A novel anoikis-related gene signature to predict the prognosis, immune infiltration, and therapeutic outcome of lung adenocarcinoma
Highlight box
Key findings
• This is the first study to use ARGs to construct a risk model to predict clinical outcomes and immune infiltration in LUAD, which contributed to the discovery that anoikis plays a large role in the TME regulation and immune pathway of LUAD.
What is known and what is new?
• Anoikis is a form of programmed cell death that is closely related to tumor metastasis, and ARGs have been shown to play an important role in the progression of various malignancies such as prostate cancer, endometrial cancer, and gastric cancer.
• This study explored the molecular alterations and clinical correlation of ARGs in LUAD, and investigated the TME and drug sensitivity in relation to ARGs.
What is the implication, and what should change now?
• Our research provided new views of anoikis between the TME and LUAD progression, and contributed to the evidence base for tumor treatment.
Introduction
Lung cancer is one of the most common cancers in the world and is the leading cause of cancer-related deaths. In 2020, 2.2 million new cases and 1.8 million deaths were estimated globally, accounting for 11.4% of total cancer cases and 18% of total cancer deaths, respectively (1). Lung cancer often has no obvious symptoms in the early stage, and is mostly detected in the advanced stage, which is often accompanied by a poor prognosis (2,3). Lung adenocarcinoma (LUAD), belonging to non-small cell lung cancers (NSCLC), is the most common histological subtype of lung cancer, the proportion of which is gradually increasing (1,4). Even though patient survival times have improved significantly due to the improved mechanisms, diagnostic, and treatment options, LUAD remains a deadly disease (5). A deeper understanding of the mechanisms of lung cancer pathogenesis and metastasis is essential to improve patient survival. Therefore, more new reliable biomarkers to predict patient prognosis are required.
Tumor metastasis is a complex, multi-step process that involves a variety of complex mechanisms, such as tumor tissue migration, survival in intravascular and circulatory domains, extravasation, homing, and metastatic colonization (6,7). For metastasis, tumor cells must detach from the substrate or cell-cell anchor of the tissue structure. Anoikis is a type of programmed cell death that results from prolonged suspension of cells, caused by the detachment of cells from the extracellular matrix (ECM). When cells separate from the substrate, endogenous and exogenous apoptotic pathways are initiated and apoptosis occurs to prevent abnormal cell proliferation (8). Indeed, both endogenous and exogenous pathways of apoptosis can be triggered by the loss of anchoring of integrins to the extracellular matrix, which is also known as anoikis. The acquisition of tumor metastatic ability requires resistance to apoptosis (9). Anoikis-related genes (ARGs) have been demonstrated to act a key factor in the progression of many aggressive malignancies such as endometrial carcinoma (10), prostate cancer (11), and gastric carcinoma (12). Multiple factors including Ras family, PI3K/AKT pathway and extracellular matrix adhesion can regulate anoikis. Controlling these factors to activate anoikis has been an important avenue for tumor therapy (13-15). Resistance to anoikis may also be an important reason of resistance to radiotherapy and chemotherapy in tumors (15,16). However, prognostic indicators based on ARGs have rarely been analyzed in LUAD. There was similar article has been published recently(17), however,our study could be more comprehensive because we not only constructed prediction risk model but also established two variously subtypes with “ConsensusClusterPlus” package and the clinical features and biological pathway in the two subtypes were also detected. In this study, we aimed to investigate the molecular alterations and clinical relevance of ARGs in LUAD, and to discuss the relationship between ARGs and LUAD prognosis, we found anoikis could regulate the immune function and metabolism procession which played great roles in prognosis in LUAD. In addition, we explored ARGs between the tumor microenvironment (TME) and drug sensitivity in LUAD. We present the following article in accordance with the TRIPOD reporting checklist (available at https://jtd.amegroups.com/article/view/10.21037/jtd-23-149/rc).
Methods
Data download
We sourced the RNA sequencing transcriptome data, gene mutation, and the corresponding clinical data from The Cancer Genome Atlas (TCGA; https://cancergenome.nih.gov/). The datasets of GSE50081 (https://www.ncbi.nlm.nih.gov/geo/query/acc.cgi?acc=GSE50081) were obtained to gain gene expression and clinical data of validation cohorts from the Gene Expression Omnibus (GEO) database (https://www.ncbi.nlm.nih.gov/geo/). Gene Expression Profiling Interactive Analysis (GEPIA; http://gepia.cancer-pku.cn/) was performed to validate the expression of ARGs. A total of 434 ARGs were extracted from previously published literature. Bioinformatic analysis was performed using R software v4.1.3 (R Foundation for statistical Computing, Vienna, Austria). The study was conducted in accordance with the Declaration of Helsinki (as revised in 2013).
Consensus clustering of ARGs
The “limma” package was used to identify differentially expressed genes (DEGs) between LUAD and normal tissues, and the genes with a P value <0.05 were adopted. We performed unsupervised cluster analysis (k-means) using the “ConsensusClusterPlus” package in R software, and the cluster values of ARGs were determined based on their expression levels. The clusters (k) were selected by the Bayesian information criterion.
Qualification and validation of the ARGs prognostic signature
Univariate Cox analysis was used to assess prognostic factors, and then 91 genes were selected for further least absolute shrinkage and selection operator (LASSO) Cox regression analysis to construct a risk model. Applying the coefficients calculated by LASSO regression with the minimum criteria for determining the penalty parameter (λ) and gene expression level, the risk score formula was obtained as follows: risk score = (exprgene1×Coefgene1) + (exprgene2×Coefgene2) + ... + (exprgenen×Coefgenen). Patients with LUAD in TCGA and GEO were then divided into high- and low-risk groups according to the median risk score. Kaplan-Meier (KM) curves were then analyzed with the R packages survival and survminer, and the packages time. Receiver operating characteristic (ROC) curves were used to assess whether our models were sensitive to different overall survival (OS) and to analyze independent risk factors for different clinical factors, including age, sex, stage, and risk score.
Statistical analysis
Gene set enrichment analysis (GSEA) and analysis of immune cell infiltration
For bioinformatics analysis, GSEA was used to search possible biological characteristics that may influence the risk groups, including the changed signaling pathway. Gene Ontology (GO) was carried out to calculate the upregulated and downregulated pathway. The single-sample GSEA (ssGSEA) with the GSVA Bioconductor package including 29 types of immune cells and immune biomarkers (https://bioconductor.org/packages/release/bioc/html/GSVA.html) was performed to explore the levels of immune function. The ESTIMATE algorithm could evaluate the amount of various cells in tumor microenvironment by estimated score, stromal score, immune score, and corresponding tumor purity, the level of cells was proportional to score. The Cell-type Identification by Estimating Relative Subsets of RNA Transcripts (CIBERSORT) algorithm with 22 types of immune cells was used to calculate the immune infiltration.
Tumor mutation burden analysis
Somatic mutation (VarScan2 Variant Aggregation and Masking) data of LUAD were downloaded from the TCGA database in mutation annotation format (MAF) form. The Maftools package in R was performed to explore the tumor mutational burden (TMB) in each LUAD patient, then median value of TMB was regarded as the cut off to divide patients into high- and low-TMB groups.
Immune check points and clinical treatment
The effectiveness of immunotherapy on LUAD was estimated with tumor immune dysfunction and exclusion (TIDE; http://tide.dfci.harvard.edu/), Tumor Cancer Immunome Atlas (TCIA; https://tcia.at/home), and Imvigor210 (http://research-pub.Gene.com/imvigor210corebiologies/). The differences of immune check points between the risk groups were also performed with R package “limma”. The “pRRophetic” package could be performed to evaluate drug sensibility based on half maximal inhibitory concentration (IC50).
Results
Clustering of ARGs and analysis of the clinical features in each subtype
We collected the ARGs from previous report (10) and identified 334 DEGs in LUAD patients from TCGA database (Figure 1A). Suitable groups were divided using the algorithm of consensus clustering with expression of DEGs. We found that 2 clusters may be optimal, namely, cluster 1 with 225 patients and cluster 2 with 242 patients (Figure 1B,1C). Principal component analysis (PCA) was conducted to detect the distribution pattern of gene expression in the 2 clusters (Figure 1D). Survival analysis showed that cluster 1 had less favorable OS (P=0.039) than cluster 2 (Figure 1E). Clinical features were significant different between the 2 diverse subtypes, in terms of gender (P<0.05), American Joint Committee on Cancer (AJCC) stage (P<0.001), T stage (P<0.01), and N stage (P<0.001) (Figure 1F). In addition, immune-related pathways were mainly enriched in cluster 2 whereas cell cycle and DNA regulation pathways were enriched in cluster 1 (Figure 1G,1H).
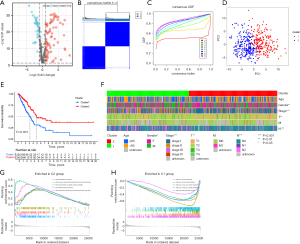
Construction and validation the ARGs risk signature
We performed univariate Cox analysis to distinguish the prognostic ARGS from DEGs, and 91 genes were significantly associated with OS in LUAD patients. Then, LASSO regression analysis was conducted to construct the prognostic model, indicating 21 genes including MCL1, TLE1, ANGPTL4, TIMP1, ITGA6, PIK3CG, FADD, CDKN3, EDA2R, EIF2AK3, CEMIP, LTB4R2, PPP1R13B, FGF2, PIK3R2, COL4A2, SHC1, CDC25C, SLCO1B3, LDHA, RHOQ (Figure 2A,2B). Univariate Cox hazard ratio (HR) values were also performed, which showed that LDHA had the highest HR value (Figure 2C). GSEA was carried out to explore the biological function of our risk model, indicating that the high-risk groups were mainly associated with ECM organization and DNA replication, whereas metabolism process was enriched in the low risk groups (Figure 2D,2E). Go analysis showed that 21 anoikis could specially modulate mitosis, microvillus regulation and cellular adhension (Figure 2F).
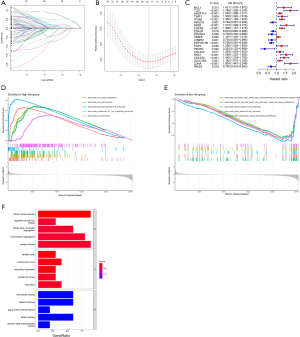
Survival analysis and proof of risk signature
In order to explore the OS in LUAD patients, KM survival analysis was carried out, which revealed that the high-risk group had a more likely poor prognosis than the low-risk group (P<0.001, Figure 3A). ROC curves confirmed the accuracy of risk model by comparing the area under the ROC curve (AUC), which was 0.762 for 1-year, compared to 0.747 and 0.697 for 3 and 5 years, respectively. Therefore, our risk signature could precisely predict the OS of BC patients (Figure 3B). Furthermore, we also found higher risk scores incurred a less favorable prognosis (Figure 3C,3D). The distribution of ARGs’ expression in our risk model was visualized in a heatmap (Figure 3E).
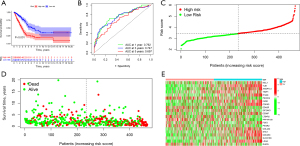
Validation cohorts of the risk signature
KM survival analysis was further conducted to confirm the validity of our risk model, whereby low-risk groups had better OS in GSE50081 (P=0.041) (Figure 4A). The ROC curves suggested that our risk model was accurate (Figure 4B). In GSE50081, increasing risk scores were correlated with less favorable survival (Figure 4C,4D). The landscape of risk ARGs expression was explored with the heatmap in GSE50081 (Figure 4E).
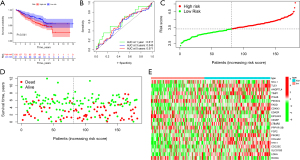
Clinical characteristic signatures of ARGs in LUAD
Based on univariate Cox analysis and multivariate Cox analysis, we found that risk score was an independent predictor of OS [HR =4.655, 95% confidence interval (CI): 3.419–6.337 and HR =4.025, 95% CI: 2.884–5.617] (Figure 5A,5B). In addition, our risk model had the highest AUC value (AUC =0.762) compared with other clinical characteristics including age (AUC =0.485), gender (AUC =0.438), AJCC stage (AUC =0.725), T stage (AUC =0.664), N stage (AUC =0.659), and M stage (AUC =0.504) (Figure 5C). The distribution of clinical characteristic signatures suggested that the high-risk group tended to have higher AJCC stage and T and N stages (Figure 5D). The survival of LUAD patients in 1-, 3-, and 5-year were assessed according to a nomogram (Figure 5E). The predictions of OS were effective, as presented in the calibration plot, suggesting that both the risk and nomogram models were accurate (Figure 5F).
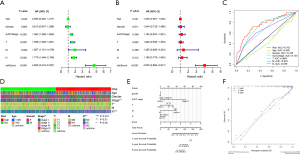
Association between TMB and ARGs risk signature
Since gene mutation could lead to tumor progression, we explored the differences of somatic mutations between high- and low-risk groups. The distribution of mutated genes and mutation types were explored with waterfall plots (Figure 6A,6B). Interestingly, high-risk groups showed high TMB and the level of TMB was correlated to risk score (Figure 6C,6D). However, because a high level of TMB suggested more favorable OS (Figure 6E), we then explored the cooperative effect of the TMB and risk score to predict the prognosis of LUAD patients, which indicated that high TMB with low risk group had the most favorable OS whereas TMB with high risk group had the least favorable OS (Figure 6F).
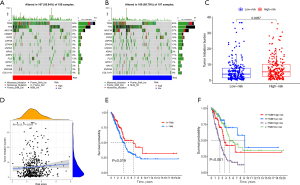
Immune infiltration and TME in ARGs risk signature
The distribution of immune status in diverse risk groups was analyzed with ssGSEA. A heatmap with 29 immune markers showed a comprehensive immune infiltration landscape for the TCGA LUAD cohort (Figure 7A). CIBERSORT was applied to determine how immune cell expressed; T cells CD4 memory activated (P=0.007), natural killer (NK) cells resting (P=0.007), macrophages M0 (P<0.001), and mast cells activated (P=0.037) were enriched in high risk groups, whereas plasma cells (P=0.002), T cells CD4 memory resting (P=0.036), T cells follicular helper (P=0.047), and mast cells resting (P<0.001) expressed more in low risk groups (Figure 7B). The association between level of immune cell and risk score was also presented (Figure 7C-7H). Exploration of the TME showed that high risk scores were expressed more in low estimate score, low immune score, and low tumor purity, but there were no significant differences between high- and low-stromal score (Figure 7I-7L).
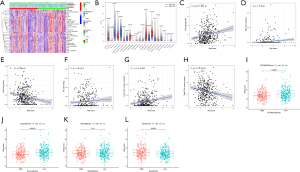
Clinical response of ARGs risk signature
There is no doubt that immune checkpoint inhibitors (ICIs) can influence therapeutic effects of immunotherapy(18); the expression of BTLN2, LOXL2, MSH6, CTLA4, BTLA, POLE2, and MSH2 displayed statistical differences in our risk model (Figure 8A). The risk score was proportionate to the level of MSH6, MSH2, POLE2, and LOXL2 but was contrary to BTLN2, CTLA4, and BTLA (Figure 8B). TIDE, TCIA, and IMvigor210 were used to predict the immunotherapy response in ARGs risk model, which indicated that high-risk groups might benefit more from immunotherapy based on TIDE (Figure 8C). Notably, low-risk groups that were CTLA4 negative with PD1 negative and CTLA4 positive with PD1 negative displayed a better response in TCIA (Figure 8D-8G). IMvigor210 is a database including advanced or metastatic urothelial carcinoma patients treated with atezolizumab (19). We used the ARGs risk model to analyze IMvigor210, which suggested that our model could divide the included patients into high- and low-risk groups, but there no differences of immunotherapy response associated with risk score (Figure 9). Based on “pRRophetic” package we found the values of IC50 were higher in patients in low risk group suggesting they could benefit more from chemotherapy including cisplatin, docetaxel, erlotinib, gemcitabine, sunitinib and vinorelbine (Figure 10).
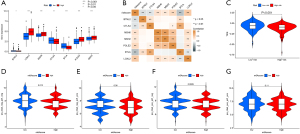
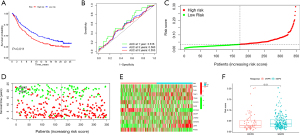
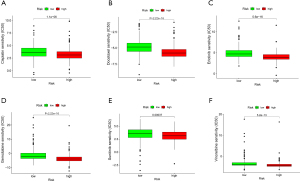
The expression of 21 ARGs in tissues
The expression distribution of 21 ARGs in normal tissues (n=54) and tumor tissues (n=501) were explored among TCGA LUAD patients (Figure 11A). Furthermore, the expression differences between normal and tumor tissues were also investigated (Figure 11B-11V). Finally, we used GEPIA database including 347 normal tissues and 483 tumor tissues of LUAD to prove the expression of 21 ARGs based on TCGA (Figure 12).
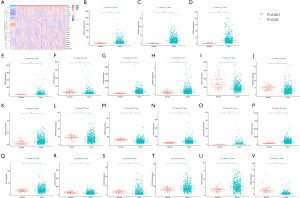
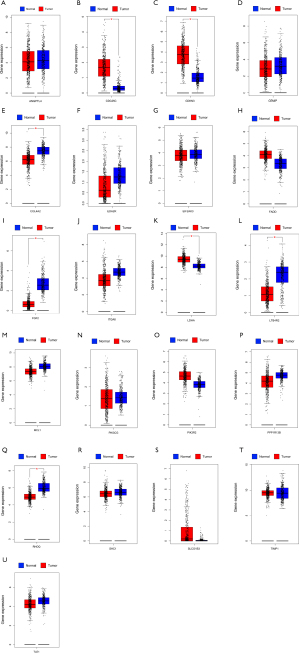
Discussion
Anoikis is a type of programmed cell death occurring when cells cleave away from their extracellular adhesion site to prevent dysplastic cells from growing or attaching to an inappropriate matrix (20). Cancer cells could overcome anoikis which would contribute to tumor progression, metastasis, and resistance of therapy (21). Previous studies (13,22) have demonstrated that anoikis plays great roles in the development of lung cancer. Cellular retinoic acid binding protein 2 (CRABP2) was shown to promote integrin β1/FAK/ERK signaling and inhibit anoikis; overexpressed CRABP2 was found to increase lymph node and liver metastasis through in vivo tail vein injection of lung cancer cell models (23). Neurotrophic tyrosine kinase receptor 2 (NTRK2/TrkB) is a neurotrophin that serves as a growth factor regulating embryonic stem cells and promoting tumorigenesis in cancer cells. TrkB is upregulated in anoikis-resistant lung-derived tumor cells isolated from malignant ascites (24). In addition anoikis could also promote the fibrosis in cardiovascular diseases and progression of diabetic retinopathy (25). However, to our knowledge, few articles have investigated how ARGs impact on prognosis, immune infiltration, and clinical response in lung cancer, thus, we constructed the risk signature to predict prospective treatment targets and prognostic markers for LUAD.
In our study, we firstly used the R package consensus clustering to identify 2 subtypes, including cluster 1 and cluster 2, based on the DEGs of anoikis. The 2 disparate clusters had various prognosis, biological pathways, clinical features, and immune infiltration. Cluster 1 had less favorable prognosis and advanced tumor stage. Besides, we also found that immune-related pathways were enriched in cluster 2 and mitosis-related pathways were enriched in cluster 1, suggesting that ARGs could be involved in cell cycle and immune infiltration. Algorithms of ssGSEA were performed to detect immune function and TME in LUAD patients, which revealed that cluster 2 had richer immune cell levels; however, the relationship between immune function and anoikis remains unclear in LUAD. We hope that our research can provide a perspective for exploration in the future.
Subsequently, we constructed a prognostic prediction model using the LUAD database from TCGA consisting of 21 ARGs. Our risk model could predict the prognosis of LUAD based on the division of low- and high-risk groups. In our model, patients in high-risk groups had less favorable OS, which was confirmed through the independent database GSE5008. In addition, the predictive accuracy of the risk model was detected by ROC curves, in which the highest value of AUC was our risk model compared with that of other clinical features, and based on univariate and multivariate Cox analyses, the risk score was the independent factor. Moreover, based on GSEA and Go, we found ARGs could involve in cellular mitosis, adhesion and DNA replication to regulate therapeutic target, prognosis and tumor progression (26,27). Among our 21 risk ARGs, we found that MCL1, TLE1, ANGPTL4, TIMP1, ITGA6, FADD, CDKN3, CEMIP, FGF2, COL4A2, SHC1, CDC25C, SLCO1B3, and LDHA were associated with poor prognosis, particularly LDHA. Lactate dehydrogenase (LDHA) is associated with glycolysis, which serves as a target gene of Wnt/β-catenin signaling and is activated when there is loss of dual serine/threonine tyrosine protein kinase (DSTYK), leading to the promotion of tumorigenesis of lung cancer (28). MCL1 could promote the RET fusion MET exon 14 skipping mutation, when MCL1 was inhibited the prognosis of LUAD would be better (29). Interestingly, TLE1, ANGPTL4, TIMP1, ITGA6, FADD, CDKN3, CEMIP, FGF2, SHC1, CDC25C and SLCO1B3 were also associated with metabolism and tumor microenvironment in LUAD (30-32), suggesting that impact factors of anoikis could also play roles in metabolism and progression in LUAD. Besides, EDA2R and PPP1R13B were firstly identified as the protective factors for LUAD. Ectodysplasin A2 receptor (EDA2R) could be a transcriptional activator of P53, which is a tumor suppressor, and coordinates a multitude of cellular and organismal processes (33). PPP1R13B is a major member of the apoptosis-stimulating proteins of the p53 family which might promote the proliferation and migration of lung fibroblasts through endoplasmic reticulum stress (ERS) and autophagy, and plays a crucial role in the development of pulmonary fibrosis in silicosis (34). Thus, our study might provide new insights for future research.
Notably we found that anoikis could play critical roles in regulation of immune infiltration. High-risk groups tended to have higher levels of mast cells activated, NK cells resting, and T cells memory activated, whereas mast cells resting and T cells CD4 memory resting were enriched in low-risk groups. Previous report has demonstrated that increased CD4+ T cells are a favorable independent prognostic factor for lung cancer (35). Mast cells are a major component of the immune microenvironment and modulate tumor progression by releasing pro-tumorigenic and antitumorigenic molecules, in LUAD, several published studies have shown that mast cells abundance is correlated with prolonged survival in early-stage LUAD patients (36,37). In our opinion, anoikis serves as a critical biological process that antagonizes lung cancer metastasis associated with extracellular matrix, cell adhesion-related membrane proteins, cytoskeletal regulators, and epithelial-mesenchymal transition (13). However, no previous studies have shown that anoikis might play roles in the immune microenvironment in LUAD; our research suggested that anoikis might be involved in regulation of immune function, and we hope that related mechanism research will be performed in future.
The TME plays a critical role in tumorigenesis and tumor progression (38). Interestingly, anoikis could greatly influence the regulation of the TME. Based on our model, we found that high immune score was associated with low-risk score, suggesting that active immune cells could lead patients in this group to better OS. Our conclusion agrees with the report by Qu et al., who constructed a risk model according to immune-related genes (39). Deng et al. reported that the low tumor purity group was mainly enriched in immune-related pathways, such as T cell, B cell, and macrophage pathways, they thought immune cells might kill tumor cells leading to low tumor purity associated with better prognosis (40). In this study, we found that high tumor purity suggested poor prognosis which is consistent with the conclusion of Deng. Therefore, the various scores of TME were enrich in different risk scores of our model, indicating that anoikis could influence the progression of tumor microenvironment and regulate the prognosis.
The effectiveness of clinical treatment and differences in 2 groups were studied to predict immunotherapy response in LUAD patients. ICIs were various in the 2 divided risk groups, suggesting that ARGs could regulate the expression level of ICIs. In addition, the low-risk group showed better response to immunotherapy in CTLA4 negative with PD1 negative and CTLA4 positive with PD1 negative in TCIA database, however, the high risk group benefited more from immunotherapy in the TIDE database, and there was no difference between high- and low-risk groups in IMvigor210, which suggested that different databases could lead to various clinical responses, but these results further highlight that ARGs have an impact in immunotherapy in LUAD patients.
Besides, we also explored the effectiveness of chemotherapeutic agents in two risk groups, we found that low risk groups response better to all of 6 drugs including cisplatin, docetaxel, erlotinib, gemcitabine, sunitinib and vinorelbine. Our study might provide guidance in clinical practice.
The expression of CDC25, CDKN3 and LDHA was enriched in tumor tissues and COL4A2, FGF2, LTB4R2 and RHOQ expressed more in normal tissues from GEPIA. However, in TCGA, gene LTB4R2 was more in tumor tissues which was contrary from the result based on GEPIA, thus patients from different databases could lead to various conclusions, suggesting that multicenter trials to provide high-level evidence for clinical application.
However, the present study had several limitations, there were few cohorts available for validation of our risk model. The number of normal tissue samples in the TCGA cohort was relatively small which might lead to bias. The tumor immune microenvironment data was obtained based on algorithms rather than through clinical validation. More experiments should be performed to validate the pathway of anoikis.
Conclusions
To our knowledge, this is the first study to use ARGs to construct a risk signature for predicting the clinical outcomes and immune infiltration in LUAD. Our model based on 21 ARGs could divide patients into low- and high-risk subgroups efficiently with diverse prognoses and immune cell infiltration. This represents the first time that anoikis has been noticed to play great roles in TME regulation and immune pathways in LUAD. Thus, we are firmly convinced that our findings will provide a new perspective for the future exploration in anoikis. Our research could offer new opinions regarding the importance of anoikis in the TME and LUAD development.
Acknowledgments
Funding: None.
Footnote
Reporting Checklist: The authors have completed the TRIPOD reporting checklist. Available at https://jtd.amegroups.com/article/view/10.21037/jtd-23-149/rc
Peer Review File: Available at https://jtd.amegroups.com/article/view/10.21037/jtd-23-149/prf
Conflicts of Interest: All authors have completed the ICMJE uniform disclosure form (available at https://jtd.amegroups.com/article/view/10.21037/jtd-23-149/coif). The authors have no conflicts of interest to declare.
Ethical Statement: The authors are accountable for all aspects of the work in ensuring that questions related to the accuracy or integrity of any part of the work are appropriately investigated and resolved. The study was conducted in accordance with the Declaration of Helsinki (as revised in 2013).
Open Access Statement: This is an Open Access article distributed in accordance with the Creative Commons Attribution-NonCommercial-NoDerivs 4.0 International License (CC BY-NC-ND 4.0), which permits the non-commercial replication and distribution of the article with the strict proviso that no changes or edits are made and the original work is properly cited (including links to both the formal publication through the relevant DOI and the license). See: https://creativecommons.org/licenses/by-nc-nd/4.0/.
References
- Sung H, Ferlay J, Siegel RL, et al. Global Cancer Statistics 2020: GLOBOCAN Estimates of Incidence and Mortality Worldwide for 36 Cancers in 185 Countries. CA Cancer J Clin 2021;71:209-49. [Crossref] [PubMed]
- Lurienne L, Cervesi J, Duhalde L, et al. NSCLC Immunotherapy Efficacy and Antibiotic Use: A Systematic Review and Meta-Analysis. J Thorac Oncol 2020;15:1147-59. [Crossref] [PubMed]
- Walters S, Maringe C, Coleman MP, et al. Lung cancer survival and stage at diagnosis in Australia, Canada, Denmark, Norway, Sweden and the UK: a population-based study, 2004-2007. Thorax 2013;68:551-64. [Crossref] [PubMed]
- Barta JA, Powell CA, Wisnivesky JP. Global Epidemiology of Lung Cancer. Ann Glob Health 2019;85:8. [Crossref] [PubMed]
- Howlader N, Forjaz G, Mooradian MJ, et al. The Effect of Advances in Lung-Cancer Treatment on Population Mortality. N Engl J Med 2020;383:640-9. [Crossref] [PubMed]
- Valastyan S, Weinberg RA. Tumor metastasis: molecular insights and evolving paradigms. Cell 2011;147:275-92. [Crossref] [PubMed]
- Wan L, Pantel K, Kang Y. Tumor metastasis: moving new biological insights into the clinic. Nat Med 2013;19:1450-64. [Crossref] [PubMed]
- Frisch SM, Screaton RA. Anoikis mechanisms. Curr Opin Cell Biol 2001;13:555-62. [Crossref] [PubMed]
- Simpson CD, Anyiwe K, Schimmer AD. Anoikis resistance and tumor metastasis. Cancer Lett 2008;272:177-85. [Crossref] [PubMed]
- Chen S, Gu J, Zhang Q, et al. Development of Biomarker Signatures Associated with Anoikis to Predict Prognosis in Endometrial Carcinoma Patients. J Oncol 2021;2021:3375297. [Crossref] [PubMed]
- Berezovskaya O, Schimmer AD, Glinskii AB, et al. Increased expression of apoptosis inhibitor protein XIAP contributes to anoikis resistance of circulating human prostate cancer metastasis precursor cells. Cancer Res 2005;65:2378-86. [Crossref] [PubMed]
- Ye G, Yang Q, Lei X, et al. Nuclear MYH9-induced CTNNB1 transcription, targeted by staurosporin, promotes gastric cancer cell anoikis resistance and metastasis. Theranostics 2020;10:7545-60. [Crossref] [PubMed]
- Wang J, Luo Z, Lin L, et al. Anoikis-Associated Lung Cancer Metastasis: Mechanisms and Therapies. Cancers (Basel) 2022;14:4791. [Crossref] [PubMed]
- Yu Y, Liu B, Li X, et al. ATF4/CEMIP/PKCα promotes anoikis resistance by enhancing protective autophagy in prostate cancer cells. Cell Death Dis 2022;13:46. [Crossref] [PubMed]
- Yu Y, Song Y, Cheng L, et al. CircCEMIP promotes anoikis-resistance by enhancing protective autophagy in prostate cancer cells. J Exp Clin Cancer Res 2022;41:188. [Crossref] [PubMed]
- Zhu Z, Fang C, Xu H, et al. Anoikis resistance in diffuse glioma: The potential therapeutic targets in the future. Front Oncol 2022;12:976557. [Crossref] [PubMed]
- Diao X, Guo C, Li S. Identification of a novel anoikis-related gene signature to predict prognosis and tumor microenvironment in lung adenocarcinoma. Thorac Cancer 2023;14:320-30. [Crossref] [PubMed]
- Dong S, Guo X, Han F, et al. Emerging role of natural products in cancer immunotherapy. Acta Pharm Sin B 2022;12:1163-85. [Crossref] [PubMed]
- Mariathasan S, Turley SJ, Nickles D, et al. TGFβ attenuates tumour response to PD-L1 blockade by contributing to exclusion of T cells. Nature 2018;554:544-8. [Crossref] [PubMed]
- Li S, Zhang J, Qian S, et al. S100A8 promotes epithelial-mesenchymal transition and metastasis under TGF-β/USF2 axis in colorectal cancer. Cancer Commun (Lond) 2021;41:154-70. [Crossref] [PubMed]
- Kim H, Choi P, Kim T, et al. Ginsenosides Rk1 and Rg5 inhibit transforming growth factor-β1-induced epithelial-mesenchymal transition and suppress migration, invasion, anoikis resistance, and development of stem-like features in lung cancer. J Ginseng Res 2021;45:134-48. [Crossref] [PubMed]
- Phetphoung T, Malla A, Rattanapisit K, et al. Expression of plant-produced anti-PD-L1 antibody with anoikis sensitizing activity in human lung cancer cells via., suppression on epithelial-mesenchymal transition. PLoS One 2022;17:e0274737. [Crossref] [PubMed]
- Wu JI, Lin YP, Tseng CW, et al. Crabp2 Promotes Metastasis of Lung Cancer Cells via HuR and Integrin β1/FAK/ERK Signaling. Sci Rep 2019;9:845. [Crossref] [PubMed]
- Miłoszewska J, Przybyszewska M, Gos M, et al. TrkB expression level correlates with metastatic properties of L1 mouse sarcoma cells cultured in non-adhesive conditions. Cell Prolif 2013;46:146-52. [Crossref] [PubMed]
- Taddei ML, Giannoni E, Fiaschi T, et al. Anoikis: an emerging hallmark in health and diseases. J Pathol 2012;226:380-93. [Crossref] [PubMed]
- Bruno S, Ghelli Luserna di Rorà A, Napolitano R, et al. CDC20 in and out of mitosis: a prognostic factor and therapeutic target in hematological malignancies. J Exp Clin Cancer Res 2022;41:159. [Crossref] [PubMed]
- Hamidi H, Ivaska J. Every step of the way: integrins in cancer progression and metastasis. Nat Rev Cancer 2018;18:533-48. [Crossref] [PubMed]
- Zhong C, Chen M, Chen Y, et al. Loss of DSTYK activates Wnt/β-catenin signaling and glycolysis in lung adenocarcinoma. Cell Death Dis 2021;12:1122. [Crossref] [PubMed]
- Hirai S, Idogawa M, Sumi T, et al. Dual inhibition of BCL2L1 and MCL1 is highly effective against RET fusion-positive or MET exon 14 skipping mutation-positive lung adenocarcinoma cells. Biochem Biophys Res Commun 2022;630:24-9. [Crossref] [PubMed]
- Tang B, Hu L, Jiang T, et al. A Metabolism-Related Gene Prognostic Index for Prediction of Response to Immunotherapy in Lung Adenocarcinoma. Int J Mol Sci 2022;23:12143. [Crossref] [PubMed]
- Zhong H, Wang J, Zhu Y, et al. Comprehensive Analysis of a Nine-Gene Signature Related to Tumor Microenvironment in Lung Adenocarcinoma. Front Cell Dev Biol 2021;9:700607. [Crossref] [PubMed]
- Qin J, Xu Z, Deng K, et al. Development of a gene signature associated with iron metabolism in lung adenocarcinoma. Bioengineered 2021;12:4556-68. [Crossref] [PubMed]
- Brosh R, Sarig R, Natan EB, et al. p53-dependent transcriptional regulation of EDA2R and its involvement in chemotherapy-induced hair loss. FEBS Lett 2010;584:2473-7. [Crossref] [PubMed]
- Cheng Y, Luo W, Li Z, et al. CircRNA-012091/PPP1R13B-mediated Lung Fibrotic Response in Silicosis via Endoplasmic Reticulum Stress and Autophagy. Am J Respir Cell Mol Biol 2019;61:380-91. [Crossref] [PubMed]
- Bremnes RM, Al-Shibli K, Donnem T, et al. The role of tumor-infiltrating immune cells and chronic inflammation at the tumor site on cancer development, progression, and prognosis: emphasis on non-small cell lung cancer. J Thorac Oncol 2011;6:824-33. [Crossref] [PubMed]
- Bao X, Shi R, Zhao T, et al. Mast cell-based molecular subtypes and signature associated with clinical outcome in early-stage lung adenocarcinoma. Mol Oncol 2020;14:917-32. [Crossref] [PubMed]
- Stankovic B, Bjørhovde HAK, Skarshaug R, et al. Immune Cell Composition in Human Non-small Cell Lung Cancer. Front Immunol 2018;9:3101. [Crossref] [PubMed]
- Itoh H, Kadomatsu T, Tanoue H, et al. TET2-dependent IL-6 induction mediated by the tumor microenvironment promotes tumor metastasis in osteosarcoma. Oncogene 2018;37:2903-20. [Crossref] [PubMed]
- Qu Y, Cheng B, Shao N, et al. Prognostic value of immune-related genes in the tumor microenvironment of lung adenocarcinoma and lung squamous cell carcinoma. Aging (Albany NY) 2020;12:4757-77. [Crossref] [PubMed]
- Deng Y, Song Z, Huang L, et al. Tumor purity as a prognosis and immunotherapy relevant feature in cervical cancer. Aging (Albany NY) 2021;13:24768-85. [Crossref] [PubMed]
(English Language Editor: J. Jones)