Molecular typing and prognostic model of lung adenocarcinoma based on cuprotosis-related lncRNAs
Highlight box
Key findings
• Our research identified new molecular subtypes and constructed a novel prognostic model of LUAD based on CR-lncRNAs.
What is known and what is new?
• Cuprotosis plays an important role in the proliferation and metastasis of cancer cells. CR-lncRNAs can predict the prognosis and immune microenvironmental characteristics of many cancers.
• CR-lncRNAs coud divided LUADs into two novel molecular subtypes and predicted the prognosis and choice of treatment. Cluster A and low-risk group had longer OS, more immune cells infiltration and higher TME scores. TMB and TIDE analyses showed better immunotherapy response in high-risk group.
What is the implication, and what should change now?
• Our study provided new ideas for molecular classification and prediction of survival of LUAD.
Introduction
Global morbidity and mortality from lung cancer (LC) continue to grow (1), and lung adenocarcinoma (LUAD) is its most common pathological subtype (2,3). Despite the rapid advancement of molecular biology and precise medicine, the 5-year overall survival (OS) of LUAD remains low, at only 15% (4). A previous study has clarified LUAD as a highly though heterogeneous and complex cancer caused by multiple genes, multiple factors, and steps, and that traditional histological classification has significant limitations in guiding its clinical treatment and predicting survival (5). In recent years, the identification of LUAD molecular subtypes based on polymerase chain reaction (PCR), next-generation sequencing (NGS), fluorescence in situ hybridization (FISH) and other molecular biological technologies has shifted the classification of LUAD from morphology to molecular characteristics, which provides the basis for individual therapy. For example, epidermal growth factor receptor (EGFR), v-raf murine viral oncogene homolog B1 (BRAF), and mesenchymal-epithelial transition factor (MET) mutational, as well as anaplastic lymphoma kinase (ALK) and ROS proto-oncogene 1, receptor tyrosine kinase (ROS1) translocated LUADs, have corresponding targeted inhibitors for clinical treatment (6). In addition, more and more researches were devoted to further developing novel molecular subtypes of LUAD. Zhang et al. divided more than 1,000 LUADs into three subtypes based on 14 ferroptosis genes, and clarified the differences in prognosis, immune microenvironment, and enrichment pathways among them (7). Liu et al. separated LUADs into three molecular subtypes based on pyroptosis genes, and illustrated the differences of clinicopathological features, prognosis, and immune cells infiltration of the subtypes (8). However, as the clinical responses of these classifications is not ideal, it is of great significance to further complement LUAD molecular types from an additional perspective.
Copper is an essential micronutrient for all organisms and plays important roles in cellular respiration, free radical detoxification, enzymatic activity catalysis, and neuropeptide processing (9-11). In living organisms, active intracellular copper homeostasis maintains low copper concentrations because its excess is detrimental (12-15). For instance, in mouse hepatocytes, copper induces oxidative stress and promotes apoptosis by activating the tumor necrosis factor receptor-1 (TNF-R1) signaling pathway (16). Copper can also disrupt calcium homeostasis and activate apoptosis and autophagy related pathways to promote tumor cell death (17,18) and induce apoptosis-like death of cancer cells by inhibiting 19S proteasome associated deubiquitinase and/or 20S proteasome activity (19). In view of this, copper has attracted great attention and become a hot spot in the field of tumor therapy (20). The copper chelator elesclomol has been revealed to possess significant anticancer activity in LC, melanoma, colorectal cancer, and sarcoma (21). The copper ion complexes CuL1Cl2 and CuL2Cl2 showed high cytotoxic activity against many tumor cells and low cytotoxic activity against normal cells and were regarded as ideal choices for oncology treatment (17). However, recent studies found copper-induced cell death was distinct from other programmed cell death forms such as necroptosis, apoptosis, and ferroptosis. Copper-induced cell death refers to the programmed cell death induced by copper ions through the accumulation of lipoacylated proteins and the loss of iron-sulfur cluster proteins under condition of mitochondrial stress. This new type of programmed cell death is defined as “cuprotosis”. It is distinct from copper toxicosis, in that cuprotosis can occur even when the concentration of copper ions in the cell is appropriate (22). Further tumor studies showed cuprotosis genes were differentially expressed between cancer and normal tissues. Cuprotosis genes play important roles in the proliferation and metastasis of cancer cells by influencing many cancer signaling pathways, including receptor tyrosine kinase (RTK), epithelial-mesenchymal transition (EMT), and cell cycle (23). Given the relationship between cuprotosis and LUAD remains unclear, it is valuable to investigate cuprotosis from the perspective of tumor typing and survival prediction.
Long non-coding RNAs (lncRNAs) refer to RNA transcripts over 200 nucleotides that do not encode proteins but play important roles in the regulation of gene expression (24). LncRNAs are thought to play critical roles in pathological or physiological processes such as cell cycle progression, cell differentiation, metabolic diseases, and viral infections (24), and in many tumors are also considered as diagnostic and prognostic markers and therapeutic targets (25). Numerous research has focused on molecular typing and predicting survival using lncRNAs. For example, in hepatocellular carcinoma, tumor typing based on ferroptosis-related lncRNAs (FR-lncRNAs) provided better predictions of prognosis and efficacy of immunotherapy and targeted therapy (26). In head and neck squamous cell carcinoma (HNSC), the signatures of FR-lncRNAs could also predict patient outcomes (27), while in acute myeloid leukemia, m6A-related lncRNAs could predict the tumor microenvironment (TME) and survival (28). In addition, lncRNAs also participate in tumor progression by regulating cuprotosis genes. LncRNA LFAR1 regulates the crocin-inhibited mouse hepatic stellate cell activation by the MTF-1/GDNF pathway (29), and lncRNA MALAT1 protects human osteoblasts from dexamethasone-induced injury by activating the PPM1E-NFE2L2 signaling pathway (30). Further, cuprotosis-related lncRNAs (CR-lncRNAs) have been found to predict the prognosis and immune microenvironmental characteristics of soft tissue sarcoma (31). And in HNSC, a prognostic model based on 8 CR-lncRNAs can be regarded as a prognostic factor and marker of immunotherapy and drug sensitivity (32). However, the roles of CR-lncRNAs in LUAD and their relationship with the prognosis and immune cells infiltration and efficacy of immunotherapy of LUAD patients remain unclear.
In this study, we investigated the expression and prognostic significance of 19 cuprotosis genes in LUAD in The Cancer Genome Atlas (TCGA) database and authenticated 501 CR-lncRNAs which were closely related to them. Univariate Cox analysis identified 34 prognosis-related CR-lncRNAs which were then used to molecularly type LUAD and analyze the differences in prognosis, clinical characteristics, pathway enrichment, immune cells infiltration, and TME between the subtypes. In addition, the 34 CR-lncRNAs were subjected to least absolute shrinkage and selection operator (LASSO) regression to reduce the fitting, and finally 10 lncRNAs were used to establish a Cox regression prognostic model. We then analyzed the differences in clinical characteristics, pathway enrichment, immune cells infiltration, TME, mutation, and drug sensitivity between low and high-risk groups. Our research elucidated the potential role of CR-lncRNAs in molecular typing and prognosis of LUAD and provided new ideas for its diagnosis and survival prediction. We present the following article in accordance with the TRIPOD reporting checklist (available at https://jtd.amegroups.com/article/view/10.21037/jtd-22-1534/rc).
Methods
Download and process LUAD data from TCGA
We downloaded RNA expression profiling sequencing and clinical information data of LUAD from the TCGA (https://portal.gdc.cancer.gov/), which included 59 normal lung samples and 535 LUAD samples (33). Normal lung samples and LUAD samples without complete clinical information were removed, and the remaining samples were used for molecular typing and construction and validation of the prognostic model. R (4.1.3) and Perl (Strawberry version) software were used to process and analyze the data. The study was conducted in accordance with the Declaration of Helsinki (as revised in 2013).
Identification of CR-LncRNAs in LUAD
Initially, we obtained 19 cuprotosis genes (NLRP3, GLS, DBT, ATP7A, MTF1, NFE2L2, SLC31A1, FDX1, DLD, DLAT, LIAS, LIPT1, PDHB, GCSH, PDHA1, CDKN2A, DLST, ATP7B, and LIPT2) from previous literature for our study (21,22,34,35). Then, we analyzed the expression differences of 19 cuprotosis genes between normal lung and LUAD samples. The correlation between cuprotosis genes and OS of LUAD patients was then performed using a Kaplan-Meier (K-M) curve. Subsequently, we used the “biotype.pl” program to differentiate the expression matrices of mRNAs and lncRNAs, and a Pearson correlation coefficient was used to evaluate the expression correlation between 19 cuprotosis genes and lncRNAs. Under the condition |R| >0.4 and P<0.001, we identified 501 CR-lncRNAs (available at: https://cdn.amegroups.cn/static/public/jtd-22-1534-1.xls). Univariate Cox was then used to further identified 34 prognosis-related CR-lncRNAs with the condition P<0.01.
Unsupervised clustering of LUAD based on CR-lncRNAs
We applied an unsupervised clustering algorithm of the “Consensus Cluster Plus” R package to cluster 474 LUAD samples according to the expression levels of 34 prognosis-related CR-lncRNAs. To ensure the stability of the clustering, 100 iterations were carried out with a resampling rate of 80%, and the clustering number was ascertained by the cumulative distribution function (CDF) curve (36).
Construction and validation of the prognostic model
Firstly, 474 LUAD samples were randomly and equally divided into a training set and testing set, and Lasso Cox regression was performed on 34 prognosis-related CR-lncRNAs in the training set (37). And we established a prognostic model of LUAD based on 10 CR-lncRNAs. The risk score for each sample was calculated based on the expression level of each lncRNA and its corresponding regression coefficient. The risk score formula = esum (each lncRNA expression × corresponding regression coefficient). LUAD patients were divided into low-risk and high-risk groups based on the median risk score, and a K-M curve was used to analyze the difference of OS in the two risk groups. A receiver operating characteristic (ROC) curve was used to calculate the reliability of the model. The risk score of the samples in testing and entire sets was then calculated according to the modeling formula. Similarly, patients were divided into low and high-risk groups based on the median risk score of the training set. K-M and ROC analyses in test and entire sets were then performed to demonstrate the stability of the model, and nomograms and calibration plots in the entire set were used to test the model's accuracy in predicting survival.
Clinical characteristics and prognostic analysis of clusters and risk groups
Associations of clustering and risk score with clinical characteristics, age, sex, stage, tumor size (T), lymph node metastasis (N), and survival status were analyzed using the chi-square test, and the results were depicted using heatmaps by means of the “pheatmap” package. Further, the relationship between risk scores and clinical characteristics was also analyzed by Kruskal or Wilcoxon tests. The K-M curve analysed the relationship between the OS of LUAD patients and cuprotosis gene expression, clustering, and risk score, and univariate and multivariate Cox assessed the value of the risk score as a prognostic indicator for LUAD.
Functional enrichment analysis of clusters and risk groups
To clarify differences in biological function between different clusters and risk groups, we performed the gene set variation analysis (GSVA) (38) using the “c2.cp.kegg.v6.2.-symbols gmt” set from the MSigDB database (http://software.broadinstitute.org/gsea/msigdb/index.jsp), and P<0.05 was considered to be differentially enriched. In addition, the “limma” R package was used to select the differential genes between the different risk groups of the entire sample using |logFC| >1 and P<0.05 as the selected condition. Differential genes were then subjected to Gene Ontology (GO) and Kyoto Encyclopedia of Genes and Genomes (KEGG) enrichment analysis.
TME landscape and immune checkpoint evaluation
We used the CIBERSORT algorithm to identify differences in the abundance of infiltration of 28 immune cells in different clusters and risk groups (39). Further, the Single-Sample Gene Set Enrichment Analysis (ssGSEA) R package was used to analyze immune cell infiltration and immune function across different clusters and risk groups (40). The StromalScore, ImmunScore, and EstimatedScore were calculated separately for each LUAD patient using the ESTIMATION algorithm, and the “ggpubr” package visualized the differences (41).
Mutation, immunotherapy, and drug sensitivity analysis
The mutation data of LUAD was downloaded from TCGA. We analysed somatic mutations in different risk groups by the package “maftools” and assessed the tumor mutational burden (TMB) score in the low and high-risk groups. Tumor Immune Dysfunction and Exclusion (TIDE) analysis was performed to estimate the response of immune checkpoint blockade (ICB) therapy (42). In addition, the “pRRophetic” package was used to analyze the relationship between the semi-inhibitory concentration (IC50) and risk score of some chemotherapeutic drugs.
Statistical analysis
Kruskal and Wilcoxon tests were used for multigroups and two groups, respectively. Pearson correlation coefficient was used for analysing the correlation, and the survival curve was drawn by K-M analysis. Univariate and multivariate Cox were used to calculate 95% confidence intervals (CI) and hazard ratios (HR). R (4.1.3) software was used for statistical analysis and P<0.05 was considered statistically significant.
Results
Expression and prognosis analyses of cuprotosis genes in LUAD
We investigated the expression of 19 cuprotosis genes in normal lung and LUAD tissues, and compared with normal lung tissues, NLRP3 (P<0.001), MTF1 (P<0.001), NFE2L2 (P<0.001), SLC31A1 (P=0.008), and FDX1 (P<0.001) were less expressed in tumor tissues. In addition, DLD (P=0.019), DLAT (P<0.001), LIAS (P<0.001), PDHB (P=0.008), GCSH (P<0.001), PDHA1 (P<0.001), CDKN2A (P<0.001), LIPT1 (P=0.003), ATP7B (P=0.029), and LIPT2 (P=0.032) were more expressed in tumor tissues, while there was no differences in GLS (P=0.054), DBT (P=0.202), ATP7A (P=0.657), and DLST (P=0.466) expression between normal lung and LUAD tissues (Figure 1A). We also analyzed the correlation among the expression of 19 cuprotosis genes (Figure 1B), and the association between cuprotosis genes expression and the OS of LUAD patients. K-M curves indicated the expression of NLRP3 (P=0.004), GLS (P=0.007), DBT (P=0.009), MTF1 (P=0.009), and SLC31A1 (P=0.005) were positively correlated with OS, while the expression of FDX1 (P=0.027), DLD (P=0.041), DLAT (P=0.021), LIPT1 (P=0.002), PDHA1 (P=0.005), CDKN2A (P<0.001), and DLST (P=0.038) were negatively related to OS (Figure 1C-1N). No correlation was seen between the expression of ATP7A (P=0.247), NFE2L2 (P=0.151), LIAS (P=0.184), PDHB (P=0.203), GCSH (P=0.059), ATP7B (P=0.175), LIPT2 (P=0.071), and OS (Figure S1A-S1G).
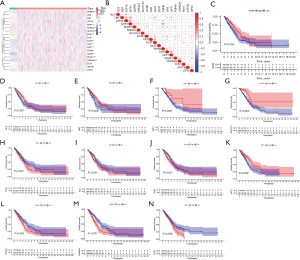
Identification of CR-LncRNAs in LUAD
The expression matrices of lncRNAs were extracted from TCGA LUAD data, and according to the expression level of the previous 19 cuprotosis genes, we screened out 501 CR-lncRNAs of LUAD using the Pearson Correlation Coefficient test (Figure 2A) (available at: https://cdn.amegroups.cn/static/public/jtd-22-1534-1.xls). Subsequently, 34 prognosis-related CR-lncRNAs were identified by univariate Cox analysis (Figure 2B). Figure 2C shows the differential expression of these lncRNAs in normal lung tissues and LUAD tissues, while correlation of their expression is displayed in Figure 2D.
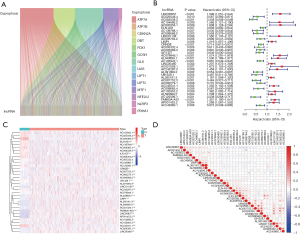
Consensus clustering of LUAD based on CR-lncRNAs
To understand the role of CR-lncRNAs in LUAD, we performed unsupervised consensus clustering analysis on 474 LUAD patients. After repeated 100 iterations it was found that the stability of consensus cluster was the highest when divided into a cluster A and cluster B (Figure 3A-3C). Both principal component (PCA) and t-distributed stochastic neighbor embedding (t-SNE) analyses showed identifiable dimensions between cluster A and B (Figure 3D-3E), while the K-M curve showed the OS of cluster A was better than that of cluster B (Figure 3F). Differential analysis of clinical characteristics illustrated gender, stage, T, N, and survival status were significantly different in clusters, while there was no difference of age between cluster A and cluster B (Figure 3G). GSVA functional enrichment showed that compared with cluster A, cluster B was more significantly enriched in the pentose phosphate pathway, glycolysis gluconeogenesis, fructose and mannose metabolism, amino sugar and nucleotide sugar metabolism, phenylalanine metabolism, citrate cycle TCA cycle, and other sugars and amino acids metabolism-related pathways (Figure 3H).
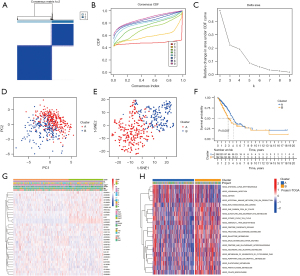
Immune cell infiltration and TME evaluation of clusters
Functional enrichment analysis showed cluster B was significantly enriched in the energy and amino acid metabolism related pathways. As previous research has clarified energy and amino acid metabolisms affect immune cell infiltration by changing the TME (43), we analyzed the differences of immune cells infiltration and TME between clusters A and B. The CIBERSORT analysis showed activated B cells, eosinophils, immature B cells, immature dendritic cells, mast cells, myeloid-derived suppressor cells (MDSCs), memory B cells, natural killer cells, plasmacytoid dendritic cells, and T follicular helper cells infiltrated more in cluster A and CD56 dim natural killer cells and neutrophils infiltrated more in cluster B (Figure 4A). The ssGSEA analysis showed B cells, iDC cells, mast cells, T helper cells, and TILs infiltrated more in cluster A (Figure 4B), while the immune function analysis of ssGSEA revealed cluster A had higher HLA and type II IFN response activities (Figure 4C). In addition, the TME score was calculated using the ESTIMATE algorithm, and the StromalScore, ImmuneScore, and ESTIMATEScore of cluster A were all higher than cluster B (Figure 4D).
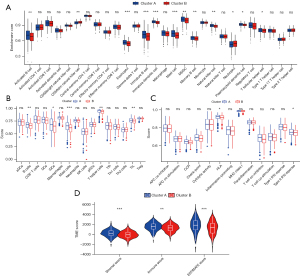
Construction of CR-lncRNAs prognostic model in LUAD
To better elucidate the association of CR-lncRNAs with survival in LUAD patients, we constructed a CR-lncRNAs prognostic model. First, 474 LUADs were divided into training and testing sets randomly and equally. After further screening by Lasso regression in the training set of 34 prognosis-related CR-lncRNAs, the Cox regression model was constructed with 10 CR-lncRNAs of AC026356.1, AC099568.2, LINC01138, CRNDE, AC026369.3, AL049836.1, AC021028.1, U91328.1, AC123595.1, and AC243960.1 (Figure 5A,5B). K-M survival analysis showed the OS of the high-risk group was significantly worse than the low-risk group (P=4.885e-6) (Figure 5C), while Figure 5D shows the high-risk group had higher risk score, and the survival rate of high-risk patients was poor. The ROC curve clarified the values of area under the curve (AUC) of the model in 1, 3, and 5 years were 0.825, 0.824 and 0.854, respectively (Figure 5E). We also analyzed the expression differences of the 10 CR-lncRNAs between risk groups. Figure 5F shows AC026356.1, LINC01138, AL049836.1, and AC021028.1 were highly expressed in the high-risk group, and AC099568.2, CRNDE, AC026369.3, U91328.1, AC123595.1, and AC243960.1 were highly expressed in low-risk group.
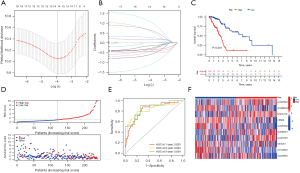
Validation of the CR-lncRNAs prognostic model of LUAD
To test the reliability and stability of the model, we performed validation in the testing and entire set. In the testing set, the K-M analysis indicated worse prognosis in the high-risk group (P<0.001) (Figure 6A), and the high-risk group had higher risk score and lower survival rate (Figure 6B). The AUC of the ROC curve for 1, 3, and 5 years were 0.711, 0.652 and 0.654, respectively (Figure 6C). Likewise, in the entire set, the risk score in the high-risk group was higher and the survival rate in the high-risk group was worse (Figure 6D,6E). The 1-, 3-, and 5-year AUC for the entire group were 0.765, 0.737 and 0.768, respectively (Figure 6F). In addition, in the entire set, the ROC curve demonstrated the risk score (AUC: 0.765) had the highest accuracy in predicting patient survival compared to age (AUC: 0.550), gender (AUC: 0.592), stage (AUC: 0.712), T (AUC: 0.649), and N (AUC: 0.626) (Figure 6G). Univariate Cox analysis showed stage (HR: 1.658, P<0.001), T (HR: 1.523, P<0.001), N (HR: 2.720, P<0.001), and risk score (HR: 1.114, P<0.001) were prognostic factors in patients with LUAD (Figure 6H). Multivariate Cox analysis showed that stage (HR: 1.362, P<0.007), N (HR: 1.731, P=0.01), and risk score (HR: 1.128, P<0.001) could be considered as independent prognostic indicators (Figure 6I). To more accurately predict patient survival, we created a nomogram including risk score and other clinical characteristics (Figure 6J) and visualized the performance of the nomogram with calibration plots at 1, 2, and 3 years (Figure 6K). The alluvial diagram shows the distribution for each individual patient in clusters and risk groups (Figure 6L).
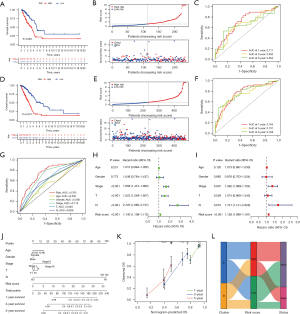
Clinical characteristics and functional enrichment analyses
PCA and t-SNE analyses showed that the high-risk group and low-risk group in the entire set were well differentiated (Figure 7A,7B). The Chi-square test displayed the risk score was related to gender (P<0.05), stage (P<0.001), T (P<0.001), N (P<0.001), and survival status(P<0.001), and not related to age (P>0.05) (Figure 7C). The Kruskal test indicated the level of risk score was positively correlated with stage (P=0.0013) and T (P=0.025) (Figure 7D,7E), and among N0, N1, and N2, the risk score was positively connected with N (P<0.001). The low number of N3 patients might explain the lack of statistical significance in the four-group analysis (P=0.98) (Figure 7F). The Wilcox test showed patients with a death status had a higher risk score (P<0.001) (Figure 7G). Subsequently, to understand the differences in functional enrichment of risk groups, we identified differential genes between them and performed enrichment analysis, and according to LogFC >1 and false discovery rate (FDR) <0.05, 320 differential genes were screened (Figure 7H). KEGG analysis showed the differential genes were enriched in focal adhesion, ECM-receptor interaction, protein digestion and absorption, the PI3K-Akt signaling pathway, and the nitrogen metabolism pathway (Figure 7I). GO analysis showed they were observably enriched in ubiquitin protein ligase binding, protein kinase complex, response to lipopolysaccharide, response to ketone, response to reactive oxygen species, cellular response to oxidative stress, response to decreased oxygen levels, response to steroid hormone c, and other pathways (Figure 7J). In addition, differential GSVA enrichment showed the high-risk group was more enriched in the pentose phosphate pathway, ubiquitin mediated proteolysis, cell cycle, base excision repair, spliceosome, nucleotide excision repair, mismatch repair, and other pathways, while the low-risk group was more enriched in asthma, fatty acid metabolism, alpha linolenic acid metabolism, and arachidonic acid metabolism pathways (Figure 7K).
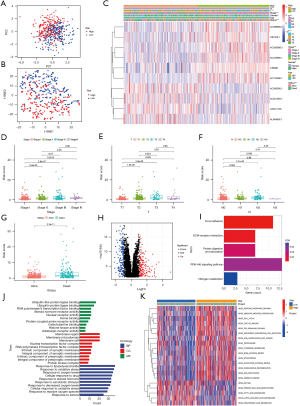
Analyses of immune cell infiltration and TME in risk groups
CIBERSORT analysis showed that compared with the high-risk group, activated B cells, activated CD8 T cells, activated dendritic cells, central memory CD4 T cells, central memory CD4 T cells, eosinophils, immature B cells, immature dendritic cells, immature dendritic cells, mast cells, MDSCs, monocytes, natural killer cells, and T follicular helper cells were infiltrated more in the low-risk group, and activated CD4 T cells and type 2 T helper cells were infiltrated more in the high-risk group (Figure 8A). The ssGSEA showed aDCs, B cells, DCs, iDCs, mast cells, neutrophils, pDCs, T helper cells, Tfh, and TIL cells infiltrated more in the low-risk group (Figure 8B), and immune function analysis showed the function of HLA, T cell co-inhibition, T cell co-stimulation, and type II IFN response were suppressed in the high-risk group compared with the low-risk group. The high-risk group had stronger MHC class I function (Figure 8C), while ImmuneScore (P<0.001) and ESTIMATEScore (P<0.01) were lower in the high-risk group (Figure 8D).
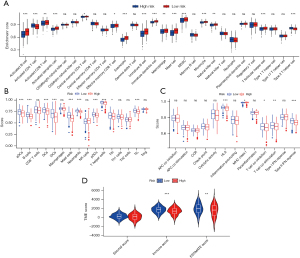
Mutation, immunotherapy and chemotherapy sensitivity analyses
Somatic mutation frequency is relevant to tumor immune cell infiltration. Therefore, we analyzed the changes of frequency of somatic mutations in the two risk groups using the “maftools” package. We found the overall somatic mutation rate in the high-risk group (93.33%) was higher compared to the low-risk group (87.32%). Among the top 10 genes with the highest mutation rates, TP53, TTN, CSMD3, RYR2, LRP1B, ZFHX4, USH2A, KRAS, and XIRP2 had a higher mutation rate in the high-risk group, and only the MUC16 gene had a higher mutation rate in the low-risk group (Figure 9A,9B). In addition, research has shown tumors with high mutational burden are more immunogenic and generate more neoantigens, and TMB is considered as a potential predictive marker of an immune checkpoint blockade (ICB) response (44,45). In our research, we found the TMB score was significantly increased in the high-risk group compared to the low-risk group (P=0.0069) (Figure 9C) and was positively associated with longer OS (Figure 9D). In addition, patients with a higher TMB in both groups had a better prognosis (Figure 9E), while the high-risk group had lower TIDE scores than the low-risk group (P<0.001) (Figure 9F). Furthermore, we assessed the association of risk score with the sensitivity of common drugs for LUAD and found it was inversely associated with the sensitivity to docetaxel (R=−0.18, P=8.4e−05), cisplatin (R=−0.4, P<2.2e−16), paclitaxel (R=−0.3, P=2.3e−11), gemcitabine (R=−0.25, P=4.8e−08), vinorelbine (R=−0.23, P=2.4e−07), axitinib (R=−0.28, P=2.9e−10), and saracatinib (R=−0.22, P=1.8e−06), and positively connected with erlotinib (R=0.23, P=2.4e−07) (Figure 9G-9N).
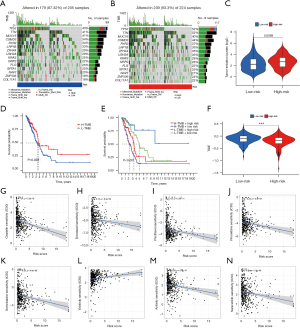
Discussion
LUAD is a dominant cause of cancer death worldwide (1). Due to the high heterogeneity of the disease, given the same treatment regimen, patients may have different clinical outcomes (46,47). For example, programmed cell death protein 1 (PD1), cytotoxic T lymphocyte-associated antigen 4 (CTLA4), and EGFR inhibitors significantly prolong the survival of some patients with advanced LUAD, but offer no benefit in others (48-50). Therefore, it is important to authenticate new LUAD subtypes for providing precise treatment and prognosis prediction for patients.
A previous study has shown copper participates in many biological processes such as tumorigenesis, development, angiogenesis, and epithelial-mesenchymal transition (51). While dysregulation of copper homeostasis can lead to mitochondrial dysfunction and promote cell death through the Fenton reaction or elevated ROS levels, copper can also bind proteasome subunits to promote cell death by inducing protein ubiquitinating (20). Therefore, some copper ionophores including bis (thiosemicarbazide) ligands, dithiocarbamates, and flavonoids have been identified as anticancer agents (52). However, the latest research showed cuprotosis is a novel type of cell death caused by copper-induced aggregation of mitochondrial proteins (53). Given the function of cuprotosis genes and their regulators in LUAD remains unclear, it is significant to explore the role of the cuprotosis genes and CR-lncRNAs in the molecular typing and prognosis of the disease.
In our research, we first investigated the expression and prognosis of 19 cuprotosis genes in LUAD. Subsequently, we divided 474 LUAD cases into cluster A and cluster B with an unsupervised consensus clustering method based on 34 CR-lncRNAs, and K-M analysis showed cluster B had worse OS compared to cluster A. To understand the reasons for the difference in prognosis, we analyzed the TME of the two clusters, and found StromalScore, ImmuneScore, and ESTIMATEScore were all significantly elevated in cluster A. Previous study reported longer OS was generally accompanied by higher stromal and immune scores (54), which was consistent with our results. Immune cells play critical roles in the TME, and immune infiltration is closely related to patient survival (55). Our results showed activated B cells, eosinophils, immature B cells, immature dendritic cells, mast cells, MDSC, memory B cells, natural killer cells, plasmacytoid dendritic cells, and T follicular helper cells infiltrated more in cluster A, and CD56dim natural killer cells and neutrophils infiltrated more in cluster B. Different immune cells play tumor-promoting or tumor-suppressing roles in different ways. For instance, B cells can inhibit tumor progression via secreting immunoglobulins, promoting T cell responses, and directly killing cancer cells (56), and tumor-infiltrating eosinophils synthesize granulin to kill cancer cells or inhibit tumor progression by remodeling the TME (57). Dendritic cells can promote T cell immunity and immunotherapy responses by presenting endogenous or exogenous antigens to T cells to enhance memory T cell responses (58), while MCs inhibit cancer cell proliferation and induce death by releasing various cytokines such as IL-1, IL-6, MCP-4, TNF-α, IFN-γ, and TGF-β (59). T follicular helper cells enhanced the function of CD8+ T and inhibited tumor proliferation in vivo (60), and MDSCs promoted tumor progression by producing ROS, NO, Arg1, and suppressor cytokines for anti-tumor immunity (61). Neutrophils have become an important part of the TME, and TAN can promote tumor recurrence and metastasis by driving angiogenesis, extracellular matrix remodeling, and immunosuppression (62). NK cells exert antitumor effects indirectly through direct cytolytic activity and production of cytokines such as IFN-γ (63). Therefore, we speculate that the better prognosis of cluster A patients may be caused by more immune cell infiltration. Although cluster B had more NK cell infiltration, we speculate this could not reverse its immunosuppressive microenvironment.
To better elucidate the relationship between CR-lncRNAs and the prognosis of LUAD, we constructed a Lasso COX regression model based on 10 CR-lncRNAs. The OS of the high-risk patients was significantly lower compared to the low-risk patients, and the internal multiple validation analysis demonstrated the model had high accuracy. Subsequently, we found immune cell infiltration and immune function were significantly suppressed in the high-risk group, which could partly explain the poorer prognosis of that group. We then analyzed somatic mutations and TMB to further understand the reason for the difference in immune cell infiltration between the high and low-risk groups. Interestingly, the waterfall plot showed the high-risk group had a higher mutation frequency and TMB score, suggesting immunosuppression was more severe in the high-risk group compared with the low-risk group.
Previous study identified TMB as a potentially important biomarker of the immunotherapy response and that it positively correlated with anti-PD-1 and anti-PD-L1 responses (64). Therefore, we conjectured the efficacy of ICB therapy in the high-risk group was better. In addition, research has shown patients with higher TIDE scores were more likely to escape from antitumor immunity and that they had had lower response rates to ICB therapy. Compared with TMB, TIDE is more accurate in predicting survival outcomes of ICB-treated cancer patients (42). In our study, patients in the high-risk group had lower TIDE scores, which further supported the enhanced effect of ICB treatment in the high-risk group. Therefore, we believe the CR-lncRNA model could be available as a credible biomarker for immunotherapy of LUAD patients. In addition, drug sensitivity analysis also provided a theoretical basis for the selection of chemotherapy drugs.
Conclusions
In conclusion, our research identified two molecular subtypes and constructed a prognostic model of LUAD based on CR-lncRNAs. We elucidated the differences in clinical characteristics, prognosis, immune cells infiltration, TME, and functional enrichment between subtypes and risk groups, and investigated the differences of immunotherapy and chemotherapeutic drugs sensitivity between the risk groups. Our study provides new ideas for the molecular classification of LUAD and the prediction of patient survival. However, our research is based on the analysis of bioinformatics data, and in the future, experimental research is required to validate the results.
Acknowledgments
Funding: This study was funded by grants from the Special research Project of Nantong Municipal Health and Family Planning Commission, Jiangsu, China (No. MB2020068).
Footnote
Reporting Checklist: The authors have completed the TRIPOD reporting checklist. Available at https://jtd.amegroups.com/article/view/10.21037/jtd-22-1534/rc
Conflicts of Interest: All authors have completed the ICMJE uniform disclosure form (available at https://jtd.amegroups.com/article/view/10.21037/jtd-22-1534/coif). The authors have no conflicts of interest to declare.
Ethical Statement: The authors are accountable for all aspects of the work in ensuring that questions related to the accuracy or integrity of any part of the work are appropriately investigated and resolved. The study was conducted in accordance with the Declaration of Helsinki (as revised in 2013).
Open Access Statement: This is an Open Access article distributed in accordance with the Creative Commons Attribution-NonCommercial-NoDerivs 4.0 International License (CC BY-NC-ND 4.0), which permits the non-commercial replication and distribution of the article with the strict proviso that no changes or edits are made and the original work is properly cited (including links to both the formal publication through the relevant DOI and the license). See: https://creativecommons.org/licenses/by-nc-nd/4.0/.
References
- Sung H, Ferlay J, Siegel RL, et al. Global Cancer Statistics 2020: GLOBOCAN Estimates of Incidence and Mortality Worldwide for 36 Cancers in 185 Countries. CA Cancer J Clin 2021;71:209-49. [Crossref] [PubMed]
- Goldstraw P, Ball D, Jett JR, et al. Non-small-cell lung cancer. Lancet 2011;378:1727-40. [Crossref] [PubMed]
- Comprehensive molecular profiling of lung adenocarcinoma. Nature 2014;511:543-50. [Crossref] [PubMed]
- Spella M, Stathopoulos GT. Immune Resistance in Lung Adenocarcinoma. Cancers (Basel) 2021.
- Hensing T, Chawla A, Batra R, et al. A personalized treatment for lung cancer: molecular pathways, targeted therapies, and genomic characterization. Adv Exp Med Biol 2014;799:85-117. [Crossref] [PubMed]
- Imyanitov EN, Iyevleva AG, Levchenko EV. Molecular testing and targeted therapy for non-small cell lung cancer: Current status and perspectives. Crit Rev Oncol Hematol 2021;157:103194. [Crossref] [PubMed]
- Zhang W, Yao S, Huang H, et al. Molecular subtypes based on ferroptosis-related genes and tumor microenvironment infiltration characterization in lung adenocarcinoma. Oncoimmunology 2021;10:1959977. [Crossref] [PubMed]
- Liu LP, Lu L, Zhao QQ, et al. Identification and Validation of the Pyroptosis-Related Molecular Subtypes of Lung Adenocarcinoma by Bioinformatics and Machine Learning. Front Cell Dev Biol 2021;9:756340. [Crossref] [PubMed]
- García-Torra V, Cano A, Espina M, et al. State of the Art on Toxicological Mechanisms of Metal and Metal Oxide Nanoparticles and Strategies to Reduce Toxicological Risks. Toxics 2021;9:195. [Crossref] [PubMed]
- Balsano C, Porcu C, Sideri S. Is copper a new target to counteract the progression of chronic diseases? Metallomics 2018;10:1712-22. [Crossref] [PubMed]
- Zheng A, Zhang X, Gao J, et al. Peroxidase-like catalytic activity of copper ions and its application for highly sensitive detection of glypican-3. Anal Chim Acta 2016;941:87-93. [Crossref] [PubMed]
- Kim BE, Nevitt T, Thiele DJ. Mechanisms for copper acquisition, distribution and regulation. Nat Chem Biol 2008;4:176-85. [Crossref] [PubMed]
- Ge EJ, Bush AI, Casini A, et al. Connecting copper and cancer: from transition metal signalling to metalloplasia. Nat Rev Cancer 2022;22:102-13. [Crossref] [PubMed]
- Lutsenko S, Bhattacharjee A, Hubbard AL. Copper handling machinery of the brain. Metallomics 2010;2:596-608. [Crossref] [PubMed]
- Rae TD, Schmidt PJ, Pufahl RA, et al. Undetectable intracellular free copper: the requirement of a copper chaperone for superoxide dismutase. Science 1999;284:805-8. [Crossref] [PubMed]
- Liu H, Guo H, Jian Z, et al. Copper Induces Oxidative Stress and Apoptosis in the Mouse Liver. Oxid Med Cell Longev 2020;2020:1359164. [Crossref] [PubMed]
- Gul NS, Khan TM, Chen M, et al. New copper complexes inducing bimodal death through apoptosis and autophagy in A549 cancer cells. J Inorg Biochem 2020;213:111260. [Crossref] [PubMed]
- Fu Y, Liu Y, Wang J, et al. Calcium release induced by 2-pyridinecarboxaldehyde thiosemicarbazone and its copper complex contributes to tumor cell death. Oncol Rep 2017;37:1662-70. [Crossref] [PubMed]
- Chen X, Zhang X, Chen J, et al. Hinokitiol copper complex inhibits proteasomal deubiquitination and induces paraptosis-like cell death in human cancer cells. Eur J Pharmacol 2017;815:147-55. [Crossref] [PubMed]
- Jiang Y, Huo Z, Qi X, et al. Copper-induced tumor cell death mechanisms and antitumor theragnostic applications of copper complexes. Nanomedicine (Lond) 2022;17:303-24. [Crossref] [PubMed]
- Gao W, Huang Z, Duan J, et al. Elesclomol induces copper-dependent ferroptosis in colorectal cancer cells via degradation of ATP7A. Mol Oncol 2021;15:3527-44. [Crossref] [PubMed]
- Tsvetkov P, Coy S, Petrova B, et al. Copper induces cell death by targeting lipoylated TCA cycle proteins. Science 2022;375:1254-61. [Crossref] [PubMed]
- Qi X, Wang J, Che X, et al. The potential value of cuprotosis (copper-induced cell death) in the therapy of clear cell renal cell carcinoma. Am J Cancer Res 2022;12:3947-66.
- Bridges MC, Daulagala AC, Kourtidis A. LNCcation: lncRNA localization and function. J Cell Biol 2021;220:e202009045. [Crossref] [PubMed]
- Bhan A, Soleimani M, Mandal SS. Long Noncoding RNA and Cancer: A New Paradigm. Cancer Res 2017;77:3965-81. [Crossref] [PubMed]
- Zhang Z, Zhang W, Wang Y, et al. Construction and Validation of a Ferroptosis-Related lncRNA Signature as a Novel Biomarker for Prognosis, Immunotherapy and Targeted Therapy in Hepatocellular Carcinoma. Front Cell Dev Biol 2022;10:792676. [Crossref] [PubMed]
- Tang Y, Li C, Zhang YJ, et al. Ferroptosis-Related Long Non-Coding RNA signature predicts the prognosis of Head and neck squamous cell carcinoma. Int J Biol Sci 2021;17:702-11. [Crossref] [PubMed]
- Zhong F, Yao F, Cheng Y, et al. m6A-related lncRNAs predict prognosis and indicate immune microenvironment in acute myeloid leukemia. Sci Rep 2022;12:1759. [Crossref] [PubMed]
- Xuan J, Zhu D, Cheng Z, et al. Crocin inhibits the activation of mouse hepatic stellate cells via the lnc-LFAR1/MTF-1/GDNF pathway. Cell Cycle 2020;19:3480-90. [Crossref] [PubMed]
- Fan JB, Zhang Y, Liu W, et al. Long Non-Coding RNA MALAT1 Protects Human Osteoblasts from Dexamethasone-Induced Injury via Activation of PPM1E-AMPK Signaling. Cell Physiol Biochem 2018;51:31-45. [Crossref] [PubMed]
- Han J, Hu Y, Liu S, et al. A Newly Established Cuproptosis-Associated Long Non-Coding RNA Signature for Predicting Prognosis and Indicating Immune Microenvironment Features in Soft Tissue Sarcoma. J Oncol 2022;2022:8489387. [Crossref] [PubMed]
- Yang L, Yu J, Tao L, et al. Cuproptosis-Related lncRNAs are Biomarkers of Prognosis and Immune Microenvironment in Head and Neck Squamous Cell Carcinoma. Front Genet 2022;13:947551. [Crossref] [PubMed]
- Wang Z, Jensen MA, Zenklusen JC. A Practical Guide to The Cancer Genome Atlas (TCGA). Methods Mol Biol 2016;1418:111-41. [Crossref] [PubMed]
- Dong J, Wang X, Xu C, et al. Inhibiting NLRP3 inflammasome activation prevents copper-induced neuropathology in a murine model of Wilson's disease. Cell Death Dis 2021;12:87. [Crossref] [PubMed]
- Xu B, Wang S, Li R, et al. Disulfiram/copper selectively eradicates AML leukemia stem cells in vitro and in vivo by simultaneous induction of ROS-JNK and inhibition of NF-kappaB and Nrf2. Cell Death Dis 2017;8:e2797. [Crossref] [PubMed]
- Wilkerson MD, Hayes DN. ConsensusClusterPlus: a class discovery tool with confidence assessments and item tracking. Bioinformatics 2010;26:1572-3. [Crossref] [PubMed]
- Gao J, Kwan PW, Shi D. Sparse kernel learning with LASSO and Bayesian inference algorithm. Neural Netw 2010;23:257-64. [Crossref] [PubMed]
- Hänzelmann S, Castelo R, Guinney J. GSVA: gene set variation analysis for microarray and RNA-seq data. BMC Bioinformatics 2013;14:7. [Crossref] [PubMed]
- Newman AM, Steen CB, Liu CL, et al. Determining cell type abundance and expression from bulk tissues with digital cytometry. Nat Biotechnol 2019;37:773-82. [Crossref] [PubMed]
- Liu Y, Hu J, Liu D, et al. Single-cell analysis reveals immune landscape in kidneys of patients with chronic transplant rejection. Theranostics 2020;10:8851-62. [Crossref] [PubMed]
- Yoshihara K, Shahmoradgoli M, Martínez E, et al. Inferring tumour purity and stromal and immune cell admixture from expression data. Nat Commun 2013;4:2612. [Crossref] [PubMed]
- Jiang P, Gu S, Pan D, et al. Signatures of T cell dysfunction and exclusion predict cancer immunotherapy response. Nat Med 2018;24:1550-8. [Crossref] [PubMed]
- Ringel AE, Drijvers JM, Baker GJ, et al. Obesity Shapes Metabolism in the Tumor Microenvironment to Suppress Anti-Tumor Immunity. Cell 2020;183:1848-1866.e26. [Crossref] [PubMed]
- Sharabi A, Kim SS, Kato S, et al. Exceptional Response to Nivolumab and Stereotactic Body Radiation Therapy (SBRT) in Neuroendocrine Cervical Carcinoma with High Tumor Mutational Burden: Management Considerations from the Center For Personalized Cancer Therapy at UC San Diego Moores Cancer Center. Oncologist 2017;22:631-7. [Crossref] [PubMed]
- Campesato LF, Barroso-Sousa R, Jimenez L, et al. Comprehensive cancer-gene panels can be used to estimate mutational load and predict clinical benefit to PD-1 blockade in clinical practice. Oncotarget 2015;6:34221-7. [Crossref] [PubMed]
- Yang D, Liu Y, Bai C, et al. Epidemiology of lung cancer and lung cancer screening programs in China and the United States. Cancer Lett 2020;468:82-7. [Crossref] [PubMed]
- Topalian SL, Hodi FS, Brahmer JR, et al. Safety, activity, and immune correlates of anti-PD-1 antibody in cancer. N Engl J Med 2012;366:2443-54. [Crossref] [PubMed]
- Topalian SL, Taube JM, Anders RA, et al. Mechanism-driven biomarkers to guide immune checkpoint blockade in cancer therapy. Nat Rev Cancer 2016;16:275-87. [Crossref] [PubMed]
- Leighl NB. Meeting Immunotherapy Resistance in Lung Cancer. J Thorac Oncol 2021;16:187-90. [Crossref] [PubMed]
- Wu SG, Shih JY. Management of acquired resistance to EGFR TKI-targeted therapy in advanced non-small cell lung cancer. Mol Cancer 2018;17:38. [Crossref] [PubMed]
- da Silva DA, De Luca A, Squitti R, et al. Copper in tumors and the use of copper-based compounds in cancer treatment. J Inorg Biochem 2022;226:111634. [Crossref] [PubMed]
- Oliveri V. Selective Targeting of Cancer Cells by Copper Ionophores: An Overview. Front Mol Biosci 2022;9:841814. [Crossref] [PubMed]
- Kahlson MA, Dixon SJ. Copper-induced cell death. Science 2022;375:1231-2. [Crossref] [PubMed]
- Wang H, Wu X, Chen Y. Stromal-Immune Score-Based Gene Signature: A Prognosis Stratification Tool in Gastric Cancer. Front Oncol 2019;9:1212. [Crossref] [PubMed]
- Bruni D, Angell HK, Galon J. The immune contexture and Immunoscore in cancer prognosis and therapeutic efficacy. Nat Rev Cancer 2020;20:662-80. [Crossref] [PubMed]
- Tokunaga R, Naseem M, Lo JH, et al. B cell and B cell-related pathways for novel cancer treatments. Cancer Treat Rev 2019;73:10-9. [Crossref] [PubMed]
- Grisaru-Tal S, Itan M, Klion AD, et al. A new dawn for eosinophils in the tumour microenvironment. Nat Rev Cancer 2020;20:594-607. [Crossref] [PubMed]
- Gerhard GM, Bill R, Messemaker M, et al. Tumor-infiltrating dendritic cell states are conserved across solid human cancers. J Exp Med 2021;218:e20200264. [Crossref] [PubMed]
- Ribatti D, Crivellato E. Mast cells, angiogenesis, and tumour growth. Biochim Biophys Acta 2012;1822:2-8. [Crossref] [PubMed]
- Basu A, Ramamoorthi G, Albert G, et al. Differentiation and Regulation of T(H) Cells: A Balancing Act for Cancer Immunotherapy. Front Immunol 2021;12:669474. [Crossref] [PubMed]
- Gabrilovich DI, Ostrand-Rosenberg S, Bronte V. Coordinated regulation of myeloid cells by tumours. Nat Rev Immunol 2012;12:253-68. [Crossref] [PubMed]
- Jaillon S, Ponzetta A, Di Mitri D, et al. Neutrophil diversity and plasticity in tumour progression and therapy. Nat Rev Cancer 2020;20:485-503. [Crossref] [PubMed]
- Vivier E, Ugolini S, Blaise D, et al. Targeting natural killer cells and natural killer T cells in cancer. Nat Rev Immunol 2012;12:239-52. [Crossref] [PubMed]
- Yarchoan M, Hopkins A, Jaffee EM. Tumor Mutational Burden and Response Rate to PD-1 Inhibition. N Engl J Med 2017;377:2500-1. [Crossref] [PubMed]
(English Language Editor: B. Draper)