Characterization of driver mutations in Chinese non-small cell lung cancer patients using a novel targeted sequencing panel
Introduction
As one of the common malignant epithelial tumors, lung cancer can be classified into small cell lung cancer (SCLC) and non-small cell lung cancer (NSCLC) according to their distinct histopathological characteristics (1). NSCLC accounts for 80–85% of total lung cancer, which can be further divided into lung adenocarcinoma (LUAD), lung squamous cell carcinoma (LUSC) and large cell lung cancer (LCLC) (2). Because of the high incidence rate and the fact that many patients already have advanced lung cancer at the time of diagnosis, lung cancer has become the leading cause of cancer-related death worldwide (3). Therefore, there is an urgent need to deeply understand the molecular pathogenesis of this deadly disease in order to develop novel therapeutic strategies to improve prognosis of lung cancer patients.
Many driver genes have been identified in NSCLC, and driver mutations on these genes were reported to play key roles in NSCLC tumorigenesis and development by affecting core cellular signaling pathways like cell proliferation and survival (4). Luckily, some therapies have been developed to specifically target these driver mutations, such as EGFR exon-19 del, exon-21 L858R, KRAS G12C and BRAF V600E mutations, and a subgroup of NSCLC patients who harbor these mutations can significantly get better clinical outcome after targeted therapies (5). Thus to improve overall prognosis for NSCLC patients, it is necessary to identify patients who can benefit from targeted therapies through efficient and specific detection methods for driver mutations (6).
With the development of next-generation sequencing (NGS) in the 21th century, simultaneous sequencing of millions of tumor sample-derived DNA fragments can be achieved, after data processing and variant calling, driver mutations can be identified in individual tumors (7). Currently there are three sequencing methods including whole-genome sequencing (WGS), whole-exome sequencing (WES) and targeted-panel sequencing (TPS), and all of them can be used to detect driver mutations but the balance between benefit and cost should be considered to select the specific method for clinical application (8). The advantage of WGS and WES is that they can uncover mutational spectrum of the whole genome or exome with little probability of missing important mutations, but the high cost and complicate data analysis process greatly limit their application in clinical diagnosis (9-11). In contrast, TPS only focuses on a certain number of genes with strong clinical relevance and also simplifies the subsequent data analysis procedure, and has been wildly utilized in clinic (12).
However, the cost of TPS still remains a heavy burden for both the NSCLC patients and the whole medical system (13,14), especially for developing countries like China, WES and large gene panels including hundreds of genes may not be applicable to most NSCLC patients. It is important to design a clinically cost-effective gene panel, which contains most important genes closely related to cancer treatment and prognosis but excludes redundant genes (15). Some targeted panels have already been designed for NSCLC patients (16,17), but most of them were examined and applied in cohorts mainly composed of western population. Due to some reported difference on NSCLC mutational spectrum between Chinese patients and western patients (18,19), it may not be reasonable to directly apply those panels for Chinese patients. In order to design a small-size but effective sequencing panel suitable for Chinese patients, the genes should be selected and adjusted according to Chinese NSCLC mutational characteristics. Therefore, we want to develop a drive gene-based sequencing panel, examine its reliability and effectiveness in a Chinese NSCLC cohort, and further promote its clinical application.
In this study, we first selected 21 driver genes with both high prevalence and clear oncogenic role according to public cancer databases, and used this 21-gene panel to sequence 134 LUAD patients and 126 LUSC patients in Peking Union Medical College Hospital (PUMCH) cohort. Next, we comprehensively analyzed the sequencing result, not only checked the frequency of driver mutations, but also deciphered the downstream pathway alteration and clinical utility, and further performed inter-subtype and inter-cohort comparison. Overall, we have provided a novel gene sequencing panel to characterize driver mutations and guide clinical treatment for Chinese NSCLC patients. We present the following article in accordance with the STROBE reporting checklist (available at https://jtd.amegroups.com/article/view/10.21037/jtd-22-909/rc).
Methods
Cohort design and sample collection
We established a Chinese NSCLC sequencing cohort composed of 260 patients with complete surgically resected NSCLC at Peking Union Medical College Hospital (PUMCH) from 2009 to 2011. The study was conducted in accordance with the Declaration of Helsinki (as revised in 2013). The study was approved by the ethics committee of the Peking Union Medical College Hospital (No. JS-1410) and informed consent was taken from all the patients. The clinical characteristics were obtained and summarized in Supplementary file (available online: https://cdn.amegroups.cn/static/public/jtd-22-909-1.xlsx), the resected tumor tissues were collected for pathological diagnosis. Only samples with definite diagnosis of adenocarcinoma or squamous cell carcinoma were sent for DNA sequencing, while other rare NSCLC subtypes like adenosquamous carcinoma or large cell lung carcinoma were excluded.
DNA extraction, library preparation and sequencing
Formalin-fixed paraffin-embedded (FFPE) tumor tissue was collected from each patient to extract DNA. The DNA was extracted with TIANquick FFPE DNA Kit (TianGen Biotech Co. Ltd.) according to the manual. The DNA samples were amplified by two rounds of PCR, and the primers used were summarized in Supplementary file (available online: https://cdn.amegroups.cn/static/public/jtd-22-909-1.xlsx). The mixture of the second round PCR were purified by the magnetic particle of the Agencourt AMPure XP. Then the PCR product of each samples were quantified and mixed as the same concentration. The library of the mixed PCR product was constructed by Illumina TruSeq DNA PCR-Free kit based on the instruction, and then sequenced by the Illumina NextSeq 500.
Sequencing data processing
The raw paired-end reads obtained from Illumina were merged by PEAR software (v0.9.6), and then aligned to human reference genome (hg19) by Burrows-Wheeler Aligner (BWA) software (v0.7.10). The genetic variants were called by the samtools software (v1.0), and the minimum mutation allele frequency for SNVs and INDELs was set to 10%. All the variants were manually checked on the Integrative Genomics Viewer, and included in Supplementary file (available online: https://cdn.amegroups.cn/static/public/jtd-22-909-1.xlsx).
Downstream data analysis
The genetic variants identified above were uploaded to Cancer Genome Interpreter (CGI) (https://www.cancergenomeinterpreter.org) (20), and classified into driver mutations, passenger mutations and non-protein affecting variants. In addition, the information of druggable levels to targeted therapies was also obtained from CGI according to driver mutation status. The driver mutations were further uploaded to PathScore (http://pathscore.publichealth.yale.edu) (21), to identify the significantly altered pathways in patients (P value <0.01, effect size >100).
Statistical analysis
All statistical analyses were performed by GraphPad Prism software version 9.3 and R version 3.6.1. Categorical variables were expressed as numbers (percentages), two-sided Fisher’s exact test was performed to compare any two groups, and P<0.05 was considered significant. The significance of mutual-exclusivity/co-occurrence was calculated by cBioPortal (https://www.cbioportal.org).
Results
The establishment of the NSCLC target sequencing panel clinical cohort.
To develop a comprehensive sequencing panel including the most meaningful cancer driver genes in NSCLC, we have utilized two public cancer mutation databases [COSMIC (22) and OncoKB (23)] to select the genes harboring mutations with both significant prevalence and well-identified roles in oncogenesis. Specifically, our panel were composed of 21 genes including ALK, AKT1, BRAF, CDKN2A, DDR2, ERBB2, EGFR, FGFR1, FGFR2, IDH1, KRAS, MAP2K1, MET, NRAS, PDGFRA, PIK3CA, PTEN, RET, ROS1, STK11 and TP53, which had mutation frequency above 1% in the lung cancer samples of COSMIC database, and 17 genes of them were identified as oncogenes and the other 4 genes were identified as tumor suppressor genes by OncoKB database (Figure 1). Moreover, we overlapped the 21-gene panel with other 4 previously published NSCLC sequencing panel which have similar size to ours (24-27), the overlapping results indicated that our panel has not only shared core driver genes (TP53, EGFR, PIK3CA, etc.) with them, but also uniquely covered some clinically important genes (DDR2, ROS1, IDH1, etc.), indicating the robustness of our panel (Figure S1). Given the rationale above, we next decided to perform targeted exon sequencing of the 21 genes in our PUMCH NSCLC cohort (Figure 2). The cohort enrolled a total of 260 Chinese NSCLC patients, including 134 LUAD patients and 126 LUSC patients, and their clinical characteristics, such as age at surgery, gender, smoking status etc. were summarized in Table 1.
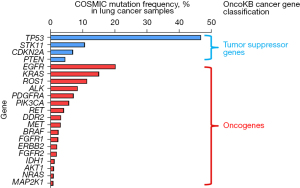
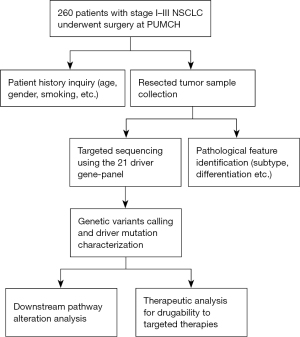
Table 1
Variable | Adenocarcinoma (N=134) | Squamous cell carcinoma (N=126) |
---|---|---|
Age at surgery (years) | ||
Median | 61 | 63 |
Range | 35–82 | 42–82 |
Sex, n (%) | ||
Male | 59 (44.0) | 110 (87.3) |
Female | 75 (56.0) | 16 (12.7) |
Smoking status, n (%) | ||
Current smoker | 43 (32.1) | 84 (66.7) |
Former smoker | 4 (3.0) | 15 (11.9) |
Never smoker | 87 (64.9) | 27 (21.4) |
Tumor stage, n (%) | ||
I | 96 (71.6) | 59 (46.8) |
II | 19 (14.2) | 29 (23.0) |
III | 19 (14.2) | 38 (30.2) |
T stage, n (%) | ||
T1 | 68 (50.7) | 28 (22.2) |
T2 | 51 (38.1) | 69 (54.8) |
T3 | 8 (6.0) | 24 (19.0) |
T4 | 7 (5.2) | 5 (4.0) |
N stage, n (%) | ||
N0 | 106 (79.1) | 75 (59.5) |
N1 | 15 (11.2) | 19 (15.1) |
N2 | 12 (9.0) | 30 (23.8) |
N3 | 1 (0.7) | 2 (1.6) |
M stage, n (%) | ||
M0 | 134 (100.0) | 126 (100.0) |
Differentiation, n (%) | ||
Poorly differentiated | 28 (20.9) | 46 (36.5) |
Moderately differentiated | 48 (35.8) | 70 (55.6) |
Highly differentiated | 58 (43.3) | 10 (7.9) |
NSCLC, non-small cell lung cancer; T/N/M stages were identified according to the 8th edition of TNM Staging of Lung Cancer by American Joint Committee of Cancer. T reflects the features/extent of the primary tumor, N reflects regional lymph node(s) involvement, and M reflects distant metastases.
Characterization of genetic variants in LUAD cohort
A total of 127 types of genetic variants on 17 genes except AKT1, RET, ROS1 and DDR2 were identified in LUAD cohort, and further classified into 58.27% driver mutations, 25.20% passenger mutations and 16.53% non-protein affecting variants by Cancer Genome Interpreter (CGI) (20) (Figure 3A, available online: https://cdn.amegroups.cn/static/public/jtd-22-909-1.xlsx). Next, we analyzed the mutation status for each gene specifically, including the number of patients with variants (Figure 3B) and the number of variant types (Figure S2A). The results revealed 59% (79/134) of LUAD patients harbored genetic variants on the EGFR gene, suggesting it as the most frequently altered gene in LUAD. Other frequently altered genes which affected more than 10% of patients included PDGFRA (35%), TP53 (34%), PIK3CA (19%), CDKN2A (16%), ALK (13%) and FGFR2 (12%). Notably, most of the patients with genetic variants on EGFR, TP53, PIK3CA, CDKN2A and FGFR2 harbored driver mutations on these genes, contrast to the patients with altered PDGFRA and ALK who had mainly passenger mutations and non-protein affecting variants respectively. As for variant types, the TP53 gene had the most variant types among the 17 altered genes, accounting for 35% (45/127) of all identified ones. Besides TP53, the EGFR and CDKN2A genes also had more than 10 identified variants types, indicating that the variants on these genes were distributed in a variety of subtypes rather than enriched in a few ones. To further explore the interactions between any pair of these genes, we performed mutation co-occurrence/mutual-exclusivity analysis for the genes with mutation frequency above 5% (Figure 3C). The analysis results highlighted the significant co-occurrence between PIK3CA-FGFR2, CDKN2A-BRAF, EGFR-BRAF, EGFR-CDKN2A, CDKN2A-PDFGRA pairs, suggesting the potential mechanical cooperation between these two co-occurring mutations.
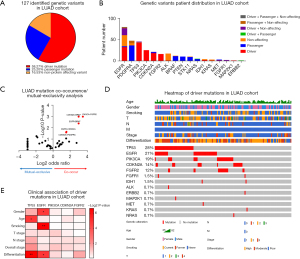
Given that compared to passenger mutations and non-protein affecting variants, driver mutations play more predominant roles in promoting tumorigenesis and clinical progression (28,29), we therefore focused on driver mutations for further analysis on their clinical association, downstream pathway alteration and therapeutic utility. The information about driver mutation, age, gender, smoking, stage and differentiation of 134 LUAD patients were visualized in the heatmap (Figure 3D). The statistical association between driver mutation and other clinical characteristics were analyzed on the genes with driver mutation frequency above 5% including TP53, EGFR, PIK3CA, CDKN2A and FGFR2 (Figure 3E, available online: https://cdn.amegroups.cn/static/public/jtd-22-909-1.xlsx). In particular, the occurrence of driver mutations on the TP53 gene was significantly associated with age and differentiation, with an enrichment in younger group and poor differentiation group respectively. And the occurrence of driver mutations on the EGFR gene was significantly associated with gender and smoking, with an enrichment in female group and never smoker group respectively.
Characterization of genetic variants in LUSC cohort
We analyzed the genetic variants in LUSC cohort in the same way as we did for LUAD cohort. A total of 115 types of genetic variants on 18 genes except MAP2K1, ROS1 and RET were identified in LUSC cohort, and further classified into 54.78% driver mutations, 29.57% passenger mutations and 15.65% non-protein affecting variants by CGI (Figure 4A, available online: https://cdn.amegroups.cn/static/public/jtd-22-909-1.xlsx). Next, we analyzed the mutation status for each gene specifically, including the number of patients with variants (Figure 4B) and the number of variant types (Figure S2B). The results revealed 56.3% (71/126) of LUSC patients harbored genetic variants on the EGFR gene, suggesting it as the most frequently altered gene in LUSC. Other frequently altered genes which affected more than 10% of patients included TP53 (48%), PDGFRA (44%), PIK3CA (16%), CDKN2A (16%), PTEN (14%) and STK11 (10%). Notably, most of the patients with genetic variants on TP53, PIK3CA, and CDKN2A harbored driver mutations on these genes, contrast to the patients with altered EGFR, PDGFRA, PTEN and STK11 who had mainly passenger mutations or non-protein affecting variants. As for variant types, the TP53 gene had the most variant types among the 18 altered genes, accounting for 43% (50/115) of all identified ones. Besides TP53, the EGFR gene also had more than 10 identified variants types, indicating that the variants on these genes were distributed in a variety of subtypes rather than enriched in a few ones. To further explore the interactions between any pair of these genes, we performed mutation co-occurrence/mutual-exclusivity analysis for the genes with mutation frequency above 5% (Figure 4C). The analysis results highlighted the significant co-occurrence between PDGFRA-TP53, TP53-CDKN2A, PDGFRA-CDKN2A, PIK3CA-FGFR2 mutation pairs, suggesting the potential mechanical cooperation between these two co-occurring mutations. In contrast, EGFR-FGFR2 mutation pair showed mutual-exclusivity which may represent functional redundancy or antagonism between the two mutations.
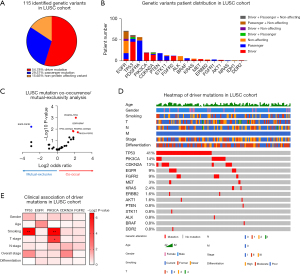
Next, we focused on driver mutations for further analysis on their clinical association, downstream pathway alteration and therapeutic utility. The information about driver mutation, age, gender, smoking, stage and differentiation of 126 LUSC patients were visualized in the heatmap (Figure 4D). The statistical association between driver mutation and other clinical characteristics were analyzed on the genes with driver mutation frequency above 5% including TP53, EGFR, PIK3CA, CDKN2A and FGFR2 (Figure 4E, available online: https://cdn.amegroups.cn/static/public/jtd-22-909-1.xlsx). In particular, the occurrence of driver mutations on the TP53 gene was significantly associated with smoking, with an enrichment in current and former smoker groups. And the occurrence of driver mutations on the PIK3CA gene was significantly associated with smoking and T stage, with an enrichment in current and former smoker groups and T3 stage group respectively.
Inter-subtype comparison of driver mutations and altered pathways in PUMCH LUAD and LUSC cohorts
Although as two subtypes of NSCLC, LUAD and LUSC share some common characteristics, the inter-subtype differences in mutation landscapes and downstream pathway alterations leading to different clinical outcomes should be taken into consideration (30,31), thus we compared driver mutations and altered pathways between our LUAD and LUSC cohorts to identify their similarities and differences. First, the percentage of patients with driver mutations was similar, about 35% of patients didn’t have any identified driver mutations, while the other 65% of patients had at least one driver mutation, most of the latter had one or two and a few had three to four driver mutations (Figure 5A). The overlap of identified driver mutations revealed that driver mutations on 9 genes including EGFR, TP53, PIK3CA, CDKN2A, FGFR2, ALK, KRAS, MET and ERBB2 were shared between LUAD and LUSC cohorts, while driver mutations on other 9 genes were unique in one subtype including LUAD-unique IDH1, NRAS, FGFR1 and MAP2K1 driver mutations and LUSC-unique PTEN, STK11, BRAF, AKT1 and DDR2 driver mutations (Figure 5B). Moreover, the frequency comparison of top 5 driver mutations exhibited that TP53 driver mutations were more significantly enriched in LUSC patients while EGFR driver mutations showed the opposite trend (Figure 5C). In addition to the comparison on gene level, through the application of PathScore (21), a web tool which can quantify the enrichment of altered pathways associated with certain gene mutations, 59 and 54 significantly altered pathways were uncovered in LUAD and LUSC cohorts respectively. Among these altered pathways, 38 pathways were commonly altered in both LUAD and LUSC cohorts, 21 pathways were uniquely altered in LUAD cohort and 16 pathways were uniquely altered in LUSC cohort (Figure 5D,5E, available online: https://cdn.amegroups.cn/static/public/jtd-22-909-1.xlsx). The pathways shared between two cohorts, like ‘G1_AND_S1_PHASES’, ‘ACTIVATION_OF_BH3_ONLY_PROTEINS’ and ‘ARF_PATHWAY’, may represent consensus cancer-driving pathways in NSCLC, while subtype-unique pathways may confer specific functions to each subtype, like LUAD-unique pathway ‘SHC1_EVENTS_IN_EGFR_SIGNALING’, ‘SYNDECAN_3_PATHWAY’ and ‘EGFR_SMRTE_PATHWAY’, and LUSC-unique pathway ‘P38_ALPHA_BETA_DOWNSTREAM_PATHWAY’, ‘AURORA_A_PATHWAY’ and ‘ATRBRCA_PATHWAY’.
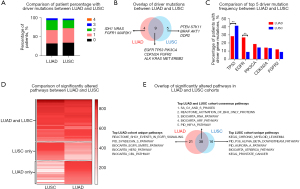
Inter-cohort comparison of driver mutations and altered pathways in different LUAD and LUSC cohorts
To further examine whether the findings in our cohort can be reproduced in other NSCLC cohorts, we followed the same procedure to analyze driver mutations in the OrigiMed and TCGA NSCLC cohorts mainly composed of Chinese and Caucasian patients respectively, and then compared our results with them. First, we compared the driver mutation frequency in LUAD among three cohorts (Figure 6A), and overlapped the top 5 frequent driver mutations in each cohort (Figure 6B). The results revealed that TP53 and EGFR driver mutations were identified as top frequent mutations in all three cohorts, suggesting them as classic driver mutations in both Chinese and Caucasian LUAD patients. Notably, PIK3CA and CDKN2A driver mutations occurred more frequently in PUMCH and OrigiMed cohorts than TCGA cohort, which may represent Chinese LUAD patients’ unique features. Moreover, FGFR2 driver mutation was only frequently enriched in PUMCH LUAD cohort but not in other two LUAD cohorts, which may be caused by cancer heterogeneity of different patients or the different sequencing methods. Next, we compared the significantly altered pathways in each LUAD cohort (Figure 6C, available online: https://cdn.amegroups.cn/static/public/jtd-22-909-1.xlsx), and checked the overlap among them (Figure 6D). There were 27 pathways significantly altered in all three LUAD cohorts like ‘G1_AND_S1_PHASES’ pathway, representing consensus cancer-driving pathways in LUAD. Other 15 pathways like ‘TFF_PATHWAY’ were significantly altered in PUMCH and OrigiMed cohorts but not TCGA cohort, which may reflect functional changes unique in Chinese LUAD patients, and 16 pathways like ‘FGFR2_LIGAND_BINDING_AND_ACTIVATION’ only showed significant alteration in PUMCH LUAD cohort but not in other two LUAD cohorts maybe due to tumor heterogeneity or sequencing method difference. Similar analysis and comparison were performed in LUSC among PUMCH, OrigiMed and TCGA cohorts. The driver mutation analysis identified TP53, PIK3CA and CDKN2A driver mutations as top frequent mutations in all three cohorts, suggesting them as classic driver mutations in both Chinese and Caucasian LUSC patients (Figure 6E,6F). Moreover, FGFR2 and EGFR driver mutations were only frequently enriched in PUMCH LUSC cohort but not in other two LUSC cohorts. The downstream pathway analysis uncovered 41 significantly altered pathways in all three LUSC cohorts like ‘G1_AND_S1_PHASES’ pathway, representing consensus cancer-driving pathways in LUSC, while 13 pathways like ‘FGFR2_LIGAND_BINDING_AND_ACTIVATION’ were uniquely altered in PUMCH LUSC cohort (Figure 6G,6H, available online: https://cdn.amegroups.cn/static/public/jtd-22-909-1.xlsx). Taken together, the inter-cohort comparison of driver mutations and downstream pathways exhibited robust overlap between our PUMCH cohort and other two NSCLC cohorts, verifying the reliability of our sequencing panel. Interestingly, some Chinese cohort-unique or PUMCH cohort-unique driver mutations or altered pathways were identified, which may represent intertumoral heterogeneity at patient and cohort level, and require further exploration.
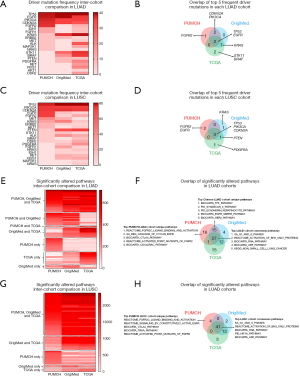
Therapeutic utility of driver mutations detected in LUAD and LUSC cohorts
Besides downstream pathway alterations caused by these driver mutations, we were also interested in their therapeutic utility which may provide guidance for personalized treatment. Using Cancer Biomarkers database on CGI, we could get the information including the patients with targetable driver mutations as well as druggable levels to certain targeted therapies. Level A corresponds to targets of FDA approved drugs, and levels B, C and D correspond to targets verified in clinical trials, case studies and pre-clinical studies respectively. The results showed that among 134 LUAD patients, 26.81%, 18.84%, 11.60% and 1.45% of them had level A, B, C and D druggable mutations to targeted therapy respectively, while other 41.30% had no predicted druggable mutations to targeted therapies (Figure 7A, available online: https://cdn.amegroups.cn/static/public/jtd-22-909-1.xlsx). Specifically, 37 patients carried level A targetable driver mutations, including ALK V1180L mutation and EGFR G719S, S768I, L858R, L861Q and exon 19 del mutations, other 84 patients carried level B–D targetable driver mutations, including mutations on KRAS, ERBB2, FGFR1, MET, TP53, MAP2K1, PIK3CA, NRAS and FGFR2 genes (Table 2). Among 126 LUSC patients, 10.32%, 37.30%, 9.52% and 1.59% of them had level A, B, C and D druggable mutations to targeted therapy respectively, while other 41.27% had no predicted druggable mutations to targeted therapies (Figure 7B, available online: https://cdn.amegroups.cn/static/public/jtd-22-909-1.xlsx). Specifically, 13 patients carried level A targetable driver mutations, including ALK D1203N mutation, BRAF D594N mutation and EGFR H709A, G719A, K754E, H773Y, L858R and exon 19 del mutations, other 104 patients carried level B-D targetable driver mutations, including mutations on KRAS, ERBB2, MET, TP53, DDR2, PIK3CA, FGFR2 and STK11 genes (Table 3).
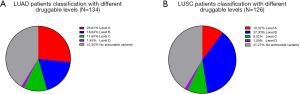
Table 2
Level | Gene | Targetable mutations | Patients | Sensitive therapies |
---|---|---|---|---|
A | ALK | V1180I | 1 | Alectinib, Crizotinib, Brigatinib |
EGFR | G719S, S768I, L858R, L861Q, exon19 del | 36 | Afatinib, Erlotinib, Gefitinib, Osimertinib | |
B | KRAS | G12C | 1 | Trametinib, Lysergide, Abemaciclib |
ERBB2 | L786Q, R811W, E812K | 1 | Trastuzumab, Dacomitinib | |
FGFR1 | A290T, P293L | 2 | Dovitinib, Nvp-Bgj398 | |
MET | R1040Q | 1 | Crizotinib | |
TP53 | M169I, A159P, V173L/M, P250L, E294X, V172F, R267Q, R283C, P278L, C275W/R, R273H, G266V, I255F, R249S, R248Q, G245D/S, S241T, Y220C, V216X, R213L, G199V, R181C, H179R, H168R, T155N/P | 36 | Adjuvant Chemotherapy | |
C | MAP2K1 | K57N | 1 | Cobimetinib + Trametinib |
PIK3CA | E545A, N1044K, M1040I | 25 | Pi-103 | |
D | NRAS | Q43P | 1 | Selumetinib + Trametinib, Erlotinib |
FGFR2 | S282C | 16 | FGFR inhibitors |
Level A: corresponds to biomarkers used in professional guidelines of FDA approved drugs; Level B: groups biomarkers observed in clinical trials; Level C: corresponds to biomarkers identified from small group studies or case studies; Level D: biomarkers have been identified in pre-clinical studies. LUAD, lung adenocarcinoma.
Table 3
Level | Gene | Targetable mutations | Patients | Sensitive therapies |
---|---|---|---|---|
A | ALK | D1203N | 1 | Alectinib, Crizotinib, Brigatinib |
BRAF | D594N | 1 | Dabrafenib, Dabrafenib + Trametinib | |
EGFR | H709A, H773Y, G719A, K754E, L858R, exon19 del | 11 | Afatinib, Erlotinib, Gefitinib, Osimertinib | |
B | KRAS | G12D | 1 | Trametinib, Lysergide, Abemaciclib |
ERBB2 | I788S, V810A | 2 | Trastuzumab, Dacomitinib | |
MET | H997Y, A1004V, T1010I | 4 | Crizotinib | |
TP53 | P301X, V157F, G245D, P151S, C176F, G245C, R248Q, T155P, H179R, P190L, E294*, D281N, P278A, R280*, P278L, C277R, R273S, V272L, R267Q, G266V, R249S, G245C, C242*/Y, N239S, C238*/F, M237I, Y236C, Y234C, E221*, H214R, G199V, R196*, I162F, H178QX, R175H, E171*, GTRVRA154-159X, A159P | 51 | Adjuvant chemotherapy | |
C | DDR2 | V775G | 1 | Erlotinib + Dasatinib |
PIK3CA | E545A/K, Q546K, N1044S | 18 | Pi-103 | |
D | FGFR2 | S282C, G302R | 11 | FGFR inhibitors |
STK11 | ADE347-349A | 16 | MEK inhibitors, SRC inhibitor + PI3K/MEK inhibitors, Phenformin |
Level A: corresponds to biomarkers used in professional guidelines of FDA approved drugs; Level B: groups biomarkers observed in clinical trials; Level C: corresponds to biomarkers identified from small group studies or case studies; Level D: biomarkers have been identified in pre-clinical studies. LUSC, lung squamous cell carcinoma; FGFR, fibroblast growth factor receptor; MEK, mitogenactivated protein kinase; SRC, Proto-oncogene tyrosine-protein kinase; PI3K, phosphatidylinositol 3-kinase.
Discussion
NSCLC is a malignant disease highly associated with genetic alterations, and it is of great significance to decipher the driver mutation spectrum of NSCLC patients for both diagnosis and treatment. Our study performed comprehensive profiling of driver mutations in a Chinese NSCLC cohort using a driver gene-based sequencing panel, which improved the understanding of pathway alterations and therapeutic insights underlying driver mutations of Chinese NSCLC patients. Moreover, the comparison between our in-house cohort with OrigiMed cohort and TCGA cohort further revealed the inter-cohort similarity and difference of driver mutations and downstream pathway alterations, which could provide useful insights for the understanding of NSCLC tumor heterogeneity.
In our comparative analysis between Chinese LUAD and LUSC patients, we have revealed their similarities and differences in driver mutations and downstream pathway alterations. We found that the driver mutations on TP53, EGFR, PIK3CA, CDKN2A and FGFR2 genes occurred most frequently in both LUAD and LUSC, while TP53 mutation was more frequent in LUSC and EGFR mutation was more frequent in LUAD. These findings were generally consistent with some previously reported large-scale sequencing results. For instance, Campbell et al. analyzed the sequencing results of 660 LUAD and 484 LUSC samples and reported that TP53, RB1, ARID1A, CDKN2A, PIK3CA and NF1 genes were significantly mutated in both LUAD and LUSC (32). Except for RB1, ARID1A and NF1 which were not included in our panel, the enrichment of the other three gene mutations TP53, CDKN2A and PIK3CA was consistent with our result, and they also observed the higher enrichment of at TP53 mutation and EGFR mutation in LUSC and LUAD respectively. At downstream pathway level, we identified pathways like ‘G1 and S phases’ as significantly altered pathways in both LUAD and LUSC, stressing the core role of disrupted cell cycle regulation in driving oncogenesis, which was in concordance with previous molecular and cellular studies (33-35). Eymin et al. have thoroughly reviewed the dysregulation of major cell cycle regulators in lung cancer, and pointed out that these cell cycle abnormalities contributed to uncontrolled cellular proliferation and were crucial events for lung cancer oncogenesis (36). Although we found the majority of altered pathways in LUAD and LUSC were shared between them, some altered pathways were subtype-unique, like ‘SHC1_EVENTS_IN_EGFR_SIGNALING’ in LUAD and ‘P38_ALPHA_BETA_DOWNSTREAM PATHWAY’ in LUSC, indicating that LUAD and LUSC might be more dependent on the pathways activated by EGFR and p38, respectively. These findings were consistent with previous studies on the unique roles of EGFR activation in LUAD (37,38) as well as p38 activation in LUSC (39,40), reflecting the difference of oncogenic molecular mechanism between LUAD and LUSC.
Cancer is a highly heterogeneous disease due to genomic stability and clonal evolution, tumor heterogeneity including intratumoral heterogeneity and intertumoral heterogeneity has been recognized as one of the major obstacles for cancer diagnosis and treatment (41-43). The intertumoral heterogeneity represents the heterogeneity among patients harboring tumors of the same histological type, and can be influenced by patient-specific factors like germline genetic variations and environmental factors (44,45). To further understand the genetic intertumoral heterogeneity of LUAD and LUSC at patient and cohort level, we explored the concordance of driver gene mutational spectrum among PUMCH, TCGA and OrigiMed cohorts. We found the high mutation frequency of the driver gene TP53 was consistently identified not only in two NSCLC subtypes, but also in three NSCLC cohorts. Some downstream pathways like ‘G1_AND_S_PHASES’ and ‘ACTIVATION_OF_BH3_ONLY_PROTEINS’ also showed highly consistent alterations among different subtypes and cohorts. Despite these inter-cohort consistencies, there were still many differences among different cohorts. For instance, we observed that the driver mutations on the EGFR gene and downstream pathway alterations were mainly enriched in two Chinese LUAD cohorts, which was consistent with previous reports showing higher EGFR mutation rate in Asians than Caucasians (46). In addition, we also found the unique enrichment of FGFR2 mutation and pathway alterations in PUMCH NSCLC cohorts, but not in other two cohorts. These findings reflected the intertumoral heterogeneity not only between Chinese and Caucasian NSCLC cohorts, but also between Chinese NSCLC cohorts, which highlighted the importance of characterizing the driver gene mutation spectrum of Chinese NSCLC patients through comprehensive genetic sequencing studies.
Lastly, we sought to further explore the therapeutic utility of our sequencing panel, by using the sequencing results to guide the selection of targeted therapies clinical treatment. In recent years, great progress has been made in the field of targeted therapies for NSCLC, many small molecule inhibitors have been designed and proved to have therapeutic effects for a subgroup of patients by specifically diminishing growth of the tumors with targetable mutations (47,48). Therefore, it is important to decipher the gene mutational spectrum of NSCLC patients, so that the patients who carry druggable mutations can be selected to receive personalized targeted therapy. We performed integrative analysis of the driver mutations carried by the patients and the sensitivity to targeted therapies, and found different levels of evidence of sensitivity to targeted therapies for about 60% of the patients in both LUAD and LUSC cohorts. In particular, some of the patients had Level A evidence for driver mutations like EGFR, ALK and BRAF mutations, which means they can get clear clinical benefits from the targeted therapy approved by FDA. Taking the classic EGFR mutation targeted therapy as an example, since EGFR tyrosine kinase inhibitors (TKIs) were discovered to be effective for NSCLC with EGFR mutations, three generations of EGFR TKIs have been put into clinical application, which provide multiple choices for clinical treatment of EGFR-mutated NSCLC patients (49,50). Notably, the third generation EGFR TKI Osimertinib exhibited striking therapeutic effect in untreated advanced EGFR-mutated NSCLC patients by improving the median progression-free survival to 18.9 months (51). Taken together, our sequencing panel can effectively uncover druggable driver mutations carried by Chinese NSCLC patients, providing guidance for clinical treatment. Moreover, this panel is not fixed, we can include more newly identified targetable driver genes to it according to the latest basic and clinical research progress.
Conclusions
In summary, we reported the development of a driver gene-based sequencing panel and its application in a Chinese NSCLC cohort. The analysis results verified the robustness of this panel to identify driver mutations and guide targeted therapies for Chinese NSCLC patients.
Acknowledgments
The authors also would like to thank the patients who contributed samples to the study as well as all the clinicians and staff for their efforts in collecting tissues and clinical information.
Funding: This work was supported by Capital Clinical Characteristic Applied Research Funds (Project No. Z131107002213105) and the CAPTRA-Lung Research Funds (to Jing Zhao).
Footnote
Reporting Checklist: The authors have completed the STROBE reporting checklist. Available at https://jtd.amegroups.com/article/view/10.21037/jtd-22-909/rc
Data Sharing Statement: Available at https://jtd.amegroups.com/article/view/10.21037/jtd-22-909/dss
Peer Review File: Available at https://jtd.amegroups.com/article/view/10.21037/jtd-22-909/prf
Conflicts of Interest: All authors have completed the ICMJE uniform disclosure form (available at https://jtd.amegroups.com/article/view/10.21037/jtd-22-909/coif). The authors have no conflicts of interest to declare.
Ethical Statement: The authors are accountable for all aspects of the work in ensuring that questions related to the accuracy or integrity of any part of the work are appropriately investigated and resolved. The study was conducted in accordance with the Declaration of Helsinki (as revised in 2013). The study was approved by the ethics committee of the Peking Union Medical College Hospital (No. JS-1410) and informed consent was taken from all the patients.
Open Access Statement: This is an Open Access article distributed in accordance with the Creative Commons Attribution-NonCommercial-NoDerivs 4.0 International License (CC BY-NC-ND 4.0), which permits the non-commercial replication and distribution of the article with the strict proviso that no changes or edits are made and the original work is properly cited (including links to both the formal publication through the relevant DOI and the license). See: https://creativecommons.org/licenses/by-nc-nd/4.0/.
References
- Travis WD, Brambilla E, Nicholson AG, et al. The 2015 World Health Organization Classification of Lung Tumors: Impact of Genetic, Clinical and Radiologic Advances Since the 2004 Classification. J Thorac Oncol 2015;10:1243-60. [Crossref] [PubMed]
- Zheng M. Classification and Pathology of Lung Cancer. Surg Oncol Clin N Am 2016;25:447-68. [Crossref] [PubMed]
- Mao Y, Yang D, He J, et al. Epidemiology of Lung Cancer. Surg Oncol Clin N Am 2016;25:439-45. [Crossref] [PubMed]
- Zhu QG, Zhang SM, Ding XX, et al. Driver genes in non-small cell lung cancer: Characteristics, detection methods, and targeted therapies. Oncotarget 2017;8:57680-92. [Crossref] [PubMed]
- Majeed U, Manochakian R, Zhao Y, et al. Targeted therapy in advanced non-small cell lung cancer: current advances and future trends. J Hematol Oncol 2021;14:108. [Crossref] [PubMed]
- Lazarus DR, Ost DE. How and when to use genetic markers for nonsmall cell lung cancer. Curr Opin Pulm Med 2013;19:331-9. [Crossref] [PubMed]
- Kamps R, Brandão RD, Bosch BJ, et al. Next-Generation Sequencing in Oncology: Genetic Diagnosis, Risk Prediction and Cancer Classification. Int J Mol Sci 2017;18:308. [Crossref] [PubMed]
- Meldrum C, Doyle MA, Tothill RW. Next-generation sequencing for cancer diagnostics: a practical perspective. Clin Biochem Rev 2011;32:177-95.
- Bao R, Huang L, Andrade J, et al. Review of current methods, applications, and data management for the bioinformatics analysis of whole exome sequencing. Cancer Inform 2014;13:67-82. [Crossref] [PubMed]
- Ekblom R, Wolf JB. A field guide to whole-genome sequencing, assembly and annotation. Evol Appl 2014;7:1026-42. [Crossref] [PubMed]
- Chrystoja CC, Diamandis EP. Whole genome sequencing as a diagnostic test: challenges and opportunities. Clin Chem 2014;60:724-33. [Crossref] [PubMed]
- Nagahashi M, Shimada Y, Ichikawa H, et al. Next generation sequencing-based gene panel tests for the management of solid tumors. Cancer Sci 2019;110:6-15. [Crossref] [PubMed]
- Marino P, Touzani R, Perrier L, et al. Cost of cancer diagnosis using next-generation sequencing targeted gene panels in routine practice: a nationwide French study. Eur J Hum Genet 2018;26:314-23. [Crossref] [PubMed]
- Gordon LG, White NM, Elliott TM, et al. Estimating the costs of genomic sequencing in cancer control. BMC Health Serv Res 2020;20:492. [Crossref] [PubMed]
- Bewicke-Copley F, Arjun Kumar E, Palladino G, et al. Applications and analysis of targeted genomic sequencing in cancer studies. Comput Struct Biotechnol J 2019;17:1348-59. [Crossref] [PubMed]
- DiBardino DM, Rawson DW, Saqi A, et al. Next-generation sequencing of non-small cell lung cancer using a customized, targeted sequencing panel: Emphasis on small biopsy and cytology. Cytojournal 2017;14:7. [Crossref] [PubMed]
- Zhao X, Wang A, Walter V, et al. Combined Targeted DNA Sequencing in Non-Small Cell Lung Cancer (NSCLC) Using UNCseq and NGScopy, and RNA Sequencing Using UNCqeR for the Detection of Genetic Aberrations in NSCLC. PLoS One 2015;10:e0129280. [Crossref] [PubMed]
- Meng H, Guo X, Sun D, et al. Genomic Profiling of Driver Gene Mutations in Chinese Patients With Non-Small Cell Lung Cancer. Front Genet 2019;10:1008. [Crossref] [PubMed]
- Wang C, Yin R, Dai J, et al. Whole-genome sequencing reveals genomic signatures associated with the inflammatory microenvironments in Chinese NSCLC patients. Nat Commun 2018;9:2054. [Crossref] [PubMed]
- Tamborero D, Rubio-Perez C, Deu-Pons J, et al. Cancer Genome Interpreter annotates the biological and clinical relevance of tumor alterations. Genome Med 2018;10:25. [Crossref] [PubMed]
- Gaffney SG, Townsend JP. PathScore: a web tool for identifying altered pathways in cancer data. Bioinformatics 2016;32:3688-90. [Crossref] [PubMed]
- Tate JG, Bamford S, Jubb HC, et al. COSMIC: the Catalogue Of Somatic Mutations In Cancer. Nucleic Acids Res 2019;47:D941-7. [Crossref] [PubMed]
- Chakravarty D, Gao J, Phillips SM, et al. OncoKB: A Precision Oncology Knowledge Base. JCO Precis Oncol 2017;
- Kaderbhai CG, Boidot R, Beltjens F, et al. Use of dedicated gene panel sequencing using next generation sequencing to improve the personalized care of lung cancer. Oncotarget 2016;7:24860-70. [Crossref] [PubMed]
- D'Haene N, Le Mercier M, De Nève N, et al. Clinical Validation of Targeted Next Generation Sequencing for Colon and Lung Cancers. PLoS One 2015;10:e0138245. [Crossref] [PubMed]
- Jiang R, Zhang B, Teng X, et al. Validating a targeted next-generation sequencing assay and profiling somatic variants in Chinese non-small cell lung cancer patients. Sci Rep 2020;10:2070. [Crossref] [PubMed]
- Tsoulos N, Papadopoulou E, Metaxa-Mariatou V, et al. Tumor molecular profiling of NSCLC patients using next generation sequencing. Oncol Rep 2017;38:3419-29. [Crossref] [PubMed]
- Bailey MH, Tokheim C, Porta-Pardo E, et al. Comprehensive Characterization of Cancer Driver Genes and Mutations. Cell 2018;173:371-385.e18. [Crossref] [PubMed]
- Tokheim CJ, Papadopoulos N, Kinzler KW, et al. Evaluating the evaluation of cancer driver genes. Proc Natl Acad Sci U S A 2016;113:14330-5. [Crossref] [PubMed]
- Wang BY, Huang JY, Chen HC, et al. The comparison between adenocarcinoma and squamous cell carcinoma in lung cancer patients. J Cancer Res Clin Oncol 2020;146:43-52. [Crossref] [PubMed]
- Pikor LA, Ramnarine VR, Lam S, et al. Genetic alterations defining NSCLC subtypes and their therapeutic implications. Lung Cancer 2013;82:179-89. [Crossref] [PubMed]
- Campbell JD, Alexandrov A, Kim J, et al. Distinct patterns of somatic genome alterations in lung adenocarcinomas and squamous cell carcinomas. Nat Genet 2016;48:607-16. [Crossref] [PubMed]
- Shapiro GI, Edwards CD, Kobzik L, et al. Reciprocal Rb inactivation and p16INK4 expression in primary lung cancers and cell lines. Cancer Res 1995;55:505-9.
- Gautschi O, Ratschiller D, Gugger M, et al. Cyclin D1 in non-small cell lung cancer: a key driver of malignant transformation. Lung Cancer 2007;55:1-14. [Crossref] [PubMed]
- Pateras IS, Apostolopoulou K, Koutsami M, et al. Downregulation of the KIP family members p27(KIP1) and p57(KIP2) by SKP2 and the role of methylation in p57(KIP2) inactivation in nonsmall cell lung cancer. Int J Cancer 2006;119:2546-56. [Crossref] [PubMed]
- Eymin B, Gazzeri S. Role of cell cycle regulators in lung carcinogenesis. Cell Adh Migr 2010;4:114-23. [Crossref] [PubMed]
- Liu TC, Jin X, Wang Y, et al. Role of epidermal growth factor receptor in lung cancer and targeted therapies. Am J Cancer Res 2017;7:187-202.
- Bethune G, Bethune D, Ridgway N, et al. Epidermal growth factor receptor (EGFR) in lung cancer: an overview and update. J Thorac Dis 2010;2:48-51.
- Greenberg AK, Basu S, Hu J, et al. Selective p38 activation in human non-small cell lung cancer. Am J Respir Cell Mol Biol 2002;26:558-64. [Crossref] [PubMed]
- Chung LY, Tang SJ, Sun GH, et al. Galectin-1 promotes lung cancer progression and chemoresistance by upregulating p38 MAPK, ERK, and cyclooxygenase-2. Clin Cancer Res 2012;18:4037-47. [Crossref] [PubMed]
- de Sousa VML, Carvalho L. Heterogeneity in Lung Cancer. Pathobiology 2018;85:96-107. [Crossref] [PubMed]
- Meacham CE, Morrison SJ. Tumour heterogeneity and cancer cell plasticity. Nature 2013;501:328-37. [Crossref] [PubMed]
- Fisher R, Pusztai L, Swanton C. Cancer heterogeneity: implications for targeted therapeutics. Br J Cancer 2013;108:479-85. [Crossref] [PubMed]
- Dagogo-Jack I, Shaw AT. Tumour heterogeneity and resistance to cancer therapies. Nat Rev Clin Oncol 2018;15:81-94. [Crossref] [PubMed]
- Cusnir M, Cavalcante L. Inter-tumor heterogeneity. Hum Vaccin Immunother 2012;8:1143-5. [Crossref] [PubMed]
- Nahar R, Zhai W, Zhang T, et al. Elucidating the genomic architecture of Asian EGFR-mutant lung adenocarcinoma through multi-region exome sequencing. Nat Commun 2018;9:216. [Crossref] [PubMed]
- Kumarakulasinghe NB, van Zanwijk N, Soo RA. Molecular targeted therapy in the treatment of advanced stage non-small cell lung cancer (NSCLC). Respirology 2015;20:370-8. [Crossref] [PubMed]
- Minuti G, D'Incecco A, Cappuzzo F. Targeted therapy for NSCLC with driver mutations. Expert Opin Biol Ther 2013;13:1401-12. [Crossref] [PubMed]
- Linardou H, Dahabreh IJ, Bafaloukos D, et al. Somatic EGFR mutations and efficacy of tyrosine kinase inhibitors in NSCLC. Nat Rev Clin Oncol 2009;6:352-66. [Crossref] [PubMed]
- Russo A, Franchina T, Ricciardi GR, et al. A decade of EGFR inhibition in EGFR-mutated non small cell lung cancer (NSCLC): Old successes and future perspectives. Oncotarget 2015;6:26814-25. [Crossref] [PubMed]
- Soria JC, Ohe Y, Vansteenkiste J, et al. Osimertinib in Untreated EGFR-Mutated Advanced Non-Small-Cell Lung Cancer. N Engl J Med 2018;378:113-25. [Crossref] [PubMed]