Histone acetylation modification regulator-mediated tumor microenvironment infiltration characteristics and prognostic model of lung adenocarcinoma patients
Introduction
At present, the incidence and mortality rates of lung cancer ranks first among malignant tumors in China, and non-small cell lung cancer (NSCLC) is the most common pathological type of lung cancer, accounting for 85% of all lung cancer (1). Lung adenocarcinoma (LUAD) is the most common subtype of NSCLC, accounting for 40% (2). For anti-tumor studies, effectively killing cancer cells without damaging normal cells is a challenge. The key to this is to identify the unique characteristics of tumor cells.
Various diseases, particularly cancer, involve epigenetic changes (3,4). Histone is the main protein component of chromatin and plays a central role in gene regulation, acting as a spool winding DNA (5). Histones undergo various modifications, including phosphorylation, acetylation, glycosylation, methylation, ubiquitination, and citrullination, which affect gene transcription (6,7). The importance of histone acetylation has been confirmed in cancer; it regulates gene transcription and cellular processes such as immune response, apoptosis, autophagy, tumor mutational burden (TMB), cell cycle arrest, DNA damage repair, and metabolism (8). Abnormal histone acetylation is a hallmark of many cancers, particularly NSCLC (9-11).
Histone acetylation is involved in the regulation of various biological processes, which is also a research hotspot in recent years (12). Histone acetylation helps to open chromatin by neutralizing the positive charge of lysine residues on histone and provides a binding platform for “reader” protein (13). Histone acetylation status depends on the competition between histone acetyltransferase (HAT) and histone deacetylase (HDAC) in terms of activity (14,15). The abnormal binding of HDAC to specific promoter region, inhibits the transcription of normal functional genes, which may be one of the mechanisms of underlying malignant tumorigenesis (16). Acetylation modification of lysine residues in histone controls many important functions, including carbon metabolism, transcriptional regulation, amino acid metabolism and so on (16,17). Therefore, Histone acetylation modification has become the target in anti-tumor drug development (18).
Therapeutic agents targeting the epigenetic regulatory family of HDACs have demonstrated clinical success in treating some hematological malignancies (19-21). Preclinical models of melanoma and LUAD have demonstrated that HDAC inhibitors can upregulate the expression of ligand programmed death-ligand 1 (PD-L1) and T-cell chemokines, thereby enhancing the sensitivity of immune responses to anti-programmed death 1 (PD-1)/PD-L1 treatment and improving clinical outcomes (22,23). Recent studies have reported that histone acetylation is closely related to tumor microenvironment (TME); specifically, histone acetylation regulates the cytolytic activity of CD8+ T cells, polarization of macrophages, and immunosuppressive function of regulatory T cells (24-26).
Because of technical limitations, current relevant research is mostly limited to one or two histone acetylation regulators and cell types; however, antitumor effect is characterized by the interaction of many tumor suppressors in a highly coordinated manner. In this regard, bioinformatic analysis is helpful. We developed histone acetylation modification scores to predict prognosis, clinical characteristics, and immune status in patients with LUAD. Our results suggested that histone acetylation modification score is a powerful prognostic marker that can accurately predict prognosis and immunotherapy response. We present the following article in accordance with the TRIPOD reporting checklist (available at https://jtd.amegroups.com/article/view/10.21037/jtd-22-1000/rc).
Methods
Data collection
The RNA-Seq data and corresponding clinical information of patients with LUAD were collected from The Cancer Genome Atlas (TCGA) database. In total, 497 LUAD and 54 normal samples were obtained. The normalized matrix files of the three cohorts (GSE30219, GSE72094 and GSE50081) from the Gene Expression Omnibus (GEO) database were downloaded for the validation of data sets. Genomic mutation data, including somatic mutation and copy number variation (CNV) of TCGA-LUAD were obtained from the UCSC Xena database. Both TCGA and GEO database are publicly available. Thus, the present study did not require the approval of local ethics committees. The literature related to histone acetylation modification was retrieved, and 36 acknowledged histone acetylation genes were curated and analyzed to identify distinct histone acetylation modification patterns. The study was conducted in accordance with the Declaration of Helsinki (as revised in 2013).
Construction of prognostic model based on histone acetylation regulators
The survival R package was used for univariate Cox regression analysis to identify the genes involved in histone acetylation and associated with overall survival (OS).
The risk score calculating formula was shown as follows:
where Expi was the gene expression level and xi was the regression coefficient which was calculated by multivariate Cox regression.
Prediction of immunotherapy response
Tumor immune dysfunction and exclusion (TIDE) is a computational method to simulate the main mechanism of tumor immune escape, which can predict the immune checkpoint blockade response. We downloaded the TIDE scores related to patients with LUAD from http://tide.dfci.harvard.edu for further analysis. In addition, to further study the differential effects of immune checkpoints such as PD-L1 and CTLA4 in different groups, we downloaded the immunophenotypic score (IPS) data of immune checkpoint inhibitors (ICIs) from the cancer immunization database (http://tcia.at/home).
Establishment and validation of nomogram
We constructed a nomogram by integrating traditional clinical variables such as age, gender, T stage, M stage, and N stage, and the risk score derived from the prognostic signature to analyze the probable 1-, 3-, and 5-year overall survival of patients with LUAD. Furthermore, calibration curves of the nomogram were generated to examine the concordance between predicted survival and observed survival after bias correction.
Functional enrichment analysis
To explore the underlying mechanisms of histone acetylation and risk-score-related differentially expressed genes (DEGs) (high- vs. low-risk groups), we performed Gene Ontology (GO) enrichment analysis, involving cellular component (CC), molecular function (MF), and biological process (BP). Further, we performed Kyoto Encyclopedia of Genes and Genomes (KEGG) pathway analyses.
Statistical analysis
The data was processed using the PERL programming language (Version 5.32.0, http://www.perl.org). All statistical analyses were performed using the R software (version 4.1.2, https://www.r-project.org/). P<0.05 was considered statistically significant.
Results
Expression, mutation and interaction of histone acetylation regulated genes in LUAD
A total of 36 histone acetylation regulated genes including 9 writers (HAT1, KAT2A, KAT2B, KAT8, KAT6A, KAT6B, KAT7, EP300 and CREBBP), 12 erasers (HDAC1, HDAC2, HDAC3, HDAC8, SIRT2, HDAC4, HDAC5, HDAC6, HDAC7, HDAC9, HDAC10 and HDAC11) and 15 readers (BRD2, BRD3, BRD4, BRDT, BPTF, ATAD2B, BAZ2B, TAF1, YEATS4, DPF3, SMARCA2, SMARCA4, PBRM1, DPF1 and DPF2) were identified in this study (Table S1). Using the WilCoxon test method in R software, significant differences were observed in the expression of most genes between normal and tumor tissues (Figure 1A). After clarifying the differences in the expression of these genes, we assessed the CNVs and somatic mutations of 36 histone acetylation regulators in patients with LUAD from TCGA cohort. In the TCGA-LUAD cohort, 38.15% of the samples had mutations in histone acetylation regulatory genes, and the mutation type was mainly missense mutation, followed by nonsense mutation (Figure 1B). Among the 36 histone acetylation regulation genes, the mutation rate of smarca4 accounted for 7%, and the mutation rate of HDAC9, BPTF, BAZ2B, and CREBBP was also higher than 3%. The investigation of CNV change frequency revealed that CNV change was common in 36 regulators (Figure 1C). The location of CNV alteration of histone acetylation regulators on 23 chromosomes (Figure 1D). Further, we constructed a network of histone acetylation regulators, revealed the interaction among regulators, and comprehensively presented the prognostic significance of each regulator in patients with LUAD (Figure 1E). The high mutation rate indicates the instability of histone acetylation regulatory genes in the TCGA-LUAD cohort, and suggests the potential relationship between histone acetylation modification and tumors.
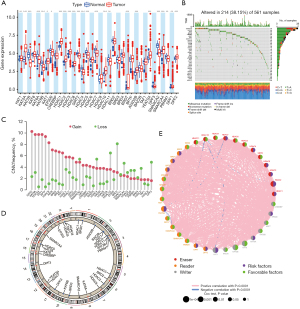
Construction of a prognostic model in the TCGA cohort
Using the WilCoxon test method in R software, we identified 7 genes from the TCGA database (Figure 2A). Only one gene HDAC2 was considered as the risk factor with HR >1 in patients with LUAD, whereas another 6 genes, KAT2B, KAT8, SIRT2, HDAC5, BAZ2B, and TAF1, were considered as protective factors with HR <1. After LASSO-Cox regression analysis, 5 genes were screened to establish the prognosis model using the Cox regression method (Figure 2B,2C). The risk score was calculated using the following formula: risk score = (-0.08807 × expression value of KAT2B) + (−0.03254 × expression value of KAT8) + (−0.036396 × expression value of HDAC2) + (−0.03734 × expression value of SIRT2) + (−0.04152 × expression value of HDAC5). Further, patients in the training cohort were divided into high- and low-risk groups based on the risk scores. The high- and low-risk groups could be clearly distinguished using the principal component analysis (PCA) and t-distributed stochastic neighbor embedding (t-SNE) analysis (Figures 2D,2E). The 5 genes exhibited a certain correlation (Figure 2F). The Kaplan–Meier survival curve revealed the significantly worse survival in the high-risk group (P=6.24e−03; Figure 2G). The expression trend of 5 hub genes is shown in the heat map in Figure 2H. The risk distribution of gene expression in the training set and the survival status of each patient are given in Figure 2I,2J. Overall, these 5 histone acetylation characteristics can distinguish the prognosis of patients with LUAD to some extent.
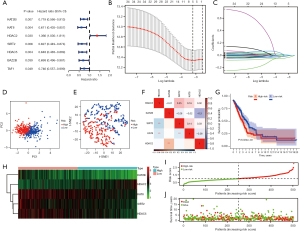
Clinicopathologic features analysis and subgroup analysis
We analyzed the correlation between risk score and clinicopathologic features, several clinical features including gender, TNM stage are correlated with risk score, except for age Figure 3A. We conducted a subgroup analysis to clarify the relationship between TNM stage subgroups and risk group Figure 3B-3G. In T1–2 stage, the higher the significant risk score, the more obvious the tumor progression, but there was no significant difference in T3–4 stage. In stage N0, the higher the significant risk score was, the more obvious the lymph node metastasis was. However, there was no significant difference in stage N1–2. The same situation happens between M0 and M1 stage. In conclusion, the risk score is related to the occurrence, development and invasion of earlier stage.
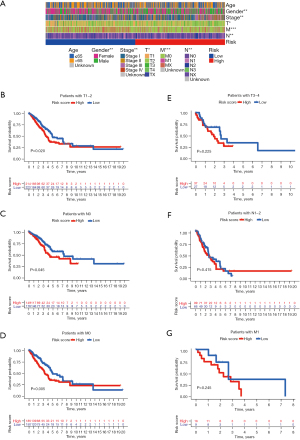
Establishment and validation of nomogram
Univariate and multivariate analyses revealed that risk score was an independent prognostic predictor in the TCGA cohort. The results of univariate Cox regression analyses revealed that the risk score was significantly associated with OS (HR =2.457, 95% CI: 1.786–3.379, P<0.001; Figure 4A). After correcting for other confounding factors, multivariate analyses revealed that risk score still proved to be an independent prognostic predictor in the TCGA (HR =2.311, 95% CI: 1.649–3.237, P<0.001; Figure 4B). We established a nomogram to accurately predict the OS of the patients using risk score calculated from the prognostic signature of histone-acetylation-related genes and other clinicopathological factors, including age, gender, T stage, M stage, N stage, and risk score (Figure 4C). Calibration plots for, 3-, and 5-year OS were used to visualize the performances of the nomograms (Figure 4D). Receiver operating characteristic (ROC) curve revealed that the AUC of nomogram for predicting 5-year survival was 0.735 (Figure 4E). The results indicated that this model performed well in predicting OS.
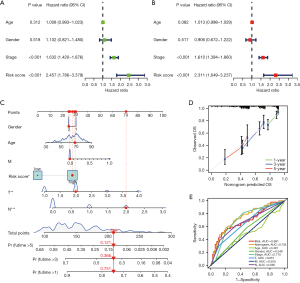
Correlation analysis between risk score and TMB
We divided the patients with LUAD in TCGA into H-TMB and L-TMB groups based on the TMB. After grouping TMB and risk groups, survival analysis was performed again, and the survival curve was plotted (Figure 5A). It confirmed the adverse effect of low mutation on survival. Correlation analysis confirmed the correlation between risk score and TMB (R=0.21, P=2.33e-06; Figure 5B). The difference between mutation and low and high scores was revealed by drawing waterfall diagram (Figure 5C,5D). Obviously, the proportion of mutations was higher in the high-risk group.
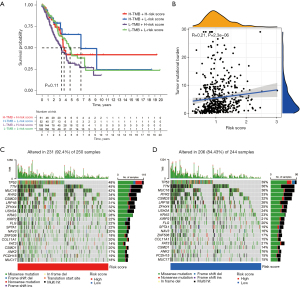
Function and pathway enrichment analysis
First, we analyzed the differences between the high- and low-risk groups and determined the DEGs. To elucidate the biological functions and pathways, the DEGs were used to perform GO enrichment and KEGG pathway analyses. GO enrichment analysis indicated that DEGs were enriched in humoral immune response, collagen-containing extracellular matrix, receptor ligand activity, and signaling receptor activator activity (P<0.05; Figure 6A,6B). It is worth noting that negative regulation of protein processing was confirmed by enrichment analysis (Padjust<0.05). Interestingly, KEGG enrichment indicated that DEGs were enriched in IL-17 signaling pathway and amoebiasis (Padjust<0.05; Figure 6C,6D).
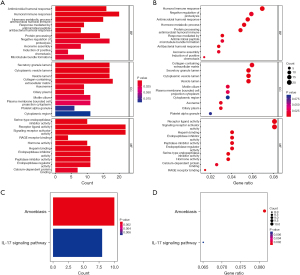
Analysis of immune cell infiltration and subgroup analysis
Considering the important influence of immune cell infiltration on tumor progression, we compared the differences in immune cell infiltration between the two groups (Figure 7A). T cells, macrophages, mast cells, and neutrophils were different in terms of levels between the high- and low-risk groups (P<0.01). We conducted a subgroup analysis to clarify the relationship between immune cell subgroups and risk groups (Figure 7B-7G). The results indicated that different degrees of immune cell infiltration were closely related to the prognosis of patients with LUAD.
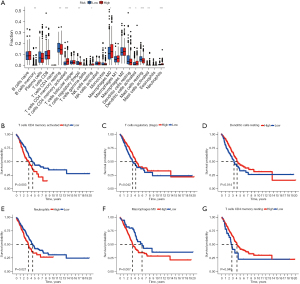
Immune-relevance and drug sensitivity analyses
To better understand the complex relationship between immunity and histone acetylation, we compared the differences of immune status between the two groups (Figure 8A). B cells, DCS, HLA, and immune checkpoints were significantly different between the two groups, which may indicate that targeted histone acetylation can change the immune state of LUAD or enhance the response to the immunotherapy in LUAD. Gene set variation analysis (GSVA) enrichment revealed the activation of various pathways under the high- and low-risk groups (Figure 8B). The correlation heat map revealed the correlation between the 5 candidate genes and immune checkpoints (Figure 8C). The correlation between KAT2B and immune checkpoints cannot be ignored. Moreover, the immune microenvironment of the high- and low-risk groups was different (Figure 8D-8F). The low-risk group exhibited higher ESTMATEscore, Immunescore, and Stromalscore. (Figure 8G-8J) show the sensitivity of PDL1 and CTLA4 immunotherapy in the high- and low-risk groups. Low risk means higher sensitivity to immunotherapy. Comparison of TIDE score, microsatellite instability (MSI), or other immune-related characteristics in high risk and low risk groups (Figure 8K). TIDE score can be used to evaluate the immune escape of tumor. Our study demonstrated that the low-risk group was more prone to immune escape. Further, to improve the guiding significance of risk score for clinical application, we identified the sensitivity of patients in different groups to LUAD using the GDSC database (Figure 8L,8M). The results revealed significantly lower IC50 values of paclitaxel and etoposide in the high-risk group.
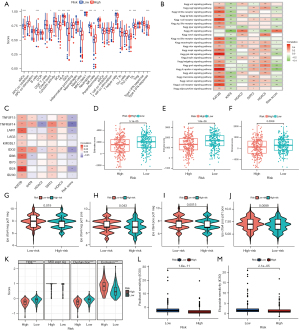
Validation of the 5-gene signature in the GEO cohort
We combined three cohorts (GSE30219, GSE72094, and GSE50081) from GEO database to verify the above results. Based on the same calculation and grouping method, we divided the patients from GEO cohorts into the low- and high-risk groups. The difference in survival between the two groups was significant (Figure 9A). The survival curve indicated that the survival rate of the low-risk group was better than that of the high-risk group. The risk distribution of gene expression in the training set and the survival status of each patient are given in Figure 9B,9C. The expression trend of the 5 hub genes is given in the heat map in Figure 9D. ROC curve revealed the AUC of risk score for predicting survival for 1-, 3-, and 5-year (Figure 9E). The high- and low-risk groups could be clearly distinguished using the PCA and t-SNE analysis (Figure 9F,9G). The immune cell infiltration results from the GEO cohort verified the results from TCGA cohort, although some differences were noted (Figure 9H). The correlation heat map revealed the correlation between the 5 candidate genes and immune checkpoints (Figure 9I). KAT2B was associated with the most immune checkpoints.
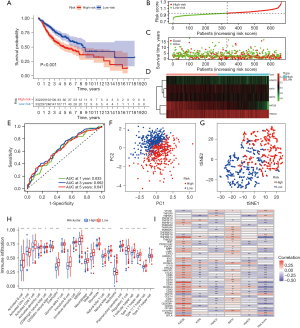
Discussion
Acetylation refers to the transfer of acetyl groups from acetyl CoA to amino acid residues in proteins by an enzymatic or nonenzymatic process (12). The more extensive function of acetylation modification is not limited to the nucleus, thus, it can be divided into histone and non-histone acetylation modifications (27). At present, acetylation modification of histones is studied most widely, which is very important in the process of apparent regulation (5). Histone acetylation modification affects the activities of various physiological processes through the interaction of proteins with various acetylation regulators, including chromatin remodeling, cell cycle, splicing, nuclear transport, mitochondrial biology, and actin nucleation (28). In biology, acetylation plays an important role in the formation of immunity, physiological rhythm and memory. Protein acetylation is a favorable target for designing new drugs against many diseases (29,30). Recent studies have report that acetylation modification plays an important role in the occurrence and development of tumors (31). Considering the close relationship between the three histone acetylation regulators (“writer,” “eraser,” and “reader”), their overall analysis of them would help to enhance our understanding of tumor epigenetic transcriptomics and guide more effective treatment strategies (32).
In this study, we found that there were significant differences in the expression of acetylated genes between tumors and normal tissues. We systematically analyzed the prognostic prediction accuracy of Histone acetylation genes in LUAD using bioinformatics and statistical tools, and 5 genes (KAT2B, SIRT2, HDAC5, KAT8, HDAC2) were screened from 36 Histone acetylation genes to construct the prognostic model and calculate the risk score. Previous studies have shown that all five candidate genes are closely related to cancer.
Both KAT2B and KAT8 belong to HAT. KAT2B, lysine acetyltransferase 2B, is closely related to immunotherapy, and low KAT2B expression was associated with unsatisfactory efficacy of immune checkpoint blockade (ICB) of patients with LUAD (33,34). In addition, KAT2B is related to congenital heart disease, pulmonary artistic hypertension and other diseases (35,36). KAT8, lysine acetyltransferase 8, contributes to tumor progression by activating epidermal growth factor receptor (EGFR) signaling in glioblastoma cells (37,38). In addition, KAT8 can regulate the level of reactive oxygen species (39). Both HDAC5 and HDAC2 (Histone Deacetylase 5 and 2) belong to Non-specific HDAC. HDAC5 expression was regulated by PI3K/Akt signaling pathway in diabetic kidney disease (40). At the same time, some studies report that catalytic activity of HDAC5 suppresses oxidative stress in cardiomyocyte and NRF2 target gene expression (41). The expression level of HDAC2 was negatively correlated with the prognosis of patients with lung cancer (42). In colorectal cancer, HDAC2 promotes epithelial mesenchymal transition (EMT) (43). Sirtuin 2 (SIRT2) is an NAD+-dependent deacetylase inhibiting T-cell metabolism by targeting key enzymes involved in glycolysis, tricarboxylic acid cycle, fatty acid oxidation and glutamine decomposition (44).
According to the median risk score, patients with LUAD were divided into low- and high-risk groups. The prognosis analysis revealed that the OS of the high-risk group was significantly shorter than that of low-risk group. Through univariate and multivariate analyses, risk score can be used as an independent risk factor for prognosis analysis of patients with LUAD. Our data revealed a significant correlation between risk score and tumor mutation load. In the high-risk group, the mutation frequency of TP53, TTN and other genes increased significantly, and it was consistent with our prediction. In clinical correlation analysis, risk score had no significant effect on T3–4, N1–2 and M1 stages of lung cancer through subgroup analysis, in other words, risk score is closely related to early-stage LUAD. To help in determining the prognosis of individual patients, we combined the 5 gene characteristics and clinicopathological parameters to construct a nomogram.
At present, the influence of immune factors on tumors has attracted much attention, not only the immune microenvironment, the immune escape of tumors and so on (45,46). Immunotherapy may become the key treatment to cure cancer (47). In recent years, immune checkpoint blocking therapy has shown significant efficacy in the treatment of various types of tumors (47,48). In our study, we paid special attention to the effect of acetylation score on immunity. Interestingly, according to our prognostic model, acetylation was closely related to the TME, and some classical immune checkpoints were identified. Importantly, the immunotherapeutic effect of PDL1 and CTLA4 was also very obvious (49,50).
TIDE algorithm is a newly developed method to calculate the potential regulators and indicators of ICI resistance (51,52). It can be used to calculate the interaction between T-cell characteristics in LUAD to simulate tumor immune escape. A higher TIDE score indicates a higher possibility of tumor immune escape and a lower response rate to ICI treatment. In our study, the calculated risk score is strongly negatively correlated with TIDE score, which means that the high-risk group was not prone to immune escape. This was unexpected, so more in-depth studies are necessary.
After determining the relationship between Histone acetylation and the immune microenvironment of LUAD, it may be necessary to provide potential therapeutic agents. So, we further identified the therapeutic effect of common chemotherapeutic drugs between high- and low-risk groups. The screening results showed that paclitaxel and etoposide showed potential sensitivity and selectivity for LUAD with high-risk group of Histone acetylation.
To verify the results obtained from the TCGA cohort, mRNA sequencing data from the tissue samples from patients with LUAD (GSE30219, GSE72094 and GSE50081) were analyzed using R software and related R packages to conduct a batch correction of sequencing results as the validation cohort. By analyzing the data of the validation cohort, results similar to those from the TCGA cohort were obtained, including those related to immune microenvironment, immune cell infiltration, immune checkpoint, and drug sensitivity.
This study has some limitations. First, we only included 505 tumor samples in this study to construct the prognostic model. Studies with a larger sample size are needed. Second, most of the patients were white, African, or Latino and did not represent all human races. Third, more samples are needed to verify the model. Finally, further biochemical experiments should be performed to explore the roles of the histone-acetylation-related genes and their interactions.
Conclusions
Our study provided a robust signature for predicting the changing survival risk of patients with LUAD, and it appears to be a potentially useful prognostic tool. Moreover, we revealed the important relationship between histone acetylation and tumor immune microenvironment.
Acknowledgments
We acknowledge the contributions of all doctors from the Department of Thoracic Surgery, Affiliated Hospital of Nantong University to the study. They also gave written permission for the publication of data and conclusions.
Funding: This work was supported by the National Natural Science Foundation of China (No. 81770266); Clinical Medical Research Center of Cardiothoracic Diseases in Nantong (No. HS2019001), Innovation Team of Cardiothoracic Disease in Affiliated Hospital of Nantong University (No. TECT-A04), and Nantong Key Laboratory of Translational Medicine of Cardiothoracic.
Footnote
Reporting Checklist: The authors have completed the TRIPOD reporting checklist. Available at https://jtd.amegroups.com/article/view/10.21037/jtd-22-1000/rc
Peer Review File: Available at https://jtd.amegroups.com/article/view/10.21037/jtd-22-1000/prf
Conflicts of Interest: All authors have completed the ICMJE uniform disclosure form (available at https://jtd.amegroups.com/article/view/10.21037/jtd-22-1000/coif). All authors report funding from National Natural Science Foundation of China (No. 81770266), Clinical Medical Research Center of Cardiothoracic Diseases in Nantong (No. HS2019001), Innovation Team of Cardiothoracic Disease in Affiliated Hospital of Nantong University (No. TECT-A04), and Nantong Key Laboratory of Translational Medicine of Cardiothoracic. The authors have no other conflicts of interest to declare.
Ethical Statement: The authors are accountable for all aspects of the work in ensuring that questions related to the accuracy or integrity of any part of the work are appropriately investigated and resolved. The study was in accordance with the Helsinki Declaration (as revised in 2013).
Open Access Statement: This is an Open Access article distributed in accordance with the Creative Commons Attribution-NonCommercial-NoDerivs 4.0 International License (CC BY-NC-ND 4.0), which permits the non-commercial replication and distribution of the article with the strict proviso that no changes or edits are made and the original work is properly cited (including links to both the formal publication through the relevant DOI and the license). See: https://creativecommons.org/licenses/by-nc-nd/4.0/.
References
- Li S, Lin Y, Wu Y, et al. The Value of Serum Exosomal miR-184 in the Diagnosis of NSCLC. J Healthc Eng 2022;2022:9713218. [Crossref] [PubMed]
- Sinha A, Zou Y, Patel AS, et al. Early-Stage Lung Adenocarcinoma MDM2 Genomic Amplification Predicts Clinical Outcome and Response to Targeted Therapy. Cancers (Basel) 2022;14:708. [Crossref] [PubMed]
- Garcia-Gomez A, Li T, de la Calle-Fabregat C, et al. Targeting aberrant DNA methylation in mesenchymal stromal cells as a treatment for myeloma bone disease. Nat Commun 2021;12:421. [Crossref] [PubMed]
- Ahmad S, Manzoor S, Siddiqui S, et al. Epigenetic underpinnings of inflammation: Connecting the dots between pulmonary diseases, lung cancer and COVID-19. Semin Cancer Biol 2022;83:384-98. [Crossref] [PubMed]
- Shvedunova M, Akhtar A. Modulation of cellular processes by histone and non-histone protein acetylation. Nat Rev Mol Cell Biol 2022;23:329-49. [Crossref] [PubMed]
- Zhu D, Zhang Y, Wang S. Histone citrullination: a new target for tumors. Mol Cancer 2021;20:90. [Crossref] [PubMed]
- Liu J, Wang Q, Kang Y, et al. Unconventional protein post-translational modifications: the helmsmen in breast cancer. Cell Biosci 2022;12:22. [Crossref] [PubMed]
- Wieczorek M, Ginter T, Brand P, et al. Acetylation modulates the STAT signaling code. Cytokine Growth Factor Rev 2012;23:293-305. [Crossref] [PubMed]
- Chen TF, Hao HF, Zhang Y, et al. HBO1 induces histone acetylation and is important for non-small cell lung cancer cell growth. Int J Biol Sci 2022;18:3313-23. [Crossref] [PubMed]
- Guo K, Ma Z, Zhang Y, et al. HDAC7 promotes NSCLC proliferation and metastasis via stabilization by deubiquitinase USP10 and activation of β-catenin-FGF18 pathway. J Exp Clin Cancer Res 2022;41:91. [Crossref] [PubMed]
- Wang T, Lu Z, Han T, et al. Deacetylation of Glutaminase by HDAC4 contributes to Lung Cancer Tumorigenesis. Int J Biol Sci 2022;18:4452-65. [Crossref] [PubMed]
- Barneda-Zahonero B, Parra M. Histone deacetylases and cancer. Mol Oncol 2012;6:579-89. [Crossref] [PubMed]
- Hsu CC, Shi J, Yuan C, et al. Recognition of histone acetylation by the GAS41 YEATS domain promotes H2A.Z deposition in non-small cell lung cancer. Genes Dev 2018;32:58-69. [Crossref] [PubMed]
- Ramaiah MJ, Tangutur AD, Manyam RR. Epigenetic modulation and understanding of HDAC inhibitors in cancer therapy. Life Sci 2021;277:119504. [Crossref] [PubMed]
- Ropero S, Esteller M. The role of histone deacetylases (HDACs) in human cancer. Mol Oncol 2007;1:19-25. [Crossref] [PubMed]
- Audia JE, Campbell RM. Histone Modifications and Cancer. Cold Spring Harb Perspect Biol 2016;8:a019521. [Crossref] [PubMed]
- Liu S, Chang W, Jin Y, et al. The function of histone acetylation in cervical cancer development. Biosci Rep 2019;39:BSR20190527. [Crossref] [PubMed]
- Tran TQ, Lowman XH, Kong M. Molecular Pathways: Metabolic Control of Histone Methylation and Gene Expression in Cancer. Clin Cancer Res 2017;23:4004-9. [Crossref] [PubMed]
- Bottoni A, Rizzotto L, Lai TH, et al. Targeting BTK through microRNA in chronic lymphocytic leukemia. Blood 2016;128:3101-12. [Crossref] [PubMed]
- Lasko LM, Jakob CG, Edalji RP, et al. Discovery of a selective catalytic p300/CBP inhibitor that targets lineage-specific tumours. Nature 2017;550:128-32. [Crossref] [PubMed]
- Wang P, Wang Z, Liu J. Role of HDACs in normal and malignant hematopoiesis. Mol Cancer 2020;19:5. [Crossref] [PubMed]
- Booth L, Roberts JL, Kirkwood J, et al. Unconventional Approaches to Modulating the Immunogenicity of Tumor Cells. Adv Cancer Res 2018;137:1-15. [Crossref] [PubMed]
- Wang YF, Liu F, Sherwin S, et al. Cooperativity of HOXA5 and STAT3 Is Critical for HDAC8 Inhibition-Mediated Transcriptional Activation of PD-L1 in Human Melanoma Cells. J Invest Dermatol 2018;138:922-32. [Crossref] [PubMed]
- Kasmani MY, Cui W. Inhibiting BRD4 to generate BETter T cell memory. J Exp Med 2021;218:e20210877. [Crossref] [PubMed]
- Yang W, Feng Y, Zhou J, et al. A selective HDAC8 inhibitor potentiates antitumor immunity and efficacy of immune checkpoint blockade in hepatocellular carcinoma. Sci Transl Med 2021;13:eaaz6804. [Crossref] [PubMed]
- Zhang D, Tang Z, Huang H, et al. Metabolic regulation of gene expression by histone lactylation. Nature 2019;574:575-80. [Crossref] [PubMed]
- Narita T, Weinert BT, Choudhary C. Functions and mechanisms of non-histone protein acetylation. Nat Rev Mol Cell Biol 2019;20:156-74. [Crossref] [PubMed]
- Choudhary C, Kumar C, Gnad F, et al. Lysine acetylation targets protein complexes and co-regulates major cellular functions. Science 2009;325:834-40. [Crossref] [PubMed]
- Eckschlager T, Plch J, Stiborova M, et al. Histone Deacetylase Inhibitors as Anticancer Drugs. Int J Mol Sci 2017;18:1414. [Crossref] [PubMed]
- Wang H, Fu C, Du J, et al. Enhanced histone H3 acetylation of the PD-L1 promoter via the COP1/c-Jun/HDAC3 axis is required for PD-L1 expression in drug-resistant cancer cells. J Exp Clin Cancer Res 2020;39:29. [Crossref] [PubMed]
- Yang G, Yuan Y, Yuan H, et al. Histone acetyltransferase 1 is a succinyltransferase for histones and non-histones and promotes tumorigenesis. EMBO Rep 2021;22:e50967. [Crossref] [PubMed]
- Xu Y, Zhang S, Lin S, et al. WERAM: a database of writers, erasers and readers of histone acetylation and methylation in eukaryotes. Nucleic Acids Res 2017;45:D264-70. [PubMed]
- Zhou X, Wang N, Zhang Y, et al. KAT2B is an immune infiltration-associated biomarker predicting prognosis and response to immunotherapy in non-small cell lung cancer. Invest New Drugs 2022;40:43-57. [Crossref] [PubMed]
- Bondy-Chorney E, Denoncourt A, Sai Y, et al. Nonhistone targets of KAT2A and KAT2B implicated in cancer biology 1. Biochem Cell Biol 2019;97:30-45. [Crossref] [PubMed]
- Hou YS, Wang JZ, Shi S, et al. Identification of epigenetic factor KAT2B gene variants for possible roles in congenital heart diseases. Biosci Rep 2020;40:BSR20191779. [Crossref] [PubMed]
- Li D, Shao NY, Moonen JR, et al. ALDH1A3 Coordinates Metabolism With Gene Regulation in Pulmonary Arterial Hypertension. Circulation 2021;143:2074-90. [Crossref] [PubMed]
- Dong Z, Zou J, Li J, et al. MYST1/KAT8 contributes to tumor progression by activating EGFR signaling in glioblastoma cells. Cancer Med 2019;8:7793-808. [Crossref] [PubMed]
- Radzisheuskaya A, Shliaha PV, Grinev VV, et al. Complex-dependent histone acetyltransferase activity of KAT8 determines its role in transcription and cellular homeostasis. Mol Cell 2021;81:1749-1765.e8. [Crossref] [PubMed]
- Yin S, Jiang X, Jiang H, et al. Histone acetyltransferase KAT8 is essential for mouse oocyte development by regulating reactive oxygen species levels. Development 2017;144:2165-74. [PubMed]
- Xu Z, Jia K, Wang H, et al. METTL14-regulated PI3K/Akt signaling pathway via PTEN affects HDAC5-mediated epithelial-mesenchymal transition of renal tubular cells in diabetic kidney disease. Cell Death Dis 2021;12:32. [Crossref] [PubMed]
- Hu T, Schreiter FC, Bagchi RA, et al. HDAC5 catalytic activity suppresses cardiomyocyte oxidative stress and NRF2 target gene expression. J Biol Chem 2019;294:8640-52. [Crossref] [PubMed]
- Tang D, Zhao YC, Qian D, et al. Novel genetic variants in HDAC2 and PPARGC1A of the CREB-binding protein pathway predict survival of non-small-cell lung cancer. Mol Carcinog 2020;59:104-15. [Crossref] [PubMed]
- Hu XT, Xing W, Zhao RS, et al. HDAC2 inhibits EMT-mediated cancer metastasis by downregulating the long noncoding RNA H19 in colorectal cancer. J Exp Clin Cancer Res 2020;39:270. [Crossref] [PubMed]
- Wang Y, Yang J, Hong T, et al. SIRT2: Controversy and multiple roles in disease and physiology. Ageing Res Rev 2019;55:100961. [Crossref] [PubMed]
- Gajewski TF, Schreiber H, Fu YX. Innate and adaptive immune cells in the tumor microenvironment. Nat Immunol 2013;14:1014-22. [Crossref] [PubMed]
- Walsh SR, Simovic B, Chen L, et al. Endogenous T cells prevent tumor immune escape following adoptive T cell therapy. J Clin Invest 2019;129:5400-10. [Crossref] [PubMed]
- Steven A, Fisher SA, Robinson BW. Immunotherapy for lung cancer. Respirology 2016;21:821-33. [Crossref] [PubMed]
- Topalian SL, Taube JM, Anders RA, et al. Mechanism-driven biomarkers to guide immune checkpoint blockade in cancer therapy. Nat Rev Cancer 2016;16:275-87. [Crossref] [PubMed]
- Andrews LP, Yano H, Vignali DAA. Inhibitory receptors and ligands beyond PD-1, PD-L1 and CTLA-4: breakthroughs or backups. Nat Immunol 2019;20:1425-34. [Crossref] [PubMed]
- Vijayan D, Young A, Teng MWL, et al. Targeting immunosuppressive adenosine in cancer. Nat Rev Cancer 2017;17:709-24. [Crossref] [PubMed]
- Jiang P, Gu S, Pan D, et al. Signatures of T cell dysfunction and exclusion predict cancer immunotherapy response. Nat Med 2018;24:1550-8. [Crossref] [PubMed]
- Yi M, Jiao D, Qin S, et al. Synergistic effect of immune checkpoint blockade and anti-angiogenesis in cancer treatment. Mol Cancer 2019;18:60. [Crossref] [PubMed]