Integrating single-cell and bulk RNA sequencing to develop a cancer-associated fibroblast-related signature for immune infiltration prediction and prognosis in lung adenocarcinoma
Highlight box
Key findings
• We constructed a novel signature based on the cancer-associated fibroblasts marker genes. The novel prognostic signature had powerful predictive capability for the out-comes of patients with lung adenocarcinoma. Importantly, it was closely correlated with oncogenic signaling pathways and immune infiltration status of the tumor mi-croenvironment.
What is known and what is new?
• Lung cancer is one of the most commonly diagnosed cancers. CAFs can have an im-portant impact on lung carcinogenesis, metastasis, invasion, and immune evasion.
• We constructed and validated a prognostic signature based on 11 CAF marker genes via integration of single cell RNA-sequencing (scRNA-seq) and bulk RNA-sequencing analysis, which was useful for prognosis and estimation of immune infil-tration in LUAD.
What is the implication, and what should change now?
• We developed a biomarker-based prognostic tool to predict outcome and immuno-therapy impact which may facilitate individualized treatment and improved progno-sis. However, the predictive power of this tool should be evaluated in large, prospec-tive clinical studies.
Introduction
Lung cancer is responsible for 18% of cancer-related mortality worldwide, making it the leading cause of death due to cancer (1). Based on histology, lung cancer is broadly divided into non-small cell lung cancer (NSCLC) and small-cell lung cancer (SCLC). Approximately 85% of lung cancer cases are NSCLCs, of which lung adenocarcinoma (LUAD) is the most common type (2). Over the last two decades, research into the understanding of the etiology and management of LUAD has made considerable progress, with targeted and immunotherapies providing a basis for the rational design of treatment regimens. However, most cancers develop resistance to targeted therapy, and only a fraction of patients with LUAD benefit from immunotherapies (3). Furthermore, the 5-year overall survival rate of patients with LUAD remains below 20% (4). Therefore, continued research into prognosis-related biomarkers of LUAD is required to predict treatment effects and improve outcomes.
A growing body of evidence suggests that the tumor microenvironment (TME) is essential in to dynamically orchestrating tumor initiation and progression (5). Moreover, the TME has a crucial impact on clinical outcomes and response to therapeutic interventions (5-7). Among the stromal cells in the TME, cancer-associated fibroblasts (CAFs) are the main cell type of the tumor mesenchyme, which not only play a critical role in promoting tumor invasion and metastasis but also contribute to regulating many immune components (8,9). On the one hand, CAFs exert a direct immunoregulatory effect through the secretome, which affects almost all cell types of innate and acquired immunity. On the other hand, CAFs induce uncontrolled extra matrix remodeling, indirectly disrupting immune cell infiltration into the tumor niche (10). Furthermore, CAFs may figure prominently in lung cancer development via crosstalk with cancer cells (11-14). A study found 11 genes in CAFs that were differentially expressed and associated with prognosis, using microarray gene expression analysis (15). This indicates that changes in oncogenes or tumor suppressor genes in CAFs may have a close relationship with tumor development. Numerous studies indicate that the survival rates of various malignancies are associated with the histological features of CAFs. CAFs are correlated with poor outcomes in patients with LUAD and contribute to therapy resistance (13,16,17). In the case of colorectal cancer, previous research has linked CAFs and pro-fibroblastic responses with an unfavorable prognosis. However, another independent study found that the pro-fibroplastic type, a histologic subtype in CAFs, was a predictor of good prognosis in colorectal cancer (18). Given the roles of CAFs in tumor progression and treatment response, it is worth developing a CAF-related gene signature for LUAD and evaluating its associations with prognosis and the immune infiltration characteristics in the TME.
Recently, the TME has been extensively analyzed through single-cell RNA-sequencing (scRNA-seq). This still emerging technique allows for a finer characterization of tumor, adjacent stromal, and infiltrating immune cells (19,20). Compared with conventional transcriptomic investigation, scRNA-seq can identify specific cell types of the TME, discern the gene expression patterns of each cell, and provides clear insights into the whole tumor ensemble, thus enabling the characterization of various cell types and the identification of marker genes (21,22). Song et al. constructed a 9-gene signature for predicting LUAD prognosis based on 258 B-cell marker genes identified with scRNA-seq analysis, and the signature can serve as a predictor of immunotherapy (23).
In this study, we used scRNA-seq data of LUAD samples from the Gene Expression Omnibus (GEO) database to derive a cluster of CAFs and identify their marker genes. We then developed a prognostic signature based on these CAF marker genes through bulk RNA-seq analysis. Finally, we validated the prediction potential of the signature in 3 GEO cohorts and assessed the prognostic significance by investigating the variation of enriched biological signal pathways, immune cell infiltration characteristics, and immunotherapy response in patients with LUAD with different risk scores. As a result, we found the signature to be closely correlated with oncogenic signaling pathways and the immune infiltration status of the TME, which in turn may influence the clinical outcomes of patients and the response to immunotherapy. We present the following article in accordance with the TRIPOD reporting checklist (available at https://jtd.amegroups.com/article/view/10.21037/jtd-23-238/rc).
Methods
Data source and preprocessing
We downloaded scRNA sequencing data (GSE149655) from the National Center for Biotechnology Information (NCBI) GEO database (https://www.ncbi.nlm.nih.gov/geo/). We downloaded the transcriptome expression profiles of LUAD and matched clinical information from The Cancer Genome Atlas database (TCGA; http://cancergenome.nih.gov/) and GEO database. TCGA database contained 527 LUAD samples and 59 non-LUAD samples. We normalized RNA-seq data to transcripts per million data and then performed log2 conversion. To perform a joint analysis of smooth RNA-seq and clinical data, we removed samples with incomplete clinical information. Ultimately, 500 LUAD samples from TCGA served as the training set. We used the other LUAD samples from the GEO database, including GSE30219 (n=85), GSE31210 (n=226), and GSE72094 (n=398) for further validation. The study was conducted in accordance with the Declaration of Helsinki (as revised in 2013).
Determining the prognosis value of the CAF proportion
We estimated the proportion of CAFs in the samples from the GEO and TCGA data using the EPIC algorithm via the “EPIC” R package (The R Foundation for Statistical Computing). EPIC is one of the tools that calculates the percentage of different cell types in the TME based on RNA-seq data (24). We separated the LUAD samples into a high CAF proportion and a low CAF proportion group based on the optimal cutoff according to the “survminer” R package. To evaluate the prognosis value of the CAF proportion, we conducted a survival analysis using the “survival” and “survminer” R packages. Additionally, we assessed the activity, infiltration levels, and immune-related functions of 19 immune cells through single-sample gene set enrichment analysis (ssGSEA) using the “GSEABase” and “GSVA” R packages (25). Finally, we analyzed the correlations between CAF proportion and the infiltration level of different immune cells or functions using the Wilcoxon test. For all these analyses, we considered P<0.05 to indicate a significant difference.
Identification of CAF marker genes using scRNA-seq analysis
We analyzed scRNA-seq data using the “Seurat” (version 4.0.5) and “SingleR” (version 1.6.1) packages in R software (26,27). We removed the cells with mitochondrial gene content above 5% and mapped genes below 50. We also removed cell clusters with fewer than 3 cells. Next, we conducted principal component analysis (PCA) on the top 2,000 variable expression genes. We applied the t-distributed stochastic neighbor embedding (tSNE) algorithm to visualize the results. Finally, we performed a differential analysis between fibroblasts and other cell types. Based on the differential analysis, we considered genes showing significantly higher expression in fibroblasts as CAF marker genes [log2 fold change (FC) >1 and P<0.05].
Development and validation of a prognostic signature related to CAFs
To find CAF marker genes with significantly different expression levels in LUAD samples and non-LUAD samples, we performed a differential analysis on TCGA data set (using the “limma” R package) with a cutoff of |log2(FC)| >1 and P<0.05 (28). To investigate the prognostic CAF marker genes, we performed univariate Cox regression analyses among differentially expressed CAF marker genes using the “survival” R package. We visualized the results as a forest map using the “forestplot” R package. Subsequently, we conducted least absolute shrinkage and selection operator (LASSO) Cox regression analysis to further reduce the number of variables in the model and construct a signature related to the prognostic CAF marker genes with the following formula:
All the LUAD samples in the GEO and TCGA data sets were separated into a high-risk group and a low-risk group based on the median risk score.
We assessed the overall survival prediction ability of the signature using Kaplan-Meier survival curves, receiver operating characteristic (ROC) curves, and risk curves using the “survminer”, “timeROC”, and “pheatmap” R packages. We further used tSNE and PCA to determine the distribution of different risk groups and estimate the classification ability via the “Rtsne” R package. We also confirmed the overall survival prediction ability of the signature via Kaplan-Meier survival analysis, ROC analysis, risk scatter plot, tSNE, and PCA on the GSE30219, GSE31210, and GSE72094 data sets.
Investigation of the clinical significance of the CAF-related prognostic signature
To investigate whether the risk score is an independent prognostic factor, we performed univariate and multivariate Cox regression analysis on TCGA, GSE30219, GSE31210, and GSE7094 data sets. Next, we visualized these results as a forest map. Additionally, we verified the relationship between risk score and clinicopathological parameters in LUAD samples via the Kruskal-Wallis or Wilcoxon test and visualized the results as box violin plots.
Function and pathway enrichment analysis of the different risk groups
Based on the study of Xiao et al. (29), we established the signature of 5 well-known cancer-related pathways (Table S1), including the cell cycle, Hippo, Myc, Notch, and PI3K pathways, and calculated an enrichment score for each pathway in each LUAD sample with the ssGSEA method. Using the Wilcoxon test, we analyzed the correlation between the pathway enrichment score and risk. Using the “clusterProfiler” R package, we performed gene ontology (GO) enrichment (30). In the results, we focused on GO terms with an adjusted P value <0.01, and to avoid very general terms, we limited the final GO terms lists to the set of pathways with fewer than 300 genes examined for annotation. Meanwhile, we evaluated the similarity between the GO terms using the “GOSemSim” R package and clustered the GO terms by similarity visualized as a tree diagram (using the “ggtree” R package). We obtained 50 gene sets of hallmark pathways described in the molecular signature database (MsigDB database; http://software.broadinstitute.org/gsea/msigdb) via the “msigdbr” R package. To reduce pathway overlap and redundancies, we pruned each pathway-related gene set to keep unique genes and remove all genes related to 2 or more pathways. Subsequently, we performed gene set variation analysis (GSVA) to calculate the enrichment score of each pathway for every LUAD sample via the “GSVA” R package. Next, we performed differential analysis with the “limma” R package to identify the correlation between enrichment scores of hallmark pathways and risk groups and visualize the difference of the pathways with an adjusted P value <0.05 as a heatmap using the “pheatmap” R package. Finally, we conducted GSEA to calculate the enrichment score of Kyoto Encyclopedia of Genes and Genomes (KEGG; http://www.genome.jp/kegg) pathways using the “clusterProfiler” R package.
Evaluation of TME immunological characteristics in LUAD
We used the CIBERSORT, EPIC, MCP-counter, quanTIseq, TIMER, and xCell algorithms to calculate the infiltration level of immune cells in the TME of LUAD (24,31-35). Additionally, we used the Wilcoxon test to assess the association between the infiltration level of immune cells and risk level. We selected the immune cells with P<0.0001 and visualized them as a heatmap. Next, we downloaded hematoxylin and eosin (HE)-stained images of TCGA-LUAD samples from the Genomic Data Commons (GDC; https://portal.gdc.cancer.gov/) and obtained matched immunophenotype pathology of TCGA-LUAD samples from the supplementary research materials of Saltz et al. (36). Additionally, we obtained the list of 129 immunomodulators (Table S2), including chemokines, interleukin, interferons, receptors, and other cytokines from the study of Charoentong et al. (37). The tumor immune dysfunction and exclusion algorithm (TIDE; http://tide.dfci.harvard.edu) by Jiang et al. is based on the combination and modeling of data from 189 human malignant studies. The TIDE score can be used to assess the clinical response to immunotherapy (38). We thus used the TIDE algorithm to calculate the TIDE score of TCGA-LUAD samples and investigated the correlation between the efficacy of immunotherapy and risk score using the Wilcoxon test.
Statistical analysis
We used R software (version 4.1.0) and various R packages to analyze the data and visualize the results. For all analyses, statistical significance was defined as P<0.05.
Results
The clinical significance of the CAF proportion in LUAD
First, we analyzed the clinical significance of the CAF proportion in LUAD. We divided the patients into 2 groups (high CAF and low CAF proportions) according to the optimal cutoff points. Patients with a higher CAF proportion had poor overall survival (GSE30219, GSE31210, and GSE72094; Figure 1A-1C). Moreover, the high CAF groups had lower overall survival rates than did the low CAF groups in TCGA and GSE13213 cohorts, albeit not significantly (P=0.06 and P=0.089; Figure 1D,1E), and poor disease-free survival (GSE31210; Figure 1F). Additionally, the 2 groups differed significantly in immune cell infiltration and immune-related pathway activation, indicating a potential association between the CAF proportion and immune function in LUAD patients (Figure 1G). Taken together, these results suggest that a high CAF proportion was a potential risk factor for LUAD.
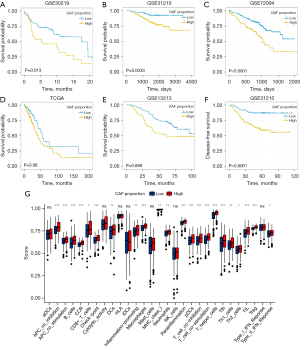
Identification of marker genes associated with CAFs
The screening yielded gene expression profiles for 1546 cells that we used for subsequent analysis from 2 LUAD samples. We performed PCA using the 2000 variable genes to reduce the dimensionality and classify cells into 14 clusters. Subsequently, we adopted tSNE analysis to visualize the results (Figure 2A). We annotated each cell cluster by cross-referencing the differentially expressed genes (DEGs) with the well-known marker genes for each cluster. The following cell types were described: epithelial cells, endothelial cells, fibroblasts, T cells, macrophages, and tissue stem cells (Figure 2B). In addition, we identified significant differential marker genes of each cluster using log2 FC >1 and P<0.05 as thresholds. Figure 2C displays the heatmap for the top 10 significantly expressed marker genes of 6 cell clusters. Ultimately, we screened 417 CAF marker genes in LUAD.
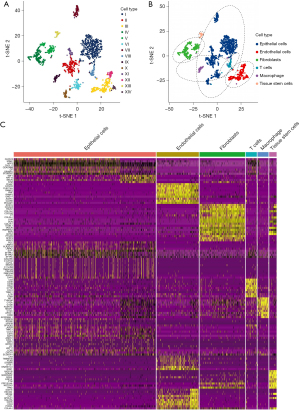
Development and validation of the CAF-related gene signature
In the process of developing a prognostic signature based on CAF marker genes in LUAD, we used TCGA data as a training cohort. We first screened 232 DEGs (47 upregulated and 185 downregulated), using P<0.05 and |log2(FC)| >1 as thresholds (Figure 3A). Next, we performed univariate Cox regression analysis. We identified 81 CAF marker genes distinctly associated with prognostication (Figure S1). We then carried out a LASSO and Cox regression analysis on these genes to select the most valuable ones for prognosis, resulting in a model containing 11 genes: AKAP12, FMO3, KRT8, FSTL3, BMP5, IGHM, SFTPB, TMEM125, CYP4B1, ID1, and IRX2 (Figure 3B). Next, we calculated each patient’s risk score according to Eq. [1].
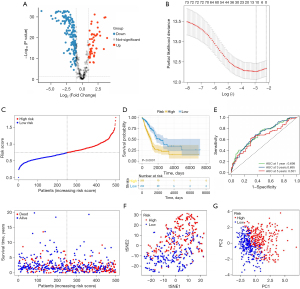
Figure 3C presents the patients’ survival times and risk score distribution. The Kaplan-Meier curve revealed greater survival in low-risk patients with LUAD than in high-risk patients with LUAD in TCGA data set (Figure 3D). To assess the accuracy of the signature, we generated ROC curves for overall survival prediction at 1, 3, and 5 years, which yielded respective area under the ROC curve (AUC) values of 0.698, 0.695, and 0.651 (Figure 3E). Figure 3F,3G present the PCA and tSNE results. Patients with LUAD were separated into high-risk and low-risk groups according to risk score. Next, we divided the patients into subgroups based on clinical characteristics and performed a Kaplan-Meier analysis for each group. The predictive capacity of the signature was further verified in subgroups of different ages, genders, and stages. In all clinical subgroups, the high-risk patients had a poor prognosis (P<0.0001; Figure S2), demonstrating the powerful predictive ability of this prognostic signature.
To further validate the robustness of the CAF-related signature, we assessed its prediction power in 3 independent cohorts (GSE30219, GSE31210 and GSE72094). We calculated the risk scores of samples in each validation data set using the same formula and divided the patients with LUAD into high- and low-risk groups based on the median value. Figure 4A-4C show the distribution of risk scores in the validation data sets, and samples in the high-risk group had poor outcomes. In line with the training cohort, the Kaplan-Meier survival curves revealed that the low-risk group had a superior survival to the high-risk group (Figure 4D-4F). Figure 4G-4I present each cohort’s ROC curves and AUCs. Thus, the prognostic signature had a good prediction ability. Furthermore, the PCA and tSNE allowed the clear distinction of the samples in each cohort (Figure S3). Moreover, we also classified the patients in the GSE31210 and GSE72094 cohorts into different subgroups based on their clinical variables. The Kaplan-Meier overall survival analysis revealed that low-risk scores were associated with better outcomes (Figure S4).
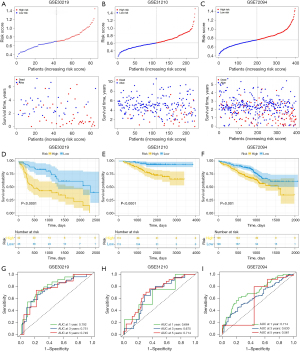
Clinical significance of the prognostic signature in LUAD
Our next step was to evaluate the correlation between this signature and clinical features, such as disease stage, in LUAD patients of the TCGA cohort. Based on the median risk score, we categorized the training cohort patients into high-risk and low-risk groups and produced a heatmap of the gene expression levels (Figure 5A). The scores significantly increased as LUAD stages progressed (Figure 5A,5B). These outcomes revealed that the CAF-related signature and the clinicopathological parameters of patients with LUAD were correlated and confirmed the clinical application value of the prognostic model. We additionally performed 2-step Cox regression analyses (univariate and multivariate) to confirm whether the risk scores were independent of other clinical characteristics, such as age, gender, and stage, in predicting prognosis. As expected, the results from TCGA data set [hazard ratio (HR) =5.913, 95% confidence interval (CI): 3.252–10.751; P=5.67×10−9] and the other 3 cohorts (GSE30219: HR =9.752, 95% CI: 2.065–46.061, P=4.04×10−3; GSE31210: HR =6.915, 95% CI: 1.547–30.900, P=1.14×10−2; GSE72094: HR =14.211, 95% CI: 5.115–39.481, P=3.57×10−7) demonstrated that the risk score was an independent prognostic factor for LUAD (Figure 5C,5D). Overall, these results support the potential clinical utility of the prognostic model.
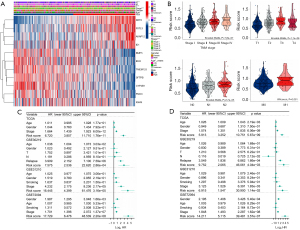
Signal differences between the risk groups
We identified the DEGs between the high-risk and low-risk groups and identified the features of the related signaling pathways. We used the ssGSEA analysis scores derived using the published signature correlated to 5 frequent oncogenic signaling pathways, including the cell cycle, Hippo, Myc, Notch, and PI3K pathways, to compare the 2 groups (29). We found that the pathways had higher levels in the high-risk group (Figure 6A). Then, we assessed the correlation between these pathways and the 11 CAF marker genes. Among them, AKAP12, FSTL3, ID1, and KRT8 were positively correlated with the carcinogen pathways (Figure S5). Moreover, we conducted GO enrichment analysis on these DEGs and grouped them according to functional theme. The analysis revealed that these genes were related to antigen processing and presentation, cell cycle, p53 signaling, T-cell receptor signaling, and regulation of genetic material pathways (Figure 6B). Through functional enrichment analysis, we found that the high-risk group had upregulated levels of cancer- and cell cycle–related gene sets, with terms including “SHEDDEN LUNG CANCER POOR SURVIVAL A6”, “GOBP CELL DIVISION”, and “GOBP MITOTIC CELL CYCLE PROCESS” (Figure S6). Meanwhile, the high-risk group had downregulated levels of gene sets correlated to immune response, with terms including “GOBP ANTIGEN BINDING” and “GOBP ADAPTIVE IMMUNE RESPONSE”.
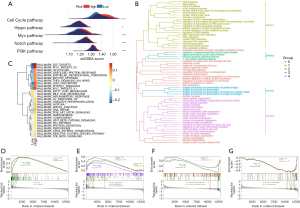
Similarly, the GSVA revealed that the high-risk group had a prevalence of cell cycle and common carcinogen pathways, including terms “MITOTIC SPINDLE”, “G2M CHECKPOINT”, “MYC TARGETS”, “PI3K AKT MTOR SIGNALING”, “WNT BETA CATENIN SIGNALING”, and “TGF BETA SIGNALING” (Figure 6C). Furthermore, the inflammatory pathway termed “INFLAMMATORY RESPONSE” was inhibited in the high-risk group, indicating immunosuppression, which was consistent with our expectations. Figure S7 presents the association between the CAF marker genes and these signaling pathways as a heatmap. The GSEA also confirmed that cell cycle and PI3K-Akt signaling pathways were activated in the high-risk group (Figure 6D,6E). Antigen processing and presentation; cytokine-cytokine receptor interaction; and T helper (Th)1, Th2-, and Th17-cell differentiation was significantly inhibited in the high-risk group (Figure 6F,6G). These results suggest that the prognostic signature was positively correlated with tumor progression-related pathways and negatively associated with immune-related pathways, further demonstrating the value of the signature in predicting clinical outcomes of patients with LUAD.
Correlation between TME immune cell infiltration characteristics and the CAF marker genes
Given the pivotal role of CAFs in the immune regulation of the TME and the association of the prognostic signature with immune-related biological pathways, we next investigated the relationship between the signature and immune cell infiltration in LUAD. We first used the CIBERSORT, EPIC, MCP-counter, quanTIseq, TIMER, and xCell algorithms to assess the proportion of immune cells infiltrating LUAD samples. Most immune cells with antitumor activity, including CD8+ T cells, B cells, monocytes, neutrophils, and myeloid dendric cells, had higher levels of infiltration in the low-risk group (Figure 7A). We next identified the relationships between the prognostic signature and the immune cells. We found that most immune cells expressed negative correlations with the 4 genes included in the signature (AKAP12, KRT8, FSTL3, and ID1), which are detrimental to the prognosis of patients with LUAD (Figure S8A). Additionally, immune cell components were positively correlated with the other 7 prognostically favorable genes, consistent with our expectations.
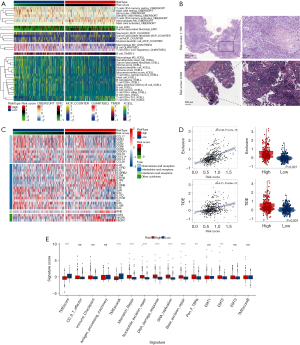
To further explore the correlation between the model and the immune cell infiltration of TME, we used the ESTIMATE algorithm to compare the immune and matrix components in the TME of LUAD. The high-risk group had higher tumor purity but a lower immune score and ESTIMATE score than did the low-risk group (Figure S8B-S8E). These results confirmed the negative correlations between the abundance of immune cell infiltration in the TME and the risk score. Furthermore, we downloaded the pathology slide and compared the levels of immune cell infiltration in the tumor tissue of patients with different risk scores from TCGA data set. Surprisingly, low-risk patients had a higher infiltration of immune cells in their tumor nests (Figure 7B, Figure S9). Moreover, using on the tumor-infiltrating lymphocyte map of TCGA HE pathology slides published by Saltz et al., we compared the discrepancy in infiltrating lymphocytes between patients with different risk scores and found higher levels of lymphocyte infiltration in patients with higher risk scores (36). Given that chemokines are crucial TME components, we analyzed the levels of chemokine mRNA expression in TCGA cohort. Higher chemokine abundance was associated with favorable lung cancer prognoses, with chemokines, including CCL17, CCR2, and CCR4, being more abundant in the low-risk group (Figure 7C). Furthermore, considering the impact of tumor immune infiltration and CAFs on antitumor therapy, we also explored the relationship between this prognostic model and immunotherapy response. We calculated the TIDE score to compare immunotherapy responses in the high-risk and low-risk groups. The high-risk group had higher TIDE and exclusion scores, which may be related to the levels of immune cell infiltration (Figure 7D). Thus, these results confirmed the correlation between this signature and TME immune cell infiltration.
Moreover, we compared the immune-related gene set scores of the different risk groups. The high-risk group had higher scores for epithelial-mesenchymal transition 2 (EMT2), pan-fibroblast TGF-β response signature (Pan-F-TBRs), and DNA repair-related gene sets and lower scores for the TME score gene set (Figure 7E). Thus, the prognostic model was strongly correlated with the level of immune cell infiltration in the TME of patients with LUAD.
Discussion
The essential regulatory role of the TME in cancer development processes, such as cancer cell survival, growth, migration, and even dormancy, is well established (5). Within the TME, CAFs are the most highly represented nonneoplastic stromal cells, and their significant roles in tumor progression have been identified in recent years (39). CAFs play a significant role in tumor progression by secreting various factors, such as growth factors, extracellular matrix proteins, and immunosuppressive ligands, which also contribute to the immunosuppressive effects of the TME (40). In LUAD, CAFs promote cancer progression by enhancing glutamine uptake in LUAD cells through CAF-specific long-chain non-coding RNA LINC01614 packaged in secreted exosomes (41). Furthermore, the reduction of extracellular CLCN3 secretion via HNRNPK knockdown inhibits CAF activation and TGF-β1 production. This, in turn, affects the expression of nuclear HNRNPK and LUAD progression in a feedback loop (42). Due to the apparent heterogeneity of CAF markers, some studies have suggested that CAFs identified by a single or different marker may constitute various subtypes of CAFs with different functional roles in cancer progression. Haichuan Hu and colleagues identified three significant functional subtypes of CAFs in their study, based on distinct expression levels of hepatocyte growth factor (HGF), fibroblast growth factor 7 (FGF7), using PDF libraries. These subtypes exhibited diverse effects, particularly when treated with EGFR and ALK TKI (43). Therefore, reliable biomarkers based on CAFs have attracted an increasing amount of attention. However, CAF-related gene signatures in LUAD studies remain rare. Developing a gene signature based on CAFs in LUAD may help to understand how CAF relates to LUAD outcome, which could help classify patients and tailor therapies.
Recent applications of scRNA-seq have provided an objective characterization of TME cells and clear insights into the whole tumor ecosystem. In this study, we first confirmed that the proportion of CAFs in patients with LUAD was strongly correlated with survival and immune status in these patients. Next, using scRNA-seq analysis, we explored the CAF marker genes in LUAD that could not be distinguished in bulk RNA sequencing. Using the CAFs marker genes, we constructed a new prognostic signature for patients with LUAD in TCGA data set and validated it in 3 independent GEO cohorts and different clinical subgroups. We then confirmed that the signature was a reliable risk predictor. A comparison of the levels of enriched biological pathways and the immune cell infiltration of the high- and low-risk subgroups showed that the risk scores were positively correlated with the activation of oncogenic biological pathways and negatively correlated with the activation of immune-related pathways and levels of immune cell infiltration in the TME. In addition to tumor growth, invasion, and metastasis, CAFs also affect tumor treatment resistance (44). In gastric cancer, CAFs are able to secrete the exosome miR-522, which inhibits iron death in cancer cells by targeting ALOX15, thereby enhancing chemotherapy resistance (45). In HNSCC, CAFs are also involved in influencing the therapeutic efficacy of cetuximab, an effect that is strongly associated with TGF-β signaling (46). In this study, high-risk LUAD patients had higher TIDE and exclusion scores than did the low-risk patients, suggesting a higher immunotherapy response rate in the low-risk group. Further validation indicated that the signature could help determine LUAD prognosis, assess immune infiltration in the TME, and predict the effects of immunotherapy. An important research objective is to stratify the subgroup of high-risk patients with poor outcomes or low overall survival times after curative surgery for early-stage lung adenocarcinoma (LUAD). In this study, all the LUAD patients in the validation dataset (GSE30219) were at TNM stage I. Using the CAF-related signature developed in this study, we were able to categorize patients into high- and low-risk groups, which showed a significant difference in overall survival (P<0.0001). Therefore, we propose that this signature has the potential to identify a high-risk subgroup of early-stage LUAD patients, where the differential expression of the 11 genes involved in the signature may underlie the aggressive phenotype of early-stage LUAD.
The biological functions of the 11 CAF marker genes have been studied. Among them, KRT8, FSTL3, ID1, and AKAP12 are associated with unfavorable outcomes. KRT8 is a type II basic intermediate filament protein; elevated KRT8 levels have been reported in multiple human cancer types (47). KRT8 has been further shown to enhance the proliferation, migration, and invasion ability of lung cancer cells and is significantly associated with survival in LUAD (48,49). FSTL3, an established oncogene, participates in the development and progression of NSCLC (50). Yang et al. confirmed that FSTL3 activates EMT, promotes the polarization of macrophages and fibroblasts, and exhausts T cells (51). Moreover, a significant correlation was found between FSTL3 expression and immune and stromal components of TMEs (51); this is in line with the findings related to our signature, which was strongly related to immune-related pathways and the level of immune cell infiltration. ID1 belongs to the helix-loop-helix (HLH) family and is a dominant negative regulator of transcription factors of the basic HLH family (52). As a direct downstream effector of the BMP/Smad pathway, ID1 has a proangiogenic effect. In addition, it exerts antitumor immunosuppressive effects by inhibiting dendritic cell differentiation and CD8+ T cell proliferation (53,54). Baraibar et al. showed that programmed cell death protein 1 (PD-1) blockade combined with ID1 inhibition increased the infiltration of CD8+ T cells and their programmed death-ligand 1 (PD-L1) expression, thereby significantly enhancing the immunotherapeutic effect (55). This result explains the lower CD8+ T lymphocyte infiltration in the high-risk group. AKAP12 belongs to the kinase-anchored protein family (56). In LUAD, however, the role of AKAP12 remains unclear. Chang et al. reported that AKAP12 expression was upregulated in LUAD, and its high expression was related to tumor progression and poor prognosis (57).
The remaining 7 genes exerted beneficial impacts on the outcome of patients with LUAD. Although FMO3 has rarely been studied in lung cancer, it does increase apoptosis and reduce cell viability in hepatocellular carcinoma cells (58). BMP5 belongs to the transforming growth factor-β superfamily and participates in PinX1-related cell proliferation and cell cycle transition (59). Breast CAFs express the BMP antagonist GREM1, which promotes the mesenchymal phenotype, stemness, and invasion of cancer cells (60). Moreover, it is downregulated in LUAD and correlated with poor prognosis (61). IGHM is an immune-related gene encoding the C region of the µ heavy chain and defines its isoform (62). It significantly affects the density of infiltrating CD20+ B cells in tumor tissues (63). In addition, Pocha et al. revealed that a high expression of surfactant genes, including SFTPB, was associated with intratumoral T-cell infiltration and a low immunosuppressive microenvironment in LUAD (64). As a xenobiotics metabolism enzyme, CYP4B1 has various biological roles. The CYP4B1 mRNA level is low in LUAD and even lower in LUAD at advanced clinical stages (65,66). In lung cancer, TMEM125 and IRX2 have been studied less extensively. IRX2 belongs to the Iroquois homeobox gene family, and there is evidence that LUADs commonly exhibit hypermethylation of the IRX2 promoter region (67). In addition, Fan et al. developed a risk model based on transmembrane proteins, such as TMEM125, and explored its relationship with immune cell infiltration profiles in the TME (68). The specific mechanisms underlying the effects of TMEM125 and IRX on the development of LUAD and its prognosis warrant further investigation.
We additionally examined the biological pathways associated with the CAF-related signature to better understand the mechanism underlying the effects of CAFs on LUAD. We found that common oncogenic pathways, including Hippo, Myc, PI3K, and Notch, as well as signaling pathways involved in cell cycle and DNA replication, were upregulated in the high-risk group. A previous study has reported that activating oncogenic pathways may inhibit immune infiltration (69). Furthermore, the Hippo pathway promotes cancer progression, and the main mediator of this pathway, yes-associated protein (YAP), participates in regulating PD-L1 expression in human NSCLC (70). Furthermore, Janse van Rensburg et al. reported that the Hippo signaling pathway targets PD-L1 and that transcriptional coactivator with PDZ-binding motif (TAZ), a Hippo pathway component, can promote immune evasion via PD-L1 (71). Meanwhile, Myc signaling can enable tumor cells to dysregulate their microenvironment and evade the host immune response (72,73). In addition, Isoyama et al. performed tumor immunotherapy combining a PI3K inhibitor and an anti-PD-1 monoclonal antibody, which suppressed T regulatory cell function and enhanced effector T cell function (74). Regarding the Notch signaling, numerous studies support its significance in immunotherapeutic efficacy (75,76). In agreement with these studies, we found that the following pathways were enriched in the low-risk group: antigen processing and presentation; cytokine–cytokine receptor interaction; and Th1, Th2, and Th17 cell differentiation. This finding prompted us to further investigate the relationship between this signature, the infiltration characteristics of the TME, and the response to immunotherapy.
The interaction of cancer cells and immune microenvironment components also regulate tumor growth and metastasis; thus, it is a crucial element for prognosis (77,78). We applied multiple algorithms to analyze the correlation between the signature and the immune cell infiltration characteristics. Our results show that the prognostic signature is significantly correlated to the immune cell infiltration characteristics in the TME. The low-risk group had higher levels of CD8+ T cells, B cells, monocytes, neutrophils, and myeloid dendric cells than did the high-risk group. Tumor-infiltrating B cells have a distinct role in antitumor immunity. They can differentiate into plasma cells and produce antibodies that recognize tumor-associated antigens and generate antitumor responses (79). Dendric cells participate in antigen presentation, CD4+ T cell differentiation, and natural killer cell recruitment; they are essential for efficient antitumor immunity (80). We also found that the high-risk group had higher infiltrating CD4+ Th2 cell levels than did the low-risk group. In another study, the imbalance in the Th1:Th2 cell ratio was associated with overall survival in patients with lung cancer (81). Tumors with poor infiltration immune cells are described as “cold tumors” and are invariably associated with poor prognosis (78). Tumor-infiltrating lymphocytes are usually considered good prognostic tools—even superior to tumor stage in colon cancer (82). We compared the lymphocyte infiltration levels of the 2 risk groups using HE images of TCGA LUAD samples and confirmed that they were higher in the low-risk group, which may be one of the reasons for the better outcome in low-risk patients.
Cancer immunotherapies target the host immune response to fight the cancer. Because intratumoral CD8+ T lymphocyte infiltration is critical to the success of immune checkpoint inhibitor therapy (83), we hypothesized that this prognostic signature could also help to predict the response to immune checkpoint inhibitor therapy in patients with LUAD. Using the TIDE algorithm, we found that high-risk patients had higher TIDE and exclusion scores, suggesting that immunotherapy is less effective in high-risk patients. Overall, low-risk patients had higher immune infiltration levels in the TME and reaped more benefits from immunotherapy.
This study has several limitations. First, it was based on a public data set, and confirming the predictive power of the obtained signature requires a large prospective clinical study. Second, the potential molecular mechanisms by which these CAF marker genes affect patient outcomes and immune infiltration need to be elucidated through further experimental studies.
Conclusions
We constructed and validated a prognostic signature based on 11 CAF marker genes by integrating scRNA and bulk RNA-seq analysis. In patients with LUAD, the signature could predict the outcome and immune infiltration characteristics well. Our study may provide a biomarker-based prognostic tool to predict outcome and immunotherapy impact, thus enhancing individualized treatment and improving prognosis.
Acknowledgments
We are very grateful to the GEO and TCGA databases for providing their platforms.
Funding: This research was funded by National Natural Science Foundation of China (No. 82172692).
Footnote
Reporting Checklist: The authors have completed the TRIPOD reporting checklist. Available at https://jtd.amegroups.com/article/view/10.21037/jtd-23-238/rc
Peer Review File: Available at https://jtd.amegroups.com/article/view/10.21037/jtd-23-238/prf
Conflicts of Interest: All authors have completed the ICMJE uniform disclosure form (available at https://jtd.amegroups.com/article/view/10.21037/jtd-23-238/coif). The authors have no conflicts of interest to declare.
Ethical Statement: The authors are accountable for all aspects of the work in ensuring that questions related to the accuracy or integrity of any part of the work are appropriately investigated and resolved. The study was conducted in accordance with the Declaration of Helsinki (as revised in 2013).
Open Access Statement: This is an Open Access article distributed in accordance with the Creative Commons Attribution-NonCommercial-NoDerivs 4.0 International License (CC BY-NC-ND 4.0), which permits the non-commercial replication and distribution of the article with the strict proviso that no changes or edits are made and the original work is properly cited (including links to both the formal publication through the relevant DOI and the license). See: https://creativecommons.org/licenses/by-nc-nd/4.0/.
References
- Sung H, Ferlay J, Siegel RL, et al. Global Cancer Statistics 2020: GLOBOCAN Estimates of Incidence and Mortality Worldwide for 36 Cancers in 185 Countries. CA Cancer J Clin 2021;71:209-49. [Crossref] [PubMed]
- Thai AA, Solomon BJ, Sequist LV, et al. Lung cancer. Lancet 2021;398:535-54. [Crossref] [PubMed]
- Herbst RS, Morgensztern D, Boshoff C. The biology and management of non-small cell lung cancer. Nature 2018;553:446-54. [Crossref] [PubMed]
- Lin JJ, Cardarella S, Lydon CA, et al. Five-Year Survival in EGFR-Mutant Metastatic Lung Adenocarcinoma Treated with EGFR-TKIs. J Thorac Oncol 2016;11:556-65. [Crossref] [PubMed]
- Bejarano L, Jordāo MJC, Joyce JA. Therapeutic Targeting of the Tumor Microenvironment. Cancer Discov 2021;11:933-59. [Crossref] [PubMed]
- Klemm F, Joyce JA. Microenvironmental regulation of therapeutic response in cancer. Trends Cell Biol 2015;25:198-213. [Crossref] [PubMed]
- Binnewies M, Roberts EW, Kersten K, et al. Understanding the tumor immune microenvironment (TIME) for effective therapy. Nat Med 2018;24:541-50. [Crossref] [PubMed]
- Chen Y, McAndrews KM, Kalluri R. Clinical and therapeutic relevance of cancer-associated fibroblasts. Nat Rev Clin Oncol 2021;18:792-804. [Crossref] [PubMed]
- Mao X, Xu J, Wang W, et al. Crosstalk between cancer-associated fibroblasts and immune cells in the tumor microenvironment: new findings and future perspectives. Mol Cancer 2021;20:131. [Crossref] [PubMed]
- Liu T, Han C, Wang S, et al. Cancer-associated fibroblasts: an emerging target of anti-cancer immunotherapy. J Hematol Oncol 2019;12:86. [Crossref] [PubMed]
- Sato R, Imamura K, Semba T, et al. TGFβ Signaling Activated by Cancer-Associated Fibroblasts Determines the Histological Signature of Lung Adenocarcinoma. Cancer Res 2021;81:4751-65. [Crossref] [PubMed]
- Lee S, Hong JH, Kim JS, et al. Cancer-associated fibroblasts activated by miR-196a promote the migration and invasion of lung cancer cells. Cancer Lett 2021;508:92-103. [Crossref] [PubMed]
- Zhang H, Jiang H, Zhu L, et al. Cancer-associated fibroblasts in non-small cell lung cancer: Recent advances and future perspectives. Cancer Lett 2021;514:38-47. [Crossref] [PubMed]
- Domen A, Quatannens D, Zanivan S, et al. Cancer-Associated Fibroblasts as a Common Orchestrator of Therapy Resistance in Lung and Pancreatic Cancer. Cancers (Basel) 2021;13:987. [Crossref] [PubMed]
- Navab R, Strumpf D, Bandarchi B, et al. Prognostic gene-expression signature of carcinoma-associated fibroblasts in non-small cell lung cancer. Proc Natl Acad Sci U S A 2011;108:7160-7165. [Crossref] [PubMed]
- Alcaraz J, Carrasco JL, Millares L, et al. Stromal markers of activated tumor associated fibroblasts predict poor survival and are associated with necrosis in non-small cell lung cancer. Lung Cancer 2019;135:151-60. [Crossref] [PubMed]
- Yotsukura M, Asamura H, Suzuki S, et al. Prognostic impact of cancer-associated active fibroblasts and invasive architectural patterns on early-stage lung adenocarcinoma. Lung Cancer 2020;145:158-66. [Crossref] [PubMed]
- Min K-W, Kim D-H, Noh Y-K, et al. Cancer-associated fibroblasts are associated with poor prognosis in solid type of lung adenocarcinoma in a machine learning analysis. Sci Rep 2021;11:16779. [Crossref] [PubMed]
- Kim N, Kim HK, Lee K, et al. Single-cell RNA sequencing demonstrates the molecular and cellular reprogramming of metastatic lung adenocarcinoma. Nat Commun 2020;11:2285. [Crossref] [PubMed]
- Wu F, Fan J, He Y, et al. Single-cell profiling of tumor heterogeneity and the microenvironment in advanced non-small cell lung cancer. Nat Commun 2021;12:2540. [Crossref] [PubMed]
- Zhou Y, Yang D, Yang Q, et al. Single-cell RNA landscape of intratumoral heterogeneity and immunosuppressive microenvironment in advanced osteosarcoma. Nat Commun 2020;11:6322. [Crossref] [PubMed]
- Maynard A, McCoach CE, Rotow JK, et al. Therapy-Induced Evolution of Human Lung Cancer Revealed by Single-Cell RNA Sequencing. Cell 2020;182:1232-1251.e22. [Crossref] [PubMed]
- Song P, Li W, Wu X, et al. Integrated analysis of single-cell and bulk RNA-sequencing identifies a signature based on B cell marker genes to predict prognosis and immunotherapy response in lung adenocarcinoma. Cancer Immunol Immunother 2022;71:2341-54. [Crossref] [PubMed]
- Racle J, Gfeller D. EPIC: A Tool to Estimate the Proportions of Different Cell Types from Bulk Gene Expression Data. Methods Mol Biol 2020;2120:233-48. [Crossref] [PubMed]
- Hänzelmann S, Castelo R, Guinney J. GSVA: gene set variation analysis for microarray and RNA-seq data. BMC Bioinformatics 2013;14:7. [Crossref] [PubMed]
- Hao Y, Hao S, Andersen-Nissen E, et al. Integrated analysis of multimodal single-cell data. Cell 2021;184:3573-3587.e29. [Crossref] [PubMed]
- Aran D, Looney AP, Liu L, et al. Reference-based analysis of lung single-cell sequencing reveals a transitional profibrotic macrophage. Nat Immunol 2019;20:163-72. [Crossref] [PubMed]
- Ritchie ME, Phipson B, Wu D, et al. limma powers differential expression analyses for RNA-sequencing and microarray studies. Nucleic Acids Res 2015;43:e47. [Crossref] [PubMed]
- Xiao Y, Ma D, Zhao S, et al. Multi-Omics Profiling Reveals Distinct Microenvironment Characterization and Suggests Immune Escape Mechanisms of Triple-Negative Breast Cancer. Clin Cancer Res 2019;25:5002-14. [Crossref] [PubMed]
- Yu G, Wang LG, Han Y, et al. clusterProfiler: an R package for comparing biological themes among gene clusters. OMICS 2012;16:284-7. [Crossref] [PubMed]
- Newman AM, Liu CL, Green MR, et al. Robust enumeration of cell subsets from tissue expression profiles. Nat Methods 2015;12:453-7. [Crossref] [PubMed]
- Becht E, Giraldo NA, Lacroix L, et al. Estimating the population abundance of tissue-infiltrating immune and stromal cell populations using gene expression. Genome Biol 2016;17:218. [Crossref] [PubMed]
- Finotello F, Mayer C, Plattner C, et al. Molecular and pharmacological modulators of the tumor immune contexture revealed by deconvolution of RNA-seq data. Genome Med 2019;11:34. [Crossref] [PubMed]
- Li B, Severson E, Pignon JC, et al. Comprehensive analyses of tumor immunity: implications for cancer immunotherapy. Genome Biol 2016;17:174. [Crossref] [PubMed]
- Aran D, Hu Z, Butte AJ. xCell: digitally portraying the tissue cellular heterogeneity landscape. Genome Biol 2017;18:220. [Crossref] [PubMed]
- Saltz J, Gupta R, Hou L, et al. Spatial Organization and Molecular Correlation of Tumor-Infiltrating Lymphocytes Using Deep Learning on Pathology Images. Cell Rep 2018;23:181-193.e7. [Crossref] [PubMed]
- Charoentong P, Finotello F, Angelova M, et al. Pan-cancer Immunogenomic Analyses Reveal Genotype-Immunophenotype Relationships and Predictors of Response to Checkpoint Blockade. Cell Rep 2017;18:248-62. [Crossref] [PubMed]
- Jiang P, Gu S, Pan D, et al. Signatures of T cell dysfunction and exclusion predict cancer immunotherapy response. Nat Med 2018;24:1550-8. [Crossref] [PubMed]
- Hu B, Wu C, Mao H, et al. Subpopulations of cancer-associated fibroblasts link the prognosis and metabolic features of pancreatic ductal adenocarcinoma. Annals of Translational Medicine 2022;10:262. [Crossref] [PubMed]
- Biffi G, Tuveson DA. Diversity and Biology of Cancer-Associated Fibroblasts. Physiol Rev 2021;101:147-76. [Crossref] [PubMed]
- Liu T, Han C, Fang P, et al. Cancer-associated fibroblast-specific lncRNA LINC01614 enhances glutamine uptake in lung adenocarcinoma. J Hematol Oncol 2022;15:141. [Crossref] [PubMed]
- Li Y, Yang Y, Ma Q, et al. HNRNPK/CLCN3 axis facilitates the progression of LUAD through CAF-tumor interaction. Int J Biol Sci 2022;18:6084-6101. [Crossref] [PubMed]
- Hu H, Piotrowska Z, Hare PJ, et al. Three subtypes of lung cancer fibroblasts define distinct therapeutic paradigms. Cancer Cell 2021;39:1531-1547.e10. [Crossref] [PubMed]
- Zhang J, Zhang N, Fu X, et al. Bioinformatic analysis of cancer-associated fibroblast related gene signature as a predictive model in clinical outcomes and immune characteristics of gastric cancer. Annals of Translational Medicine 2022;10:698. [Crossref] [PubMed]
- Zhang H, Deng T, Liu R, et al. CAF secreted miR-522 suppresses ferroptosis and promotes acquired chemo-resistance in gastric cancer. Mol Cancer 2020;19:43. [Crossref] [PubMed]
- Yegodayev KM, Novoplansky O, Golden A, et al. TGF-Beta-Activated Cancer-Associated Fibroblasts Limit Cetuximab Efficacy in Preclinical Models of Head and Neck Cancer. Cancers (Basel) 2020;12:339. [Crossref] [PubMed]
- Fang J, Wang H, Liu Y, et al. High KRT8 expression promotes tumor progression and metastasis of gastric cancer. Cancer Sci 2017;108:178-86. [Crossref] [PubMed]
- Chen H, Chen X, Pan B, et al. KRT8 Serves as a Novel Biomarker for LUAD and Promotes Metastasis and EMT via NF-κB Signaling. Front Oncol 2022;12:875146. [Crossref] [PubMed]
- Xie L, Dang Y, Guo J, et al. High KRT8 Expression Independently Predicts Poor Prognosis for Lung Adenocarcinoma Patients. Genes (Basel) 2019;10:36. [Crossref] [PubMed]
- Liu YJ, Li JP, Zhang Y, et al. FSTL3 is a Prognostic Biomarker in Gastric Cancer and is Correlated with M2 Macrophage Infiltration. Onco Targets Ther 2021;14:4099-117. [Crossref] [PubMed]
- Yang C, Cao F, Huang S, et al. Follistatin-Like 3 Correlates With Lymph Node Metastasis and Serves as a Biomarker of Extracellular Matrix Remodeling in Colorectal Cancer. Front Immunol 2021;12:717505. [Crossref] [PubMed]
- Perk J, Iavarone A, Benezra R. Id family of helix-loop-helix proteins in cancer. Nat Rev Cancer 2005;5:603-14. [Crossref] [PubMed]
- Huang L, Zeng H, Jin C, et al. Sulfated galactoglucan impedes xenografted lung cancer cell growth by blocking angiogenesis via binding BMPRs. Carbohydr Polym 2022;289:119412. [Crossref] [PubMed]
- Papaspyridonos M, Matei I, Huang Y, et al. Id1 suppresses anti-tumour immune responses and promotes tumour progression by impairing myeloid cell maturation. Nat Commun 2015;6:6840. [Crossref] [PubMed]
- Baraibar I, Roman M, Rodríguez-Remírez M, et al. Id1 and PD-1 Combined Blockade Impairs Tumor Growth and Survival of KRAS-mutant Lung Cancer by Stimulating PD-L1 Expression and Tumor Infiltrating CD8(+) T Cells. Cancers (Basel) 2020;12:3169. [Crossref] [PubMed]
- Wu X, Wu T, Li K, et al. The Mechanism and Influence of AKAP12 in Different Cancers. Biomed Environ Sci 2018;31:927-32. [PubMed]
- Chang J, Liu S, Li B, et al. MiR-338-3p improved lung adenocarcinoma by AKAP12 suppression. Arch Med Sci 2021;17:462-73. [Crossref] [PubMed]
- Hlady RA, Sathyanarayan A, Thompson JJ, et al. Integrating the Epigenome to Identify Drivers of Hepatocellular Carcinoma. Hepatology 2019;69:639-52. [Crossref] [PubMed]
- Xu Z, Chen C. Abnormal Expression and Prognostic Significance of Bone Morphogenetic Proteins and Their Receptors in Lung Adenocarcinoma. Biomed Res Int 2021;2021:6663990. [Crossref] [PubMed]
- Ren J, Smid M, Iaria J, et al. Cancer-associated fibroblast-derived Gremlin 1 promotes breast cancer progression. Breast Cancer Res 2019;21:109. [Crossref] [PubMed]
- Deng T, Lin D, Zhang M, et al. Differential expression of bone morphogenetic protein 5 in human lung squamous cell carcinoma and adenocarcinoma. Acta Biochim Biophys Sin (Shanghai) 2015;47:557-63. [Crossref] [PubMed]
- Lee H, Kwon MJ, Koo BM, et al. A novel immune prognostic index for stratification of high-risk patients with early breast cancer. Sci Rep 2021;11:128. [Crossref] [PubMed]
- Lu Z, Gao Y. Screening differentially expressed genes between endometriosis and ovarian cancer to find new biomarkers for endometriosis. Ann Med 2021;53:1377-89. [Crossref] [PubMed]
- Pocha K, Mock A, Rapp C, et al. Surfactant Expression Defines an Inflamed Subtype of Lung Adenocarcinoma Brain Metastases that Correlates with Prolonged Survival. Clin Cancer Res 2020;26:2231-43. [Crossref] [PubMed]
- Liu J, Jia J, Wang S, et al. Prognostic Ability of Enhancer RNAs in Metastasis of Non-Small Cell Lung Cancer. Molecules 2022;27:4108. [Crossref] [PubMed]
- Zhang D, Jiang Q, Ge X, et al. RHOV promotes lung adenocarcinoma cell growth and metastasis through JNK/c-Jun pathway. Int J Biol Sci 2021;17:2622-32. [Crossref] [PubMed]
- Sato T, Arai E, Kohno T, et al. Epigenetic clustering of lung adenocarcinomas based on DNA methylation profiles in adjacent lung tissue: Its correlation with smoking history and chronic obstructive pulmonary disease. Int J Cancer 2014;135:319-34. [Crossref] [PubMed]
- Fan T, Liu Y, Liu H, et al. Transmembrane Protein-Based Risk Model and H3K4me3 Modification Characteristics in Lung Adenocarcinoma. Front Oncol 2022;12:828814. [Crossref] [PubMed]
- Zhao X, Subramanian S. Oncogenic pathways that affect antitumor immune response and immune checkpoint blockade therapy. Pharmacol Ther 2018;181:76-84. [Crossref] [PubMed]
- Hsu PC, Jablons DM, Yang CT, et al. Epidermal Growth Factor Receptor (EGFR) Pathway, Yes-Associated Protein (YAP) and the Regulation of Programmed Death-Ligand 1 (PD-L1) in Non-Small Cell Lung Cancer (NSCLC). Int J Mol Sci 2019;20:3821. [Crossref] [PubMed]
- Janse van Rensburg HJ, Azad T, Ling M, et al. The Hippo Pathway Component TAZ Promotes Immune Evasion in Human Cancer through PD-L1. Cancer Res 2018;78:1457-70. [Crossref] [PubMed]
- Schaafsma E, Zhao Y, Zhang L, et al. MYC Activity Inference Captures Diverse Mechanisms of Aberrant MYC Pathway Activation in Human Cancers. Mol Cancer Res 2021;19:414-28. [Crossref] [PubMed]
- Dhanasekaran R, Deutzmann A, Mahauad-Fernandez WD, et al. The MYC oncogene - the grand orchestrator of cancer growth and immune evasion. Nat Rev Clin Oncol 2022;19:23-36. [Crossref] [PubMed]
- Isoyama S, Mori S, Sugiyama D, et al. Cancer immunotherapy with PI3K and PD-1 dual-blockade via optimal modulation of T cell activation signal. J Immunother Cancer 2021;9:e002279. [Crossref] [PubMed]
- Janghorban M, Xin L, Rosen JM, et al. Notch Signaling as a Regulator of the Tumor Immune Response: To Target or Not To Target? Front Immunol 2018;9:1649. [Crossref] [PubMed]
- Sierra RA, Trillo-Tinoco J, Mohamed E, et al. Anti-Jagged Immunotherapy Inhibits MDSCs and Overcomes Tumor-Induced Tolerance. Cancer Res 2017;77:5628-38. [Crossref] [PubMed]
- Remark R, Becker C, Gomez JE, et al. The non-small cell lung cancer immune contexture. A major determinant of tumor characteristics and patient outcome. Am J Respir Crit Care Med 2015;191:377-90. [Crossref] [PubMed]
- Galon J, Bruni D. Approaches to treat immune hot, altered and cold tumours with combination immunotherapies. Nat Rev Drug Discov 2019;18:197-218. [Crossref] [PubMed]
- Wang SS, Liu W, Ly D, et al. Tumor-infiltrating B cells: their role and application in anti-tumor immunity in lung cancer. Cell Mol Immunol 2019;16:6-18. [Crossref] [PubMed]
- Wculek SK, Cueto FJ, Mujal AM, et al. Dendritic cells in cancer immunology and immunotherapy. Nat Rev Immunol 2020;20:7-24. [Crossref] [PubMed]
- Basu A, Ramamoorthi G, Albert G, et al. Differentiation and Regulation of T(H) Cells: A Balancing Act for Cancer Immunotherapy. Front Immunol 2021;12:669474. [Crossref] [PubMed]
- Galon J, Mlecnik B, Bindea G, et al. Towards the introduction of the 'Immunoscore' in the classification of malignant tumours. J Pathol 2014;232:199-209. [Crossref] [PubMed]
- Rosenbaum SR, Wilski NA, Aplin AE. Fueling the Fire: Inflammatory Forms of Cell Death and Implications for Cancer Immunotherapy. Cancer Discov 2021;11:266-81. [Crossref] [PubMed]
(English Language Editor: J. Gray)