A semiautomated radiomics model based on multimodal dual-layer spectral CT for preoperative discrimination of the invasiveness of pulmonary ground-glass nodules
Highlight box
Key findings
• Spectral CT multimodal imaging combined with multimodal radiomics can be a non-invasive, robust, and reproducible method for the preoperative identification of GGNs invasiveness, which can help clinicians select the appropriate intervention and management for patients with pulmonary nodules before surgery.
What is known and what is new?
• Spectral CT can help differentiate the invasiveness of lung adenocarcinoma presenting as ground-glass nodules.
• A multimodal radiomics model of dual-layer spectral CT was able to discriminate invasiveness of lung adenocarcinoma presenting as ground-glass nodules well.
What does this mean, and what should change now?
• For patients with ground-glass nodules on CT who are highly suspected clinically of lung adenocarcinoma, the multimodal radiomics model of dual-layer spectral CT should be used to predict their invasiveness before operation, which can be used to predict clinically. Advise on the choice of format.
Introduction
With the widespread use of low-dose computed tomography (LDCT) for lung screening, the detection rate of pulmonary ground-glass nodules (GGNs) has increased significantly (1). GGNs include pure ground-glass nodules (pGGNs) without solid components and mixed ground-glass nodules (mGGNs) with solid components (2,3), which are mainly characterized by hazy increased density lung parenchyma but with the presentation of bronchial and vascular margins on computed tomography (CT). According to the new classification system for lung adenocarcinoma jointly released by the International Association for the Study of Lung Cancer, the American Thoracic Society, and the European Respiratory Society in 2011, lung adenocarcinomas are classified into preinvasive adenocarcinoma (PIA), minimally invasive adenocarcinoma (MIA), and invasive adenocarcinoma (IA), with PIA being subdivided into atypical adenomatous hyperplasia (AAH) and adenocarcinoma in situ (AIS) (4). The current standard of clinical treatment for IA is still lobectomy, but for patients with PIA or MIA, segmentectomy or wedge resection can be selected in order to maintain the function of the lungs to a greater extent. Studies have shown that patients of AIS or MIA having received appropriate surgical resection have a disease-free survival rate of 100% or close to 100% (5-7), and the five-year survival rate of localized IA is 70–90% (8,9). But for cases of not having the appropriate treatment in time, the median survival time of early-stage patients is only 13 months (10). However, excessive pneumonectomy can also cause unnecessary damage to the function of the lungs (11,12), so accurate preoperative differentiation of the invasiveness of GGNs is critical to the outcomes and survival rates. Studies have shown that the ratio of solid components is positively correlated with the malignancy of GGNs (13,14), but there are still 10.8% of pGGNs and 80.0% of ground-glass opacity (GGO)-predominant mGGNs pathologically diagnosed as IA (15). As such, determining patient adenocarcinoma subtypes based on the ratio of solid components may be unreliable.
Currently, the determination of whether GGNs are invasive or not is a controversial and difficult issue. Pathological biopsy is invasive and hard to palpate, and the appearance of lesions on conventional CT is not absolutely correlated with malignant invasiveness (16,17). AAH, AIS, MIA, and IA may all manifest as pGGNs (13,18,19). Enhancement of nodules on contrast-enhanced CT is generally considered to be associated with increased capillary perfusion and permeability (20,21), but it has a limited role in GGNs with deficient or few solid components (21). Compared with conventional CT, spectral CT can provide more modalities with quantitative imaging information which is helpful for diagnoses, such as iodine density (ID) map, effective atomic number (Zeff) map, electron density (ED) map, and virtual non-contrast (VNC) map. It has shown excellent performance in the qualitative diagnosis of tumors (e.g., pancreatic cancer, gastric cancer, breast cancer, etc.) in recent years (22-24). However, there are limited studies on using spectral CT to differentiate the invasiveness of GGNs. Promisingly, Yu et al. (25) found that CT value in virtual monoenergetic 130 keV images at the venous phase could help to differentiate the preinvasive lesion group from the IA group of GGNs. Qiu et al. (26) found that the ED value could provide more useful information for the differential diagnosis of GGNs invasiveness. The previous studies suggested the effectiveness of spectral CT images in the diagnosis of GGNs invasiveness, however, these studies had not combined radiomics and spectral multimodal imaging. for a comprehensive analysis and investigation. Therefore, this study aimed to establish a diagnostic model of GGNs based on multimodal spectral CT images and radiomics to differentiate the invasiveness of GGNs and provide a basis for making rational clinical decisions. We present this article in accordance with the TRIPOD reporting checklist (available at https://jtd.amegroups.com/article/view/10.21037/jtd-22-1605/rc).
Methods
Study populations
This study was conducted in accordance with the Declaration of Helsinki (as revised in 2013). The study was approved by the Ethics Committee of Union Hospital, Tongji Medical College, Huazhong University of Science and Technology (No. 2020S363) and individual consent for this retrospective analysis was waived. Between May 2020 to May 2022, 352 patients who underwent contrast-enhanced lung scans on a dual-layer detector spectral CT (IQon, Philips Healthcare, Best, the Netherlands) at two hospital sites (Hospital 1: Main Campus, Hospital 2: West Campus) of the Union Hospital were retrospectively analyzed. The inclusion criteria in this study were as follows: (I) patients who had undergone complete surgery and were pathologically confirmed as AAH, AIS, MIA, or IA; (II) the maximum diameter ≤30 mm of nodules and ≤5 mm of solid components measured on lung window CT images with 1 mm slice-thickness (4,19,27); (III) the interval between the latest dual-layer detector spectral CT examination and surgery were within one month. The exclusion criteria are as follows: (I) patients who received chemotherapy or radiotherapy before the latest CT examination (28); (II) patients who had undergone percutaneous lung nodule biopsy or with symptoms of hemoptysis or pulmonary infection before the latest CT examination.
Among these 352 patients, 227 were excluded from this study because of nodules >30 mm in maximum diameter or solid components >5 mm in maximum diameter. Finally, 125 GGNs out of 125 patients met the criteria; 87 GGNs from Hospital 1 were classified as the training set and 38 GGNs from Hospital 2 were classified as the validation set (Figure 1). An overview of the research methodology is shown in Figure 2.
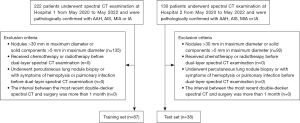
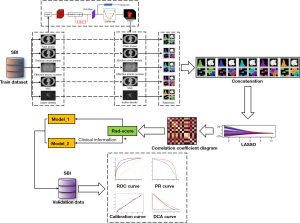
Spectral CT examination
The contrast-enhanced lung CT examinations were performed with a 64-section dual-layer detector spectral CT system. The detailed imaging parameters were as follows: 120 keV, 140–250 mA, 64×0.625 mm² collimation, a pitch of 0.984, rotation time of 0.27 seconds. All patients were injected with non-ionic iodinated contrast material with 350 mg/mL concentration (Iopamidol, Shanghai Bracco Sine Pharmaceutical, Shanghai, China) at a dose of 1.35 mL/kg body weight and a contrast injection rate of 3.0 mL/s by using a power injector (OptiVantage, Tyco Healthcare, USA). All scans started 40 seconds after the beginning of the contrast injection. After scanning, two conventional images were reconstructed using the iDose reconstruction algorithm, and Spectral Base Images (SBI) was reconstructed using the spectral reconstruction algorithm (25). All images were reconstructed with a section thickness of 1 mm, a section increment of 1 mm, and a smooth filter. The reconstructed images were analyzed with a dedicated workstation (IntelliSpace Portal V10, Philips Healthcare).
Multimodal image preprocessing and feature extraction
Considering that the density of GGNs ranged mainly between −750 and −350 HU on conventional CT, normalizing the image directly will reduce the density resolution of the GGNs lesion area (29). Therefore, the Digital Imaging and Communications in Medicine (DICOM) images were first subjected to density normalization, and the window width and window level were normalized to 1,600 and −600 HU, followed by 0–1 normalization to reduce the effects of contrast and brightness on the grayscale values of the GGNs (30,31). The spectral multimodal images (including ID, VNC, ED, and Zeff) were directly processed with 0–1 normalization. Then, the 3D-Region based Convolutional Neural Network (3D-RCNN) pre-trained network was used for the automatic detection of lung nodules; then, the 3D sphere representation-based center-points matching detection network (SCPM-Net) pre-trained convolutional neural network was used for the automatic segmentation of target nodules (32,33).
Radiomic features were extracted from each modal data (ID, VNC, ED, and Zeff) using the PyRadiomics package (34). Extracted features include morphological features and first-order features, details of which are available in Pyradiomics documentation (http://Pyradiomics.readthedocs.io/en/latest/). A detailed list of radiomic features was presented in the Appendix 1.
Feature selection and model construction
The optimal feature selection was performed using a machine-learning least absolute shrinkage and selection operator (LASSO) regression method with cross-validation. Then, the logistic-based rad-score model (radiomics model) was built based on the established optimal feature subsets of the training dataset. In addition, the clinical features that differed significantly between invasive and non-invasive GGNs were selected to combine with rad-score to develop a diagnostic model (joint model). Finally, the receiver operating characteristic (ROC) areas under the curve (AUC) of the two models were performed and compared.
Statistical analysis
Normally distributed continuous variables on the data set were compared using the two-sample t-test, non-normally distributed continuous variables were compared using the Wilcoxon rank sum test, and categorical variables were compared using the chi-square test or Fisher’s exact test. Correlations between features were compared by Spearman correlation. The area under the ROC curve (AUC) and the precision recall (PR) curve were used to evaluate model performance on the training and test sets, and calculate the odds ratio (OR) values of each selected variable through logistic regression. The calibration plots were generated to visualize the consistency of the models. The decision curve analysis (DCA) was used to calculate the net benefit of the model under different threshold probabilities. All statistical analyses from this study were performed using SPSS 26.0, R 3.5.1, and Python 3.5.6. Two-tailed P value <0.05 indicated statistical significance.
Results
Patients
A total of 125 GGNs were detected in 125 patients in this study. Among the 125 GGNs, 45 were IA, 43 were MIA and 37 were PIA (35 were AIS and 2 were AAH). The clinical characteristics of the 125 patients are summarized in Table 1.
Table 1
Variable | PIA or MIA (n=80) | IA (n=45) | P value |
---|---|---|---|
Age (year), n (%) | <0.001* | ||
<53 | 37 (29.6) | 20 (16.0) | |
≥53 | 43 (34.4) | 25 (20.0) | |
Gender, n (%) | 80 (64.0) | 45 (36.0) | <0.001* |
Male | 14 (17.5) | 21 (46.7) | |
Female | 66 (82.5) | 24 (53.3) | |
Smoke, n (%) | 5 (6.3) | 6 (13.3) | 0.201 |
History of malignant tumor or lung cancer family history, n (%) | 4 (5.0) | 2 (4.4) | 1.000 |
Chronic obstructive pulmonary disease, n (%) | 0 | 1 (2.2) | 0.360 |
Tuberculosis, n (%) | 0 | 1 (2.2) | 0.360 |
Occupational exposure, n (%) | 1 (1.3) | 0 | 1.000 |
Lesion location, n (%) | 0.556 | ||
Left upper lobe | 26 (32.5) | 13 (28.9) | |
Left lower lobe | 7 (8.8) | 4 (8.9) | |
Right upper lobe | 32 (40.0) | 15 (33.3) | |
Right middle lobe | 5 (6.3) | 2 (4.4) | |
Right lower lobe | 10 (12.5) | 11 (24.4) |
P values are a comparison between participants with PIA or MIA and IA groups. *, P value <0.05. PIA, adenocarcinoma in situ and atypical adenomatous hyperplasia; IA, invasive adenocarcinoma; MIA, minimally invasive adenocarcinoma.
Feature selection and model construction
Among these features, this study uses the LASSO logistic regression model for dimensionality reduction, and finally, 5 features with non-zero coefficients were selected to establish the rad-score (Figure 3A,3B):
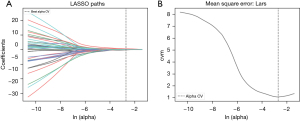
a_ED_original_firstorder_90Percentile comes from the electron cloud density at the arterial phase, a_ID_original_firstorder_Entropy comes from the iodine map at the arterial phase, p_original_shape_Maximum2DDiameterSlice comes from the plain phase, v_ED_original_firstorder_90Percentile comes from the electron cloud density at the venous phase, and v_Zeff_original_firstorder_Uniformity comes from the effective atomic number at the venous phase. The above features were significantly different between IA and PIA/MIA groups (Table 2).
Table 2
Variable | PIA or MIA | IA | P value |
---|---|---|---|
a_ED_original_firstorder_90Percentile | 47.76±13.28a | 61.53±14.90b | <0.001* |
a_ID_original_firstorder_Entropy | 3.99±0.88b | 3.47±0.54b | <0.001* |
p_original_shape_Maximum2DDiameterSlice | 9.92±3.69b | 14.51±4.03b | <0.001* |
v_ED_original_firstorder_90Percentile | 45.48±14.49b | 61.67±15.60b | <0.001* |
v_Zeff_original_firstorder_Uniformity | 0.06±0.02b | 0.08±0.03a | 0.002* |
a, normally distributed data is represented by mean ± SD; b, skewed distributed data is represented by median ± IQR. *, P value <0.05. a_ED_original_firstorder_90Percentile, the electron cloud density at the arterial phase; a_ID_original_firstorder_Entropy, the iodine map at the arterial phase; p_original_shape_Maximum2DDiameterSlice, the plain phase; v_ED_original_firstorder_90Percentile, the electron cloud density at the venous phase; v_Zeff_original_firstorder_Uniformity, the effective atomic number at the venous phase; SD, standard deviation; IQR, interquartile range; PIA, adenocarcinoma in situ and atypical adenomatous hyperplasia; IA, invasive adenocarcinoma; MIA, minimally invasive adenocarcinoma.
The correlations between the selected multimodal radiomics features are shown in Figure 4, and the correlation coefficients r between the features are all less than 0.8. The OR value of the rad-score was 2.718 (95% CI: 1.807–4.089, P<0.001), and the AUC of the radiomics model was 0.896 (95% CI: 0.830–0.962) and 0.881 (95% CI: 0.777–0.985) in the training and test sets, respectively (Figure 5). Then, the two variables of age and gender with significant differences between groups and rad-score were incorporated into the logistic regression to construct a model (joint model), and the OR values of age, gender, and rad-score were 7.922 (95% CI: 1.671–37.588, P=0.009), 10.181 (95% CI: 1.955–53.008, P=0.006), and 534.105 (95% CI: 34.598–7,794.555, P<0.001). The AUC of the joint model was 0.932 (95% CI: 0.882–0.982) and 0.887 (95% CI: 0.786, 0.988) in the training and test sets, respectively. Comparing the AUCs of the radiomics model and the joint model in the training and test sets, there was no significant difference (0.896 vs. 0.932, P=0.088; 0.881 vs. 0.887, P=0.480).

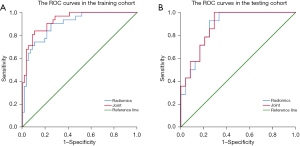
The calibration curves of training and test sets showed that both the radiomics model and joint model had good additive trends; the radiomics model showed a better linear trend in the test set (Figure 6). The area under the PR curves of the training and test sets of the radiomics model was 0.896 and 0.881, and the area under the PR curve of the joint model was 0.932 and 0.887 (Figure 7). The area under the PR curve for the joint model training set and test set was 0.932 and 0.887 (Figure 7). The analysis of the decision curves shows that when the threshold probability was between 0 and 0.70, the net gain of the model in both the training and test sets was greater than that of the “all” and “none” scenarios (Figure 8).

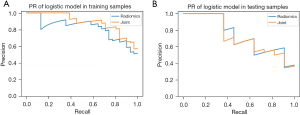
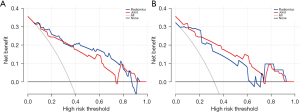
Discussion
Radiomics can extract high-throughput features and detect tumor heterogeneity, which can compensate for the drawbacks of conventional imaging diagnosis (35,36). The first-order features selected in this study are calculated from the pixel grayscale distribution of the original image. Compared with other radiomics features, the first-order features are more interpretable and can directly reflect the information of the image itself. At the same time, the application value of combined spectral CT multimodal imaging and multimodal radiomics was also taken into consideration in determining the invasiveness of GGNs. This study found that the constructed radiomics model and joint model showed ideal performance, and the AUCs in the training set were 0.896 and 0.932, respectively. The calibration curve showed that the probability predicted by the rad-score of the training set and the test set were more consistent with the actual outcome, and the area under the PR curve also showed that the model had a good discriminating ability and diagnostic effect. In a previous study, Fan et al. (37) found that radiomics features were better independent predictors of invasive GGNs than focal CT values and radiological signatures. Zhu et al. (38) selected 16 radiomic features, and the AUC of the radiomics model were 0.828. However, the predictive accuracy of their diagnostic models was lower than the results of this study, and all the above studies used conventional CT to conduct the research.
This study included 63 spectral CT multimodal radiomics features, and finally selected the 5 multimodal features described in the results section. No significant correlation was found between these features, and the r values were all below 0.8. Among these features, p_original_shape_Maximum2DDiameterSlice represents the maximum diameter of the nodule, which has a significant positive correlation with the degree of tumor malignancy and has been reported in previous studies (39,40). a_ED_original_firstorder_90Percentile (arterial phase electron cloud density map) and v_ED_original_firstorder_90Percentile (venous phase electron density map) were associated with the enhancement density of the lesion. Entropy is a measure of texture irregularity, while Uniformity reflects the distance of an image from a uniform distribution of gray levels, and they can reflect local tumoral heterogeneity in electron cloud density and effective atomic number maps. In a previous study, Qiu et al. (26) found that the AUC of the ED value for identifying benign and malignant pulmonary GGNs was 0.722, because the ED value may reflect the microstructure of the lesion. In another study, a combined model of lesion inhomogeneity on the enhanced iodine map, Zeff in the plain scan, the ED and ED-Zeff ratio in plain and enhanced phase was used to differentiate IA from MIA (41). This study is similar to the above-mentioned research, but the above-mentioned research focused on single-modality image data of spectral CT. In this study, the radiomics features of different modalities are connected in series for comprehensive analysis, which can not only compensate for the shortcomings of single-modal imaging technology but also can provide various information of lesions, then finally realize information complementation, and ensure that the prediction performance of the final model is more stable and reliable. On the other hand, although contrast-enhanced CT is not necessary, this study, as a valuable study, performed enhanced CT examination on patients with pulmonary GGN in accordance with the Chinese guidelines (42), and obtained enhanced CT data of the lungs that are difficult to obtain in other countries except China. It may be helpful for scholars who carry out similar research in the future.
In addition, Table 1 shows the demographic and clinical characteristics of all patients, and only age and gender were statistically different between groups. Therefore, this study also combined age and gender with the rad-score to construct a joint model. The joint model is better than the radiomics model in diagnostic performance, although there is no significant difference between the two models, suggesting that the rad-score plays a more important role in diagnosis, which is also evident in the OR value of the three variables. In the joint model, the OR value of the rad-score (OR =534.105) was higher than that of age (OR =7.922) and gender (OR =10.181). Nonetheless, age and gender also showed significant correlations with the degree of malignancy in the joint model. GGNs in older men are more likely to be IA, which is consistent with reports in the literature (43). In summary, the joint model achieved an AUC of 0.887 on the test set. DCA also showed that the joint model has good clinical value and can be used as an optimal diagnostic prediction model.
However, this study has several limitations. First, this is a retrospective study with a small sample, and selection bias is inevitable. Therefore, the generalizability and reliability of the results may be limited. In the future, prospective studies with large samples are needed to confirm these findings. Second, the included clinical features are few, and the joint exploration of genes and metabolomics has not been carried out. More clinical features, such as serological examinations, genetic information, etc., should be included in the follow-up research to build a more complete predictive model of clinical features. Third, the number of smokers in the cases included in this study is small, more comprehensive cohort will be constructed to develop a more clinically valuable model in the future. Fourth, in this study, we have not yet evaluated the body mass index of the subjects. In future research work, we will include body mass index and other indicators to study the impact of overweight and obese patients on the diagnostic performance of images.
Conclusions
Spectral CT multimodal imaging combined with multimodal radiomics can be a non-invasive, robust, and reproducible method for the preoperative identification of GGNs invasiveness, which can provide clinicians with more detailed information about disease risk assessment, intervention, and management.
Acknowledgments
Funding: This research was supported by the National Natural Science Foundation of China (Grant Nos. 82172034 and 82272083).
Footnote
Reporting Checklist: The authors have completed the TRIPOD reporting checklist. Available at https://jtd.amegroups.com/article/view/10.21037/jtd-22-1605/rc
Data Sharing Statement: Available at https://jtd.amegroups.com/article/view/10.21037/jtd-22-1605/dss
Conflicts of Interest: All authors have completed the ICMJE uniform disclosure form (available at https://jtd.amegroups.com/article/view/10.21037/jtd-22-1605/coif). PS, XHZ and HYH are employees of Philips Healthcare. YLW is an employee of Neusoft Medical Systems Co., Ltd. LY and YYC have a mother-son relationship. The other authors have no conflicts of interest to declare.
Ethical Statement: The authors are accountable for all aspects of the work in ensuring that questions related to the accuracy or integrity of any part of the work are appropriately investigated and resolved. This study was conducted in accordance with the Declaration of Helsinki (as revised in 2013). The study was approved by the Ethics Committee of Union Hospital, Tongji Medical College, Huazhong University of Science and Technology (No. 2020S363) and individual consent for this retrospective analysis was waived.
Open Access Statement: This is an Open Access article distributed in accordance with the Creative Commons Attribution-NonCommercial-NoDerivs 4.0 International License (CC BY-NC-ND 4.0), which permits the non-commercial replication and distribution of the article with the strict proviso that no changes or edits are made and the original work is properly cited (including links to both the formal publication through the relevant DOI and the license). See: https://creativecommons.org/licenses/by-nc-nd/4.0/.
References
- Kim YT. Management of Ground-Glass Nodules: When and How to Operate? Cancers (Basel) 2022;14:715. [Crossref] [PubMed]
- Henschke CI, Yankelevitz DF, Mirtcheva R, et al. CT screening for lung cancer: frequency and significance of part-solid and nonsolid nodules. AJR Am J Roentgenol 2002;178:1053-7. [Crossref] [PubMed]
- Hansell DM, Bankier AA, MacMahon H, et al. Fleischner Society: glossary of terms for thoracic imaging. Radiology 2008;246:697-722. [Crossref] [PubMed]
- Travis WD, Brambilla E, Noguchi M, et al. International association for the study of lung cancer/american thoracic society/european respiratory society international multidisciplinary classification of lung adenocarcinoma. J Thorac Oncol 2011;6:244-85. [Crossref] [PubMed]
- Ito M, Miyata Y, Kushitani K, et al. Prediction for prognosis of resected pT1a-1bN0M0 adenocarcinoma based on tumor size and histological status: relationship of TNM and IASLC/ATS/ERS classifications. Lung Cancer 2014;85:270-5. [Crossref] [PubMed]
- Boland JM, Froemming AT, Wampfler JA, et al. Adenocarcinoma in situ, minimally invasive adenocarcinoma, and invasive pulmonary adenocarcinoma--analysis of interobserver agreement, survival, radiographic characteristics, and gross pathology in 296 nodules. Hum Pathol 2016;51:41-50. [Crossref] [PubMed]
- Van Schil PE, Asamura H, Rusch VW, et al. Surgical implications of the new IASLC/ATS/ERS adenocarcinoma classification. Eur Respir J 2012;39:478-86. [Crossref] [PubMed]
- Shah R, Sabanathan S, Richardson J, et al. Results of surgical treatment of stage I and II lung cancer. J Cardiovasc Surg (Torino) 1996;37:169-72. [PubMed]
- Nicholson AG, Chansky K, Crowley J, et al. The International Association for the Study of Lung Cancer Lung Cancer Staging Project: Proposals for the Revision of the Clinical and Pathologic Staging of Small Cell Lung Cancer in the Forthcoming Eighth Edition of the TNM Classification for Lung Cancer. J Thorac Oncol 2016;11:300-11.
- Raz DJ, Zell JA, Ou SH, et al. Natural history of stage I non-small cell lung cancer: implications for early detection. Chest 2007;132:193-9. [Crossref] [PubMed]
- Kobayashi N, Kobayashi K, Kikuchi S, et al. Long-term pulmonary function after surgery for lung cancer. Interact Cardiovasc Thorac Surg 2017;24:727-32. [Crossref] [PubMed]
- Kim SJ, Lee YJ, Park JS, et al. Changes in pulmonary function in lung cancer patients after video-assisted thoracic surgery. Ann Thorac Surg 2015;99:210-7. [Crossref] [PubMed]
- Moon Y, Lee KY, Park JK. The prognosis of invasive adenocarcinoma presenting as ground-glass opacity on chest computed tomography after sublobar resection. J Thorac Dis 2017;9:3782-92. [Crossref] [PubMed]
- Wu H, Liu C, Xu M, et al. A Retrospective Study of Mean Computed Tomography Value to Predict the Tumor Invasiveness in AAH and Clinical Stage Ia Lung Cancer. Zhongguo Fei Ai Za Zhi 2018;21:190-6. [PubMed]
- Ye T, Deng L, Wang S, et al. Lung Adenocarcinomas Manifesting as Radiological Part-Solid Nodules Define a Special Clinical Subtype. J Thorac Oncol 2019;14:617-27. [Crossref] [PubMed]
- Kim TJ, Lee JH, Lee CT, et al. Diagnostic accuracy of CT-guided core biopsy of ground-glass opacity pulmonary lesions. AJR Am J Roentgenol 2008;190:234-9. [Crossref] [PubMed]
- Lu CH, Hsiao CH, Chang YC, et al. Percutaneous computed tomography-guided coaxial core biopsy for small pulmonary lesions with ground-glass attenuation. J Thorac Oncol 2012;7:143-50. [Crossref] [PubMed]
- Fu F, Zhang Y, Wang S, et al. Computed tomography density is not associated with pathological tumor invasion for pure ground-glass nodules. J Thorac Cardiovasc Surg 2021;162:451-459.e3. [Crossref] [PubMed]
- Naidich DP, Bankier AA, MacMahon H, et al. Recommendations for the management of subsolid pulmonary nodules detected at CT: a statement from the Fleischner Society. Radiology 2013;266:304-17. [Crossref] [PubMed]
- Yi CA, Lee KS, Kim EA, et al. Solitary pulmonary nodules: dynamic enhanced multi-detector row CT study and comparison with vascular endothelial growth factor and microvessel density. Radiology 2004;233:191-9. [Crossref] [PubMed]
- Son JY, Lee HY, Kim JH, et al. Quantitative CT analysis of pulmonary ground-glass opacity nodules for distinguishing invasive adenocarcinoma from non-invasive or minimally invasive adenocarcinoma: the added value of using iodine mapping. Eur Radiol 2016;26:43-54. [Crossref] [PubMed]
- Wang X, Liu D, Jiang S, et al. Subjective and Objective Assessment of Monoenergetic and Polyenergetic Images Acquired by Dual-Energy CT in Breast Cancer. Korean J Radiol 2021;22:502-12. [Crossref] [PubMed]
- Ebrahimian S, Singh R, Netaji A, et al. Characterization of Benign and Malignant Pancreatic Lesions with DECT Quantitative Metrics and Radiomics. Acad Radiol 2022;29:705-13. [Crossref] [PubMed]
- Li J, Dong D, Fang M, et al. Dual-energy CT-based deep learning radiomics can improve lymph node metastasis risk prediction for gastric cancer. Eur Radiol 2020;30:2324-33. [Crossref] [PubMed]
- Yu Y, Cheng JJ, Li JY, et al. Determining the invasiveness of pure ground-glass nodules using dual-energy spectral computed tomography. Transl Lung Cancer Res 2020;9:484-95. [Crossref] [PubMed]
- Qiu J, Xin X, Yang W, et al. The value of virtual monoenergetic images and electron density map derived from dual-layer spectral detector CT in differentiating benign from malignant pulmonary ground glass nodules. Chinese Journal of Radiology 2022;175-81.
- Travis WD, Brambilla E, Nicholson AG, et al. The 2015 World Health Organization Classification of Lung Tumors: Impact of Genetic, Clinical and Radiologic Advances Since the 2004 Classification. J Thorac Oncol 2015;10:1243-60. [Crossref] [PubMed]
- Henschke CI, Yankelevitz DF, Smith JP, et al. Screening for lung cancer: the early lung cancer action approach. Lung Cancer 2002;35:143-8. [Crossref] [PubMed]
- Cohen JG, Goo JM, Yoo RE, et al. Software performance in segmenting ground-glass and solid components of subsolid nodules in pulmonary adenocarcinomas. Eur Radiol 2016;26:4465-74. [Crossref] [PubMed]
- Shur JD, Doran SJ, Kumar S, et al. Radiomics in Oncology: A Practical Guide. Radiographics 2021;41:1717-32. [Crossref] [PubMed]
- Pal KK, Sudeep K. editors. Preprocessing for image classification by convolutional neural networks. 2016 IEEE International Conference on Recent Trends in Electronics, Information & Communication Technology (RTEICT); 2016: IEEE.
- Luo X, Song T, Wang G, et al. SCPM-Net: An anchor-free 3D lung nodule detection network using sphere representation and center points matching. Med Image Anal 2022;75:102287. [Crossref] [PubMed]
- Kopelowitz E, Engelhard G. Lung nodules detection and segmentation using 3D mask-RCNN. arXiv 2019. doi:
10.48550 /arXiv.1907.07676. - van Griethuysen JJM, Fedorov A, Parmar C, et al. Computational Radiomics System to Decode the Radiographic Phenotype. Cancer Res 2017;77:e104-7. [Crossref] [PubMed]
- Lambin P, Rios-Velazquez E, Leijenaar R, et al. Radiomics: extracting more information from medical images using advanced feature analysis. Eur J Cancer 2012;48:441-6. [Crossref] [PubMed]
- Gillies RJ, Kinahan PE, Hricak H. Radiomics: Images Are More than Pictures, They Are Data. Radiology 2016;278:563-77. [Crossref] [PubMed]
- Fan L, Fang M, Li Z, et al. Radiomics signature: a biomarker for the preoperative discrimination of lung invasive adenocarcinoma manifesting as a ground-glass nodule. Eur Radiol 2019;29:889-97. [Crossref] [PubMed]
- Zhu M, Yang Z, Wang M, et al. A computerized tomography-based radiomic model for assessing the invasiveness of lung adenocarcinoma manifesting as ground-glass opacity nodules. Respir Res 2022;23:96. [Crossref] [PubMed]
- Xiang W, Xing Y, Jiang S, et al. Morphological factors differentiating between early lung adenocarcinomas appearing as pure ground-glass nodules measuring ≤10 mm on thin-section computed tomography. Cancer Imaging 2014;14:33. [Crossref] [PubMed]
- Ding H, Shi J, Zhou X, et al. Value of CT Characteristics in Predicting Invasiveness of Adenocarcinoma Presented as Pulmonary Ground-Glass Nodules. Thorac Cardiovasc Surg 2017;65:136-41. [PubMed]
- Yu Y, Fu Y, Chen X, et al. Dual-layer spectral detector CT: predicting the invasiveness of pure ground-glass adenocarcinoma. Clin Radiol 2022;77:e458-65. [Crossref] [PubMed]
- Oncology Society of Chinese Medical Association. Chinese Medical Association guideline for clinical diagnosis and treatment of lung cancer (2022 edition). Zhonghua Zhong Liu Za Zhi 2022;44:457-90. [PubMed]
- Yang HH, Lv YL, Fan XH, et al. Factors distinguishing invasive from pre-invasive adenocarcinoma presenting as pure ground glass pulmonary nodules. Radiat Oncol 2020;15:186. [Crossref] [PubMed]