Construction and validation of a tumor mutational burden and immune-related prognostic model for predicting the prognosis of patients with lung squamous cell carcinoma
Highlight box
Key findings
• We screened five hub immune genes and established a prognostic model related to the tumor mutational burden (TMB) and immunity, which can effectively predict the prognosis of lung squamous cell carcinoma (LUSC).
What is known and what is new?
• The TMB has important value as a biomarker in the field of immunotherapy. Cells with a high TMB are easily recognized by the immune system. Thus, immunotherapy is more effective and the survival rate is higher in patients with a high TMB.
• We established a prognostic model related to TMB and immunity. The model was reliable and stable and could be used as a prognostic biomarker for LUSC patients, which provides a theoretical basis for the clinical immunotherapy of LUSC.
What are the implications, and what should change now?
• Our prognostic model should be further validated in large-scale and prospective studies. Also, the molecular mechanism and function of the five hub immune genes require further study.
Introduction
Lung cancer is one of the leading causes of cancer-related death worldwide (1). Based on its biological characteristics, lung cancer can be divided into small cell lung cancer (SCLC) and non-small cell lung cancer (NSCLC), with NSCLC accounting for about 83% of cases (2). NSCLC can be further subdivided into lung adenocarcinoma, lung squamous cell carcinoma (LUSC), and large cell carcinoma, among which LUSC accounts for about 20% of all lung cancers (3). Owing to its occult onset and atypical early symptoms, LUSC is usually at advanced stages when it is clinically diagnosed. The treatment of patients with advanced LUSC mainly includes chemotherapy and targeted therapy (4,5); however, LUSC is less sensitive to radiotherapy, chemotherapy, and targeted therapy than lung adenocarcinoma (6,7), so the overall prognosis is very poor. At present, the progress in targeted therapy for LUSC is abnormally slow, mainly due to the lack of clear targets and effective targeted therapy drugs for LUSC (8). Therefore, there is a pressing need to find and identify the targets related to prognosis and treatment for improving the prognosis of LUSC patients.
In recent years, immunotherapy-related research has made progress in multiple tumors (9,10). The main principle of immune checkpoint inhibitors (ICIs) in the treatment of tumors is to target the immune recognition and immune response-related escape mechanism of tumor cells (11). With the increasing research on ICIs, including programmed death-1 (PD-1) and programmed death-ligand-1 (PD-L1) inhibitors, considerable progress has been made in the treatment of gene-negative advanced NSCLC (12). However, PD-L1, as an important biomarker for predicting the efficacy of anti-PD-1/PD-L1 drugs, has some limitations (13). It is not enough to accurately screen the highest-benefit immunotherapy population by using PD-L1 alone, and it needs to be combined with other indicators (14). Nowadays, in addition to PD-L1, researchers have also explored the value of the tumor mutational burden (TMB) as a biomarker in the field of immunotherapy (15).
The TMB refers to the number of somatic mutations following the deletion of germline mutations from the cancer genome, which is a biomarker of the tumor mutation level (16). At present, several clinical trials have shown that the TMB is positively correlated with the T-lymphocyte recognition antigen and immunotherapy effectiveness (17,18). Patients with higher TMBs are more likely to produce new antigens to stimulate immunity (19,20). Hence, the TMB can be used to predict the efficacy of PD-1/PD-L1 inhibitors in various cancers, including melanoma and lung cancer (21,22). A number of large studies, such as Check Mate-026 (23) and Check Mate-227 (24), have confirmed the predictive effect of TMB on immunotherapy efficacy of NSCLC. National Comprehensive Cancer Network (NCCN) guidelines have included TMB in the molecular pathological detection of advanced lung cancer. In addition, studies also have shown that TMB is predictive factor of improved clinical outcomes in advanced LUSC patients receiving immunotherapy, and the predictive value is more pronounced in patients treated with immunotherapy as a single agent (25). In conclusion, TMB has a certain prospect in predicting the efficacy of immunotherapy.
However, predicting the prognosis of LUSC using TMB-related genes and its in-depth mechanism need to be further explored. In this study, we screened the TMB and immune-related genes based on The Cancer Genome Atlas (TCGA) database and Immunology database analysis portal (Immport), and then constructed a prognostic prediction model of LUSC, aiming to provide effective biomarkers and new therapeutic targets for patients with LUSC. We present the following article in accordance with the TRIPOD reporting checklist (available at https://jtd.amegroups.com/article/view/10.21037/jtd-23-103/rc).
Methods
Study design
We aimed to find effective biomarkers based on TMB and immune response and establish a prognostic model of LUSC using bioinformatics analysis. The TCGA database was acted as a training set and used to establish a risk model. GSE37745 database was downloaded from GEO database and used to validate the predictive value of this model. We designed and processed our study as shown in the flow chart (Figure 1).
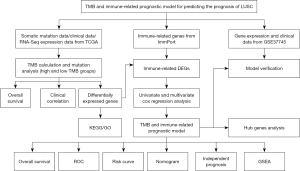
Source and processing of somatic mutation data
The somatic mutation data of LUSC was obtained from TCGA database. From the four subtypes of data files, we selected the “Masked Somatic Mutation” data, and used varscan 2.4.3 software package (Genome Institute, Washington University, Washington, DC, USA. http://www.ncbi.nlm.nih.gov/pubmed/22300766) for subsequent analysis. Loci with a depth of less than 100 were filtered out using the VarScan filter pipeline. We read the Mutation Annotation Format (MAF) of somatic variation and utilized the R maftools package (https://bioconductor.org/packages/release/bioc/html/maftools.html), which provided multiple analysis modules to perform the visualization process and draw the mutation graph.
Prognostic analysis based on the TMB
According to the above MAF files, the TMB of each sample was calculated and compared with the other TCGA cohorts using the tcgaComapare function in maftools package. The clinical data of LUSC were downloaded from TCGA database, including age, gender, tumor stage, T, N, M, survival time, survival state, etc., and samples with deficient clinical information above were excluded from the study. The surv_cutpoint function was used to obtain the optimal cut-off value, and then the LUSC samples were divided into low- and high-TMB groups according to this value. Kaplan-Meier analysis was performed using survival package to compare the relationship between the TMB and survival rate, and the correlation between the TMB level and clinical features was also evaluated.
TMB-related differentially expressed genes (DEGs) and functional pathways analysis
The RNA sequencing (RNA-seq) expression data of LUSC were downloaded from TCGA database, including 501 tumor samples. The samples were divided into high- and low-TMB groups based on the optimal cut-off value. The Limma package was used to screen LUSC-related DEGs with a Fold Change (FC) ≥0.58 and False Discovery Rate (FDR) <0.05. The results are represented by volcano and heat maps. The study was conducted in accordance with the Declaration of Helsinki (as revised in 2013).
Furthermore, we performed Gene Ontology (GO) and Kyoto Encyclopedia of Genes and Genomes (KEGG) analyses in R using the clusterProfiler function to determine the function and related pathways of the DEGs (26). Moreover, we obtained the list of immune-related genes from the Immport, which is one of the largest public repositories of human immunology data, and then screened the immune-related DEGs by Wayne analysis.
Construction and evaluation of an immune and TMB-related prognostic model
The gene expression and clinical data of LUSC were download from GSE37745 database, including 66 LUSC patients. All initial analyses were carried out in TCGA database to construct a signature based on TMB and immune sites, and then verified in GSE37745 database. We used univariate Cox analysis and multivariate Cox analysis to screen the hub genes and then constructed the prognostic model. Next, the model was evaluated by Kaplan-Meier analysis, OS was considered as the primary prognostic outcome. Receiver Operating Characteristic (ROC) curve, risk curve, and nomogram were used to verify the accuracy of the prognostic model. The survivalROC package was used for ROC curve analysis, and the rms package for nomogram analysis.
In addition, we analyzed the expression and prognostic value of the hub genes, as well as their correlation with immune cells.
Statistical analysis
The statistical analyses of this study were performed using R software (version 3.5.2). The t-test was utilized for comparisons between the two groups. P<0.05 was considered statistically significant.
Results
Landscape of mutation profiles in LUSC
The TMBs of different cancers in TCGA were shown in Figure 2A, and the TMB of LUSC ranked the second among all tumor, second only to SKCM. In summary (Figure 2B), these mutations were further classified according to different classification categories, most of which were missense mutations. The frequency of SNP was higher than that of insertion or deletion. C > T and C > A were the common SNVs in LUSC. Moreover, the number of changed bases was counted in each sample, and the mutation types of different colors in the block diagram of LUSC were displayed. Furthermore, the top 10 mutations in LUSC were presented, including TTN (68%), TP53 (77%), MUC16 (36%), CSMD3 (40%), RYR2 (35%), LRP1B (30%), USH2A (30%), SYNE1 (29%), ZFHX4 (26%), and KMT2D (22%). Meanwhile, the waterfall plot displayed the mutation information of the 30 frequently mutated genes in LUSC, and the 10 most frequently mutated genes were consistent with the above results (Figure 2C). Also, a heat map of the correlation between 20 frequently mutated genes is shown in Figure 2D, where green represented the cooccurrence relationship and brown represented the exclusion relationship.
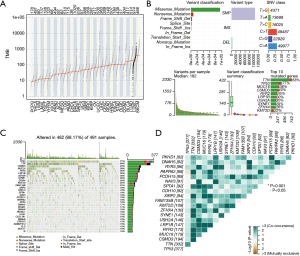
TMB was related to prognosis and pathological stages
According to the calculated TMB score and LUSC clinical data in TCGA, the optimal cut-off value was determined to be 2.605263. The samples were divided into high- and low-TMB groups according to the optimal cut-off value. Kaplan-Meier analysis revealed that the survival rate of the high TMB group was higher than that of the low TMB group, P<0.001 (Figure 3A). Moreover, higher TMB levels were associated with pathological stage, P=0.012 (Figure 3B). However, there was no significant difference in the correlation between TMB and age, gender, metastasis, lymph node metastasis, and tumor (Figure S1).
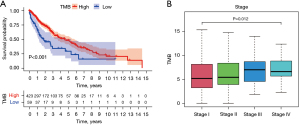
Identification of TMB-related DEGs based on TCGA database
A total of 595 DEGs were obtained in high- and low-TMB groups, including 178 up-regulated genes and 417 down-regulated genes (Figure 4A,4B). To explore the potential biological functions of these genes, we performed GO and KEGG enrichment analyses. GO analysis demonstrated that the DEGs were strongly enriched in B cell/lymphocyte mediated immunity, leukocyte/lymphocyte/B cell/macrophage/T cell activation, lymphocyte/T cell/mononuclear cell/leukocyte proliferation, humoral immune response, immunoglobulin production, external immunoglobulin complex, immune receptor activation for BP, enriched in immunoglobulin complex, external side of plasma membrane, MHC protein complex, cell-cell junction, blood microparticle for CC, and enriched in antigen binding, immunoglobulin receptor binding, MHC class II receptor activity, immune receptor activity for MF (Figure 4C). Also, KEGG analysis indicated that DEGs were markedly enriched in T helper type 1 (Th1) and T helper type 2 (Th2) cell differentiation, T helper type 17 (Th17) cell differentiation, PPAR signaling pathway, cytokine-cytokine receptor interaction, and Cell cycle (Figure 4D).
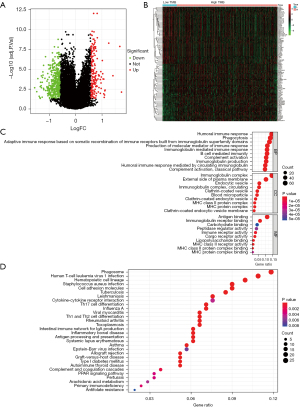
Construction and evaluation of the prognostic model
Wayne analysis showed 158 immune-related DEGs were obtained (Figure 5A). After combining 158 genes with survival data, univariate Cox regression showed that 24 genes were significant for the prognosis of LUSC (Figure 5B). Furthermore, multivariate Cox regression analysis showed that five hub genes (TINAGL1, FGFR2, CTSE, SFTPA1, and IGHV7-81) were finally included in the prognostic model (Figure 5C). The risk score formula was as follows: risk score = (TINAGL1 × 0.131846859) + [FGFR2 × (−0.095244735)] + [CTSE × (−0.100214254)] + (SFTPA1 × 0.084305089) + (IGHV7-81 × 0.15661608).
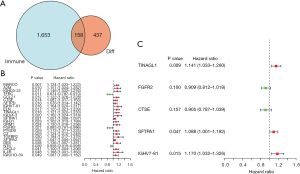
The patients with LUSC were divided into high- or low-risk groups according to the median risk score. Kaplan-Meier analysis indicated that the survival time of patients with a low-risk score was significantly longer than that of high-risk patients, P<0.001 (Figure 6A). Meanwhile, the area under the ROC curve (AUC) was calculated, as displayed in Figure S2, the AUC value was 0.658, indicating that the risk score plays an important role in prognostic evaluation. The calibration chart showed that the curve and calibration line were almost combined, indicating that the model fit well (Figure 6B). Additionally, heat maps suggested that TINAGL1, CTSE, SFTPA1, and IGHV7-81 were lowly expressed in the low-risk groups, while FGFR2 was highly expressed in the low-risk groups (Figure 7A). The risk curve showed that mortality increased with the increase of the risk score (Figure 7B,7C). A nomogram was also used to evaluate the effect of gene expression levels on the 1-, 3-, and 5-year survival rates. The results suggested that the total score of the nomogram helped provide a quantitative method for predicting the prognosis of LUSC (Figure 7D).

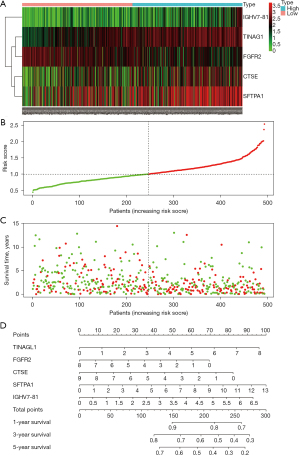
The GSE37745 dataset as an external validation showed that there was a significant difference in the survival rate between the high and low risk groups (P=0.012), and the AUC value of the area under the ROC curve was 0.644, indicating that the model had certain predictive value (Figure 8, Figure S3A). In addition, ROC analysis was performed on clinical indicators of LUSC, such as age, stage, T, N, M, etc., together with the risk score. Compared with all clinical indicators, the AUC value of risk score was the largest, indicating that the prognostic model had certain predictive value (Figure S3B).
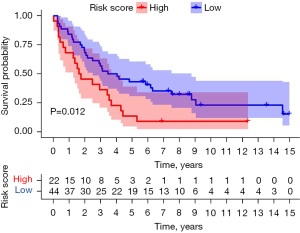
To verify whether the risk score can be used as an independent prognostic factor for patients with LUSC, univariate and multivariate Cox analyses were performed on the clinical variables and risk scores. Univariate analysis indicated that tumor, metastasis, lymph node metastasis, and risk score were prognostic factors (Figure 9A). Meanwhile, multivariate analysis indicated that tumor, metastasis, lymph node metastasis, and risk score can be used as independent prognostic factors (Figure 9B).
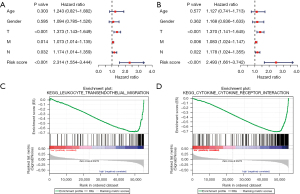
Finally, to determine the unknown function of the prognostic model, we performed Gene Set Enrichment Analysis (GSEA) to identify the possible biological function of the prognostic model. As displayed in Figure 9C,9D, these genes are closely associated with the leukocyte transendothelial migration, cytokine-cytokine receptor interaction, and other pathways, and these related biological pathways can significantly affect the tumorigenesis of LUSC.
Analysis of prognostic hub genes
To further evaluate the reliability and effectiveness of this prognostic risk model, we performed bioinformatics analyses on the hub prognostic genes (TINAGL1, FGFR2, CTSE, SFTPA1, and IGHV7-81). As presented in Figure 10A, the expression of FGFR2 was markedly decreased in the low TMB group compared to the high TMB group, while the levels of TINAGL1, CTSE, SFTPA1, and IGHV7-81 were significantly increased in the low TMB group. Importantly, patients with high TINAGL1, CTSE, SFTPA1, and IGHV7-81 have a shorter survival time, while patients with high FGFR2 have a longer survival time (Figure 10B-10F).
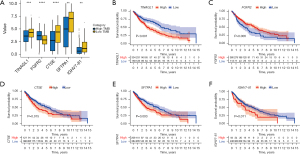
Next, we used the CIBERSORT algorithm to evaluate the relationship between gene mutation and tumor-infiltrating immune cells in the LUSC microenvironment. As a result, there were significant differences in the composition of 22 immune cell types in each sample (Figure 11A). The result obtained from the correlation matrix is shown in Figure 11B. Then, the correlation between hub genes expression and immune cells was verified, and immune cells with a correlation coefficient >0.15 and P<0.05 were shown in Figure S4. Additionally, the somatic copy-number alterations (sCNA) module in timer2.0 was used to compare the distribution of immune infiltration through the sCNA status of genes in TCGA cancer types. Figure 12A-12D shows the relative scale of the sCNA status of CTSE, FGFR2, SFTPA1, and TINAGL1 in all TCGA cancer types.

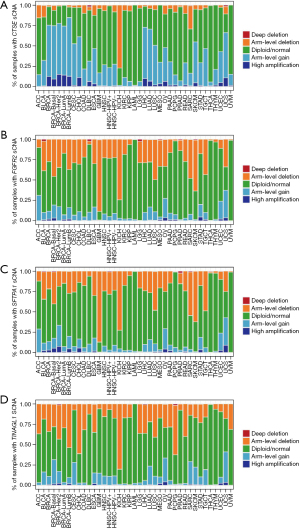
Discussion
It is well known that LUSC has high heterogeneity and extensive mutations, and the treatment is particularly challenging. Due to limited treatment strategies, the prognosis of LUSC is poor. In recent years, the treatment of LUSC has changed significantly with the introduction of ICIS-based immunotherapy, but only a small number of patients have responded to this therapy. Because of the different therapeutic responses, it is urgent to identify immune biomarkers with higher sensitivity and accuracy to predict the effect of immunotherapy and the prognosis of LUSC patients.
Currently, several studies have constructed immune-related molecular models to predict the prognosis of patients with LUSC. Yan et al. and Fu et al. constructed prognostic models of different immune genes based on the ImmPort database and identified new prognostic markers of LUSC (27,28). Yang et al. divided tumors into hot tumors and cold tumors according to a combination of immunodilation and PD-L1 expression, and developed a prediction model of 13 immune-related genes based on different expression genes between the two, which could be used for prognostic prediction of LUSC and immunotherapy response (29). Yan et al. developed a three gene risk model using TMB-associated genes to predict LUSC patient survival, suggesting that TMB might contribute to the pathogenesis of LUSC (30). All of the above studies have their own characteristics and new findings, however, there is no consensus on biomarkers that can predict the prognosis of LUSC patients. Given the complexity of tumor biology and immune microenvironments, single biomarkers may not predict the clinical outcomes of immunotherapy.
Compared with previous studies, our study combined TMB and immune genes for the first time, and constructed an immune related gene prognosis model based on TMB to predict the prognosis of LUSC patients, which is more comprehensive and convincing, and can better reflect the TMB and immune-related characteristics. Numerous evidences have shown that TMB is related to immunotherapy response. It is a novel biomarker used to predict the benefit of tumor immune checkpoint inhibitor treatment. To our knowledge, cells with a high TMB are easily recognized by the immune system and become targets of tumor immunity (31). Hence, immunotherapy is more effective and the survival rate is higher in patients with a high TMB (32,33).
In the present study, we constructed a prognostic model based on immune and TMB-related genes to predict the prognosis of LUSC patients. A total of 595 TMB-related DEGs were identified based on TCGA database, which were then intersected with immune genes downloaded from Immport, and 156 immune-related DEGs were identified. Through univariate and multivariate cox regression analyses, five hub prognostic genes (TINAGL1, FGFR2, CTSE, SFTPA1, and IGHV7-81) were screened to establish a prognostic model. As expected, the prognostic model is stable and reliable, and the risk score can be used as an independent prognostic factor for LUSC patients.
Our results are consistent with the findings of previous studies; the survival rate of patients with a high TMB is significantly higher than that of patients with a low TMB. KEGG analysis indicated that DEGs were mainly involved in Th1, Th2 and Th17 cell differentiation, and cytokine-cytokine receptor interaction. GO enrichment analysis indicated that they were mainly involved in B cell/lymphocyte mediated immunity, leukocyte/lymphocyte/B cell/macrophage/T cell activation, lymphocyte/T cell/mononuclear cell/leukocyte proliferation. These genes may be closely related to the therapeutic response of ICIs. The proliferation, activation, and differentiation of lymphocytes are essential to the immune system (34). Many studies have shown that TMB is a predictive biomarker for response to ICIs (25). Our study supports the view that TMB is closely related to immunity.
Furthermore, we conducted survival analysis on the five hub prognostic genes related to the TMB, and the results showed that the high expression genes in patients with a low TMB (TINAGL1, CTSE, SFTPA1, and IGHV7-81) are associated with poor prognosis. This indicated that TMB levels are closely related to the prognosis of patients with LUSC, and these five TMB-related genes possess significant prognostic value.
Next, the prognostic model was established using these five hub immune genes. We calculated the risk score based on the hub genes and divided the samples into low- and high-risk groups. A series of bioinformatics analyses were subsequently performed to verify the model. The results showed that high-risk patients had worse survival outcomes compared with low-risk patients, and the risk score is a valuable biomarker for the prognosis of patients with LUSC. Hence, we believe that the use of the TINAGL1, FGFR2, CTSE, SFTPA1, and IGHV7-81 prognostic model to predict the prognosis of LUSC patients is feasible and stable.
FGFR2, a fibroblast growth factor receptor, is overexpressed in breast cancer, gastric cancer, and LUSC (35-37), and the recurrent mutation of FGFR2 has been confirmed in LUSC (38). Tubulointerstitial nephritis antigen-like 1 (TINAGL1), a secretory extracellular protein, promotes cell adhesion and migration by binding to integrins such as ITGA1B1. The abnormal expression of TINAGL1 has been considered a prognostic factor in several cancers, such as triple-negative breast cancer (39) and hepatocellular carcinoma (40). Umeyama et al. found that TINAGL1 is a metastasis-promoting factor in NSCLC. Cathepsin E (CTSE), an intracellular aspartic protease, is expressed in both the immune system and cancer cells (41). CTSE is highly expressed and hypomethylated in LUAD and can be used as the hub gene in the prognostic model of LUAD patients (42,43). SFTPA1 encodes human pulmonary surfactant protein A (SP-A), which plays an important role in lung homeostasis and immunity (44), and is considered a promising molecular marker of NSCLC tumor cells (45). However, its prognostic value in LUSC has not been studied. IGHV7-81 is a kind of mutated gene and PDCD1LG2-IGHV7-81 fusion is involved in reducing T-cell activation (46,47), but its function in LUSC has not been reported. Our data suggested for the first time that ITGA1B1, CTSE, SFTPA1, and IGHV7-81 have prognostic value in LUSC and are associated with the TMB and immune response.
To our knowledge, this is the first TMB-related prognostic model that can predict survival in LUSC. We calculated the risk score and divided the patients into high- and low-risk groups. High-risk patients had worse survival outcomes compared with low-risk patients, and the risk score is a valuable biomarker for the prognosis of patients with LUSC. However, there are some limitations in this study that should be noted and considered. Firstly, our prognostic model needs to be further validated in large-scale and prospective studies. Also, the molecular mechanism and function of the five hub immune genes still need further study.
Conclusions
In summary, we screened five hub immune genes and established a prognostic model related to the TMB and immunity. The prognostic model is reliable and stable and can be used as a prognostic biomarker for LUSC patients, which provides a theoretical basis for the clinical immunotherapy of LUSC.
Acknowledgments
Funding: None.
Footnote
Reporting Checklist: The authors have completed the TRIPOD reporting checklist. Available at https://jtd.amegroups.com/article/view/10.21037/jtd-23-103/rc
Peer Review File: Available at https://jtd.amegroups.com/article/view/10.21037/jtd-23-103/prf
Conflicts of Interest: All authors have completed the ICMJE uniform disclosure form (available at https://jtd.amegroups.com/article/view/10.21037/jtd-23-103/coif). The authors have no conflicts of interest to declare.
Ethical Statement: The authors are accountable for all aspects of the work, including ensuring that any questions related to the accuracy or integrity of any part of the work have been appropriately investigated and resolved. The study was conducted in accordance with the Declaration of Helsinki (as revised in 2013).
Open Access Statement: This is an Open Access article distributed in accordance with the Creative Commons Attribution-NonCommercial-NoDerivs 4.0 International License (CC BY-NC-ND 4.0), which permits the non-commercial replication and distribution of the article with the strict proviso that no changes or edits are made and the original work is properly cited (including links to both the formal publication through the relevant DOI and the license). See: https://creativecommons.org/licenses/by-nc-nd/4.0/.
References
- Nasim F, Sabath BF, Eapen GA. Lung Cancer. Med Clin North Am 2019;103:463-73. [Crossref] [PubMed]
- Rodriguez-Canales J, Parra-Cuentas E, Wistuba II. Diagnosis and Molecular Classification of Lung Cancer. Cancer Treat Res 2016;170:25-46. [Crossref] [PubMed]
- Dela Cruz CS, Tanoue LT, Matthay RA. Lung cancer: epidemiology, etiology, and prevention. Clin Chest Med 2011;32:605-44. [Crossref] [PubMed]
- Qi L, Gao C, Feng F, et al. MicroRNAs associated with lung squamous cell carcinoma: New prognostic biomarkers and therapeutic targets. J Cell Biochem 2019;120:18956-66. [Crossref] [PubMed]
- Kuribayashi K, Funaguchi N, Nakano T. Chemotherapy for advanced non-small cell lung cancer with a focus on squamous cell carcinoma. J Cancer Res Ther 2016;12:528-34. [Crossref] [PubMed]
- Hirsch FR, Scagliotti GV, Mulshine JL, et al. Lung cancer: current therapies and new targeted treatments. Lancet 2017;389:299-311. [Crossref] [PubMed]
- Kulasingam V, Diamandis EP. Strategies for discovering novel cancer biomarkers through utilization of emerging technologies. Nat Clin Pract Oncol 2008;5:588-99. [Crossref] [PubMed]
- Lazarus KA, Hadi F, Zambon E, et al. BCL11A interacts with SOX2 to control the expression of epigenetic regulators in lung squamous carcinoma. Nat Commun 2018;9:3327. [Crossref] [PubMed]
- Yang Y. Cancer immunotherapy: harnessing the immune system to battle cancer. J Clin Invest 2015;125:3335-7. [Crossref] [PubMed]
- Ladoire S, Rébé C, Ghiringhelli F. Associating immunotherapy and targeted therapies: facts and hopes. Clin Cancer Res 2022;CCR-22-1184. [Crossref] [PubMed]
- Li B, Chan HL, Chen P. Immune Checkpoint Inhibitors: Basics and Challenges. Curr Med Chem 2019;26:3009-25. [Crossref] [PubMed]
- Dafni U, Tsourti Z, Vervita K, et al. Immune checkpoint inhibitors, alone or in combination with chemotherapy, as first-line treatment for advanced non-small cell lung cancer. A systematic review and network meta-analysis. Lung Cancer 2019;134:127-40. [Crossref] [PubMed]
- Mourah S, Louveau B, Dumaz N. Mechanisms of resistance and predictive biomarkers of response to targeted therapies and immunotherapies in metastatic melanoma. Curr Opin Oncol 2020;32:91-7. [Crossref] [PubMed]
- Xie F, Xu M, Lu J, et al. The role of exosomal PD-L1 in tumor progression and immunotherapy. Mol Cancer 2019;18:146. [Crossref] [PubMed]
- Ma J, Zeng X, Zhang B. Emerging biomarker for immunotherapy of non-small cell lung cancer: tumor mutation burden (TMB) and latest progress in related research field. Zhonghua Bing Li Xue Za Zhi 2019;48:987-92. [PubMed]
- Chan TA, Yarchoan M, Jaffee E, et al. Development of tumor mutation burden as an immunotherapy biomarker: utility for the oncology clinic. Ann Oncol 2019;30:44-56. [Crossref] [PubMed]
- Schumacher TN, Schreiber RD. Neoantigens in cancer immunotherapy. Science 2015;348:69-74. [Crossref] [PubMed]
- Hodi FS, Wolchok JD, Schadendorf D, et al. TMB and Inflammatory Gene Expression Associated with Clinical Outcomes following Immunotherapy in Advanced Melanoma. Cancer Immunol Res 2021;9:1202-13. [Crossref] [PubMed]
- McGranahan N, Furness AJ, Rosenthal R, et al. Clonal neoantigens elicit T cell immunoreactivity and sensitivity to immune checkpoint blockade. Science 2016;351:1463-9. [Crossref] [PubMed]
- Chen DS, Mellman I. Elements of cancer immunity and the cancer-immune set point. Nature 2017;541:321-30. [Crossref] [PubMed]
- Rizvi NA, Hellmann MD, Snyder A, et al. Cancer immunology. Mutational landscape determines sensitivity to PD-1 blockade in non-small cell lung cancer. Science 2015;348:124-8. [Crossref] [PubMed]
- Snyder A, Makarov V, Merghoub T, et al. Genetic basis for clinical response to CTLA-4 blockade in melanoma. N Engl J Med 2014;371:2189-99. [Crossref] [PubMed]
- Carbone DP, Reck M, Paz-Ares L, et al. First-Line Nivolumab in Stage IV or Recurrent Non-Small-Cell Lung Cancer. N Engl J Med 2017;376:2415-26. [Crossref] [PubMed]
- Hellmann MD, Ciuleanu TE, Pluzanski A, et al. Nivolumab plus Ipilimumab in Lung Cancer with a High Tumor Mutational Burden. N Engl J Med 2018;378:2093-104. [Crossref] [PubMed]
- Klempner SJ, Fabrizio D, Bane S, et al. Tumor Mutational Burden as a Predictive Biomarker for Response to Immune Checkpoint Inhibitors: A Review of Current Evidence. Oncologist 2020;25:e147-59. [Crossref] [PubMed]
- Yu G, Wang LG, Han Y, et al. clusterProfiler: an R package for comparing biological themes among gene clusters. OMICS 2012;16:284-7. [Crossref] [PubMed]
- Yan Y, Zhang M, Xu S, et al. Identification of an Immune Gene Expression Signature for Predicting Lung Squamous Cell Carcinoma Prognosis. Biomed Res Int 2020;2020:5024942. [Crossref] [PubMed]
- Fu D, Zhang B, Yang L, et al. Development of an Immune-Related Risk Signature for Predicting Prognosis in Lung Squamous Cell Carcinoma. Front Genet 2020;11:978. [Crossref] [PubMed]
- Yang Q, Gong H, Liu J, et al. A 13-gene signature to predict the prognosis and immunotherapy responses of lung squamous cell carcinoma. Sci Rep 2022;12:13646. [Crossref] [PubMed]
- Yan D, Chen Y. Tumor mutation burden (TMB)-associated signature constructed to predict survival of lung squamous cell carcinoma patients. Sci Rep 2021;11:9020. [Crossref] [PubMed]
- Goodman AM, Kato S, Bazhenova L, et al. Tumor Mutational Burden as an Independent Predictor of Response to Immunotherapy in Diverse Cancers. Mol Cancer Ther 2017;16:2598-608. [Crossref] [PubMed]
- Hellmann MD, Nathanson T, Rizvi H, et al. Genomic Features of Response to Combination Immunotherapy in Patients with Advanced Non-Small-Cell Lung Cancer. Cancer Cell 2018;33:843-852.e4. [Crossref] [PubMed]
- Duffy MJ, Crown J. Biomarkers for Predicting Response to Immunotherapy with Immune Checkpoint Inhibitors in Cancer Patients. Clin Chem 2019;65:1228-38. [Crossref] [PubMed]
- Heinzel S, Marchingo JM, Horton MB, et al. The regulation of lymphocyte activation and proliferation. Curr Opin Immunol 2018;51:32-8. [Crossref] [PubMed]
- Turner N, Pearson A, Sharpe R, et al. FGFR1 amplification drives endocrine therapy resistance and is a therapeutic target in breast cancer. Cancer Res 2010;70:2085-94. [Crossref] [PubMed]
- Matsumoto K, Arao T, Hamaguchi T, et al. FGFR2 gene amplification and clinicopathological features in gastric cancer. Br J Cancer 2012;106:727-32. [Crossref] [PubMed]
- Hibi M, Kaneda H, Tanizaki J, et al. FGFR gene alterations in lung squamous cell carcinoma are potential targets for the multikinase inhibitor nintedanib. Cancer Sci 2016;107:1667-76. [Crossref] [PubMed]
- Testa U, Castelli G, Pelosi E. Lung Cancers: Molecular Characterization, Clonal Heterogeneity and Evolution, and Cancer Stem Cells. Cancers (Basel) 2018;10:248. [Crossref] [PubMed]
- Shen M, Jiang YZ, Wei Y, et al. Tinagl1 Suppresses Triple-Negative Breast Cancer Progression and Metastasis by Simultaneously Inhibiting Integrin/FAK and EGFR Signaling. Cancer Cell 2019;35:64-80.e7. [Crossref] [PubMed]
- Sun L, Dong Z, Gu H, et al. TINAGL1 promotes hepatocellular carcinogenesis through the activation of TGF-β signaling-medicated VEGF expression. Cancer Manag Res 2019;11:767-75. [Crossref] [PubMed]
- Umeyama H, Iwadate M, Taguchi YH. TINAGL1 and B3GALNT1 are potential therapy target genes to suppress metastasis in non-small cell lung cancer. BMC Genomics 2014;15:S2. [Crossref] [PubMed]
- Kuo IY, Jen J, Hsu LH, et al. A prognostic predictor panel with DNA methylation biomarkers for early-stage lung adenocarcinoma in Asian and Caucasian populations. J Biomed Sci 2016;23:58. [Crossref] [PubMed]
- Yang Y, Wang M, Liu B. Exploring and comparing of the gene expression and methylation differences between lung adenocarcinoma and squamous cell carcinoma. J Cell Physiol 2019;234:4454-9. [Crossref] [PubMed]
- Grageda M, Silveyra P, Thomas NJ, et al. DNA methylation profile and expression of surfactant protein A2 gene in lung cancer. Exp Lung Res 2015;41:93-102. [Crossref] [PubMed]
- Nordgård O, Singh G, Solberg S, et al. Novel molecular tumor cell markers in regional lymph nodes and blood samples from patients undergoing surgery for non-small cell lung cancer. PLoS One 2013;8:e62153. [Crossref] [PubMed]
- Chong LC, Twa DD, Mottok A, et al. Comprehensive characterization of programmed death ligand structural rearrangements in B-cell non-Hodgkin lymphomas. Blood 2016;128:1206-13. [Crossref] [PubMed]
- Scinicariello F, Jayashankar L, Attanasio R. Baboon immunoglobulin variable region heavy chains: identification of genes homologous to members of the human IGHV1-IGHV7 subgroups. Immunogenetics 2002;53:815-20. [Crossref] [PubMed]
(English Language Editor: A. Kassem)