Clinical predictors of treatment efficacy and a prognostic nomogram in patients with lung adenocarcinoma receiving immune checkpoint inhibitors: a retrospective study
Introduction
Among the 36 cancers considered in the 2020 Global Cancer Statistics, lung cancer was the second most common type of cancer and the leading cause of cancer-related mortality worldwide (1). Non-small cell lung cancer (NSCLC) is the most common pathological type of lung cancer, among which adenocarcinomas accounts for about 60% of all NSCLCs and are the most common subtype of NSCLC (2).
In 2015, the New England Journal of Medicine successively published the research results of the CheckMate-017/057 trials, which marked the official commencement of immunotherapy treatment in patients with NSCLC (3,4). One year later, with the amazing results of the Keynote-024 study, the first first-line treatment indication for advanced lung cancer was approved, creating a new pattern of immunotherapy for NSCLC (5). Since then, given the excellent results of the Keynote-042 and Keynote-189/407 studies, the indications of immunotherapy have been extended to programmed death ligand 1 (PD-L1) ≥1% and the whole population (6-8). Thereafter, immunotherapeutic drugs such as camrelizumab, sintilimab, tislelizumab, and penpulimab have been successively developed (9-11). Within the past 5 years, the application of immunotherapy has spread rapidly in clinics and has become the standard first-line treatment for some patients.
The popularity of immunotherapy has drastically changed the current treatment landscape for lung cancer patients. At present, immune checkpoint inhibitors (ICIs) have successfully transitioned from being a later-line therapy to a first-line and locally advanced consolidation treatment for NSCLC, and are evolving into neoadjuvant and adjuvant treatments for early lung cancer. At the same time, multiple drug-use modes for single-drug and combined treatments are being comprehensively developed. The strategy of combined immunotherapy further improves the efficacy of immunotherapy and enhances the treatment methods. Furthermore, it also expands the cohort of patients who benefit from immunotherapy, achieves full coverage for driver gene-negative patients, and initiates a new era of immunotherapy.
However, identifying the patient cohort that will benefit from immunotherapy remains a pressing problem to be addressed. Previous studies have explored the prediction of immunotherapy efficacy from multiple perspectives, such as drug-based score (12), lung immune prognostic index (LIPI) (13), smoking (14), liver metastasis (15), lactate dehydrogenase (LDH) (16,17), tumor mutational burden (TMB) expression (18), neutrophil-to-lymphocyte ratio (NLR) (19,20), modified lung immune predictive index (mLIPI) scores (21), and so on. Despite achieving some results, the existing literature does not completely solve the problem of predicting immunotherapy efficacy.
Programmed death ligand 1 tumor proportion score (PD-L1 TPS) is the most common clinical prognostic markers used to predict the efficacy of immunotherapy. However, existing studies have shown that the expression level of PD-L1 is not completely related to the prognosis of ICIs treatment, and the data between various clinical studies are inconsistent (8,22). Similarly, tissue tumor mutation burden (TMB) as a predictor of immunotherapy has its limitations, including the lack of standardization between the testing platforms used and the lack of a fixed TMB threshold, which requires more exploration (23). An existing study show that about 60% of patients with advanced NSCLC cannot benefit from immunotherapy (24). At present, the development of biomarkers for predicting the efficacy of ICIs in patients with advanced lung adenocarcinoma is limited, and there is a lack of prediction models. In addition, immunotherapy is different from the targeted therapy commonly used in the past. Its function involves complex tumor microenvironment, and there is no precise target. Therefore, we speculate that the prediction model under the combination of multiple factors is more promising to predict the efficacy of immunotherapy. Thus, it is necessary to develop a model with high clinical accessibility to help clinicians effectively identify potentially effective populations for ICIs treatment. The existing studies have also carried out some exploration on the prediction model of immunotherapy efficacy (25,26). Yuan and his colleagues developed a nomogram to predict the prognosis of NSCLC patients treated with anti-PD-1 antibodies. His model was a retrospective study and lacked the inclusion of some important indicators, such as PD-L1 expression (25). Zeng and colleagues constructed a prognostic model for patients with NSCLC receiving ICIs in combination with chemotherapy, and their study included a total of 130 patients (26). This study included patients with lung adenocarcinoma and did not restrict the immunotherapy modalities. Thus, this study is more applicable to patients with lung adenocarcinoma. Although these existing studies have explored predictive models for immunotherapy efficacy, there are some common problems, such as small sample size, insufficient population concentration, lack of important indicators and other defects.
Therefore, this study retrospectively analyzed the survival data of 201 patients with lung adenocarcinoma who received immunotherapy in the real world to explore the correlation between clinicopathological characteristics and immunotherapy efficacy. We also sought to build an immunotherapy efficacy prediction model that is suitable for clinical use to provide greater data support for clinical decision-making. We present the following article in accordance with the TRIPOD reporting checklist (available at https://jtd.amegroups.com/article/view/10.21037/jtd-22-1270/rc).
Methods
Patients
This study was a single-center retrospective study. The medical records of lung adenocarcinoma patients who received ICIs [including anti-PD-L1 and anti-programmed cell death 1 (PD-1) therapy] treatment at Shanghai Chest Hospital between August 2017 and February 2021 were screened and reviewed.
The inclusion criteria were as follows: (I) lung adenocarcinoma patients diagnosed by histopathology or cytology; (II) patients who received ICIs; (III) those with measurable target lesions; and (IV) tumor node metastasis (TNM) stage assessed by chest computed tomography (CT), abdominal ultrasound, magnetic resonance imaging (MRI) of the brain, bone scanning, or by replacing some of the above tests with positron emission tomography (PET)-CT. The TNM status of patients was assessed according to the American Joint Committee on Cancer (AJCC) 8th edition staging system. Firstly, patients who had received immunotherapy were screened. Next, patients with lung adenocarcinoma with sufficient clinical data were selected. Finally, the patients were divided into groups according to the clinicopathological characteristics and treatment data and analyzed separately.
The exclusion criteria were as follows: (I) non-adenocarcinoma patients; (II) those with tumors of organs other than the lungs; (III) lung cancer in which the type of pathology was not specified; (IV) patients with insufficient survival data; (V) patients that did not receive regular treatment; (VI) those with other concurrent tumors; (VII) cases complicated by serious systemic diseases; and (VIII) patients who were lost to follow-up. This study was approved by the Institutional Review Board of Shanghai Chest Hospital (Shanghai, China, No. IS21127) and was carried out in accordance with the requirements of the Declaration of Helsinki (as revised in 2013). Informed consent was taken from all the patients.
Data collection
Follow-up was conducted via hospital visit or telephone conversation. Major clinicopathological characteristics, including age, sex, smoking history, TNM stage, Kirsten rat sarcoma viral oncogene homolog (KRAS) mutation status, tumor protein p53 (TP53) mutation status, tumor status, PD-L1 expression, liver metastases, brain metastases, tumor location, adverse effects (AEs), line of immunotherapy, and immunotherapy modalities were collected. Data that were not available were recorded as missing data (NA, not available). Gene mutation was detected by the amplification refractory mutation system (ARMS) or next-generation sequencing (NGS) technology. PD-L1 expression was analyzed via immunohistochemistry assay in tumor tissue with 22C3, E1L3N, or SP142 antibodies (PD-L1 expression ≥1% was considered positive).
Treatment
All lung adenocarcinoma patients included in this study had received at least one cycle of immunotherapy. The immunotherapeutic drugs involved in this study included nivolumab, pembrolizumab, tislelizumab, sintilimab, camerelizumab, atezolizumab, and durvalumab, and the doses and usage of drugs administered are shown in Table S1. Treatment was continued until disease progression, intolerable toxicity, or death. Progression assessment was conducted every 6–8 weeks until disease progression. The clinical follow-up examination items mainly included physical examination, imaging examination (such as chest CT, abdominal ultrasound, brain-enhanced MRI, bone scan or PET-CT instead of some of the above examinations), and routine laboratory examination (such as blood routine examination, liver function, renal function, tumor indicators, thyroid function, myocardial injury biomarkers).
Therapeutic response was evaluated using Response Evaluation Criteria in Solid Tumors (RECIST) version 1.1. Progression-free survival (PFS) was defined as the date from the initiation of immunotherapy until the date of progression or last follow-up visit (June 1, 2021). Overall survival (OS) was defined as the time from the start of immunotherapy to the date of death or last follow-up visit. The primary endpoint of this study was PFS, and the secondary endpoints were OS and objective response rate (ORR). The median follow-up time for the entire study cohort was 10.9 months.
Construction and verification of a prognostic nomogram
Variables that were statistically different were screened out by univariate analysis of PFS, and then a Cox regression model was constructed for multivariate analysis for those variables that were significant in the univariate analysis. Following univariate and multivariate analyses, all of the identified independent prognostic parameters were utilized to develop a prognostic nomogram for predicting the 3-month, 6-month, 1-year, and 2-year PFS outcomes of lung adenocarcinoma patients using the rms package in R version 4.1.1 (https://www.rdocumentation.org/packages/rms/versions/4.1-1). Next, the models were internally validated via the bootstrap method. The predictive power of the nomogram was quantitatively assessed by the C-index and the area under the curve (AUC). The C-index value ranges from 0.5–1.0, with 0.5 indicating a random probability and 1.0 indicating a perfectly correct prediction of the outcome using the model.
Statistical analysis
The patients’ demographic, clinicopathological, and prognostic data were analyzed. Categorical variables were compared using Fisher’s exact or chi-square test. Univariate survival analyses were conducted using the Kaplan-Meier method, and data were compared using the log-rank test. Subsequently, positive variables and important clinical variables that may affect prognosis were further analyzed using multivariate Cox proportional hazards regression models. The hazard ratio (HR) and corresponding 95% confidence interval (CI) were calculated using the Cox regression model. All variables included in the nomogram model were evaluated by the variance inflation factor (VIF); VIF >2.0 was interpreted as indicating multicollinearity. Analyses were carried out using SPSS 24.0 software (IBM, Armonk, NY, USA). A two-sided P value <0.05 was considered statistically significant.
Results
Patient characteristics
According to the inclusion and exclusion criteria, 201 eligible lung adenocarcinoma patients who received immunotherapy were enrolled. Among these patients, 101 received first-line immunotherapy, while the remaining 100 cases received second-line and above immunotherapy. The patient selection steps are shown in Figure 1, and the characteristics of the entire patient cohort treated with immunotherapy are summarized in Table 1.
Table 1
Characteristics | Total |
---|---|
Median age, years [range] | 64 [32–84] |
Sex | |
Male/female | 154 (76.6%)/47 (23.4%) |
Smoking status | |
Smoker/never-smoker | 117 (58.2%)/84 (41.8%) |
EGFR mutation status | |
Yes/no/NA | 28 (13.9%)/162 (80.6%)/11 (5.5%) |
KRAS mutation | |
Yes/no/NA | 47 (23.4%)/95 (47.3%)/59 (29.4%) |
TP53 mutation | |
Yes/no/NA | 70 (34.8%)/44 (21.9%)/87 (43.3%) |
Tumor status | |
Recurrent/primary | 59 (29.4%)/142 (70.6%) |
TNM stage | |
IIIA–IIIC/IV | 30 (14.9%)/171 (85.1%) |
PD-L1 | |
Negative/positive/NA | 40 (19.9%)/83 (41.3%)/78 (38.8%) |
Liver metastases | |
Yes/no | 19 (9.5%)/182 (90.5%) |
Brain metastases | |
Yes/no | 69 (34.3%)/132 (65.7%) |
Location | |
Central/peripheral/NA | 40 (19.9%)/121 (60.2%)/40 (19.9%) |
Adverse effects | |
Yes/no/NA | 87 (43.3%)/47 (23.4%)/67 (33.3%) |
Immunotherapy modalities | |
Immuno+ chemo*/immunotherapy alone/other** | 117 (58.2%)/63 (31.3%)/21 (10.4%) |
Treatment line | |
First-line/second-line and above | 101 (50.2%)/100 (49.8%) |
*, immunotherapy combined with chemotherapy; **, other combination modalities refer to combinations other than immunotherapy combined with chemotherapy, including the combination of bevacizumab, anlotinib, and EGFR-TKIs. EGFR, epidermal growth factor receptor; NA, not available; KRAS, Kirsten rat sarcoma viral oncogene homolog; TP53, tumor protein p53; TNM stage, tumor node metastasis stage; PD-L1, programmed cell death-ligand 1; TKIs, tyrosine kinase inhibitors.
The immunotherapy modalities involved in this study included immune monotherapy (n=63, 31.3%), immunotherapy combined with chemotherapy (n=117, 58.2%), immunotherapy combined with targeted therapy (n=2, 1.0%), immunotherapy combined with chemotherapy and bevacizumab (n=9, 4.5%), immunotherapy combined with bevacizumab (n=5, 2.5%), and immunotherapy combined with anlotinib (n=5, 2.5%).
Efficacy and survival
Univariate and multivariate analyses for PFS
Univariate analysis of the 201 lung adenocarcinoma patients who received immunotherapy showed that clinical factors such as male gender (median PFS of 9.7 vs. 5.1 months, P=0.001), smoker (median PFS of 11.6 vs. 5.9 months, P=0.003), epidermal growth factor receptor (EGFR) wild type (median PFS of 9.8 vs. 2.4 months, P<0.001), KRAS mutation (median PFS of 16.8 vs. 7.6 months, P=0.001), earlier TNM stage (median PFS of 15.9 vs. 7.4 months, P=0.039), PD-L1 positive (median PFS of 9.0 vs. 4.9 months, P=0.004), no liver metastasis (median PFS of 8.6 vs. 4.0 months, P=0.031), immune combined chemotherapy (median PFS of 10.2 vs. 3.5 months, P=0.002), adverse effects (median PFS of patients with AE vs. without AE: 13.5 vs. 1.8 months, P<0.001), and first-line of immunotherapy (median PFS of 10.4 vs. 5.9 months, P=0.003) were significantly associated with improved PFS, compared with female gender, never-smoker, EGFR mutation, KRAS negative, poor TNM stage, PD-L1 negative, liver metastasis, immunotherapy alone, and patients without adverse effects. The univariate analyses of PFS outcomes are shown in Table 2.
Table 2
Variables | Univariate analysis on PFS | Univariate analysis on OS | |||
---|---|---|---|---|---|
Hazard ratio | P value | Hazard ratio | P value | ||
Age (years) | |||||
<60 | 1.016 (95% CI, 0.706–1.462) | 0.933 | 0.638 (95% CI, 0.388–1.047) | 0.072 | |
≥60 | 1 | 1 | |||
Sex | |||||
Male | 0.521 (95% CI, 0.356–0.761) | 0.001 | 0.868 (95% CI, 0.533–1.414) | 0.569 | |
Female | 1 | 1 | |||
Tumor status | |||||
Recurrent | 1.078 (95% CI, 0.740–1.570) | 0.695 | 1.073 (95% CI, 0.675–1.707) | 0.765 | |
Primary | 1 | 1 | |||
Smoking status | |||||
Smoker | 0.595 (95% CI, 0.420–0.843) | 0.003 | 0.826 (95% CI, 0.538–1.269) | 0.382 | |
Never-smoker | 1 | 1 | |||
EGFR mutation | |||||
Yes | 2.766 (95% CI, 1.719–4.452) | <0.001 | 2.698 (95% CI, 1.585–4.591) | <0.001 | |
NA | 1.627 (95% CI, 0.783–3.365) | 0.183 | 3.071 (95% CI, 1.457–6.471) | 0.002 | |
No | 1 | 1 | |||
TP53 mutation | |||||
Yes | 0.929 (95% CI, 0.578–1.492) | 0.758 | 1.014 (95% CI, 0.535–1.925) | 0.965 | |
NA | 1.069 (95% CI, 0.680–1.681) | 0.770 | 1.515 (95% CI, 0.852–2.692) | 0.154 | |
No | 1 | 1 | |||
KRAS mutation | |||||
Yes | 0.449 (95% CI, 0.271–0.743) | 0.001 | 0.788 (95% CI, 0.432–1.440) | 0.438 | |
NA | 0.919 (95% CI, 0.622–1.358) | 0.668 | 1.220 (95% CI, 0.759–1.962) | 0.410 | |
No | 1 | 1 | |||
PD-L1 | |||||
Positive | 0.527 (95% CI, 0.336–0.825) | 0.004 | 0.414 (95%CI, 0.236–0.725) | 0.001 | |
NA | 0.534 (95% CI, 0.342–0.834) | 0.007 | 0.616 (95% CI, 0.368–1.031) | 0.062 | |
Negative | 1 | 1 | |||
TNM stage | |||||
IIIA–IIIC | 0.581 (95% CI, 0.344–0.983) | 0.039 | 0.212 (95% CI, 0.077–0.578) | 0.002 | |
IV | 1 | 1 | |||
Liver metastases | |||||
Yes | 1.801 (95% CI, 1.046–3.102) | 0.031 | 1.670 (95% CI, 0.879–3.173) | 0.113 | |
No | 1 | 1 | |||
Brain metastases | |||||
Yes | 1.271 (95% CI, 0.889–1.818) | 0.186 | 1.252 (95% CI, 0.807–1.942) | 0.315 | |
No | 1 | 1 | |||
Location | |||||
Central | 0.929 (95% CI, 0.596–1.449) | 0.745 | 0.887 (95% CI, 0.499–1.579) | 0.684 | |
NA | 1.025 (95% CI, 0.648–1.619) | 0.916 | 1.222 (95% CI, 0.712–2.096) | 0.466 | |
Peripheral | 1 | 1 | |||
Immunotherapy modalities | |||||
Immuno + chemo* | 0.560 (95% CI, 0.384–0.815) | 0.002 | 0.513 (95% CI, 0.322–0.818) | 0.004 | |
Other combinations** | 0.832 (95% CI, 0.463–1.495) | 0.535 | 1.093 (95% CI, 0.566–2.111) | 0.790 | |
Immunotherapy alone | 1 | 1 | |||
Adverse effects | |||||
Yes | 0.354 (95% CI, 0.228–0.551) | <0.001 | 0.284 (95% CI, 0.165–0.487) | <0.001 | |
NA | 0.551 (95% CI, 0.353–0.860) | 0.007 | 0.588 (95% CI, 0.354–0.976) | 0.037 | |
No | 1 | 1 | |||
Line of immunotherapy | |||||
First-line | 0.596 (95% CI, 0.420–0.845) | 0.003 | 0.655 (95% CI, 0.421–1.020) | 0.059 | |
Second-line and above | 1 | 1 |
*, immunotherapy combined with chemotherapy; **, other combination modalities refer to combinations other than immunotherapy combined with chemotherapy, including the combination of bevacizumab, anlotinib, and EGFR-TKIs. PFS, progression-free survival; OS, overall survival; CI, confidence interval; EGFR, epidermal growth factor receptor; NA, not available; TP53, tumor protein p53; KRAS, Kirsten rat sarcoma viral oncogene homolog; TNM stage, tumor node metastasis stage; PD-L1, programmed cell death-ligand 1.
For multivariate analysis of the entire cohort, Cox proportional hazard multivariable modeling was performed on the variables of those with significant associations confirmed by univariate analysis (sex, smoking status, EGFR mutation, KRAS mutation, PD-L1, TNM stage, liver metastases, immunotherapy modalities, adverse effects and line of immunotherapy) to predict each outcome separately. The results demonstrated a significant PFS benefit for lung adenocarcinoma patients treated with immunotherapy exhibiting the following clinical parameters: smoker (P=0.011), KRAS mutation (P=0.006), first-line of immunotherapy (P=0.009), AEs (P=0.014) (Figure 2). While the patients with PD-L1 positive (PD-L1 TPS ≥1%) had a significantly better trend of PFS than those with PD-L1 negative (PD-L1 TPS <1%) (P=0.052), which was speculated to be false negative due to small sample size and more missing values (Figure 2).
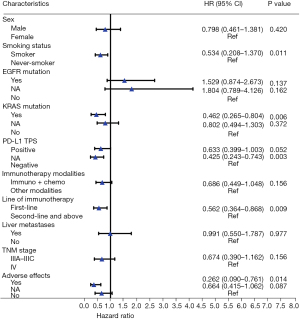
Multivariate analysis of lung adenocarcinoma patients receiving first-line immunotherapy showed that age (P=0.003), sex (P=0.001), tumor status (P=0.015), KRAS mutation (P=0.022), PD-L1 expression (P=0.006), and brain metastases (P=0.027) were independent prognostic factors for PFS (Figure S1A). In addition, multivariate analysis of lung adenocarcinoma patients receiving immunotherapy as second-line and above found that smoking status (P=0.033), EGFR mutation (P=0.003), TP53 mutation (P=0.020), PD-L1 expression (P=0.032), and immunotherapy modalities (P<0.001) are independent prognostic factors that affect PFS (Figure S1B).
Univariate and multivariate analyses for OS
Univariate analysis found that among lung adenocarcinoma patients receiving immunotherapy, those who were EGFR negative (median OS of 27.7 vs. 10.9 months, P<0.001), PD-L1 positive (median OS of not reached vs. 11.7 months, P=0.001), had a TNM stage of IIIA–IIIC (median OS of not reached vs. 20.5 months, P=0.002), received immunotherapy combined with chemotherapy (median OS of 26.9 vs. 15.0 months, P=0.004), and had adverse effects (median OS of not reached vs. 9.3 months, P<0.001) had significantly longer OS (Table 2). In addition, univariate analysis of lung adenocarcinoma patients receiving first-line immunotherapy showed that PD-L1 positive patients had significantly better OS than PD-L1 negative patients (median OS of not reached vs. 13.2 months, HR =0.439, 95% CI: 0.203–0.949, P=0.031, Figure 3A), and stage IIIA–IIIC patients had significantly longer OS than stage IV patients (median OS of not reached vs. 22.4 months, HR =0.244, 95% CI: 0.074–0.803, P=0.012, Figure 3B). Meanwhile, a statistically insignificant trend was observed between patients with and without adverse effects (median OS of not reached vs. 18.8 months, HR =0.431, 95% CI: 0.178–1.045, P=0.055, Figure S2).
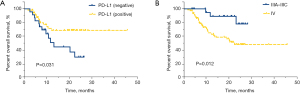
The results of univariate analysis for OS in lung adenocarcinoma patients with second-line and above immunotherapy showed that EGFR wild-type (27.7 vs. 10.9 months, HR =0.393, 95% CI: 0.217–0.712, P=0.001, Figure 4A), PD-L1 positive (not reached vs. 7.6 months, HR =0.381, 95% CI: 0.168–0.866, P=0.017, Figure 4B), immunotherapy combined with chemotherapy (26.9 vs. 13.5 months, HR =0.514, 95% CI: 0.274–0.964, P=0.035, Figure 4C), and adverse reactions (not reached vs. 8.5 months, HR =0.210, 95% CI: 0.104–0.423, P<0.001, Figure 4D) were significantly correlated with improved OS.
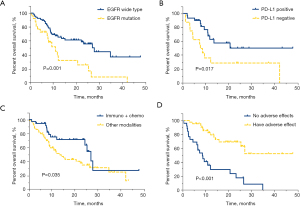
Among lung adenocarcinoma patients who received first-line immunotherapy, multivariate analysis showed that patients younger than 60 years old and PD-L1 positive were significantly associated with longer OS (Figure 5A). Moreover, multivariate analysis of patients receiving second-line and above immunotherapy found that patients younger than 60 years old, male, non-smoking, EGFR wild type, and patients with adverse effects were significantly associated with improved OS (Figure 5B).
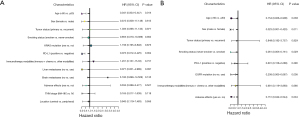
Best response outcomes
The best response outcome results for patients with different clinical characteristic factors are shown in Table 3. The best response outcomes were statistically different in terms of sex (P=0.037), smoking status (P=0.031), EGFR mutation status (P<0.001), KRAS mutation (P<0.001), PD-L1 expression (P=0.001), line of immunotherapy (P<0.001), immunotherapy modalities (P=0.022), and adverse effects (P<0.001).
Table 3
Variables | Number | CR | PR | SD | PD | NA | ORR | DCR | P value |
---|---|---|---|---|---|---|---|---|---|
Age (years) | 0.445 | ||||||||
<60 | 69 | 1 | 20 | 32 | 19 | 0 | 30.43% | 76.81% | |
≥60 | 132 | 0 | 37 | 68 | 26 | 1 | 28.03% | 79.55% | |
Sex | 0.037 | ||||||||
Male | 154 | 1 | 51 | 72 | 29 | 1 | 33.77% | 80.52% | |
Female | 47 | 0 | 6 | 28 | 13 | 0 | 12.77% | 72.34% | |
Tumor status | 0.097 | ||||||||
Recurrent | 59 | 0 | 11 | 35 | 12 | 1 | 18.64% | 77.97% | |
Primary | 142 | 1 | 46 | 65 | 30 | 0 | 33.10% | 78.87% | |
Smoking status | 0.031 | ||||||||
Smoker | 117 | 0 | 40 | 58 | 19 | 0 | 34.19% | 83.76% | |
Never-smoker | 84 | 1 | 17 | 42 | 23 | 1 | 21.43% | 71.43% | |
EGFR mutation | <0.001 | ||||||||
Yes | 28 | 0 | 1 | 13 | 14 | 0 | 3.57% | 50.00% | |
No | 162 | 1 | 53 | 81 | 26 | 1 | 33.33% | 83.33% | |
TP53 mutation | 0.372 | ||||||||
Yes | 70 | 0 | 23 | 33 | 14 | 0 | 32.86% | 80.00% | |
No | 44 | 1 | 10 | 25 | 8 | 0 | 25.00% | 81.82% | |
KRAS mutation | <0.001 | ||||||||
Yes | 47 | 0 | 25 | 17 | 4 | 1 | 53.19% | 89.36% | |
No | 95 | 1 | 17 | 55 | 22 | 0 | 18.95% | 76.84% | |
PD-L1 | 0.001 | ||||||||
Positive | 83 | 1 | 31 | 35 | 16 | 0 | 38.55% | 80.72% | |
Negative | 40 | 0 | 3 | 23 | 14 | 0 | 7.50% | 65.00% | |
TNM stage | 0.052 | ||||||||
IIIA–IIIC | 30 | 0 | 11 | 18 | 1 | 0 | 36.67% | 96.67% | |
IV | 171 | 1 | 46 | 82 | 41 | 1 | 27.49% | 75.44% | |
Liver metastases | 0.325 | ||||||||
Yes | 19 | 0 | 3 | 9 | 7 | 0 | 15.79% | 63.16% | |
No | 182 | 1 | 54 | 91 | 35 | 1 | 30.22% | 80.22% | |
Brain metastases | 0.609 | ||||||||
Yes | 69 | 0 | 18 | 37 | 13 | 1 | 26.09% | 79.71% | |
No | 132 | 1 | 39 | 63 | 29 | 0 | 30.30% | 78.03% | |
Location | 0.490 | ||||||||
Central | 38 | 0 | 15 | 16 | 7 | 0 | 39.47% | 81.58% | |
Peripheral | 121 | 1 | 30 | 63 | 26 | 1 | 25.62% | 77.69% | |
Line of immunotherapy | <0.001 | ||||||||
First-line | 101 | 1 | 42 | 42 | 16 | 0 | 42.6% | 84.2% | |
Second-line and above | 100 | 0 | 15 | 58 | 26 | 1 | 15.0% | 73.0% | |
Immunotherapy modalities | 0.022 | ||||||||
Immuno+chemo* | 117 | 1 | 39 | 62 | 15 | 0 | 34.19% | 87.18% | |
Other combinations** | 21 | 0 | 4 | 11 | 6 | 0 | 19.05% | 71.43% | |
Immunotherapy alone | 63 | 0 | 14 | 27 | 21 | 1 | 22.22% | 65.08% | |
Adverse effects | <0.001 | ||||||||
One AE | 68 | 1 | 26 | 37 | 3 | 1 | 39.71% | 94.12% | |
Two or more AEs | 18 | 0 | 4 | 10 | 4 | 0 | 22.22% | 77.78% | |
No | 47 | 0 | 5 | 18 | 24 | 0 | 10.64% | 48.94% |
*, immunotherapy combined with chemotherapy; **, other combination modalities refer to combinations other than immunotherapy combined with chemotherapy, including the combination of bevacizumab, anlotinib, and EGFR-TKIs. CR, complete response; PR, partial response; SD, stable disease; PD, progressive disease; NA, not available; ORR, objective response rate; DCR, disease control rate; EGFR, epidermal growth factor receptor; TP53, tumor protein p53; KRAS, Kirsten rat sarcoma viral oncogene homolog; TNM stage, tumor node metastasis stage; PD-L1, programmed cell death-ligand 1; AEs, adverse effects.
Nomogram construction and validation of PFS
According to the Cox regression results of PFS in the entire cohort, our model contained the following factors: KRAS mutation, smoking status, PD-L1, lines of immunotherapy and adverse effects. We constructed a nomogram for PFS in lung adenocarcinoma patients who received immunotherapy according to the variables screened (Figure 6A). When using the nomogram to predict the PFS of patients, it is necessary to calculate the scores of each variable and then add the scores of all variables to obtain the total scores of patients. The percentage corresponding to the total score is the predicted probability of reaching PFS in 3 months, 6 months, 1 year, and 2 years.
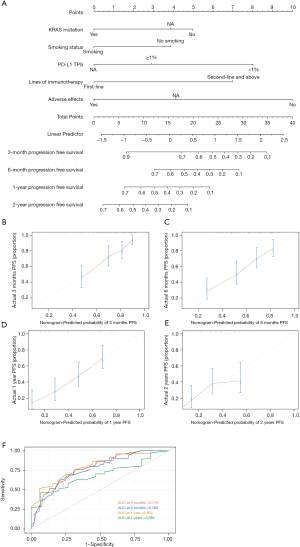
The VIF values were all <2, indicating that no collinearity existed between the variables. The C-index value was 0.714 (95% CI: 0.692–0.736). The calibration curves of the nomogram showed relatively high consistencies between the predicted and observed survival probability in lung adenocarcinoma patients treated with immunotherapy (Figure 6B-6E). The area under the ROC curve (AUC) was 0.779 (95% CI: 0.692–0.867) for 3-month PFS, 0.760 (95% CI: 0.675–0.846) for 6-month PFS, 0.802 (95% CI: 0.717–0.888) for 1-year PFS and 0.689 (95% CI: 0.543–0.831) for 2-year PFS, indicating that this prognostic model performed well as a predictor of PFS (Figure 6F). Therefore, the nomogram for the PFS of lung adenocarcinoma patients receiving immunotherapy showed considerable predictive and calibrating abilities.
Discussion
As the most common pathological type of lung cancer, lung adenocarcinoma seriously affects the health of patients. For lung adenocarcinoma diagnosed in the early stage, complete remission can be achieved through surgery. However, most patients are already at advanced stages at the time of diagnosis, and the 5-year survival rate is less than 20%. Previously, chemotherapy was the most commonly used treatment for patients with advanced lung adenocarcinoma. For lung adenocarcinoma patients with driver-oncogene mutations, such as EGFR and anaplastic lymphoma kinase (ALK) mutations, targeted therapy has greatly improved their outcomes. In recent years, with the rapid development of biomedical technology, numerous immunotherapeutic drugs have been approved for clinical treatment after a series of clinical studies, which has not only restored hope for patients with lung adenocarcinoma but also greatly changed the current lung cancer treatment landscape. However, despite the increasing popularity of immunotherapy in clinical practice, the efficacy of immunotherapy is inconsistent, with some patients receiving a long-term survival benefit, while others are not sensitive to immunotherapy. At present, identifying the potential beneficiaries of immunotherapy is an important clinical problem that remains to be solved.
In this study, the PFS and OS of lung adenocarcinoma patients treated with anti-PD-1/PD-L1 ICIs were statistically analyzed to explore the prognostic differences of lung adenocarcinoma patients with different clinicopathological characteristics after anti-PD-1/PD-L1 ICIs treatment. In addition, a clinical prediction model was established to predict the PFS of lung adenocarcinoma patients treated with ICIs, with the aim of providing a reference for clinical treatment decision-making.
Previous studies on lung cancer have proposed some factors that potentially affect the prognosis of patients with lung adenocarcinoma treated with immunotherapy, such as PD-L1 expression, tumor mutational burden status, smoking status, driver gene mutation status, and so on (14,27-29). These factors were fully considered in our present study. Gainor et al. reported that the degree of smoking in patients is correlated with immunotherapy efficacy. Their study found that never and light smokers had relatively shorter PFS and duration of overall response (DOR) compared to heavy smokers, although the difference was not statistically significant (14). This is consistent with our findings; statistical analysis in the present study showed that PFS was markedly longer in smoking patients with lung adenocarcinoma receiving immunotherapy compared to non-smokers.
In addition, previous studies have explored the relationship between different driver gene mutations and immunotherapy efficacy. Liu et al. confirmed that NSCLC patients with KRAS mutations have notable immunotherapy clinical benefits (30). Furthermore, the clinical study conducted by Skoulidis et al. found that the change of liver kinase B1/serine-threonine kinase 11 (STK11/LKB1) is the most common genomic driver of primary resistance to PD-1 inhibitors in lung adenocarcinoma patients with KRAS mutations (31). In this study, due to the relatively small number of patients enrolled, we did not conduct a subgroup analysis of the specific subtypes of KRAS mutation. Notably, patients with KRAS mutations in this study achieved surprising survival outcomes after immunotherapy.
Previously, the AMG510 clinical study, which included 59 NSCLC patients with KRAS p.G12C mutation, reported a median PFS of 6.3 months (range, 0.0+ to 14.9 months) after receiving the KRAS p.G12C inhibitor, Sotorasib, with an ORR of 32.2% and the disease control rate (DCR) of 88.1% (32). In our study, 14 patients with KRAS p.G12C mutation who received immunotherapy were screened, and the results showed that the mean PFS was 17.649 months [range, 11.607 to 23.690 months; since only five patients (35.7%) suffered disease progression before the last follow-up, the median PFS could not be calculated], the ORR was 57.1%, and the DCR was 100.0%. This result suggests that immunotherapy is a good clinical option for patients with KRAS G12C mutations. However, only 14 patients with KRAS p.G12C mutation were included in this study, and thus, a statistical analysis could not be conducted, as a larger patient population is needed for verification.
In addition, the results of our study showed that patients with TP53 mutations achieved a better OS after received second-line and above immunotherapy. However, a study conducted by Sun et al. found that not all types of TP53 mutations are the same in predicting the efficacy of lung adenocarcinoma patients treated with ICIs. Their study showed that patients with TP53 missense mutations benefited more from anti-PD-L1/PD-1 treatment compared to those with nonsense mutations. However, all patients with either missense or nonsense TP53 mutations responded well to nivolumab plus ipilimumab therapy (33).
Some previous studies have attempted to predict the efficacy of immunotherapy and identify the cohort of patient who would benefit from immunotherapy. Several papers have sought to identify biomarkers that can predict immunotherapy efficacy, such as TMB levels (18), titin (TTN) mutations (34), beta-2-microglobuli (B2M) gene expression (35), EPH receptor A5 (EPHA5) mutations (36), and thymocyte selection-associated high mobility group box (TOX) expression (37). A 17-immune-related genes prognostic model has also been constructed to predict the survival rate and response to ICIs therapy in patients with lung adenocarcinoma (38). Most of these studies have focused on molecular markers, gene regulation, and the immune microenvironment. There is a currently lack of real-world data about how to better predict the efficacy of immunotherapy in clinical practice. Therefore, a prognostic model was constructed in the present study, which aims to determine the ideal patient population for immunotherapy based on the analysis of real-world clinical data and further improve the accuracy of clinicians seeking to identify the beneficiaries of immunotherapy based on existing evidence-based medicine.
However, this study also has some limitations that should be noted. Firstly, the major limitation of this study was its retrospective design. Secondly, the total number of patients enrolled in this study was relatively small. Furthermore, when verifying the predictive model, the simple resampling method was adopted for internal verification due to the small sample size, and thus, the expansion of the model still needs to be verified externally with large samples.
Conclusions
In this study, we found that lung adenocarcinoma patients who were smoker, had KRAS mutations, were treated with first-line immunotherapy and had immune-related adverse reactions were more likely to have a survival benefit after ICI treatment. Also, the nomogram in this study can be used to some extent to predict the prognosis of lung adenocarcinoma patients after receiving immunotherapy.
Acknowledgments
The authors are grateful to all of the patients who contributed to this study. The abstract was presented at the 2022 World Conference on Lung Cancer August 6-9 2022 in Vienna, Austria, and published as an abstract (https://wclc2022.iaslc.org/wp-content/uploads/2022/07/WCLC2022-Abstract-Book.pdf).
Funding: This study was supported by the Chinese Society of Clinical Oncology (CSCO) Tumor Research Fund (No. Y-2019AZZD-0038), the Beijing Medical and health public welfare foundation project (No. YWJKJJHKYJ) and the National Natural Science Foundation of China (No. 82150005).
Footnote
Reporting Checklist: The authors have completed the TRIPOD reporting checklist. Available at https://jtd.amegroups.com/article/view/10.21037/jtd-22-1270/rc
Data Sharing Statement: Available at https://jtd.amegroups.com/article/view/10.21037/jtd-22-1270/dss
Conflicts of Interest: All authors have completed the ICMJE uniform disclosure form (available at https://jtd.amegroups.com/article/view/10.21037/jtd-22-1270/coif). The authors have no conflicts of interest to declare.
Ethical Statement:
Open Access Statement: This is an Open Access article distributed in accordance with the Creative Commons Attribution-NonCommercial-NoDerivs 4.0 International License (CC BY-NC-ND 4.0), which permits the non-commercial replication and distribution of the article with the strict proviso that no changes or edits are made and the original work is properly cited (including links to both the formal publication through the relevant DOI and the license). See: https://creativecommons.org/licenses/by-nc-nd/4.0/.
References
- Sung H, Ferlay J, Siegel RL, et al. Global Cancer Statistics 2020: GLOBOCAN Estimates of Incidence and Mortality Worldwide for 36 Cancers in 185 Countries. CA Cancer J Clin 2021;71:209-49. [Crossref] [PubMed]
- Wang J, Zou K, Feng X, et al. Downregulation of NMI promotes tumor growth and predicts poor prognosis in human lung adenocarcinomas. Mol Cancer 2017;16:158. [Crossref] [PubMed]
- Brahmer J, Reckamp KL, Baas P, et al. Nivolumab versus Docetaxel in Advanced Squamous-Cell Non-Small-Cell Lung Cancer. N Engl J Med 2015;373:123-35. [Crossref] [PubMed]
- Borghaei H, Paz-Ares L, Horn L, et al. Nivolumab versus Docetaxel in Advanced Nonsquamous Non-Small-Cell Lung Cancer. N Engl J Med 2015;373:1627-39. [Crossref] [PubMed]
- Reck M, Rodríguez-Abreu D, Robinson AG, et al. Pembrolizumab versus Chemotherapy for PD-L1-Positive Non-Small-Cell Lung Cancer. N Engl J Med 2016;375:1823-33. [Crossref] [PubMed]
- Mok TSK, Wu YL, Kudaba I, et al. Pembrolizumab versus chemotherapy for previously untreated, PD-L1-expressing, locally advanced or metastatic non-small-cell lung cancer (KEYNOTE-042): a randomised, open-label, controlled, phase 3 trial. Lancet 2019;393:1819-30. [Crossref] [PubMed]
- Garassino MC, Gadgeel S, Esteban E, et al. Patient-reported outcomes following pembrolizumab or placebo plus pemetrexed and platinum in patients with previously untreated, metastatic, non-squamous non-small-cell lung cancer (KEYNOTE-189): a multicentre, double-blind, randomised, placebo-controlled, phase 3 trial. Lancet Oncol 2020;21:387-97. [Crossref] [PubMed]
- Paz-Ares L, Luft A, Vicente D, et al. Pembrolizumab plus Chemotherapy for Squamous Non-Small-Cell Lung Cancer. N Engl J Med 2018;379:2040-51. [Crossref] [PubMed]
- Markham A, Keam SJ. Camrelizumab: First Global Approval. Drugs 2019;79:1355-61. [Crossref] [PubMed]
- Hoy SM. Sintilimab: First Global Approval. Drugs 2019;79:341-6. [Crossref] [PubMed]
- Lee A, Keam SJ. Tislelizumab: First Approval. Drugs 2020;80:617-24. [Crossref] [PubMed]
- Buti S, Bersanelli M, Perrone F, et al. Predictive ability of a drug-based score in patients with advanced non-small-cell lung cancer receiving first-line immunotherapy. Eur J Cancer 2021;150:224-31. [Crossref] [PubMed]
- Mezquita L, Auclin E, Ferrara R, et al. Association of the Lung Immune Prognostic Index With Immune Checkpoint Inhibitor Outcomes in Patients With Advanced Non-Small Cell Lung Cancer. JAMA Oncol 2018;4:351-7. [Crossref] [PubMed]
- Gainor JF, Rizvi H, Jimenez Aguilar E, et al. Clinical activity of programmed cell death 1 (PD-1) blockade in never, light, and heavy smokers with non-small-cell lung cancer and PD-L1 expression ≥50. Ann Oncol 2020;31:404-11. [Crossref] [PubMed]
- Qin BD, Jiao XD, Liu J, et al. The effect of liver metastasis on efficacy of immunotherapy plus chemotherapy in advanced lung cancer. Crit Rev Oncol Hematol 2020;147:102893. [Crossref] [PubMed]
- Zhang D, Li Y, Li H, et al. A Preliminary Study of the Complement Component 1q Levels in Predicting the Efficacy of Combined Immunotherapy in Patients with Lung Cancer. Cancer Manag Res 2021;13:7131-7. [Crossref] [PubMed]
- Ke L, Wang L, Yu J, et al. Prognostic Significance of SUVmax Combined With Lactate Dehydrogenase in Advanced Lung Cancer Patients Treated With Immune Checkpoint Inhibitor Plus Chemotherapy: A Retrospective Study. Front Oncol 2021;11:652312. [Crossref] [PubMed]
- Chan TA, Yarchoan M, Jaffee E, et al. Development of tumor mutation burden as an immunotherapy biomarker: utility for the oncology clinic. Ann Oncol 2019;30:44-56. [Crossref] [PubMed]
- Bagley SJ, Kothari S, Aggarwal C, et al. Pretreatment neutrophil-to-lymphocyte ratio as a marker of outcomes in nivolumab-treated patients with advanced non-small-cell lung cancer. Lung Cancer 2017;106:1-7. [Crossref] [PubMed]
- Nakaya A, Kurata T, Yoshioka H, et al. Neutrophil-to-lymphocyte ratio as an early marker of outcomes in patients with advanced non-small-cell lung cancer treated with nivolumab. Int J Clin Oncol 2018;23:634-40. [Crossref] [PubMed]
- Zhao Q, Li B, Xu Y, et al. Three models that predict the efficacy of immunotherapy in Chinese patients with advanced non-small cell lung cancer. Cancer Med 2021;10:6291-303. [Crossref] [PubMed]
- Gandhi L, Rodríguez-Abreu D, Gadgeel S, et al. Pembrolizumab plus Chemotherapy in Metastatic Non-Small-Cell Lung Cancer. New Engl J Med 2018;378:2078-92. [Crossref] [PubMed]
- Bodor JN, Boumber Y, Borghaei H. Biomarkers for immune checkpoint inhibition in non-small cell lung cancer (NSCLC). Cancer 2020;126:260-70. [Crossref] [PubMed]
- Duma N, Santana-Davila R, Molina JR. Non-Small Cell Lung Cancer: Epidemiology, Screening, Diagnosis, and Treatment. Mayo Clinic proceedings 2019;94:1623-40. [Crossref] [PubMed]
- Yuan S, Xia Y, Shen L, et al. Development of nomograms to predict therapeutic response and prognosis of non-small cell lung cancer patients treated with anti-PD-1 antibody. Cancer Immunol Immunother 2021;70:533-46. [Crossref] [PubMed]
- Zeng H, Huang WW, Liu YJ, et al. Development and Validation of a Nomogram for Predicting Prognosis to Immune Checkpoint Inhibitors Plus Chemotherapy in Patients With Non-Small Cell Lung Cancer. Front Oncol 2021;11:685047. [Crossref] [PubMed]
- Gu M, Xu T, Chang P. KRAS/LKB1 and KRAS/TP53 co-mutations create divergent immune signatures in lung adenocarcinomas. Ther Adv Med Oncol 2021;13:17588359211006950. [Crossref] [PubMed]
- Wu CH, Hwang MJ. Risk stratification for lung adenocarcinoma on EGFR and TP53 mutation status, chemotherapy, and PD-L1 immunotherapy. Cancer Med 2019;8:5850-61. [Crossref] [PubMed]
- Zhou B, Gao S. Construction and validation of a novel immune and tumor mutation burden-based prognostic model in lung adenocarcinoma. Cancer Immunol Immunother 2022;71:1183-97. [Crossref] [PubMed]
- Liu C, Zheng S, Jin R, et al. The superior efficacy of anti-PD-1/PD-L1 immunotherapy in KRAS-mutant non-small cell lung cancer that correlates with an inflammatory phenotype and increased immunogenicity. Cancer Lett 2020;470:95-105. [Crossref] [PubMed]
- Skoulidis F, Goldberg ME, Greenawalt DM, et al. STK11/LKB1 Mutations and PD-1 Inhibitor Resistance in KRAS-Mutant Lung Adenocarcinoma. Cancer Discov 2018;8:822-35. [Crossref] [PubMed]
- Hong DS, Fakih MG, Strickler JH, et al. KRASG12C Inhibition with Sotorasib in Advanced Solid Tumors. N Engl J Med 2020;383:1207-17. [Crossref] [PubMed]
- Sun H, Liu SY, Zhou JY, et al. Specific TP53 subtype as biomarker for immune checkpoint inhibitors in lung adenocarcinoma. EBioMedicine 2020;60:102990. [Crossref] [PubMed]
- Wang Z, Wang C, Lin S, et al. Effect of TTN Mutations on Immune Microenvironment and Efficacy of Immunotherapy in Lung Adenocarcinoma Patients. Front Oncol 2021;11:725292. [Crossref] [PubMed]
- Zhao Y, Cao Y, Chen Y, et al. B2M gene expression shapes the immune landscape of lung adenocarcinoma and determines the response to immunotherapy. Immunology 2021;164:507-23. [Crossref] [PubMed]
- Chen Z, Chen J, Ren D, et al. EPHA5 mutations predict survival after immunotherapy in lung adenocarcinoma. Aging (Albany NY) 2020;13:598-618. [Crossref] [PubMed]
- Guo L, Li X, Liu R, et al. TOX correlates with prognosis, immune infiltration, and T cells exhaustion in lung adenocarcinoma. Cancer Med 2020;9:6694-709. [Crossref] [PubMed]
- Yi M, Li A, Zhou L, et al. Immune signature-based risk stratification and prediction of immune checkpoint inhibitor's efficacy for lung adenocarcinoma. Cancer Immunol Immunother 2021;70:1705-19. [Crossref] [PubMed]
(English Language Editor: A. Kassem)