The development and validation of a m6A-lncRNAs based prognostic model for overall survival in lung squamous cell carcinoma
Introduction
Lung cancer is the leading cause of death among all the cancers, and accounts for 23–24% of all cancer-related deaths (1,2). Small cell lung cancer (SCLC) and non-small cell lung cancer (NSCLC) are the 2 main pathological types of lung cancer. NSCLC accounts for 85% of all lung cancers and is mainly classified into lung adenocarcinoma (LUAD) and lung squamous cell carcinoma (LUSC) (3). The treatment of LUSC, which constitutes about 40% of NSCLCs, is still facing great challenges and the prognosis of LUSC patients remains poor (4). The prognostic value of the tumor node metastasis (TNM) stage system is widely used for LUSC, but is limited, as patients with the same TNM stage have been shown to have different survival outcomes (5,6). More and more gene mutations have been identified as biomarkers for the diagnosis and prognosis of LUAD patients (7), such as EGFR, ALK, and ROS1. However, to date, no biomarkers have been identified for the diagnosis and prognosis of LUSC (8).Therefore, biomarkers that can provide diagnostic and prognostic information of LUSC is warranted.
The N6-methylandenosine (m6A) modification is the most common methylated modification of messenger ribonucleic acid (mRNA). It plays a vital role in many biological processes, including mRNA processing, mRNA exporting, mRNA translation and the synthesis of long non-coding RNA (lncRNA), and micro RNA (miRNA) (9,10). m6A modification is regulated by 3 types of regulators; that is, methyltransferases, signal transducers, and demethylases (11). There is increasing evidence that m6A has great effects on tumor development, including increasing the self-renewal of cancer stem cells, promoting cell proliferation, and increasing resistance to radiotherapy and chemotherapy (12-15). Research has shown that the knockdown of the m6A-related gene, FTO, inhibits the proliferation and invasion of LUSC (16).
The dysregulation of lncRNA appears to play an important role in the development of a number of cancers, including prostate cancer, breast cancer, liver cancer, and lung cancer (17). For example, lncRNA MALAT1 is overexpressed in lung cancer, breast cancer, bladder carcinoma, and ovarian cancer and is a potential prognostic biomarker and therapeutic target (18,19). MALAT1 also promotes the proliferation of lung cancer and is associated with gefitinib resistance (20). It has been reported that risk models based on m6A-related lncRNAs help to predict survival in cancers, including colon cancer (21), pancreatic ductal adenocarcinoma (22), and osteosarcoma (23). A 31 m6A-associated lncRNAs signature showed a strong predictive power for survival in colon cancer patients, with a area under the curve (AUC) of 0.855–0.883 (21). A six m6A-lncRNAs risk model showed a AUC of 0.787–0.816 for predicting survival in osteosarcoma (23). However, very few studies have reported m6A-related lncRNA risk models in LUSC. Though a four lncRNAs signature was reported to be a prognostic biomarker in non-small cell lung cancer, the signature was not validated independently in LUSC data set (24).
In the current study, we aimed to construct a m6A-lncRNA related prognostic model for survival in LUSC. We identified 13 prognostic m6A-related lncRNAs in LUSC based on The Cancer Genome Atlas (TCGA) database. And 7 of these m6A-related lncRNAs showed to have independent prognostic value, and were used to construct a risk-score model using the bioinformatic method. and the prognostic value of the risk-score model for overall survival (OS) was validated. We present the following article in accordance with the TRIPOD reporting checklist (available at https://jtd.amegroups.com/article/view/10.21037/jtd-22-1185/rc).
Methods
Data extraction and m6A-related gene identification
The study was conducted in accordance with the Declaration of Helsinki (as revised in 2013). The RNA-sequencing data and clinical information of LUSC patients were downloaded from TCGA database (https://portal.gdc.cancer.gov/) in July 2021. In total, 504 cases were downloaded in this study. The OS and other clinical characteristic parameters, including age, gender, T stage, N stage, M stage and clinical stage, were extracted. OS was defined as the time interval from diagnosis to death for any reason. Patients with no survival time data were excluded. The expression of 21 m6A-related genes were extracted, including the expression data of writers (METTL3, METTL14, METTL16, WTAP, VIRMA, ZC3H13, RBM15, and RBM15B), readers (YTHDC1, YTHDC2, YTHDF1, YTHDF2, YTHDF3, HNRNPC, FMR1, HNRNPA2B1, IGFBP1, IGFBP2, and RBMX), and erasers (FTO and ALKBH5) (25). Next, the expression data of the m6A-related lncRNAs were extracted.
Annotation of lncRNAs and identification of m6A-related lncRNAs
Genome Reference Consortium Human Build 38 (GRCh38) was downloaded from GENCODE (https://www.gencodegenes.org/human/) and used to annotate the lncRNAs in the data sets from TCGA. In total, 14,086 lncRNAs were identified. A Pearson correlation analysis was performed to identify the m6A-related lncRNAs. A univariate Cox regression analysis was used to filter m6A-related the lncRNAs with prognostic value. In total, 13 prognostic m6A-related lncRNAs were identified.
Clustering of prognostic m6A-related lncRNAs
The ConsensusClusterPlus algorithm (26) was used to cluster the prognostic m6A-related lncRNAs. The prognostic lncRNAs were classified into 2 clusters. The survival, clinical characteristics, immune checkpoint genes, immune cell infiltration in the tumor microenvironment, immune scores, and stromal scores between the 2 clusters were compared. Patients with incomplete parameter information were excluded. A gene set enrichment analysis (GSEA) was performed to analyze the genes enriched in the 2 clusters.
Construction and validation of m6A-related lncRNA risk model
Half of the patients (totally 248 patients) from TCGA database were randomly chosen as the training set. The “glmnet” package of R software (R x64 v4.0.5, https://www.r-project.org/) was used to perform the least absolute shrinkage and selection operator (LASSO) Cox regression analysis (27). Seven m6A-related lncRNAs were identified to have independent prognostic value to OS and these lncRNAs were used to construct a 7 m6A-related lncRNA prognostic risk-score model. The risk-score model was calculated using the following formula:
Risk score = 0.2996 × Exp (AP001189.3) – 0.2655 × Exp (AC254562.3) – 0.3442 × Exp (AC138035.1) – 0.1882 × Exp (AC010422.4) – 0.5289 × Exp (AL591686.1) + 0.4162 × Exp (WT1-AS) – 0.0858 × Exp (AP001469.3)
The risk scores of all the patients were calculated. Half of the patients were randomly chosen as the training set and were classified into high- and low-risk groups using the median risk score as cutoff value. The receiver operating characteristic (ROC) curves were plotted and the AUCs were calculated. The clinical characteristics, immune infiltration cell in the tumor environment, immune checkpoint genes, and survival in the high- and low-risk groups were compared. Patients with incomplete parameter information were excluded. The risk-score model was validated in the total TCGA data sets. The independent prognostic value of the risk model was tested by univariate and multivariate cox regression analyses.
Construction of ceRNA network
The target miRNAs of the prognostic m6A-related lncRNAs were predicted using the lncBase V.2 (http://carolina.imis.athena-innovation.gr/diana_tools/web/index.php?r=lncbasev2%2Findex). The target miRNAs with the highest scores and that had at least 2 lncRNAs in common were included in the ceRNA network. The target mRNAs of these miRNAs were predicted by Tarbase V.8 (http://carolina.imis.athena-innovation.gr/diana_tools/web/index.php?r=tarbasev8%2Findex). The mRNAs with the top 10 highest predicted scores were included in the competing endogenous RNA (ceRNA) network. The “cytoscape” software (v 3.8.3) was used to plot the ceRNA network (28). The functional and pathway enrichment analyses of the target mRNAs included in the ceRNA network were performed using “Metascape” (https://metascape.org) (29).
Statistical analysis
Survival variations between the different groups were compared using Kaplan-Meier curves and tested by log-rank tests. The risk scores between different groups of clinical characteristics were compared and tested by the Wilcox test. A Pearson correlation analysis was used to examine the correlations between the lncRNAs and the m6A-related genes. A univariate and multivariate Cox regression analysis was used to validate the prognostic value of the m6A-related lncRNAs and the risk-score model. The prognostic ability of the risk-score model was examined by the ROC curves and the AUCs were calculated. R software (v4.0.5) was used to perform the statistical analysis. Results were considered to be statistically significant if P<0.05.
Results
Identification of prognostic m6A-related lncRNAs
The data of 504 LUSC patients with clinical and RNA-sequencing information were downloaded from TCGA database. The data of 8 patients with no survival time data were excluded; thus, ultimately, 496 patients were included in the study. The patients’ characteristics are set out in Table 1. In total 14,086 lncRNAs were identified. Data on the expression of 21 m6A-related genes (i.e., METTL3, METTL14, METTL16, WTAP, VIRMA, ZC3H13, RBM15, RBM15B, YTHDC1, YTHDC2, YTHDF1, YTHDF2, YTHDF3, HNRNPC, FMR1, HNRNPA2B1, IGFBP1, IGFBP2, RBMX, FTO, and ALKBH5) were extracted (available at https://cdn.amegroups.cn/static/public/jtd-22-1185-1.xls). A Pearson correlation analysis was performed to examine the correlations between the lncRNAs and the m6A-related genes. A total of 670 lncRNAs with a |Pearson R| value >0.35 and a P value <0.001 were identified as the m6A-related lncRNAs (see Figure 1A and available at https://cdn.amegroups.cn/static/public/jtd-22-1185-2.xls). In total, 13 m6A-related lncRNAs (i.e., AC243919.2, AC254562.3, AC138035.1, AC010422.4, AC107884.1, AL591686.1, HORMAD2-AS1, DSCR9, PRC1-AS1, AP001189.3, AL122125.1, and WT1-AS, AP001469.3) were identified as prognostic lncRNAs by the univariate Cox regression analysis. A forest plot and heatmap were used to visualize the hazard ratios and expression patterns of the prognostic m6A-related lncRNAs, respectively (see Figure 1B,1C).
Table 1
Characteristics | N (%) |
---|---|
Age (years) | |
≤65 | 190 (37.70) |
>65 | 305 (60.52) |
Unknown | 9 (1.78) |
Gender | |
Female | 131 (25.99) |
Male | 373 (74.01) |
T | |
1 | 114 (22.62) |
2 | 295 (58.53) |
3 | 71 (14.09) |
4 | 24 (4.76) |
N | |
0 | 320 (63.49) |
1 | 133 (26.39) |
2 | 40 (7.94) |
3 | 5 (0.99) |
Unknown | 6 (1.19) |
M | |
0 | 414 (82.14) |
1 | 7 (1.39) |
Unknown | 83 (16.47) |
Stage | |
I | 245 (48.61) |
II | 163 (32.34) |
III | 85 (16.87) |
IV | 7 (1.39) |
Unknown | 4 (0.79) |
Total | 504 (100.00) |
T, tumor; N, node; M, metastasis.
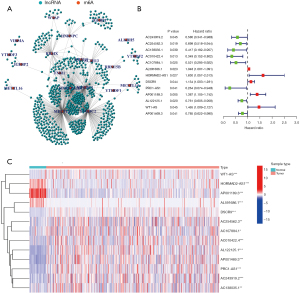
M6A-related lncRNA cluster analysis and the correlation to clinical characteristics
An unsupervised cluster analysis of the prognostic m6A-related lncRNAs was performed using the ConsensusClusterPlus algorithm (26). The patients were classified into 2 clusters. The clinical characteristics and m6A-related lncRNA expressions of the 2 clusters of patients were analyzed. A heatmap was used to visualize the results (see Figure 2A). The survival analysis showed that the OS of patients in cluster A was significantly better than that of patients in cluster B (P<0.001; see Figure 2B). We also compared the expression of programmed death-ligand 1 (PD-L1), programmed death-ligand 2 (PD-L2), and cytotoxic T-lymphocyte-associated protein 4 (CTLA4) between the 2 clusters. Patients in cluster B had higher expressions of PD-L1, PD-L2, and CTLA4 (for PD-L1, P<0.01; for PD-L2, P<0.001; for CTLA4, P<0.001; see Figure 2C-2E). The correlations between PD-L1, PD-L2, and CTLA4 and the prognostic m6A-related lncRNAs were also explored. PD-L1 was positively correlated with AL591686.1 and DSCR9, and negatively correlated with AC254562.3. PD-L2 was positively correlated with AP001189.3, and negatively correlated with AC243919.2, AC254562.3, AC138035.1, PRC1-AS1, AL122125.1, and AP001469.3. CTLA4 was positively correlated with AC010422.4, AC107884.1, HORMAD2-AS1, and AP001189.3, and negatively correlated with AL122125.1 and AP001469.3 (see Figure 2F).
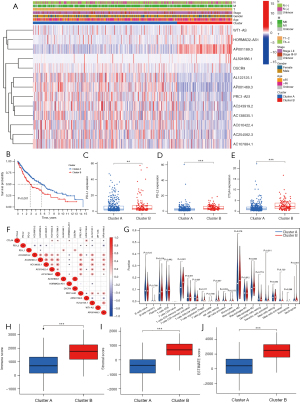
Correlation between the m6A-related lncRNA clusters and the tumor microenvironment
The immune cell infiltration of the tumor microenvironment was analyzed. In comparison with cluster A, cluster B had a higher infiltration level of resting memory cluster of differentiation (CD)4 T cells (P<0.001), M2 macrophages (P=0.008), resting mast cells (P=0.05), and neutrophils (P<0.001), and a lower infiltration level of follicular helper T cells (P<0.001) (see Figure 2G). The Estimation of Stromal and Immune cells in Malignant Tumor tissues using Expression data (ESTIMATE) (30) algorithm was used to calculate the immune and stomal scores. It was shown that immune score, stromal score and ESTIMATE score were higher in cluster B than cluster A (P<0.001; see Figure 2H-2J).
Prognostic m6A-related lncRNA associated pathways identified by the GSEA
A GSEA was performed to identify the signaling pathways associated with the m6A-related lncRNAs. It was shown that 62 gene sets were significantly enriched in cluster B and 34 gene sets were significantly enriched in cluster A. Of the enriched pathways in cluster A and cluster B, 10 are shown in Figure 3A,3B. The signaling pathways enriched in cluster B included the cytokine-cytokine receptor interaction, leukocyte transendothelial migration, the JAK_STAT signaling pathway, ECM receptor interaction, natural killer mediated cytotoxicity, chemokine signaling pathway, the Toll-like receptor signaling pathway, antigen processing and presentation, intestinal immune network for immunoglobulin A (IgA) production, and the MAPK signaling pathway. The signaling pathways enriched in cluster A included the homologous recombination, oxidative phosphorylation, RNA polymerase, mismatch repair, pyrimidine metabolism, basal transcription factors, cell cycle, glutathione metabolism, proteasome, and aminoacyl transfer RNA biosynthesis pathways. The enrichment score of each pathway is shown in Table 2.
Table 2
Pathways | ES | NES | NOM P value | FDR q value |
---|---|---|---|---|
Pathway enriched in cluster B | ||||
KEGG_CYTOKINE_CYTOKINE_RECEPTOR_INTERACTION | 0.71 | 2.56 | 0 | 0 |
KEGG_LEUKOCYTE_TRANSENDOTHELIAL_MIGRATION | 0.7 | 2.45 | 0 | 0 |
KEGG_JAK_STAT_SIGNALING_PATHWAY | 0.6 | 2.32 | 0 | 0 |
KEGG_ECM_RECEPTOR_INTERACTION | 0.79 | 2.31 | 0 | 0 |
KEGG_NATURAL_KILLER_CELL_MEDIATED_CYTOTOXICITY | 0.64 | 2.31 | 0 | 0 |
KEGG_CHEMOKINE_SIGNALING_PATHWAY | 0.63 | 2.23 | 0 | 0 |
KEGG_TOLL_LIKE_RECEPTOR_SIGNALING_PATHWAY | 0.59 | 2.18 | 0.002 | 0.001 |
KEGG_ANTIGEN_PROCESSING_AND_PRESENTATION | 0.68 | 2.16 | 0.002 | 0.001 |
KEGG_INTESTINAL_IMMUNE_NETWORK_FOR_IGA_PRODUCTION | 0.86 | 2.15 | 0 | 0.001 |
KEGG_MAPK_SIGNALING_PATHWAY | 0.44 | 1.8 | 0 | 0.022 |
Pathway enriched in cluster A | ||||
KEGG_HOMOLOGOUS_RECOMBINATION | –0.83 | –2.21 | 0 | 0.001 |
KEGG_OXIDATIVE_PHOSPHORYLATION | –0.7 | –2.14 | 0.002 | 0.004 |
KEGG_RNA_POLYMERASE | –0.72 | –2.11 | 0 | 0.004 |
KEGG_MISMATCH_REPAIR | –0.81 | –2.1 | 0.002 | 0.003 |
KEGG_PYRIMIDINE_METABOLISM | –0.58 | –2.02 | 0 | 0.006 |
KEGG_BASAL_TRANSCRIPTION_FACTORS | –0.64 | –2.02 | 0.004 | 0.006 |
KEGG_CELL_CYCLE | –0.57 | –1.86 | 0.027 | 0.022 |
KEGG_GLUTATHIONE_METABOLISM | –0.54 | –1.76 | 0.022 | 0.039 |
KEGG_PROTEASOME | –0.67 | –1.89 | 0.014 | 0.019 |
KEGG_AMINOACYL_TRNA_BIOSYNTHESIS | –0.64 | –1.88 | 0.016 | 0.018 |
ES, Enrichment Score; NES, Normalized Enrichment Score; NOM, Nominal; FDR, false discovery rate.
Construction of the m6A-related lncRNA risk-score model in the training set
In total, 248 patients were randomly chosen for the training set (23). In the database, 106 patients in the training set were recorded as dead. Based on the 13 m6A-related prognostic lncRNAs, LASSO Cox regression was performed to construct the m6A-related lncRNA prognostic risk model for the training set patients. In total, 7 m6A-related lncRNAs (i.e., AP001189.3, AC254562.3, AC138035.1, AC010422.4, AL591686.1, WT1-AS, and AP001469.3) and the corresponding coefficients were generated (see Figure 4A-4C). Patients in the training set were divided into high- and low-risk groups using the median risk score as the cut-off value. Kaplan-Meier survival curves of the low- and high-risk groups were plotted, and showed that patients in the high-risk group had a worse prognosis than patients in the low-risk group (log-rank test, P<0.001; see Figure 4D). The ROC curve of the training showed that the risk-score model had an ability to predict 1-year OS in the training set with an AUC of 0.639 (see Figure 4E). The heatmap of the 7 m6A-related lncRNAs, risk score, and survival distributions of the training set are shown in Figure 4F-4H.
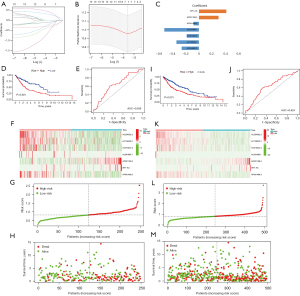
Validation of the m6A-related lncRNA risk-score model
The prognostic value of the m6A-related lncRNA risk-score model was validated in the whole TCGA-LUSC data set. The LUSC patients were classified into low- and high-risk groups according to their risk scores. Patients in the high-risk group had worse survival than patients in the low-risk group (log-rank test, P<0.001; see Figure 4I). The ROC curve of TCGA-LUSC data set indicated that the risk-score model had prognostic value with an AUC of 0.624 (see Figure 4J). The heatmap of the 7 m6A-related lncRNAs, risk score, and the survival distributions of TCGA-LUSC data set are shown in Figure 4K-4M. We also validated the prognostic value of each lncRNA included in the risk-score model. The results showed that patients with higher expressions of AC010422.4, AC138035.1, AC254562.3, and AP001469.3 had better survival, and patients with higher expressions of AP001189.3 and WT1-AS had worse survival (see Figure 5A-5F). These results indicated that AC010422.4, AC138035.1, AC254562.3, and AC001469.3 were predictors of favorable survival outcomes while AP001189.3 and WT1-AS were predictors of poor survival outcomes.
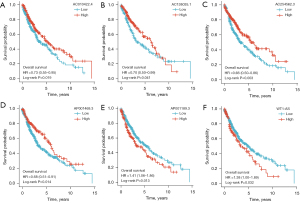
Correlations between the m6A-related lncRNA risk-score model and the clinicopathological characteristics
We analyzed whether the m6A-related lncRNA risk-score model was related to any of the clinicopathological characteristics. A heatmap was used to visualize the expression of the 7 m6A-related lncRNAs and the clinicopathological characteristics (see Figure 6A). The results showed that the patients in cluster B, with high immune scores and stage III–IV disease had higher risk scores than patients in cluster A, with lower immune scores and stage I−II disease (see Figure 6B-6D). However, risk scores were not related to age, gender, T stage, lymph node invasion, or metastasis (see Figure S1). Patients in the high-risk group also had increased expressions of PD-L1, PD-L2, and CTLA4 (see Figure 6E-6G). We also analyzed the relationship between risk scores and different immune cells. The results showed that risk scores were positively correlated with M2 macrophages, neutrophils, active memory CD4 T cells, resting memory CD4 T cells, and γδ T cells. Naïve B cells, active natural killer cells, follicular helper T cells were negatively correlated with risk scores (see Figure 6H-6O).
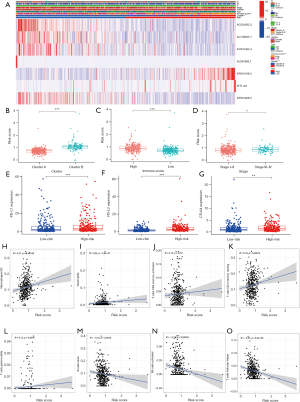
Prognostic analysis of the m6A-related lncRNA risk-score model
Univariate and multivariate Cox regression analyses of the training set showed that the risk score had independent prognostic value for OS [for the univariate analysis, hazard ratio =3.140 (1.822−5.412), P<0.001; for the multivariate analysis, hazard ratio =3.053 (1.781−5.233), P<0.001; see Figure 7A,7B]. The validation of the prognostic value in TCGA-LUSC data set showed consistent results, with a hazard ratio of 1.975 (1.386−2.813) (P<0.001) in the univariate Cox regression analysis and a hazard ratio of 2.059 (1.421−2.982) (P<0.001) in the multivariate Cox regression analysis (see Figure 7C,7D). To better assess the prognostic value of the risk-score model, a stratification analysis was performed to confirm whether the risk-score model retained its independent prognostic value in different subgroups. Compared to low-risk patients, high-risk patients had worse OS in both the ≤65 and >65 years subgroups, and female and male subgroups (see Figure 7E-7H). The risk-score model retained its independent prognostic value for patients with or without lymph node invasion, patients with T1–2 stage or with T3–4 stage disease, and stage I–II or stage III–IV disease (see Figure 7I-7N). These results indicated that the m6A-related lncRNA risk-score model was an independent prognostic factor for OS in LUSC patients.
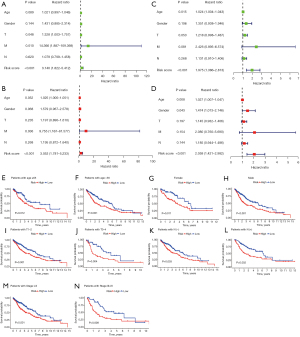
CeRNA network construction and functional enrichment analysis
A ceRNA network was constructed to analyze how the m6A-related lncRNAs sponge miRNAs and regulate mRNA expression. In total, 22 miRNAs were predicted by lncBase V.2, and 162 target mRNAs of the miRNAs were predicted by Tarbase V.8. A total of 228 interaction pairs were identified. All the above lncRNAs, miRNAs, and mRNAs were included in the ceRNA network (see Figure 8A). The target mRNAs were input in the functional enrichment analysis using the Metascape tool. The results showed that these target genes were enriched in the pathways of regulation of translation, negative regulation of protein modification process, regulation of cellular protein catabolic process, cell division, G1/S transition of mitotic cell cycle, protein-containing complex localization, RNA localization, negative regulation of growth, the PID RB1 pathway, and response to endoplasmic reticulum stress (see Figure 8B-8D).
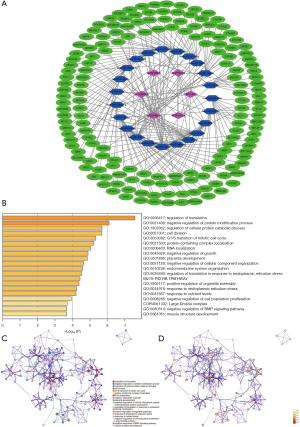
Discussion
In the current study, 13 m6A-related lncRNAs were found to have prognostic value in LUSC patients from TCGA database. Next, 7 of these lncRNAs, which had independent prognostic value for survival, were used to construct the prognostic model for OS of LUSC patients. A high-risk score was associated with worse survival. We also explored the correlations between the risk model and the clinicopathological characteristics and established the ceRNA network of 6 m6A-related lncRNAs, 22 miRNAs, and 162 target mRNAs.
There is increasing evidence that m6A modification has a dual role in the initiation and progression of tumors. The downregulation of the m6A level is related to the development of cancers, including hepatocellular carcinoma (HCC), breast cancer, endometrial cancer, pancreatic cancer, and lung cancer (31-35). However, some research has indicated that increased m6A modification promotes tumor development in some types of cancers. Specifically, increased m6A modification on SP1, BCL2, MYB, MYC, and PTEN was found to be related to the development of acute myeloid leukemia (AML) (36,37). The upregulation of the m6A level of SOCS2 promotes the progression of HCC (38).
The role of m6A methylation in lung cancer and how it relates to prognosis has been reported (31,39,40). However, little is known about the lncRNA-dependent way in which the m6A modification acts during lung cancer progression. In liver cancer, VIRMA increases the m6A methylation of lncRNA GATA3 and promotes tumor progression (41). YTHDF3 suppresses colorectal progression by negatively regulating the m6A modification of lncRNA GAS5 and causing YAP degradation (42). METTL14 inhibits colon cancer progression by decreasing the m6A methylation of lncRNA XIST (43). The above research revealed that the m6A methylation of lncRNAs plays a vital role in the initiation and progression of cancer. Thus, we directed our attention to the interactions between lncRNA and m6A methylation and tried to discover its prognostic value in LSUC.
We identified 13 prognostic m6A-related lncRNAs and 7 of these lncRNAs, which had independent prognostic value, were used to establish the risk-score model; that is, AC254562.3, AC138035.1, AC010422.4, AL591686.1, AP001189.3, WT1-AS and AP001469.3, with the respective coefficients of –0.2655, –0.3442, –0.1882, –0.5289, 0.2996, 0.4162, and –0.0858. The coefficients indicated that WT1-AS and AP001189.3, which increased the risk score, were associated with poor survival. The other 5 lncRNAs, which decreased the risk score, were favorably associated with survival. Similarly, higher expressions of AC010422.4, AC138035.1, AC254562.3, and AC001469.3 were associated with favorable survival outcomes, and higher expressions of AC001189.3 and WT1-AS were associated with unfavorable survival outcomes. Patients with advanced stage disease, who usually had a worse prognosis, had higher risk scores, which supports the prognostic values of the risk model. It should be noticed that in the current study, the AUC of the 7 m6A-related lncRNAs prognostic model was 0.639. Commonly, the model was considered to have a mild predictive value if the AUC is between 0.5 and 0.7, and moderate predictive value if it is between 0.7 and 0.85, and if the AUC was larger than 0.85, the model was considered to have a strong predictive value. Though the 7 m6A-related lncRNAs prognostic model in the current study only have mile predictive value to OS, it was an independent prognostic factor and can help to judge the prognosis to a certain extent.
A ceRNA network was constructed to explore the interaction of lncRNAs, miRNAs, and the target mRNAs. Next, an enrichment analysis of the target mRNA was performed, and we found that the target mRNAs were enriched in pathways involved in the regulation of mRNA translation, protein modification, cell cycle, cell growth, etc. These results may provide some clues for further explorations of the underlying mechanism of m6A methylation of lncRNAs.
We also analyzed the correlations between risk scores and immune checkpoint expression and the immune environment. We found that patients with high risk scores had higher expressions of PD-L1, PD-L2, and CTLA4. Our risk-score model may help to predict patients’ responses to immune therapy; however, it needs to be validated clinically.
Recently, several m6A-related risk models have been reported for lung cancer. A risk model based on 18 m6A-related lncRNAs was constructed in NSCLC and the model was shown to be an independent prognostic factor for OS in NSCLC (44). A m6A-related lncRNA model in LUAD was shown to be correlated with survival and the tumor immune microenvironment (45). Yu et al. (46) constructed a prognostic model for LUSC based on 7 m6A-related autophagy genes. The risk model had a strong power to predict prognosis in LUSC. However, no prognostic risk model based on m6A-related lncRNAs in LUSC had previously been reported. Thus, we constructed a risk model based on m6A-related lncRNAs in the current study and validated its prognostic value for OS in LUSC.
This study had a number of limitations. First, the risk model was constructed based on cases randomly chosen from TCGA database and validated by the whole TCGA-LUSC cohort. However, the risk-score model should be validated in more LUSC cohorts clinically. Second, the expression of m6A-related lncRNAs was not validated experimentally and clinically, and the interactions between the lncRNAs and m6A modification need to be confirmed by in vivo and in vitro experiments. Third, the patients used to construct the risk model were mostly from the United States of America and thus do not represent patients from other regions.
Conclusions
The 7 m6A-related lncRNA risk model may serve as a potential prognostic biomarker in LUSC, but need to be validated in more LUSC cohorts clinically.
Acknowledgments
Funding: This work was supported by the Funds of Medical Science and Technology Innovation Platform Construction Project, which was a sub-item of Foshan Science and Technology Innovation Project (Contract Nos. FSOAA-KJ218-1301-0036 and FSOAA-KJ218-1301-0037 to Yiyu Lu).
Footnote
Reporting Checklist: The authors have completed the TRIPOD reporting checklist. Available at https://jtd.amegroups.com/article/view/10.21037/jtd-22-1185/rc
Conflicts of Interest: All authors have completed the ICMJE uniform disclosure form (available at https://jtd.amegroups.com/article/view/10.21037/jtd-22-1185/coif). YL reports that this work was supported by the Funds of Medical Science and Technology Innovation Platform Construction Project, which was a sub-item of Foshan Science and Technology Innovation Project (Contract Nos. FSOAA-KJ218-1301-0036 and FSOAA-KJ218-1301-0037 to Yiyu Lu). The other authors have no conflicts of interest to declare.
Ethical Statement: The authors are accountable for all aspects of the work in ensuring that questions related to the accuracy or integrity of any part of the work are appropriately investigated and resolved. The study was conducted in accordance with the Declaration of Helsinki (as revised in 2013).
Open Access Statement: This is an Open Access article distributed in accordance with the Creative Commons Attribution-NonCommercial-NoDerivs 4.0 International License (CC BY-NC-ND 4.0), which permits the non-commercial replication and distribution of the article with the strict proviso that no changes or edits are made and the original work is properly cited (including links to both the formal publication through the relevant DOI and the license). See: https://creativecommons.org/licenses/by-nc-nd/4.0/.
References
- Siegel RL, Miller KD, Jemal A. Cancer statistics, 2019. CA Cancer J Clin 2019;69:7-34. [Crossref] [PubMed]
- Siegel RL, Miller KD, Fuchs HE, et al. Cancer statistics, 2022. CA Cancer J Clin 2022;72:7-33. [Crossref] [PubMed]
- Gao M, Kong W, Huang Z, et al. Identification of Key Genes Related to Lung Squamous Cell Carcinoma Using Bioinformatics Analysis. Int J Mol Sci 2020;21:2994. [Crossref] [PubMed]
- Chen Z, Fillmore CM, Hammerman PS, et al. Non-small-cell lung cancers: a heterogeneous set of diseases. Nat Rev Cancer 2014;14:535-46. [Crossref] [PubMed]
- Chassagnon G, Bennani S, Revel MP. New TNM classification of non-small cell lung cancer. Rev Pneumol Clin 2017;73:34-9. [Crossref] [PubMed]
- Kutob L, Schneider F. Lung Cancer Staging. Surg Pathol Clin 2020;13:57-71. [Crossref] [PubMed]
- Calvayrac O, Pradines A, Pons E, et al. Molecular biomarkers for lung adenocarcinoma. Eur Respir J 2017;49:1601734. [Crossref] [PubMed]
- Hirsch FR, Scagliotti GV, Mulshine JL, et al. Lung cancer: current therapies and new targeted treatments. Lancet 2017;389:299-311. [Crossref] [PubMed]
- Zhao BS, Roundtree IA, He C. Post-transcriptional gene regulation by mRNA modifications. Nat Rev Mol Cell Biol 2017;18:31-42. [Crossref] [PubMed]
- Dai D, Wang H, Zhu L, et al. N6-methyladenosine links RNA metabolism to cancer progression. Cell Death Dis 2018;9:124. [Crossref] [PubMed]
- Zaccara S, Ries RJ, Jaffrey SR. Reading, writing and erasing mRNA methylation. Nat Rev Mol Cell Biol 2019;20:608-24. [Crossref] [PubMed]
- Zhang S, Zhao BS, Zhou A, et al. m6A Demethylase ALKBH5 Maintains Tumorigenicity of Glioblastoma Stem-like Cells by Sustaining FOXM1 Expression and Cell Proliferation Program. Cancer Cell 2017;31:591-606.e6. [Crossref] [PubMed]
- Cui Q, Shi H, Ye P, et al. m6A RNA Methylation Regulates the Self-Renewal and Tumorigenesis of Glioblastoma Stem Cells. Cell Rep 2017;18:2622-34. [Crossref] [PubMed]
- Lin S, Choe J, Du P, et al. The m(6)A Methyltransferase METTL3 Promotes Translation in Human Cancer Cells. Mol Cell 2016;62:335-45. [Crossref] [PubMed]
- Zhang C, Zhi WI, Lu H, et al. Hypoxia-inducible factors regulate pluripotency factor expression by ZNF217- and ALKBH5-mediated modulation of RNA methylation in breast cancer cells. Oncotarget 2016;7:64527-42. [Crossref] [PubMed]
- Liu J, Ren D, Du Z, et al. m6A demethylase FTO facilitates tumor progression in lung squamous cell carcinoma by regulating MZF1 expression. Biochem Biophys Res Commun 2018;502:456-64. [Crossref] [PubMed]
- Bhan A, Soleimani M, Mandal SS. Long Noncoding RNA and Cancer: A New Paradigm. Cancer Res 2017;77:3965-81. [Crossref] [PubMed]
- Gutschner T, Hämmerle M, Eissmann M, et al. The noncoding RNA MALAT1 is a critical regulator of the metastasis phenotype of lung cancer cells. Cancer Res 2013;73:1180-9. [Crossref] [PubMed]
- Arun G, Diermeier S, Akerman M, et al. Differentiation of mammary tumors and reduction in metastasis upon Malat1 lncRNA loss. Genes Dev 2016;30:34-51. [Crossref] [PubMed]
- Feng C, Zhao Y, Li Y, et al. LncRNA MALAT1 Promotes Lung Cancer Proliferation and Gefitinib Resistance by Acting as a miR-200a Sponge. Arch Bronconeumol 2019;55:627-33. (Engl Ed). [PubMed]
- Zhang P, Liu G, Lu L. N6-Methylandenosine-Related lncRNA Signature Is a Novel Biomarkers of Prognosis and Immune Response in Colon Adenocarcinoma Patients. Front Cell Dev Biol 2021;9:703629. [Crossref] [PubMed]
- Hu Y, Chen Y. N6-methylandenosine-related lncRNAs play an important role in the prognosis and immune microenvironment of pancreatic ductal adenocarcinoma. Sci Rep 2021;11:17844. [Crossref] [PubMed]
- Zhang P, Xu K, Wang J, et al. Identification of N6-methylandenosine related LncRNAs biomarkers associated with the overall survival of osteosarcoma. BMC Cancer 2021;21:1285. [Crossref] [PubMed]
- Li A, Yu WH, Hsu CL, et al. Modular signature of long non-coding RNA association networks as a prognostic biomarker in lung cancer. BMC Med Genomics 2021;14:290. [Crossref] [PubMed]
- Tu Z, Wu L, Wang P, et al. N6-Methylandenosine-Related lncRNAs Are Potential Biomarkers for Predicting the Overall Survival of Lower-Grade Glioma Patients. Front Cell Dev Biol 2020;8:642. [Crossref] [PubMed]
- Wilkerson MD, Hayes DN. ConsensusClusterPlus: a class discovery tool with confidence assessments and item tracking. Bioinformatics 2010;26:1572-3. [Crossref] [PubMed]
- Meng Z, Yuan Q, Zhao J, et al. The m6A-Related mRNA Signature Predicts the Prognosis of Pancreatic Cancer Patients. Mol Ther Oncolytics 2020;17:460-70. [Crossref] [PubMed]
- Shannon P, Markiel A, Ozier O, et al. Cytoscape: a software environment for integrated models of biomolecular interaction networks. Genome Res 2003;13:2498-504. [Crossref] [PubMed]
- Zhou Y, Zhou B, Pache L, et al. Metascape provides a biologist-oriented resource for the analysis of systems-level datasets. Nat Commun 2019;10:1523. [Crossref] [PubMed]
- Yoshihara K, Shahmoradgoli M, Martínez E, et al. Inferring tumour purity and stromal and immune cell admixture from expression data. Nat Commun 2013;4:2612. [Crossref] [PubMed]
- Liu J, Eckert MA, Harada BT, et al. m6A mRNA methylation regulates AKT activity to promote the proliferation and tumorigenicity of endometrial cancer. Nat Cell Biol 2018;20:1074-83. [Crossref] [PubMed]
- Ma JZ, Yang F, Zhou CC, et al. METTL14 suppresses the metastatic potential of hepatocellular carcinoma by modulating N6 -methyladenosine-dependent primary MicroRNA processing. Hepatology 2017;65:529-43. [Crossref] [PubMed]
- Zhang C, Samanta D, Lu H, et al. Hypoxia induces the breast cancer stem cell phenotype by HIF-dependent and ALKBH5-mediated m6A-demethylation of NANOG mRNA. Proc Natl Acad Sci U S A 2016;113:E2047-E2056. [PubMed]
- He Y, Hu H, Wang Y, et al. ALKBH5 Inhibits Pancreatic Cancer Motility by Decreasing Long Non-Coding RNA KCNK15-AS1 Methylation. Cell Physiol Biochem 2018;48:838-46. [Crossref] [PubMed]
- Li J, Han Y, Zhang H, et al. The m6A demethylase FTO promotes the growth of lung cancer cells by regulating the m6A level of USP7 mRNA. Biochem Biophys Res Commun 2019;512:479-85. [Crossref] [PubMed]
- Vu LP, Pickering BF, Cheng Y, et al. The N6-methyladenosine (m6A)-forming enzyme METTL3 controls myeloid differentiation of normal hematopoietic and leukemia cells. Nat Med 2017;23:1369-76. [Crossref] [PubMed]
- Barbieri I, Tzelepis K, Pandolfini L, et al. Promoter-bound METTL3 maintains myeloid leukaemia by m6A-dependent translation control. Nature 2017;552:126-31. [Crossref] [PubMed]
- Chen M, Wei L, Law CT, et al. RNA N6-methyladenosine methyltransferase-like 3 promotes liver cancer progression through YTHDF2-dependent posttranscriptional silencing of SOCS2. Hepatology 2018;67:2254-70. [Crossref] [PubMed]
- Sun S, Han Q, Liang M, et al. Downregulation of m6 A reader YTHDC2 promotes tumor progression and predicts poor prognosis in non-small cell lung cancer. Thorac Cancer 2020;11:3269-79. [Crossref] [PubMed]
- Gu C, Shi X, Qiu W, et al. Comprehensive Analysis of the Prognostic Role and Mutational Characteristics of m6A-Related Genes in Lung Squamous Cell Carcinoma. Front Cell Dev Biol 2021;9:661792. [Crossref] [PubMed]
- Lan T, Li H, Zhang D, et al. KIAA1429 contributes to liver cancer progression through N6-methyladenosine-dependent post-transcriptional modification of GATA3. Mol Cancer 2019;18:186. [Crossref] [PubMed]
- Ni W, Yao S, Zhou Y, et al. Long noncoding RNA GAS5 inhibits progression of colorectal cancer by interacting with and triggering YAP phosphorylation and degradation and is negatively regulated by the m6A reader YTHDF3. Mol Cancer 2019;18:143. [Crossref] [PubMed]
- Yang X, Zhang S, He C, et al. METTL14 suppresses proliferation and metastasis of colorectal cancer by down-regulating oncogenic long non-coding RNA XIST. Mol Cancer 2020;19:46. [Crossref] [PubMed]
- Zhang Z, Li J, Lu K, et al. Identification of Prognostic Markers of N6-Methylandenosine-Related Noncoding RNAs in Non-Small-Cell Lung Cancer. J Oncol 2022;2022:3657349. [Crossref] [PubMed]
- Zhao J, Lin X, Zhuang J, et al. Relationships of N6-Methyladenosine-Related Long Non-Coding RNAs With Tumor Immune Microenvironment and Clinical Prognosis in Lung Adenocarcinoma. Front Genet 2021;12:714697. [Crossref] [PubMed]
- Yu X, Liu J, Xie R, et al. Construction of a prognostic model for lung squamous cell carcinoma based on seven N6-methylandenosine-related autophagy genes. Math Biosci Eng 2021;18:6709-23. [Crossref] [PubMed]
(English Language Editor: L. Huleatt)