Prediction models for respiratory outcomes in patients with COVID-19: integration of quantitative computed tomography parameters, demographics, and laboratory features
Introduction
Coronavirus disease 2019 (COVID-19), caused by severe acute respiratory syndrome coronavirus (SARS-CoV-2), has been the most important health concern worldwide over the past couple of years (1). Its impact is still ongoing, and a large amount of material and human resources have been devoted to the diagnosis and treatment of COVID-19, which has a wide clinical spectrum, ranging from mild to critical disease (2,3). Most confirmed cases are classified as mild, while some require hospitalization or even progress to respiratory failure and death (4). Timely detection of high-risk patients is important for delivering proper management and follow-up assessments while optimizing the use of limited resources.
Previous studies have suggested a few models that can be used for the early identification of high-risk patients based on clinical characteristics and laboratory evaluations (5-7). Zhou et al. developed a multivariable prediction model based on demographic, comorbidity, and laboratory data using territory-wide electronic health records of 4,442 patients (5). Various clinical characteristics, including sex, age, presence of cardiovascular disease, and several initial laboratory findings, including neutrophil count, and urea, D-dimer, and lactate dehydrogenase (LDH) levels, were included in the model. Similarly, Hu et al. proposed a clinical model to predict mortality early; age, lymphocyte count, and levels of high-sensitivity C-reactive protein (CRP) and D-dimer were informative for patient outcomes (7). In addition, recent advances in machine learning have enabled the extraction of features from multiple clinical and laboratory variables and more accurate modelling (8-10). Although these studies showed good performance of their models, incorporating imaging biomarkers, such as quantitative chest computed tomography (CT) parameters, into other clinical features may further enhance accuracy.
Chest CT is an important imaging tool for diagnosing COVID-19. Previous studies have shown that the use of artificial intelligence (AI) in CT analysis may facilitate more effective diagnosis of COVID-19 (11-13). Öztürk et al. showed that rapid detection of COVID-19 was made with a machine learning method by analyzing chest X-ray and CT images (11). The extent of pneumonia can also be automatically quantified on chest CT images using AI, which is useful in predicting the progression to critical illness in patients with COVID-19 (14-16). Although previous studies have reported on the accuracy of machine-learning models that were trained based on the pattern and texture of COVID-19 pneumonia from CT images, such models require a learning process. Instead, automated CT quantification parameters can be easily measured and obtained through software. We hypothesized that incorporating quantitative CT parameters, especially parameters that quantify the pneumonia extent, into other clinical variables would help build a prediction model with favorable performance. This integrative model may enable simple and fast identification of high-risk patients at an early stage of the disease. This study aimed to develop integrative machine-learning models using quantitative CT parameters in addition to initial clinical features to predict the respiratory outcomes of COVID-19. We present the following article in accordance with the STARD reporting checklist (available at https://jtd.amegroups.com/article/view/10.21037/jtd-22-1076/rc).
Methods
Study patients and data collection
This was a retrospective cohort study. Patients hospitalized at Ilsan Paik Hospital for COVID-19 between September 1 and December 31, 2021 were included. Although genotyping of SARS-CoV-2 was not performed in our study patients, the Delta variant may have been the predominant type among the study patients because the detection rate of the Delta variant was greater than 50% of the local cases in our country by the end of July 2021. The Omicron variant had not yet become the dominant variant until January 2022. All cases were confirmed by reverse transcriptase-polymerase chain reaction (RT-PCR). Patients admitted during the acute stage of disease were included. Baseline characteristics, including age, sex, height, weight, vaccination history, comorbidities, and initial oxygen saturation were obtained from electronic medical records. The cycle threshold (Ct) values of the RdRp gene from RT-PCR were also recorded. All patients underwent radiological evaluation. Some patients underwent chest CT, and the need for chest CT was determined by each attending physician.
Definition of respiratory outcomes
Prediction models were developed for the following respiratory outcomes: pneumonia, hypoxia, and respiratory failure. Pneumonia was defined as newly developed pulmonary infiltrates detected on chest radiography or chest CT. Hypoxia was defined as an oxygen saturation <94% on room air. Respiratory failure was defined as the requirement of oxygen supply via a high-flow nasal cannula, mechanical ventilation, and/or extracorporeal membrane oxygenation.
Laboratory test measurements
In all patients, routine blood tests were performed at admission, including complete blood cell count with differentials, liver function tests, and LDH, CRP, procalcitonin, fibrinogen, D-dimer, and ferritin levels. Tests for SARS-CoV-2 were performed using ExiPrep 48 Dx (Bioneer, Daejeon, Korea) for nucleic acid extraction and the STANDARD M nCoV Real-Time Detection Kit (SD Biosensor, Suwon, Korea) for RT-PCR targeting the RdRp gene of SARS-CoV-2. All procedures were performed in accordance with the manufacturer’s instructions.
Quantitative chest CT analyses
Chest CT images were obtained using standardized CT screening protocols at a tube voltage of 120 kVP and current of 50 mA, which were applied in the high-pitch spiral mode (Aquilion One, Toshiba). The acquired CT images were reconstructed using kernel conversion with 1.0 mm slice thickness and analyzed using commercial software (Aview® system; Coreline Soft Inc., Seoul, Republic of Korea) which was based on deep learning artificial intelligence and customized for our CT protocol. Whole-lung images were extracted from the chest wall, mediastinum, and large airways, and attenuation coefficients of pixels were measured sequentially for indexes including the quantified percentage of low-attenuation area (LAA) less than −950 Hounsfield units (HU), high-attenuation area (HAA) between −600 and −250 HU, and consolidation between −100 and 0 HU using a multilayer convolutional neural network (17). At least one board-certified radiologist reviewed the CT images.
Ethical statement
This study was conducted in accordance with the Declaration of Helsinki (as revised in 2013). The study protocol was approved by the Institutional Review Board of Ilsan Paik Hospital (No. 2022-01-025). The need for informed consent was waived due to the retrospective nature of the study.
Statistical analysis
Patient characteristics are presented as means and standard deviations for continuous variables and as relative frequencies for categorical variables. Statistical analyses were performed using R software (version 3.6.0). Continuous variables were compared using a Student’s t-test or analysis of variance, and categorical variables were compared using a chi-squared test or Fisher’s exact test. For multivariable analysis of respiratory outcomes, logistic regression was performed using demographic variables, Ct values, blood biomarkers, and quantitative CT parameters. We filtered for multicollinearity of the variables to ensure that all variance inflation factors were <10. The best logistic regression model was selected using backward elimination. To assess the accuracy of each model, the area under the curve (AUC) of the receiver operating characteristic (ROC) curve was calculated using the ROCR package. To assess the predictive validity, 10-fold cross-validation was performed using the boot package. Machine learning was performed by random forest using the randomForest package, and the developed models were cross-validated with 10-fold cross-validation for accuracy.
Results
Patient clinical characteristics
A total of 389 hospitalizations due to COVID-19 were identified during the study period. Two patients who were transferred from other hospitals for post-acute care were excluded, leaving 387 patients included in the current study. The clinical characteristics of patients are shown in Table 1. The mean patient age was 57.8 years, and 194 (50.1%) were female. Of them, 204 (52.7%) were fully vaccinated. Among the study patients, 147 patients underwent chest CT whereas 240 patients did not at the time of diagnosis. The baseline characteristics between patients with and without chest CT scan are compared in Table S1.
Table 1
Variables | Total (N=387) |
---|---|
Demographics | |
Age, years | 57.8±18.2 |
Sex | |
Male | 193 (49.9) |
Female | 194 (50.1) |
Body mass index, kg/m2 | 25.4±4.4 |
Vaccination | 204 (52.7) |
Comorbidities | |
Hypertension | 151 (39.0) |
Diabetes | 72 (18.6) |
Cardiovascular disease | 44 (11.4) |
Cancer | 27 (7.0) |
Chronic lung disease | 23 (5.9) |
Chronic kidney disease | 17 (4.4) |
Cerebrovascular disease | 17 (4.4) |
Solid organ transplantation | 5 (1.3) |
Clinical course | |
Asymptomatic infection | 40 (10.3) |
Time to admission from symptom onset, days (n=347) | 4.0 [2.0, 6.0] |
Respiratory outcomes | |
Pneumonia | 195 (50.4) |
Hypoxia | 85 (22.0) |
Respiratory failure | 19 (4.9) |
Treatment | |
Regdanvimab | 215 (55.6) |
Corticosteroid | 107 (27.6) |
Remdesivir | 22 (18.8) |
Tocilizumab | 9 (2.3) |
Time to respiratory failure, days (n=19) | 2.0 [1.0, 4.0] |
Duration of supplemental oxygen, days (n=85) | 4.0 [2.0, 5.0] |
Duration of hospitalization, days | 7.0 [5.0, 9.0] |
Data are presented as numbers (%), mean ± standard deviation or medians [interquartile ranges].
At the initial presentation, 40 patients (10.3%) did not have any symptoms. In symptomatic patients, the median interval between symptom onset and hospital admission was four days. A total of 195 (50.4%) patients developed pneumonia, 85 (22.0%) developed hypoxia, and 19 (4.9%) progressed to respiratory failure during their clinical course. A Venn diagram of these outcomes is shown in Figure S1. The median time to respiratory failure from hospitalization was 2 days, and the median duration of oxygen supplementation was 4 days.
Comparison of the baseline demographic, laboratory, and CT characteristics according to the occurrence of respiratory outcomes
Table 2 compares the baseline demographic, microbiological, laboratory, and quantitative CT features of patients according to the occurrence of each respiratory outcome: pneumonia, hypoxia, and respiratory failure. Patients with pneumonia accounted for a significantly higher proportion of unvaccinated patients than those without pneumonia (61.5% vs. 32.8%, P<0.001). They showed significantly higher levels of LDH, aspartate aminotransferase (AST), CRP, fibrinogen, ferritin, and neutrophil percentages, and significantly lower platelet counts and lymphocyte percentages than those without pneumonia.
Table 2
Variables | Pneumonia | Hypoxia | Respiratory failure | ||||||||
---|---|---|---|---|---|---|---|---|---|---|---|
Present, 195 (50.4) | Absent, 192 (49.6) | P | Present, 85 (22.0) | Absent, 302 (78.0) | P | Present, 19 (4.9) | Absent, 368 (95.1) | P | |||
Demographics | |||||||||||
Age, years | 58.5±17.5 | 57.1±18.9 | 0.466 | 62.5±16.3 | 56.5±18.5 | 0.006 | 66.2±12.1 | 57.4±18.4 | 0.006 | ||
Female, sex | 92 (47.4) | 102 (53.1) | 0.308 | 41 (48.8) | 153 (50.7) | 0.859 | 10 (52.6) | 184 (50.1) | >0.999 | ||
Body mass index | 25.4±4.3 | 25.4±4.6 | 0.976 | 25.9±4.1 | 25.2±4.5 | 0.238 | 26.5±3.3 | 25.3±4.5 | 0.246 | ||
Vaccination | 75 (38.5) | 129 (67.2) | <0.001 | 32 (37.6) | 172 (57.0) | 0.002 | 7 (36.8) | 197 (53.5) | 0.236 | ||
Initial SpO2 | 94.9±5.6 | 97.1±1.6 | <0.001 | 92.5±7.7 | 97.0±1.5 | <0.001 | 86.8±13.7 | 96.5±2.2 | 0.007 | ||
Hypertension | 68 (34.9) | 83 (43.2) | 0.114 | 36 (42.4) | 115 (38.1) | 0.557 | 12 (63.2) | 139 (37.8) | 0.049 | ||
Diabetes | 42 (21.5) | 30 (15.6) | 0.173 | 22 (25.9) | 50 (16.6) | 0.073 | 7 (36.8) | 65 (17.7) | 0.073 | ||
Cardiovascular disease | 24 (12.3) | 20 (10.4) | 0.670 | 13 (15.3) | 31 (10.3) | 0.273 | 5 (26.3) | 39 (10.6) | 0.083 | ||
Chronic kidney disease | 10 (5.1) | 7 (3.6) | 0.643 | 4 (4.7) | 13 (4.3) | >0.999 | 3 (15.8) | 14 (3.8) | 0.056 | ||
Cerebrovascular disease | 8 (4.1) | 9 (4.7) | 0.974 | 2 (2.4) | 15 (5.0) | 0.460 | 1 (5.3) | 16 (4.3) | >0.999 | ||
RT-PCR Ct value | |||||||||||
RdRp gene | 18.9±5.8 | 19.0±5.8 | 0.915 | 19.1±5.4 | 19.0±5.9 | 0.860 | 16.7±4.1 | 19.1±5.9 | 0.081 | ||
Laboratory findings | |||||||||||
LDH, U/L | 311.1±125.1 | 219.6±70.1 | <0.001 | 379.9±138.0 | 233.6±76.5 | <0.001 | 483.1±169.5 | 254.6±95.1 | <0.001 | ||
AST, U/L | 37.7±25.5 | 29.1±16.5 | <0.001 | 42.0±23.1 | 31.0±20.9 | <0.001 | 59.9±34.3 | 32.1±20.3 | 0.003 | ||
ALT, U/L | 33.6±28.7 | 30.1±28.7 | 0.226 | 36.1±27.4 | 30.7±29.0 | 0.123 | 46.6±34.6 | 31.1±28.2 | 0.026 | ||
CRP, mg/dL | 5.3±6.4 | 1.1±1.4 | <0.001 | 8.7±8.0 | 1.7±2.1 | <0.001 | 14.2±11.5 | 2.6±3.7 | <0.001 | ||
Fibrinogen, mg/dL | 522.8±142.0 | 396.1±108.5 | <0.001 | 572.3±156. | 428.8±120.1 | <0.001 | 618.4±140.9 | 451.4±136.7 | <0.001 | ||
D-dimer, μg/dL | 1.2±3.0 | 0.8±1.9 | 0.077 | 1.7±3.4 | 0.8±2.2 | 0.029 | 2.1±4.5 | 0.9±2.4 | 0.284 | ||
Ferritin, ng/mL | 550.8±513.6 | 249.5±233.7 | <0.001 | 678.5±511.3 | 335.5±374.7 | <0.001 | 1018.3±661.0 | 369.6±391.3 | 0.013 | ||
WBC, /μL*1,000 | 5.9±2.9 | 5.4±4.6 | 0.22 | 6.9±3.4 | 5.2±3.9 | <0.001 | 8.3±4.4 | 5.5±3.7 | 0.002 | ||
Platelet, /μL*1,000 | 201.2±87.7 | 220.6±65.7 | 0.014 | 197.0±83.5 | 214.8±76.2 | 0.063 | 190.8±69.6 | 211.9±78.5 | 0.253 | ||
Neutrophil (%) | 67.1±13.8 | 58.7±11.9 | <0.001 | 74.3±12.7 | 59.7±12.0 | <0.001 | 82.6±8.9 | 61.9±13.0 | <0.001 | ||
Lymphocyte (%) | 23.5±11.9 | 29.0±10.7 | <0.001 | 17.8±10.0 | 28.6±10.9 | <0.001 | 12.0±6.5 | 27.0±11.3 | <0.001 | ||
Image findings | 103 (52.8) | 43 (22.4) | <0.001 | 55 (65.5) | 91 (30.1) | <0.001 | 14 (77.8) | 132 (35.9) | 0.001 | ||
LAA (%) | 2.8±3.3 | 4.6±4.4 | 0.008 | 3.2±3.9 | 3.4±3.6 | 0.728 | 1.3±1.1 | 3.6±3.8 | <0.001 | ||
HAA (%) | 13.5±7.5 | 7.7±3.7 | <0.001 | 16.5±7.9 | 8.9±4.7 | <0.001 | 23.0±7.7 | 10.6±6.0 | <0.001 | ||
Consolidation (%) | 0.5±0.6 | 0.2±0.4 | 0.001 | 0.6±0.7 | 0.3±0.4 | <0.001 | 0.8±1.1 | 0.3±0.5 | 0.100 |
Data are presented as numbers (%) or mean ± standard deviation. SpO2, oxygen saturation; RT-PCR, reverse transcriptase-polymerase chain reaction; Ct, cycle threshold; LDH, lactate dehydrogenase; AST, aspartate aminotransferase; ALT, alanine aminotransferase; CRP, C-reactive protein; WBC, white blood cell; LAA, low-attenuation area; HAA, high-attenuation area.
Patients who developed hypoxia were significantly older and less likely to be vaccinated than those who did not have hypoxia. In addition to the variables that were significantly higher in patients with pneumonia than in those without pneumonia, D-dimer levels and white blood cell counts were significantly higher in patients with hypoxia than in those without hypoxia.
Patients who progressed to respiratory failure were significantly older and more frequently had hypertension at baseline than those who did not develop respiratory failure. While the Ct values of the RdRp gene were lower in this group, they were not statistically different. A comparison of laboratory findings between patients with respiratory failure and those without showed a similar pattern to that between patients with and without hypoxia.
HAA (%) was significantly higher in all patients with pneumonia, hypoxia, or respiratory failure than in those without. LAA (%) was significantly lower in patients with pneumonia or respiratory failure than in those without pneumonia. Consolidation (%) was significantly higher in patients with pneumonia and hypoxia. Density plots displaying the distributions of HAA (%) and LAA (%) in each outcome group are summarized in Figure 1.
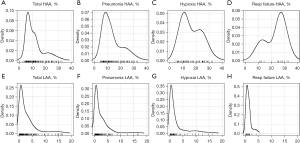
Logistic regression model for the prediction of respiratory outcomes
The results of the unadjusted logistic regression analysis of the respiratory outcomes are summarized in Table S2. Age was significantly associated with hypoxia and respiratory failure. Unvaccinated status was associated with pneumonia and hypoxia, but not with respiratory failure. Hypertension, diabetes, cardiovascular disease, and chronic kidney disease were significantly associated with respiratory failure. Neutrophil and lymphocyte percentages and levels of AST, CRP, HAA (%), and consolidation (%) were associated with all three respiratory outcomes.
In the multivariable analysis, vaccination status and levels of LDH, CRP, and fibrinogen were selected as independent variables to predict pneumonia. To predict hypoxia, the presence of hypertension and levels of LDH, CRP, HAA (%), and consolidation (%) were chosen. For respiratory failure, the presence of diabetes and levels of AST, CRP, HAA (%), and consolidation extent were selected (Table 3). The corresponding ROC curves are shown in Figure 2. The AUC values of the ROC curve were 0.904 for pneumonia, 0.890 for hypoxia, and 0.969 for respiratory failure. The predictive validities using the 10-fold cross-validation of the models for predicting pneumonia, hypoxia, and respiratory failure were 0.872, 0.878, and 0.945, respectively.
Table 3
Variables | Odds ratio | 95% CI |
---|---|---|
Pneumonia | ||
Unvaccinated | 3.85 | 2.27−6.67 |
LDH, U/L | 1.01 | 1.00−1.01 |
CRP, mg/dL | 1.41 | 1.17−1.70 |
Fibrinogen, mg/dL | 1.01 | 1.00−1.01 |
Hypoxia | ||
Hypertension | 2.80 | 1.05−7.45 |
LDH, U/L | 1.01 | 1.00−1.02 |
CRP, mg/dL | 1.19 | 1.02−1.38 |
HAA. % | 1.09 | 1.00−1.19 |
Consolidation, % | 2.97 | 1.10−8.00 |
Respiratory failure | ||
Diabetes | 9.29 | 1.36−63.58 |
AST, U/L | 1.05 | 1.02−1.09 |
CRP, mg/dL | 1.15 | 1.04−1.28 |
HAA, % | 1.24 | 1.10−1.39 |
The model was developed with demographic, microbiological, and laboratory features, in addition to quantitative CT parameters. CI, confidence interval; LDH, lactate dehydrogenase; CRP, C-reactive protein; HAA, high-attenuation area; AST, aspartate aminotransferase; CT, computed tomography.
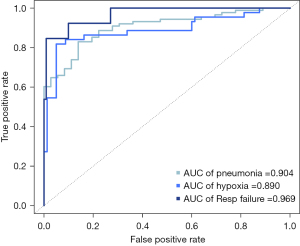
Random forest model for prediction of respiratory outcomes
Figure 3 shows the variable importance in the random forest prediction models for the feature selection of the occurrence of pneumonia, hypoxia, and respiratory failure; they are described in order of importance based on the mean decrease in the Gini index. The top 10 predictors for pneumonia were ferritin, CRP, fibrinogen, platelet count, neutrophil percentage, HAA (%), LDH, age, vaccination status, and white blood cell; predictors for hypoxia were LDH, CRP, neutrophil percentage, fibrinogen, procalcitonin, ferritin, HAA (%), LAA (%), lymphocyte percentage, and AST; and predictors for respiratory failure were HAA (%), CRP, LDH, AST, procalcitonin, Ct value of RdRp gene, ferritin, presence of chronic kidney disease, neutrophil percentage, and body mass index. A random forest model was developed for each respiratory outcome. The ROC curves are shown in Figure S2. The AUC values of the ROC curve were 0.828 for pneumonia, 0.797 for hypoxia, and 0.922 for respiratory failure. To validate the outcome prediction, 10-fold cross-validation was performed. The accuracies of the random forest models for the cross-validation of pneumonia, hypoxia, and respiratory failure were 0.769, 0.835, and 0.934, respectively.
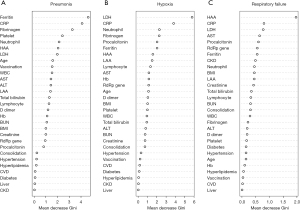
Discussion
In this study, we developed integrative prediction models for the respiratory outcomes of COVID-19 using quantitative CT parameters, demographics, and laboratory variables. The performance of the logistic regression model was satisfactory, especially in predicting respiratory failure (AUC of 0.969). Interestingly, HAA was one of the top features in the random forest models for predicting respiratory failure, supporting the role of quantitative CT in predicting the respiratory outcomes of patients with COVID-19. The performance of the random forest prediction model was also satisfactory for predicting respiratory failure (AUC of 0.916). Integrative machine-learning models may help provide an accurate prediction of respiratory outcomes in patients with COVID-19.
Chest CT is crucial for the diagnosis, evaluation of severity, and follow-up of COVID-19 (18,19). Previous studies have shown that both visual evaluation by radiologists and rapid automated assessment using AI software are useful for the evaluation of COVID-19 (16,20-23). Pang et al. used AI software to analyze the chest CT images of 140 patients with COVID-19 (16). They found that the percentage of pneumonia volume was positively correlated with inflammatory markers, such as neutrophil percentage, erythrocyte sedimentation rate, and LDH levels. Using a cut-off value of 22.6%, the percentage of pneumonia volume showed good performance (AUC of 0.868) for predicting critical illness with a sensitivity and specificity of 81.3% and 80.6%, respectively. Li et al. also found that the proportion of lungs with pneumonia measured by a deep learning-based algorithm predicted COVID-19 patients who later developed severe disease (24). They suggested that a CT scan performed as early as five days after the initial onset of symptoms can be used to identify patients who may progress to severe disease. In our study, HAA, the quantified percentage of imaged lung volume with attenuation values between −600 and −250 HU, was consistently higher in patients with pneumonia, hypoxia, or respiratory failure than in those without, and it was a significant predictor of hypoxia and respiratory failure in the logistic regression models. Quantitative CT analysis using AI software has the advantage of being faster and less labor intensive than visual assessment, which could be particularly useful in situations such as the current COVID-19 pandemic.
Machine-learning-based models have been widely adopted to increase diagnostic accuracy and predictability in various medical research areas (25). Random forest was used to rank features to predict the respiratory outcomes of pneumonia, hypoxia, and respiratory failure in patients with COVID-19. Several laboratory variables, such as ferritin, CRP, and LDH, were found to predict respiratory outcomes from the models, which is in line with the results of previous studies (5,26-28). Notably, HAA was the most important predictor of respiratory failure. HAA reflects the areas of consolidation and ground-glass opacities, thus representing the extent of pneumonia. This finding supports the role of quantitative CT analysis in determining the prognosis of COVID-19 patients. Further studies to expand this work and external validation are required.
This study has some limitations that should be considered. First, due to the retrospective nature of the study, Chest CT was not regularly performed in all study patients. The reasons for performing chest CT included unclear diagnosis of pneumonia with chest radiographs alone, need for more precise evaluation of the extent of pneumonia, or suspicion of pulmonary embolism. In the rest of the patients, chest CT was not performed because pneumonia was evident with chest radiograph alone or patients’ unstable vital signs did not allow chest CT examinations. This heterogeneity may limit generalizability of the study results. Our approach with integrative prediction models should be tested in further studies. Second, a relatively small number of patients developed respiratory failure. In the Republic of Korea, all patients with COVID-19 are classified according to their initial severity to determine where they should be treated according to the Korea Disease Control and Prevention Agency guidelines. Our hospital is designated to treat patients with mild, moderate, or severe COVID-19. Patients with critical illnesses at the time of diagnosis were not admitted to our hospital. Therefore, the results of our study may be limited to predicting later respiratory outcomes in patients who initially present with mild, moderate, or severe disease. Third, we did not perform a visual assessment of chest CT images. Although HAA can be considered to represent the extent of pneumonia, it fails to distinguish other causes of increased density, such as atelectasis or post-inflammatory sequelae. Fourth, treatment for COVID-19 was not considered in the prediction model. Since the aim of this study was to develop prediction models with initial clinical, laboratory, and imaging features, treatment given during the hospital course was not taken into account in the models. So far, an effective cure for COVID-19 has not been established, but treatment with corticosteroids or remdesivir is considered helpful in select patients with COVID-19 (20,29). Therefore, how these therapies might alter the clinical course of patients remains unclear. Lastly, our models were not validated externally. Although we performed cross-validation, overestimation of the generalizability might have occurred. Further studies need to validate our models using new data from different settings.
Conclusions
In conclusion, we developed machine-learning-based prediction models for respiratory outcomes in patients with COVID-19, incorporating quantitative CT parameters into clinical and laboratory variables. The models exhibited good performance and accuracy. The early identification of at-risk patients using this strategy will help triage patients and deliver management plans more efficiently.
Acknowledgments
Funding: None.
Footnote
Provenance and Peer Review: This article was commissioned by the Guest Editors (Jing Cheng, Tao Xu, Zifeng Yang, Wenda Guan) for the series “Current Status of Diagnosis and Forecast of COVID-19” published in Journal of Thoracic Disease. The article has undergone external peer review.
Reporting Checklist: The authors have completed the STARD reporting checklist. Available at https://jtd.amegroups.com/article/view/10.21037/jtd-22-1076/rc
Data Sharing Statement: Available at https://jtd.amegroups.com/article/view/10.21037/jtd-22-1076/dss
Peer Review File: Available at https://jtd.amegroups.com/article/view/10.21037/jtd-22-1076/prf
Conflicts of Interest: All authors have completed the ICMJE uniform disclosure form (available at https://jtd.amegroups.com/article/view/10.21037/jtd-22-1076/coif). The series “Current Status of Diagnosis and Forecast of COVID-19” was commissioned by the editorial office without any funding or sponsorship. The authors have no other conflicts of interest to declare.
Ethical Statement: The authors are accountable for all aspects of the work in ensuring that questions related to the accuracy or integrity of any part of the work are appropriately investigated and resolved. This study was conducted in accordance with the Declaration of Helsinki (as revised in 2013). The study protocol was approved by the Institutional Review Board of Ilsan Paik Hospital (No. 2022-01-025). The need for informed consent was waived because of the retrospective nature of the study.
Open Access Statement: This is an Open Access article distributed in accordance with the Creative Commons Attribution-NonCommercial-NoDerivs 4.0 International License (CC BY-NC-ND 4.0), which permits the non-commercial replication and distribution of the article with the strict proviso that no changes or edits are made and the original work is properly cited (including links to both the formal publication through the relevant DOI and the license). See: https://creativecommons.org/licenses/by-nc-nd/4.0/.
References
- Wang C, Horby PW, Hayden FG, et al. A novel coronavirus outbreak of global health concern. Lancet 2020;395:470-3. [Crossref] [PubMed]
- Zhou F, Yu T, Du R, et al. Clinical course and risk factors for mortality of adult inpatients with COVID-19 in Wuhan, China: a retrospective cohort study. Lancet 2020;395:1054-62. [Crossref] [PubMed]
- Poston JT, Patel BK, Davis AM. Management of Critically Ill Adults With COVID-19. JAMA 2020;323:1839-41. [Crossref] [PubMed]
- Wu Z, McGoogan JM. Characteristics of and Important Lessons From the Coronavirus Disease 2019 (COVID-19) Outbreak in China: Summary of a Report of 72 314 Cases From the Chinese Center for Disease Control and Prevention. Jama 2020;323:1239-42. [Crossref] [PubMed]
- Zhou J, Lee S, Wang X, et al. Development of a multivariable prediction model for severe COVID-19 disease: a population-based study from Hong Kong. NPJ Digit Med 2021;4:66. [Crossref] [PubMed]
- Banoei MM, Dinparastisaleh R, Zadeh AV, et al. Machine-learning-based COVID-19 mortality prediction model and identification of patients at low and high risk of dying. Crit Care 2021;25:328. [Crossref] [PubMed]
- Hu C, Liu Z, Jiang Y, et al. Early prediction of mortality risk among patients with severe COVID-19, using machine learning. Int J Epidemiol 2021;49:1918-29. [Crossref] [PubMed]
- Zhou K, Sun Y, Li L, et al. Eleven routine clinical features predict COVID-19 severity uncovered by machine learning of longitudinal measurements. Comput Struct Biotechnol J 2021;19:3640-9. [Crossref] [PubMed]
- Liang W, Liang H, Ou L, et al. Development and Validation of a Clinical Risk Score to Predict the Occurrence of Critical Illness in Hospitalized Patients With COVID-19. JAMA Intern Med 2020;180:1081-9. [Crossref] [PubMed]
- Liang W, Yao J, Chen A, et al. Early triage of critically ill COVID-19 patients using deep learning. Nat Commun 2020;11:3543. [Crossref] [PubMed]
- Öztürk Ş, Özkaya U, Barstuğan M. Classification of Coronavirus (COVID-19) from X-ray and CT images using shrunken features. Int J Imaging Syst Technol 2021;31:5-15. [Crossref] [PubMed]
-
Özkaya U Öztürk Ş Serkan B Classification of COVID-19 in Chest CT Images using Convolutional Support Vector Machines. arXiv:201105746. -
Barstuğan M Özkaya U Öztürk Ş. Coronavirus (COVID-19) classification using CT images by machine learning methods. arXiv:200309424. - Cai W, Liu T, Xue X, et al. CT Quantification and Machine-learning Models for Assessment of Disease Severity and Prognosis of COVID-19 Patients. Acad Radiol 2020;27:1665-78. [Crossref] [PubMed]
- Lanza E, Muglia R, Bolengo I, et al. Quantitative chest CT analysis in COVID-19 to predict the need for oxygenation support and intubation. Eur Radiol 2020;30:6770-8. [Crossref] [PubMed]
- Pang B, Li H, Liu Q, et al. CT Quantification of COVID-19 Pneumonia at Admission Can Predict Progression to Critical Illness: A Retrospective Multicenter Cohort Study. Front Med (Lausanne) 2021;8:689568. [Crossref] [PubMed]
- Grassi R, Belfiore MP, Montanelli A, et al. COVID-19 pneumonia: computer-aided quantification of healthy lung parenchyma, emphysema, ground glass and consolidation on chest computed tomography (CT). Radiol Med 2021;126:553-60. [Crossref] [PubMed]
- Liu J, Yu H, Zhang S. The indispensable role of chest CT in the detection of coronavirus disease 2019 (COVID-19). Eur J Nucl Med Mol Imaging 2020;47:1638-9. [Crossref] [PubMed]
- Liu Q, Pang B, Li H, et al. Machine learning models for predicting critical illness risk in hospitalized patients with COVID-19 pneumonia. J Thorac Dis 2021;13:1215-29. [Crossref] [PubMed]
- Salvatore C, Roberta F, Angela L, et al. Clinical and laboratory data, radiological structured report findings and quantitative evaluation of lung involvement on baseline chest CT in COVID-19 patients to predict prognosis. Radiol Med 2021;126:29-39. [Crossref] [PubMed]
- Sun D, Li X, Guo D, et al. CT Quantitative Analysis and Its Relationship with Clinical Features for Assessing the Severity of Patients with COVID-19. Korean J Radiol 2020;21:859-68. [Crossref] [PubMed]
- Liu F, Zhang Q, Huang C, et al. CT quantification of pneumonia lesions in early days predicts progression to severe illness in a cohort of COVID-19 patients. Theranostics 2020;10:5613-22. [Crossref] [PubMed]
- Yin X, Min X, Nan Y, et al. Assessment of the Severity of Coronavirus Disease: Quantitative Computed Tomography Parameters versus Semiquantitative Visual Score. Korean J Radiol 2020;21:998-1006. [Crossref] [PubMed]
- Li K, Liu X, Yip R, et al. Early prediction of severity in coronavirus disease (COVID-19) using quantitative CT imaging. Clin Imaging 2021;78:223-9. [Crossref] [PubMed]
- Sidey-Gibbons JAM, Sidey-Gibbons CJ. Machine learning in medicine: a practical introduction. BMC Med Res Methodol 2019;19:64. [Crossref] [PubMed]
- Ke C, Yu C, Yue D, et al. Clinical characteristics of confirmed and clinically diagnosed patients with 2019 novel coronavirus pneumonia: a single-center, retrospective, case-control study. Med Clin (Barc) 2020;155:327-34. [Crossref]
- Gao Y, Li T, Han M, et al. Diagnostic utility of clinical laboratory data determinations for patients with the severe COVID-19. J Med Virol 2020;92:791-6. [Crossref] [PubMed]
- Ma X, Ng M, Xu S, et al. Development and validation of prognosis model of mortality risk in patients with COVID-19. Epidemiol Infect 2020;148:e168. [Crossref] [PubMed]
- Horby P, Lim WS, Emberson JR, et al. Dexamethasone in Hospitalized Patients with Covid-19. N Engl J Med 2021;384:693-704. [Crossref] [PubMed]