cell infiltration may play a major anti-tumor role (21).
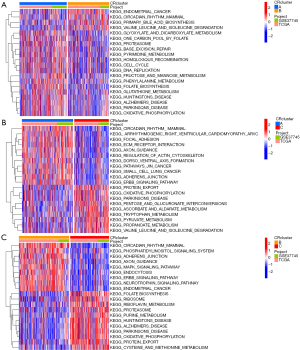
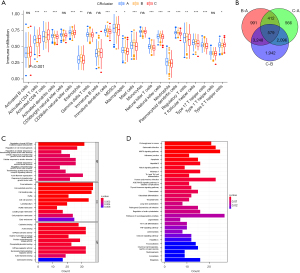
Identification of DEGs related to CR
Based on the three CR clusters, we conducted a differential analysis of the amalgamated cohort to identify DEGs related to CR. There were 5,230 DEGs between CRcluster A and CRcluster B; 3,653 DEGs between CRcluster A and CRcluster C; and 7,865 DEGs between CRcluster B and CRcluster C (Figure 4B). A total of 579 DEGs related to CR with adjusted P values of <0.001 were selected based on the co-intersection of the CR typing differential genes. These genes were analyzed using GO and KEGG enrichment analyses to identify their functions (Figure 4C,4D). We identified 151 GO terms and 49 KEGG pathways (P<0.05, Q<0.05). The top 30 GO terms and KEGG pathways with the greatest number of genes were screened. The results showed that the GO terms and KEGG pathways above were mainly involved in the MAPK signaling pathway, the cellular response to environmental stimuli, the cellular response to abiotic stimuli, protein serine or threonine kinase activity, and melanogenesis. We then performed the univariate Cox regression analysis on the 579 DEGs related to CR and identified 110 DEGs (P<0.05). We identified 16 prognosis-related DEGs (Figure 5A), which showed a significant ability to predict patient survival (P<0.05). Coincidentally, the patients were still divided into three clusters using the unsupervised cluster analysis of the 110 CRRGs (Figure 5B). Patients with gene cluster B had the greatest survival rate in the survival analysis (Figure 5C). We found that the proportion of patients with LUAD stage I–II was particularly large, and these patients were mainly concentrated in gene cluster B (Figure 6A). Subsequently, on the basis of the three gene clusters, we analyzed the differential expression of the seven CRRGs in the merged cohort using the limma package. As expected, there were remarkable differences in CRRG expression among the three gene clusters (Figure 6B). The regulatory mechanism of CRRGs was verified based on the above results.
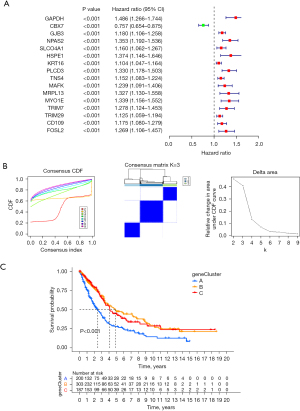
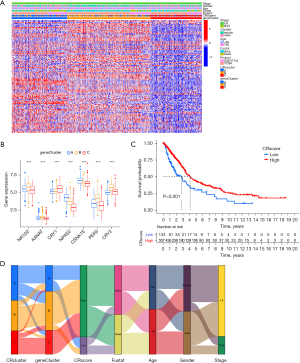
Relationship between the CR score and traits of each subtype
We established a scoring system (CRscore) to quantify the expression of the 110 DEGs related to CR as prognostic predictors. The survival rate of patients with a high CR score was significantly higher than the survival rate of patients with a low CR score according to the survival analysis (Figure 6C). Changes in the clinical characteristics (LUAD stage, age, gender, alive/deceased status) and subgroups of patients are shown in the Sankey diagram (Figure 6D). Most immune cells were negatively correlated with the CR score (Figure 7A), and the infiltrating immune cells were significantly negatively correlated with the CRscore (Figure 7B). In other words, the lower the CR score, the stronger the immunity. The Kruskal-Wallis test showed a significant difference in the CR score between the CR clusters and the gene clusters. CRcluster A had the lowest score, while CRcluster C had the highest score. As a result, we hypothesized that patients with high and low C Rscores were more inclined to suppress and develop tumors, respectively. This is well supported by the survival curves of the high and low CR score groups (Figure 6B). In terms of the gene clusters, the CR score sequence was gene cluster C > gene cluster B > gene cluster A (Figure 8A). The same was true for the CR clusters (CRcluster C > Crcluster B > Crcluster A) (Figure 8B).
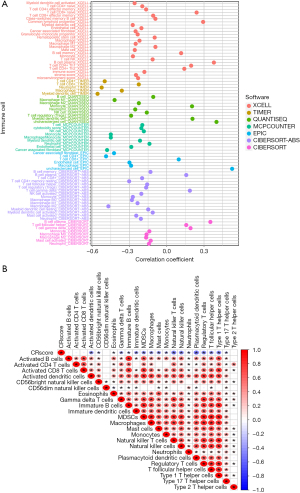
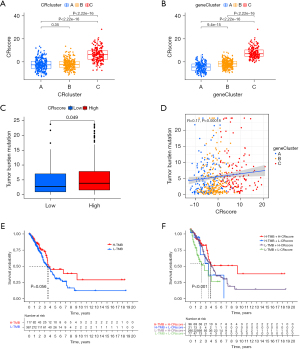
We also analyzed the relationship between the TMB of LUAD and the CR score to explore the relationship between the CR score and tumor occurrence and development. Tumors with a high CR score showed a high TMB (Figure 8C). In other words, the CR score was positively correlated with the TMB (P<0.05; r=0.17) (Figure 8D). The survival curves of the TMB and CR score show that there was no significant difference in survival between the high and low TMB groups (Figure 8E), but the TMB combined with the CR score predicted a significant difference in survival (Figure 8F). Patients with a high TMB and a high CR score had a longer survival time. It can be speculated that combining the CR score with the TMB can enhance the sensitivity of TMB to forecast the effectiveness of immunotherapy in patients with LUAD.
The CR score as a prognostic biomarker
There were no significant differences in the clinical features (except for LUAD stage) and CR score (Figure 9A-9H). Stage I−II LUAD accounted for 82% and 73% of samples in the high and low CR score groups, respectively, and stage III−IV LUAD accounted for 18% and 27% of samples in the high and low CR score groups, respectively (Figure 9A). The quantitative analysis showed that the CR score was significantly different between stage I−II LUAD and stage III−IV LUAD (P<0.05) (Figure 9E). Female patients who were deceased and ≤65 years of age accounted for a large proportion of the high CR score group, and male patients who survived and were >65 years of age accounted for a large proportion of the low CR score group (Figure 9B-9D). According to the quantitative analysis, the CR score of patients with stage I−II LUAD was higher than that of patients with stage III−IV LUAD (Figure 9A). The survival status of patients in the high and low CR score groups with the same clinical characteristics was analyzed to evaluate the universality of the CRscore tool. By comparing the survival status of the two groups for each clinical feature, we identified that patients with higher CR scores had better survival (Figure 10A-10H). Although the P value of patients with stage III–IV LUAD was >0.05, the usefulness of the CRscore tool in predicting prognosis should not be underestimated (Figure 10H). Next, the univariate and multivariate Cox regression analyses were performed for the CR score and clinical characteristics (LUAD stage, gender, and age). Both the univariate (Figure 11A) and multivariate (Figure 11B) analyses showed that age, stage, and CR score were independent prognostic factors in this study.
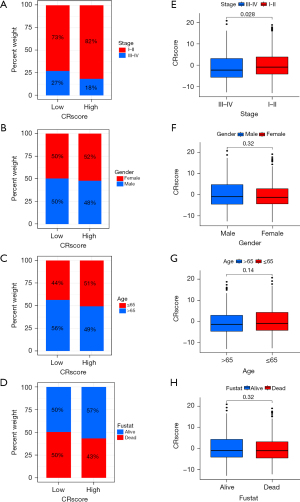
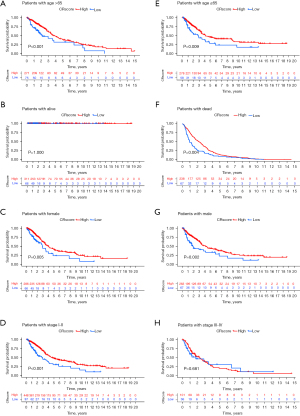
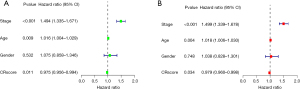
Immunotherapy for LUAD based on the CR score
Based on the CR score, we evaluated the differences in the expression of four common immune checkpoint proteins (PD-1, PD-L1, PD-L2, and CTLA-4). The expression of immune checkpoint proteins was inversely related to high and low CR scores. In other words, the expression of immune checkpoint proteins was low in patients with high CR scores and high in patients with low CR scores (Figure 12A-12D). On the basis of the CR score, the IPS of LUAD was analyzed to predict its immunogenicity. Patients with high CR scores had higher IPS and IPS-CTLA4 scores (Figure 12E-12H). These results suggest that patients with LUAD with high CR scores may have a good response to CTLA4 immunotherapy (24). We analyzed the relationship between the CR score and the sensitivity of chemotherapy agents, which are used to treat LUAD, including cisplatin, gemcitabine, paclitaxel, vinorelbine, and methotrexate. Patients with high CR scores were sensitive to cisplatin, gemcitabine, paclitaxel, and vinorelbine (P<0.05) (Figure 13A-13D), and patients with low CR scores were sensitive to methotrexate (P<0.05) (Figure 13E). These results suggest that the CRscore tool is a dependable biological index of prognostic immunotherapy and clinical efficacy.
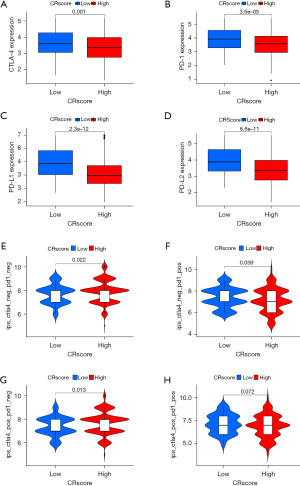
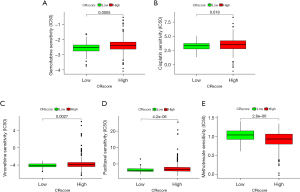
Clinical validation of CRRGs
To validate the accuracy of our CRscore tool and the strictness of the conclusions, we conducted a clinical trial on NPAS2, which was one of the CRRGs under scrutiny. We compared the expression of NPAS2 protein in LUAD tissues. As expected, western blot showed that NPAS2 was significantly elevated in LUAD tissues compared with healthy lung tissues (P<0.05) (Figure 14A,14B). Furthermore, we compared the expression of NPAS2 mRNA in LUAD tissues. It was more highly expressed in LUAD tissues compared with healthy lung tissues according to qRT-PCR (P<0.05) (Figure 14C).
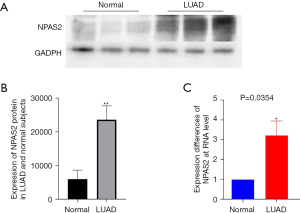
Discussion
There are many good prognostic markers such as runt-related transcription factor 3 (RUNX3), estrogen receptor and chemokine receptor. Meanwhile, Kruppel-like factor 6 (KLF6) and histone deacetylases (HDACs) have been demonstrated as poor prognostic markers (19). Although many genetic targets for predicting LUAD have been found, the prognosis of LUAD is still not optimistic, with the mortality rate as high as 18.4%. Therefore, it is very necessary to find specific targets and markers for LUAD.
The CR is a natural internal homeostatic mechanism that regulates the physiological light-dark cycle. Disruption of systemic and tissue-specific circadian mechanisms leads to changes in cell function, such as metabolism and cell division, both of which are highly associated with cancer (25). Pharmacological regulation of core CRRGs is a new approach for cancer treatment, and integrating circadian biology into cancer research offers new options for more effective cancer prevention, diagnosis, and treatment. Therefore, CRRGs may be a potential prognostic marker for LUAD.
In this study, we found that the expression of CRRGs in samples from patients with LUAD was high under our preliminary exploration of the TCGA database. We speculate that CRRGs play an essential role in the occurrence and development of LUAD. We conducted an in-depth analysis of CRRDs in LUAD samples and established a CR scoring system (CRscore). We combined the CRscore tool with the expression of CRRGs, clinical features, TMB, and immune cell infiltration. As expected, the CR score was markedly associated with tumor mutations, immune cell infiltration, LUAD stage, and gender. Moreover, we showed that patients with higher CR scores had better survival. Encouragingly, the results were also tenable when we analyzed patients who have clinical characteristics uniformly to reduce the influence of other factors. The CR score was an independent prognostic factor according to the results of the univariate and multivariate Cox regression analyses.
Seven genes (NR1D2, AANAT, CRY1, NPAS2, CSNK1E, PER3, and CRY2) were significantly different between the LUAD group and the healthy group (P<0.001). In the subsequent genotyping group of prognosis-associated CRRGs, the expression of these seven CRRGs was also significantly different among the three groups (P<0.05). Therefore, we speculate that CRRGs play an essential role in the occurrence and development of LUAD. During the CR cluster analysis, the order of survival was CRcluster C > CRcluster B > CRcluster A. Coincidentally, the same conclusion was drawn among each CR gene cluster, as follows: gene cluster C > gene cluster B > gene cluster A. We hypothesized that the CR score was inextricably related to patient survival. This conjecture was confirmed in the subsequent analysis. As can be seen from the relationship between the CR score and the TMB, the CR score was positively correlated with the TMB. We speculated that high and low CR scores would reveal an anti-tumor process and tumor cell proliferation, respectively. A series of analyses on the gene population confirmed this speculation.
As can be seen from the survival analysis, the lower the CR score, the poorer the survival and the higher the tumor malignancy. Further, we detected four common immune checkpoint proteins and predicted their immunogenicity. We suggest that CTLA-4 immunotherapy is more suitable for patients with high CR scores. The sensitivity analysis of common chemotherapeutic drugs showed that most chemotherapeutic drugs were more effective in patients with high CR scores. From another perspective, we also know that the higher the CR score, the better the prognosis.
Although the CR score is a prognostic guide and a positive predictor of prognosis in patients with LUAD, some limitations of this study still need to be considered. First, the samples were obtained from public databases, which may have led to selection bias. Second, CRRGs in the database were transcribed from tumor tissues, making it improbable to recognize where the CRRGs identified in this study came from. Finally, not all patients with high CR scores will gain greater immunotherapy benefits, so more clinical factors need to be added to the prediction model to improve its accuracy.
In conclusion, we elucidated the significance of CRRGs in clinical practice, immune infiltration, and immunotherapy and gained several important insights. Our findings may guide the selection of combination strategies or lead to the manufacture of new immunotherapy drugs in the future. Our results provide new ideas for improving the clinical response of patients to immunotherapy, exploring new therapeutic targets, and promoting personalized cancer immunotherapy in the future.
Acknowledgments
Funding: This work was supported by Nantong Municipal Bureau of Science and Technology (No. #MS12021070).
Footnote
Reporting Checklist: The authors have completed the TRIPOD reporting checklist. Available at https://jtd.amegroups.com/article/view/10.21037/jtd-22-1112/rc
Conflicts of Interest: All authors have completed the ICMJE uniform disclosure form (available at https://jtd.amegroups.com/article/view/10.21037/jtd-22-1112/coif). The authors have no conflicts of interest to declare.
Ethical Statement: The authors are accountable for all aspects of the work in ensuring that questions related to the accuracy or integrity of any part of the work are appropriately investigated and resolved. The study was conducted in accordance with the Declaration of Helsinki (as revised in 2013).
Open Access Statement: This is an Open Access article distributed in accordance with the Creative Commons Attribution-NonCommercial-NoDerivs 4.0 International License (CC BY-NC-ND 4.0), which permits the non-commercial replication and distribution of the article with the strict proviso that no changes or edits are made and the original work is properly cited (including links to both the formal publication through the relevant DOI and the license). See: https://creativecommons.org/licenses/by-nc-nd/4.0/.
References
- Li S, Zhang L. Circadian Control of Global Transcription. Biomed Res Int 2015;2015:187809. [Crossref] [PubMed]
- Sulli G, Lam MTY, Panda S. Interplay between Circadian Clock and Cancer: New Frontiers for Cancer Treatment. Trends Cancer 2019;5:475-94. [Crossref] [PubMed]
- Ercolani L, Ferrari A, De Mei C, et al. Circadian clock: Time for novel anticancer strategies? Pharmacol Res 2015;100:288-95. [Crossref] [PubMed]
- Sulli G, Manoogian ENC, Taub PR, et al. Training the Circadian Clock, Clocking the Drugs, and Drugging the Clock to Prevent, Manage, and Treat Chronic Diseases. Trends Pharmacol Sci 2018;39:812-27. [Crossref] [PubMed]
- Hrushesky WJ, Bjarnason GA. Circadian cancer therapy. J Clin Oncol 1993;11:1403-17. [Crossref] [PubMed]
- Shostak A. Circadian Clock, Cell Division, and Cancer: From Molecules to Organism. Int J Mol Sci 2017;18:873. [Crossref] [PubMed]
- Kelleher FC, Rao A, Maguire A. Circadian molecular clocks and cancer. Cancer Lett 2014;342:9-18. [Crossref] [PubMed]
- Papagiannakopoulos T, Bauer MR, Davidson SM, et al. Circadian Rhythm Disruption Promotes Lung Tumorigenesis. Cell Metab 2016;24:324-31. [Crossref] [PubMed]
- Bray F, Ferlay J, Soerjomataram I, et al. Global cancer statistics 2018: GLOBOCAN estimates of incidence and mortality worldwide for 36 cancers in 185 countries. CA Cancer J Clin 2018;68:394-424. [Crossref] [PubMed]
- Yang D, Ma Y, Zhao P, et al. Systematic screening of protein-coding gene expression identified HMMR as a potential independent indicator of unfavorable survival in patients with papillary muscle-invasive bladder cancer. Biomed Pharmacother 2019;120:109433. [Crossref] [PubMed]
- Zhang X, Gao F, Li N, et al. Peroxiredoxins and Immune Infiltrations in Colon Adenocarcinoma: Their Negative Correlations and Clinical Significances, an In Silico Analysis. J Cancer 2020;11:3124-43. [Crossref] [PubMed]
- Chen S, Yang D, Lei C, et al. Identification of crucial genes in abdominal aortic aneurysm by WGCNA. PeerJ 2019;7:e7873. [Crossref] [PubMed]
- Sun G, Sun K, Shen C. Human nuclear receptors (NRs) genes have prognostic significance in hepatocellular carcinoma patients. World J Surg Oncol 2021;19:137. [Crossref] [PubMed]
- Subramanian A, Tamayo P, Mootha VK, et al. Gene set enrichment analysis: a knowledge-based approach for interpreting genome-wide expression profiles. Proc Natl Acad Sci U S A 2005;102:15545-50. [Crossref] [PubMed]
- Li M, Chen Z, Jiang T, et al. Circadian rhythm-associated clinical relevance and Tumor Microenvironment of Non-small Cell Lung Cancer. J Cancer 2021;12:2582-97. [Crossref] [PubMed]
- Zhang LH, Li LQ, Zhan YH, et al. Identification of an IRGP Signature to Predict Prognosis and Immunotherapeutic Efficiency in Bladder Cancer. Front Mol Biosci 2021;8:607090. [Crossref] [PubMed]
- Barbie DA, Tamayo P, Boehm JS, et al. Systematic RNA interference reveals that oncogenic KRAS-driven cancers require TBK1. Nature 2009;462:108-12. [Crossref] [PubMed]
- Charoentong P, Finotello F, Angelova M, et al. Pan-cancer Immunogenomic Analyses Reveal Genotype-Immunophenotype Relationships and Predictors of Response to Checkpoint Blockade. Cell Rep 2017;18:248-62. [Crossref] [PubMed]
- Lu M, Fan X, Liao W, et al. Identification of significant genes as prognostic markers and potential tumor suppressors in lung adenocarcinoma via bioinformatical analysis. BMC Cancer 2021;21:616. [Crossref] [PubMed]
- Liu J, Wu Z, Wang Y, et al. A prognostic signature based on immune-related genes for cervical squamous cell carcinoma and endocervical adenocarcinoma. Int Immunopharmacol 2020;88:106884. [Crossref] [PubMed]
- Song C, Guo Z, Yu D, et al. A Prognostic Nomogram Combining Immune-Related Gene Signature and Clinical Factors Predicts Survival in Patients With Lung Adenocarcinoma. Front Oncol 2020;10:1300. [Crossref] [PubMed]
- An J, Lai J, Sajjanhar A, et al. J-Circos: an interactive Circos plotter. Bioinformatics 2015;31:1463-5. [Crossref] [PubMed]
- Chen Y, Miao S, Zhao W. Identification and validation of significant gene mutations to predict clinical benefit of immune checkpoint inhibitors in lung adenocarcinoma. Am J Transl Res 2021;13:1051-63. [PubMed]
- Yi M, Li A, Zhou L, et al. Immune signature-based risk stratification and prediction of immune checkpoint inhibitor's efficacy for lung adenocarcinoma. Cancer Immunol Immunother 2021;70:1705-19. [Crossref] [PubMed]
- Bass J, Takahashi JS. Circadian integration of metabolism and energetics. Science 2010;330:1349-54. [Crossref] [PubMed]
(English Language Editor: J. Jones)