A novel pyroptosis-related model for prognostic prediction in esophageal squamous cell carcinoma: a bioinformatics analysis
Highlight box
Key findings
• Three prognosis pyroptosis-related genes, AADAC, GSTA1, and KCNS3, were investigated in ESCC.
• The high-risk and low-risk groups distinguished by AADAC, GSTA1, and KCNS3 showed significant differences in T staging and N staging.
• These 2 groups also showed obvious discrepancies in immune infiltration cell score and immune checkpoint molecules.
What is known and what is new?
• Studies assessing the role of pyroptosis in cardiovascular, neurological, and immune systems, as well as cancer are being increasing conducted.
• Our study identified genes correlated with pyroptosis of ESCC and established a prognostic risk model.
What is the implication, and what should change now?
• The prognostic pyroptosis-related biomarkers may interact with the tumor immune microenvironment to regulate ESCC, and further study needs to be conducted to refine the immunotherapy for this disease.
Introduction
Esophageal squamous cell carcinoma (ESCC), a subtype of esophageal cancer (EC), is highly prevalent, lacks effective treatments, and has an extremely poor prognosis (1). ESCC is mainly found in Eastern to Central Asia, with these regions accounting for almost 90% of all EC cases each year. In China, ESCC ranks sixth in terms of morbidity and mortality among all types of cancers (2). Primary treatments for patients with ESCC include a combination of surgery, radiotherapy, chemotherapy, and immunotherapy based on disease staging (3). Unfortunately, a large proportion of patients with ESCC are diagnosed with advanced disease due to the lack of reliable screening methods, leading to low 5-year survival rates (4). Therefore, there is an urgent need for biomarkers and viable models to predict the prognosis of ESCC and guide targeted therapy.
The incidence of ESCC varies significantly and is not fully understood in terms of the known mechanisms and environmental risk factors (5). To better understand the molecular characteristics of ESCC, a growing number of contributing factors are being investigated. Hanahan explored 10 hallmarks to obtain a more comprehensive understanding of cancer development and malignancy. This investigation expanded to 14 hallmarks, including “unlocking phenotypic plasticity”, “non-mutational epigenetic reprogramming”, “polymorphic microbiomes”, and “senescent cells” (6). In recent years, there has been an increasing focus on studying the mutations of genes related to ESCC patients’ overall survival (OS) and progression-free survival (PFS), as researchers search for biomarkers or risk models for the disease. Lian et al. explored the survival rate of the high-risk samples distinguished by 8 genes (FABP7, TLR1, SYTL1, APLN, OSM, EGFR, IL17RD, MYH9) was significantly lower than that of low-risk samples (7).Additionally, Pu et al. generated an independent prognostic signature based on the expression of YTHDF3, RBM15, KIAA1429, and ALKBH5 genes and their overexpression predicted better OS of ESCC patients (8). However, most studies were single-center retrospective research which the number of patients was relatively small. On the other hand, the short follow-ups may not reach the median survival time. So, we need a large cohort and longer follow-up for further validation to find effective biomarkers for the diagnosis, treatment, and prognosis of ESCC patients.
Cell death is now recognized not only as a stress response but also as a physiological regulator of proliferation and homeostasis. Additionally, it serves as a mechanism for inhibiting cancer. Pyroptosis, triggered by caspase 4, 5, or 11, is a type of programmed cell death that is both proinflammatory and inflammatory (9). An increasing number of studies have been published that examine the role of pyroptosis in cardiovascular, neurological, immune system, and carcinoma research (10). Pyroptosis has a significant dual role in tumorigenesis and antitumor resistance, promoting or suppressing cancer depending on the circumstances, primarily determined by growth type, inflammatory and immune status, and other factors. The dual impact of pyroptosis is believed to have opposing effects on tumor growth. Prolonged and persistent injury may accelerate tumor growth as the exacerbation caused by pyroptosis is amplified by age and an inflammatory microenvironment surrounding the diseased cells. However, on the other hand, the strong impact of pyroptosis triggers the activation of various immune cells to suppress cancer growth (11). In summary, we are urgently seeking a series of biomarkers for ESCC to guide our understanding of its development and associated immune effects. This series of biomarkers can also help us find a new treatment method or combine with relevant immunotherapy to achieve more precise treatment of ESCC patients.
Despite the recent progress in ESCC research, the exact role of pyroptosis in malignant growth remains unclear, and its association with ESCC has not been extensively studied. Therefore, our aim was to identify the genes correlated with pyroptosis and ESCC, then develop a prognostic risk model. In this study, we investigated various pyroptosis-inducing mechanisms and related molecules in ESCC. Our findings can provide valuable insights for the development of therapies for ESCC. We present the following article in accordance with the TRIPOD reporting checklist (available at https://jtd.amegroups.com/article/view/10.21037/jtd-23-206/rc).
Methods
Data source
The RNA-seq data of 75 patients with ESCC who had the relevant clinical and survival information were obtained from The Cancer Genome Atlas (TCGA) (https://portal.gdc.cancer.gov/). The clinical data included gender, age, tumor-node-metastasis (TNM) stage, and overall survival (OS). The “Limma” package in R was used to clarify the database background and normalize raw RNA-seq data. The downstream analyses were conducted using RPKM values.
Weighted gene coexpression network analysis
Gene set enrichment analysis (GSEA) was used to calculate the pyroptosis-related pathway score (Pys) using the gene set of the pyroptosis pathway. Outliers were first detected and excluded by sample clustering. Weighted gene coexpression network analysis (WGCNA) was carried out using gene expression data and the selected traits (TNM stages and Pys) of ESCC samples. Briefly, the optimal β (soft threshold) was identified using the pickSoftThreshold function, which was followed by the establishment of a network by converting the adjacent matrix to a topological overlap matrix and the determination of a gene dendrogram and module color. After the clustering and merging similar modules, the modules most correlated with TNM stages and Pys were identified with Pearson correlation analysis.
Development of a Pys-related risk score
Univariate Cox regression was first carried out to obtain Pys-related genes (PRGs) associated with ESCC prognosis with P<0.05 being used as the cutoff. Next, least absolute shrinkage and selection operator (LASSO) regression was applied to further screen the prognostic signature, and following formula was used to calculate the risk score:
in which x is the screened prognostic signature. We then classified patients with ESCC into 2 risk groups according to median risk score. Kaplan-Meier curves were plotted to analyze and compare the overall survival of the 2 risk groups.
Gene set variation analysis
We used the “GSVA” and “Limma” R packages (The R Foundation of Statistical Computing) to score and classify the Kyoto Encyclopedia of Genes and Genomes (KEGG) pathways into 2 risk groups and identified the significantly differentially enriched pathways between the 2 risk groups using P<0.05 as the cutoff.
Identification of differentially infiltrated immune cells between the two risk groups
We used the “CIBERSORT” (12) R package to evaluate the levels of 22 immune cells in the 2 risk groups. The Student t-test was applied to investigate whether there was a significant difference of immune cells between the 2 risk groups using P<0.05 as the cutoff.
Results
Identification of the PRGs in ESCC
WGCNA subsequently screened the relationship between tumor staging and the pyroptosis-related genes. First, 4 outliers were screened out by calculating the Euclidean distance (Figure 1A) and were not included in further analysis. The soft threshold was set to 12 to build a scale-free network with an R2 of approximately 0.9 (Figure 1B). Finally, 3 modules were identified (Figure 1C). The module-trait heatmap showed that the pink module was most correlated with Pys [correlation (cor) =0.34; P=0.003] and N staging (cor =−0.54; P<0.001) (Figure 1D). Therefore, 283 genes in the pink module were chosen for downstream analyses.
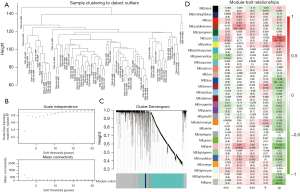
Identification of prognostic PRGs in ESCC
The 283 PRGs from the pink model were then input into univariate Cox regression, through which 85 PRGs significantly associated with ESCC prognosis (P<0.05; Table 1). To acquire more robust signature genes, 85 PRGs were further analyzed with LASSO. Three pyroptosis-related genes, including arylacetamide deacetylase (AADAC), GSTA1, and KCNS3 were identified (Figure 2A), and their coefficients are shown in Figure 2B.
Table 1
Genes | OR | 95% CI | P |
---|---|---|---|
GSTA1 | 1.43 | 1.16–1.77 | 9.00E-04 |
ABCC5 | 1.92 | 1.27–2.9 | 0.0019 |
AADAC | 1.85 | 1.23–2.81 | 0.0034 |
DSE | 0.3 | 0.13–0.68 | 0.004 |
KCNS3 | 2 | 1.24–3.24 | 0.0047 |
TMEM14A | 2.45 | 1.31–4.59 | 0.0051 |
UGT1A6 | 1.82 | 1.18–2.81 | 0.0067 |
ALDH1A1 | 1.33 | 1.08–1.64 | 0.007 |
PRDX1 | 2.41 | 1.26–4.6 | 0.0077 |
TIGD1 | 3.83 | 1.41–10.41 | 0.0084 |
GSTM4 | 1.56 | 1.12–2.18 | 0.0087 |
PLEKHF1 | 0.4 | 0.21–0.8 | 0.009 |
ALDH3A2 | 2.11 | 1.2–3.7 | 0.0093 |
NQO1 | 1.55 | 1.11–2.16 | 0.0098 |
CBX2 | 2.03 | 1.18–3.49 | 0.0104 |
SLC48A1 | 2.78 | 1.27–6.1 | 0.0107 |
CORO2A | 2.34 | 1.21–4.5 | 0.0111 |
C6orf141 | 0.52 | 0.32–0.87 | 0.0117 |
GPX2 | 1.31 | 1.06–1.63 | 0.0119 |
CILK1 | 2.06 | 1.17–3.61 | 0.012 |
EHD1 | 0.26 | 0.09–0.74 | 0.0121 |
PCARE | 410.73 | 3.57–47,282.85 | 0.0129 |
IGSF11 | 2.52 | 1.21–5.24 | 0.0138 |
TMEM183A | 5.67 | 1.41–22.87 | 0.0146 |
AKR1C2 | 1.29 | 1.05–1.57 | 0.0147 |
AGPAT4 | 0.3 | 0.11–0.8 | 0.0158 |
PANX2 | 1.52 | 1.08–2.15 | 0.016 |
EPHX1 | 1.61 | 1.09–2.36 | 0.0163 |
MTARC1 | 2.65 | 1.19–5.92 | 0.0175 |
UBE2Q1 | 5.03 | 1.31–19.34 | 0.0189 |
CLDN8 | 1.5 | 1.07–2.1 | 0.019 |
ALDH5A1 | 1.96 | 1.11–3.44 | 0.0194 |
ZDHHC9 | 3.31 | 1.21–9.05 | 0.02 |
CES1 | 1.19 | 1.03–1.39 | 0.0207 |
PGD | 1.52 | 1.06–2.17 | 0.0216 |
ADSS2 | 3.55 | 1.2–10.48 | 0.0217 |
OSGIN1 | 1.43 | 1.05–1.96 | 0.0244 |
ETFB | 2.32 | 1.11–4.85 | 0.0253 |
MRAP2 | 1.53 | 1.05–2.24 | 0.0261 |
GCLC | 1.58 | 1.05–2.36 | 0.0265 |
ARHGAP17 | 0.15 | 0.03–0.8 | 0.0266 |
KIAA0319 | 2.02 | 1.08–3.75 | 0.0266 |
SLC27A2 | 1.73 | 1.07–2.81 | 0.0266 |
MDGA1 | 1.71 | 1.06–2.75 | 0.0274 |
SELENOI | 2.49 | 1.1–5.62 | 0.0279 |
AKR1C3 | 1.24 | 1.02–1.5 | 0.0282 |
ANXA10 | 1.44 | 1.04–2 | 0.0297 |
AKR1C1 | 1.23 | 1.02–1.48 | 0.0299 |
ABHD4 | 1.85 | 1.06–3.22 | 0.0304 |
PTGR1 | 1.5 | 1.04–2.16 | 0.0309 |
KLHL31 | 9.99 | 1.23–80.9 | 0.0311 |
ETNK2 | 1.73 | 1.05–2.85 | 0.0319 |
TMEM116 | 1.69 | 1.04–2.75 | 0.0326 |
TXNRD1 | 1.42 | 1.03–1.96 | 0.0331 |
TDP2 | 2.22 | 1.06–4.62 | 0.0336 |
CCDC190 | 1.32 | 1.02–1.7 | 0.0339 |
GCLM | 1.38 | 1.02–1.86 | 0.0343 |
UST | 2.19 | 1.06–4.52 | 0.0348 |
PPP1R3B | 0.54 | 0.3–0.96 | 0.0355 |
MAGEF1 | 1.96 | 1.04–3.66 | 0.036 |
MPP3 | 2.48 | 1.06–5.85 | 0.0372 |
TFB2M | 2.69 | 1.06–6.83 | 0.0374 |
FECH | 1.91 | 1.04–3.51 | 0.0375 |
MARCHF3 | 3.14 | 1.07–9.26 | 0.0377 |
G6PD | 1.46 | 1.02–2.09 | 0.0396 |
FLVCR1 | 2.24 | 1.04–4.84 | 0.0406 |
ME1 | 1.49 | 1.02–2.17 | 0.0406 |
RAB3B | 1.57 | 1.02–2.43 | 0.0415 |
MCCC1 | 1.95 | 1.02–3.7 | 0.0419 |
CBR1 | 1.47 | 1.01–2.14 | 0.0425 |
CYP26A1 | 1.42 | 1.01–2 | 0.044 |
TSPAN7 | 1.33 | 1.01–1.75 | 0.0445 |
PLAAT2 | 1.72 | 1.01–2.91 | 0.0448 |
LYN | 1.91 | 1.01–3.59 | 0.0457 |
CYP4F11 | 1.22 | 1–1.48 | 0.0462 |
COG2 | 3.74 | 1.02–13.7 | 0.0464 |
LRP8 | 1.93 | 1.01–3.69 | 0.0464 |
GSTM2 | 1.39 | 1–1.91 | 0.0466 |
COA6 | 1.64 | 1.01–2.67 | 0.0471 |
PYCR2 | 1.89 | 1.01–3.54 | 0.0471 |
NFE2L2 | 1.84 | 1.01–3.35 | 0.0477 |
ADH7 | 1.23 | 1–1.51 | 0.0479 |
NMNAT3 | 1.98 | 1.01–3.89 | 0.0479 |
ABHD2 | 1.72 | 1–2.94 | 0.0485 |
TKT | 1.66 | 1–2.74 | 0.0494 |
OR, odds ratio; CI, confidence interval.
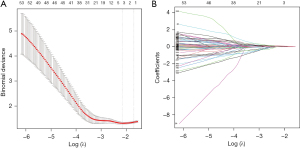
According to their expressions and coefficients, we calculated the risk scores of patients with ESCC using the following formula: expression (AADAC) × coefficient (AADAC) + expression (GSTA1) × coefficient (GSTA1) + expression (KCNS3) × coefficient (KCNS3). We then classified patients with ESCC into 2 (high and low) risk groups (Figure 3A). We observed that more patients died when their risk score was higher (Figure 3B). Moreover, compared to those in the high-risk group, the tumor stage and TNM of patients in the low-risk group were significantly low (Figure 4).
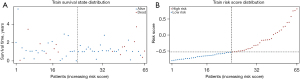
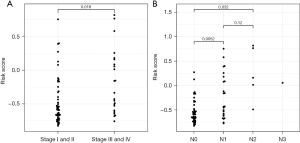
GSVA and CIBERSORT revealed cell proliferation and immune infiltration-related mechanisms in ESCC
To investigate the association of the risk score built using AADAC, GSTA1, and KCNS3 with ESCC prognosis, the KEGG pathways enriched in the 2 risk groups were analyzed with GSVA. We found the top 30 differentially enriched KEGG pathways, including fructose catabolism pathway, fructose metabolism pathway, sterol esterase activity, xenobiotic glucuronidation pathway, and fructose catabolic process pathway (Figure 5A), indicating that the prognostic pyroptosis-related biomarkers may regulate proliferation of ESCC cells. In addition, we evaluated the levels of multiple immune cells in the 2 risk groups using CIBERSORT and found that the infiltration of CD4+ T cells, keratinocytes cells, monocytes cells, mesenchymal stem cells, nerve cells, natural killer T cells, activated mast cells, and neutrophils were greatly varied between 2 groups (Figure 5B), indicating that prognostic pyroptosis-related biomarkers may interact with the tumor immune microenvironment to regulate ESCC.
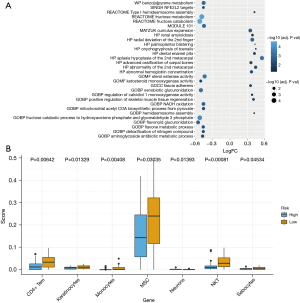
Discussion
ESCC, the dominant pathological type of EC, is the fourth main cause of cancer-associated death in China. Despite advancements in ESCC-related diagnosis and treatment, the prognosis of patients with ESCC is still low, with a 5-year survival rate of less than 30%. This is due to the lack of ESCC-related hallmarks and corresponding target treatment, and although several biomarkers for ESCC have been explored, their clinical and therapeutic value has not been clearly determined. We thus aimed to identify and evaluate the biomarkers associated with the target treatment of ESCC. In our research, we came to learn that cell death acts as a critical barrier against the development ESCC, with pyroptosis being one of the major types of programmed cell death (13). Thus, searching for valid and significant biomarkers for ESCC pyroptosis is a crucial step in developing treatment.
Research into the relationship of pyroptosis with ESCC is rare, but studies on this subject are gradually emerging. By comprehensive analyzing 857 patients’ transcriptomes and 124 patients’ proteomic profiles, Zhang and Chen discovered that the pathway of necroptosis, ferroptosis, and pyroptosis is closely linked with the activation of immunity in ESCC (14). Zheng et al. found that a high level of STAT3β expression resulted in promoting the sensitivity of cisplatin and strengthening the reliant pyroptosis of gasdermin E (GSDME) in ESCC cells after exposuring to cisplatin (15). They further reported alpinumisoflavone (AIF) could trigger GSDME-dependent pyroptosis in ESCC via caspase-3 activation (16). Furthermore, Jiang et al. found that large bubbles grow in the cell membrane, which is followed by the low expression of PKM2, the stimulation of caspase-3/8, and the generation of GSDME-NT, ultimately leading to the pyroptosis in ESCC (17). Wu et al. found that BI2536, a PLK1 kinase inhibitor, increases the rate of pyroptosis by suppressing the DNA damage repair pathway to enhance the chemosensitivity of cisplatin in ESCC (18). Wang et al. found that metformin might induce EC cell pyroptosis by targeting the microRNA-497/PELP1 (proline, glutamate and leucine rich protein 1) axis (19). Overall, these researches have focused on the induction of pyroptosis with drugs working via various pathways. However, no coherent study has yet explored the mechanisms related to the pyroptosis of ESCC. Thus, in our study, we first evaluated patients’ ESCC PRGs and obtained a total of 283 modular genes that correlated with cell pyroptosis and N stage using WGCNA. To further explore the key genes acting on ESCC, we found 3 signature genes AADAC, GSTA1, and KCNS3 associated with cell pyroptosis using LASSO regression algorithms.
AADAC, as an esterase functioning at the endoplasmic reticulum (ER), is expressed chiefly in the gastrointestinal tract and liver and has been linked with cancer in some studies. Wang et al. reported that AADAC is an important prognostic factor whose high expression is significantly correlated with better OS and disease-free survival (DFS) for patients with Borrmann type III advanced gastric cancer (GC). Additionally, AADAC messenger RNA (mRNA) and protein expression levels have been found to be increased in differentiated GC cells (20). Through examining American and European patient populations of The Cancer Genome Atlas stomach adenocarcinoma (TCGA-STAD) data set, it was speculated that AADAC may enhance the expression of amino and fatty acids in tumor cells to promote cell growth by supplying energy for the rapid proliferation. In another study, Wang et al. identified AADAC as being upregulated in ovarian tumor tissues and reported that patients with a high expression level of AADAC had favorable survival compared to those with low AADAC expression. Additionally, overexpression of AADAC was demonstrated to inhibit the malignant progression of ovarian cancer cells. Both cisplatin and imatinib could suppress malignant cancer cell progression, while overexpression of AADAC synergistically enhanced such inhibition (21).
Glutathione S-transferases (GSTs) enhance the electrophilic centers’ response to lipophilic and glutathione compounds, resulting in the products of oxidative stress, xenobiotics, and neutralization of toxic compounds. Glutathione S-transferase A1 (GSTA1), a GST member, may correlate with lung (22,23), bladder (24,25), gastric (26), breast (27,28), and colorectal cancer (29-31). In their study, Tong et al. identified 6 differentially expressed genes (SLC17A3, SLC17A4, NAT8, GSTA1, GSTA2, and FABP7) which were downregulated in clear cell renal cell carcinoma (ccRCC) and may be related to the immune signature (32). However, the mechanism underlying this effect of GSTA1 has not been clarified.
KCNS3, the gene encoding Kv9.3 potassium channel α-subunit, is selectively expressed by parvalbumin neurons. The correlation between KCNS3 and cancer was not clear and need more exploration. One study reported that adenoma/advanced adenoma risk correlated with 49 mutations, single-nucleotide polymorphisms, or haplotypes in 23 genes or chromosomal regions including KCNS3 (3p24.1, 9q33.2, 13q33.2, APC, ALOX12/15, COX1/2, CYP2C9/24A1/7A1, DRD2, EGFR, EPHB1/KY, FAM188b, IL23R, IGSF5, KCNS3, KRAS, PGDH, ODC, SRC, UGT1A6) (33).
In our study, we used these 3 signature genes to establish a risk panel to classify TCGA-ESCC cohort patients into a high-risk group and low-risk group. We discovered there to be a close relationship between ESCC T and N tumor stage and this risk score. Our results confirmed that the risk panel may have an important role in the prognosis of ESCC.
Our findings suggest that the prognostic biomarkers related to pyroptosis may have a role in regulating ESCC through interactions with the tumor immune microenvironment. However, the mechanisms behind the relationship between pyroptosis and immunity have not been studied extensively, and there are few existing studies in this area. While Liu et al. did identify potential biomarkers related to immune infiltration in heart failure (CALU and PALLD) (34), more research is required to fully understand this relationship in the context of ESCC.
However, there were several limitations in our study. First, we collected 75 ESCC patients which might not be adequate for a comprehensive analysis. Second, the vertical extent of our study which deeply explored the mechanisms of these 3 genes and the interaction with pyroptosis and immunity was not sufficient. Third, this research was short of other cohort and a follow-up for further validation. Thus, the pyroptosis and immunity associated signature explored in this study requires further validation by more prospective studies.
Conclusions
AADAC, GSTA1, and KCNS3 significantly related to ESCC pyroptosis. This risk model incorporating these 3 biomarkers may help predict pyroptosis of ESCC, and these genes may serve as therapeutic targets in the future treatment of ESCC.
Acknowledgments
Funding: None.
Footnote
Reporting Checklist: The authors have completed the TRIPOD reporting checklist. Available at https://jtd.amegroups.com/article/view/10.21037/jtd-23-206/rc
Peer Review File: Available at https://jtd.amegroups.com/article/view/10.21037/jtd-23-206/prf
Conflicts of Interest: All authors have completed the ICMJE uniform disclosure form (available at https://jtd.amegroups.com/article/view/10.21037/jtd-23-206/coif). The authors have no conflicts of interest to declare.
Ethical Statement: The authors are accountable for all aspects of the work in ensuring that questions related to the accuracy or integrity of any part of the work are appropriately investigated and resolved. The study was conducted in accordance with the Declaration of Helsinki (as revised in 2013).
Open Access Statement: This is an Open Access article distributed in accordance with the Creative Commons Attribution-NonCommercial-NoDerivs 4.0 International License (CC BY-NC-ND 4.0), which permits the non-commercial replication and distribution of the article with the strict proviso that no changes or edits are made and the original work is properly cited (including links to both the formal publication through the relevant DOI and the license). See: https://creativecommons.org/licenses/by-nc-nd/4.0/.
References
- Sung H, Ferlay J, Siegel RL, et al. Global Cancer Statistics 2020: GLOBOCAN Estimates of Incidence and Mortality Worldwide for 36 Cancers in 185 Countries. CA Cancer J Clin 2021;71:209-49. [Crossref] [PubMed]
- Wei W, Zeng H, Zheng R, et al. Cancer registration in China and its role in cancer prevention and control. Lancet Oncol 2020;21:e342-9. [Crossref] [PubMed]
- Reichenbach ZW, Murray MG, Saxena R, et al. Clinical and translational advances in esophageal squamous cell carcinoma. Adv Cancer Res 2019;144:95-135. [Crossref] [PubMed]
- Gong W, Xu J, Wang Y, et al. Nuclear genome-derived circular RNA circPUM1 localizes in mitochondria and regulates oxidative phosphorylation in esophageal squamous cell carcinoma. Signal Transduct Target Ther 2022;7:40. [Crossref] [PubMed]
- Moody S, Senkin S, Islam SMA, et al. Mutational signatures in esophageal squamous cell carcinoma from eight countries with varying incidence. Nat Genet 2021;53:1553-63. [Crossref] [PubMed]
- Hanahan D. Hallmarks of Cancer: New Dimensions. Cancer Discov 2022;12:31-46. [Crossref] [PubMed]
- Lian L, Teng SB, Xia YY, et al. Development and verification of a hypoxia- and immune-associated prognosis signature for esophageal squamous cell carcinoma. J Gastrointest Oncol 2022;13:462-77. [Crossref] [PubMed]
- Pu Y, Lu X, Yang X, et al. Estimating the prognosis of esophageal squamous cell carcinoma based on The Cancer Genome Atlas (TCGA) of m6A methylation-associated genes. J Gastrointest Oncol 2022;13:1-12. [Crossref] [PubMed]
- Cui J, Zhao S, Li Y, et al. Regulated cell death: discovery, features and implications for neurodegenerative diseases. Cell Commun Signal 2021;19:120. [Crossref] [PubMed]
- Yu P, Zhang X, Liu N, et al. Pyroptosis: mechanisms and diseases. Signal Transduct Target Ther 2021;6:128. [Crossref] [PubMed]
- Galluzzi L, Buque A, Kepp O, et al. Immunogenic cell death in cancer and infectious disease. Nat Rev Immunol 2017;17:97-111. [Crossref] [PubMed]
- Newman A, Liu C, Green M, et al. Robust enumeration of cell subsets from tissue expression profiles. Nature methods 2015;12:453-7. [Crossref] [PubMed]
- Bertheloot D, Latz E, Franklin BS. Necroptosis, pyroptosis and apoptosis: an intricate game of cell death. Cell Mol Immunol 2021;18:1106-21. [Crossref] [PubMed]
- Zhang Y, Chen Y. Stratification From Heterogeneity of the Cell-Death Signal Enables Prognosis Prediction and Immune Microenvironment Characterization in Esophageal Squamous Cell Carcinoma. Front Cell Dev Biol 2022;10:855404. [Crossref] [PubMed]
- Zheng ZY, Yang PL, Li RY, et al. STAT3β disrupted mitochondrial electron transport chain enhances chemosensitivity by inducing pyroptosis in esophageal squamous cell carcinoma. Cancer Lett 2021;522:171-83. [Crossref] [PubMed]
- Zhang B, Zhu WY, Tian H, et al. Alpinumisoflavone triggers GSDME-dependent pyroptosis in esophageal squamous cell carcinomas. Anat Rec (Hoboken) 2021;304:323-32. [Crossref] [PubMed]
- Jiang M, Wu Y, Qi L, et al. Dihydroartemisinin mediating PKM2-caspase-8/3-GSDME axis for pyroptosis in esophageal squamous cell carcinoma. Chem Biol Interact 2021;350:109704. [Crossref] [PubMed]
- Wu M, Wang Y, Yang D, et al. A PLK1 kinase inhibitor enhances the chemosensitivity of cisplatin by inducing pyroptosis in oesophageal squamous cell carcinoma. EBioMedicine 2019;41:244-55. [Crossref] [PubMed]
- Wang L, Li K, Lin X, et al. Metformin induces human esophageal carcinoma cell pyroptosis by targeting the miR-497/PELP1 axis. Cancer Lett 2019;450:22-31. [Crossref] [PubMed]
- Wang Y, Fang T, Wang Y, et al. Impact of AADAC gene expression on prognosis in patients with Borrmann type III advanced gastric cancer. BMC Cancer 2022;22:635. [Crossref] [PubMed]
- Wang H, Wang D, Gu T, et al. AADAC promotes therapeutic activity of cisplatin and imatinib against ovarian cancer cells. Histol Histopathol 2022;37:899-907. [PubMed]
- Kumar M, Martin A, Nirgude S, et al. Quinacrine inhibits GSTA1 activity and induces apoptosis through G1/S arrest and generation of ROS in human non-small cell lung cancer cell lines. Oncotarget 2020;11:1603-17. [Crossref] [PubMed]
- Sorensen M, Autrup H, Tjonneland A, et al. Genetic polymorphisms in CYP1B1, GSTA1, NQO1 and NAT2 and the risk of lung cancer. Cancer Lett 2005;221:185-90. [Crossref] [PubMed]
- Matic M, Pekmezovic T, Djukic T, et al. GSTA1, GSTM1, GSTP1, and GSTT1 polymorphisms and susceptibility to smoking-related bladder cancer: a case-control study. Urol Oncol 2013;31:1184-92. [Crossref] [PubMed]
- Savic-Radojevic A, Djukic T, Simic T, et al. GSTM1-null and GSTA1-low activity genotypes are associated with enhanced oxidative damage in bladder cancer. Redox Rep 2013;18:1-7. [Crossref] [PubMed]
- Nguyen TV, Janssen MJ, van Oijen MG, et al. Genetic polymorphisms in GSTA1, GSTP1, GSTT1, and GSTM1 and gastric cancer risk in a Vietnamese population. Oncol Res 2010;18:349-55. [Crossref] [PubMed]
- Ahn J, Gammon MD, Santella RM, et al. Effects of glutathione S-transferase A1 (GSTA1) genotype and potential modifiers on breast cancer risk. Carcinogenesis 2006;27:1876-82. [Crossref] [PubMed]
- Terrazzino S, La Mattina P, Gambaro G, et al. Common variants of GSTP1, GSTA1, and TGFbeta1 are associated with the risk of radiation-induced fibrosis in breast cancer patients. Int J Radiat Oncol Biol Phys 2012;83:504-11. [Crossref] [PubMed]
- Tijhuis MJ, Wark PA, Aarts JM, et al. GSTP1 and GSTA1 polymorphisms interact with cruciferous vegetable intake in colorectal adenoma risk. Cancer Epidemiol Biomarkers Prev 2005;14:2943-51. [Crossref] [PubMed]
- Hezova R, Bienertova-Vasku J, Sachlova M, et al. Common polymorphisms in GSTM1, GSTT1, GSTP1, GSTA1 and susceptibility to colorectal cancer in the Central European population. Eur J Med Res 2012;17:17. [Crossref] [PubMed]
- Adnan H, Antenos M, Kirby GM. The effect of menadione on glutathione S-transferase A1 (GSTA1): c-Jun N-terminal kinase (JNK) complex dissociation in human colonic adenocarcinoma Caco-2 cells. Toxicol Lett 2012;214:53-62. [Crossref] [PubMed]
- Tong Y, Yu Y, Zheng H, et al. Differentially Expressed Genes in Clear Cell Renal Cell Carcinoma as a Potential Marker for Prognostic and Immune Signatures. Front Oncol 2021;11:776824. [Crossref] [PubMed]
- Johnstone MS, Lynch G, Park J, et al. Novel Methods of Risk Stratifying Patients for Metachronous, Pre-Malignant Colorectal Polyps: A Systematic Review. Crit Rev Oncol Hematol 2021;164:103421. [Crossref] [PubMed]
- Liu X, Xu S, Li Y, et al. Identification of CALU and PALLD as Potential Biomarkers Associated With Immune Infiltration in Heart Failure. Front Cardiovasc Med 2021;8:774755. [Crossref] [PubMed]
(English Language Editor: J. Gray)