A new prognostic model for RHOV, ABCC2, and CYP4B1 to predict the prognosis and association with immune infiltration of lung adenocarcinoma
Highlight box
Key findings
• Our new prognostic model based on lymph node metastasis-related genes RHOV, ABCC2, and CYP4B1 might predict the prognosis of LUAD patients and be associated with immune infiltration.
What is known and what is new?
• Lymph node metastasis is related to the poor prognosis of patients with LUAD.
• The molecular mechanisms of lymph node metastasis have not been fully understood in LUAD. Lymph node metastasis-related genes RHOV, ABCC2, and CYP4B1 were found to be associated with the prognosis of LUAD patients.
What is the implication, and what should change now?
• A prognostic model based on RHOV, ABCC2, and CYP4B1 might predict the prognosis of cancer patients, which might become a tool to predict the prognosis of LUAD patients.
Introduction
Lung cancer is one of the most common malignant tumors worldwide, as well as one of the main causes of cancer-related death (1,2). In China, the morbidity and mortality of lung cancer remain high throughout the entire year (3). Lung adenocarcinoma (LUAD) is one of the common subtypes of lung cancer (4). Although there are many effective targeted drugs for treating cancer patients, LUAD remains one of the most common and fatal cancers worldwide.
Early metastasis of LUAD is one of the key factors leading to cancer progression to the middle and advanced stages (5-7). Lymph node metastasis is one of the common modes of LUAD metastasis and is one of the risk factors affecting the long-term survival of patients with LUAD (6,7). Luo et al. reported that the metastasis rates of N1 and N2 stages increased with the tumor diameter (6). Dai et al. reported that 15% of LUAD patients had lymph node metastasis. Compared with node-negative patients, recurrence-free survival (RFS) and overall survival (OS) have been shown to be significantly decreased in patients with metastasis (7). However, the molecules and the signaling mechanisms of lymph node metastasis in LUAD remain unelucidated. The Cancer Genome Atlas (TCGA) project aims to improve the ability to prevent, diagnose, and treat cancer using high-throughput genome analysis technology. Multiple cancer types and data of genes, microRNAs (miRNAs), long noncoding RNAs (lncRNAs), and others are displayed in the TCGA database (8,9). Lymph node metastasis was a risk factor for poor prognosis in LUAD patients. However, the roles of lymph node metastasis-related genes in the progression of LUAD has not been fully revealed. Therefore, important molecular markers in the process of lymph node metastasis were explored based on the data from the TCGA database. The roles of the established lymph node metastasis-related the prognostic model were investigated in LUAD metastasis to improve the treatment value for LUAD patients. The relationship between the prognostic model and the prognosis, as well as immune cell infiltration of LUAD, were explored to understand the key molecules in LUAD metastasis. We present the following article in accordance with the TRIPOD reporting checklist (available at https://jtd.amegroups.com/article/view/10.21037/jtd-23-265/rc).
Methods
TCGA data download and visualization analysis
The gene expression data of 594 cases of LUAD high-throughput sequence-fragments per kilobase million (HTSeq-FPKM) and the clinical data of 522 cancer patients were downloaded from the TCGA database. The gene expression data of 535 LUAD tissues were included, and LUAD gene expression data with the N0-3 stage were extracted in our study. A total of 330 LUAD patients were lymph node-negative, whereas 171 LUAD patients were lymph node-positive. Differentially expressed genes (DEGs) of LUAD metastasis were screened using the limma package of R software (version 4.0.2; https://www.r-project.org/) with the criteria of a false discovery rate (FDR) <0.05 and |logFC| >1, and the visualization results were displayed using a volcano map. The study was conducted in accordance with the Declaration of Helsinki (as revised in 2013).
Biological functions and protein-protein interaction (PPI) network of lymph node metastasis-related genes
Biological processes, cellular components, molecular functions, and signaling mechanisms involved in lymph node metastasis-related genes were investigated using Gene Ontology (GO) and Kyoto Encyclopedia of Genes and Genomes (KEGG) analysis in the Database for Annotation, Visualization, and Integrated Discovery (DAVID) with the criterion of FDR <0.05. A PPI network of lymph node metastasis-related genes was visualized in the Search Tool for the Retrieval of Interacting Genes/Proteins (STRING) database and enriched for analysis using the MCODE method in Cytoscape software (10).
Kaplan-Meier (K-M) survival analysis
Grouped by the median value of DEGs, the relationship between high- and low-expressed DEGs and the OS of LUAD patients were explored via K-M survival analysis and with P<0.05 for filtering and screening. According to the median score, the patients were divided into high-risk and low-risk groups. The prognostic value of LUAD patients in the high-risk and low-risk groups was presented using K-M survival analysis.
Construction of nomogram and prognostic model of lymph node metastasis-related genes
The roles of DEGs in lymph node metastasis were identified in the prognosis of LUAD patients using univariate Cox regression analysis with the criterion of P<0.001. On this basis, multivariate Cox regression analysis and the Akaike information Criterion (AIC) method were performed to screen the factors affecting a poor prognosis of LUAD patients, and a nomogram and a prognostic model were constructed (11).
Construction of prognostic model-related nomogram
The risk score data and the clinicopathological characteristic data of LUAD patients were matched. The relationship between clinicopathological characteristics and the lymph node metastasis prognostic model, and the prognosis of LUAD patients were investigated using univariate and multivariate Cox regression analysis, and a nomogram was constructed based on the multivariate Cox analysis results.
Gene set enrichment analysis (GSEA)
The regulatory mechanisms in which genes might be involved were explored using GSEA (12,13). Grouping of the gene expression data of LUAD was performed according to the median prognostic model score, and the signaling pathways of the lymph node metastasis-related prognostic model were explored. The screening criterion of GSEA: NOM P<0.05.
Immune analysis of prognostic model
Tissue samples from patients with LUAD were scored using Cell-type Identification by Estimating Relative Subsets of RNA Transcripts (CIBERSORT), microenvironment cell populations (MCP)-counter, and estimation methods (14,15). Pearson correlation analysis was used to explore the relationship between the immune score and the levels of tumor purity, immune cells, and immune cell markers, and P<0.05 was considered a significant screening criterion.
Statistical analysis
Perl (https://www.perl.org/) and R were used for data processing and statistical analysis. Cox regression and K-M survival analyses were conducted to filter the risk factors of OS in patients with LUAD, and ROC analysis was carried out to assess the role of a gene-associated nomogram in lymph node metastasis. The relationship between the immune score and the levels of tumor purity, immune cells, and immune cell markers was explored using Pearson correlation analysis. The expression of DEGs in high- and low-risk groups was detected using a t-test, and P<0.05 was considered statistically significant.
Results
Identification of DEGs related to metastasis in LUAD
Comparing the tissues with lymph node-negative LUAD patients, there were 213 DEGs in the tissues of lymph node-positive LUAD patients (Table S1 and Table S2). Among them, 75 DEGs were upregulated (Table S1), and 138 DEGs were downregulated (Table S2). The top 15 DEGs in LUAD tissues were shown by fold changes (Figure 1). In detail, the expression levels of LRRC38, TAC1, CALB1, CGB5, KRT20, TRIM48, TM4SF20, NNAT, GCG, PI3, CYP2B6, SPAG11B, GUCA2B, RHCG, and MUC2 were increased in the tissues of lymph node-positive LUAD patients (Figure 1A), whereas the expression of ALB, NPY, AFP, SPINK4, WFDC12, DKK4, WFDC5, DLK1, MSTN, FABP7, HIST1H4C, HIST1H1B, GC, VTN, and FTHL17 was decreased in the tissues of lymph node-positive LUAD patients (Figure 1B).
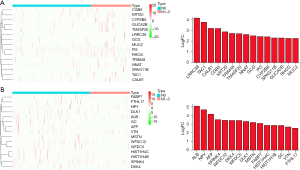
Biological functions and PPI network of lymph node metastasis-related genes
The DEGs of lymph node metastasis were found to be involved in the DNA replication-dependent nucleosome assembly, negative regulation of gene expression, cellular protein metabolic process, extracellular exosome, DNA-templated transcription and initiation, positive regulation of cytokine secretion, drug metabolic process, WNT signaling pathway, chemokine production, receptor binding, toll-like receptor 4 binding, and other functions (Figure 2A-2C and Table S3). The signaling pathways in which the DEGs of lymph node metastasis were involved were viral carcinogenesis, steroid hormone biosynthesis, drug metabolism-cytochrome P450, chemical carcinogenesis, the PPAR signaling pathway, and others (Figure 2D and Table 1). The PPI network was presented and was enriched for analysis using the MCODE method (Figure 3 and Figure S1).
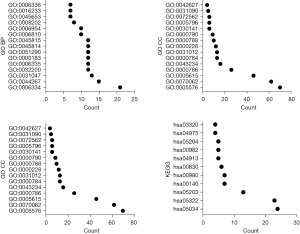
Table 1
Term | Content | Count | P value |
---|---|---|---|
Hsa05322 | Systemic lupus erythematosus | 23 | 9.88E-20 |
Hsa05034 | Alcoholism | 24 | 2.99E-18 |
Hsa05203 | Viral carcinogenesis | 13 | 5.36E-06 |
Hsa00140 | Steroid hormone biosynthesis | 7 | 6.31E-05 |
Hsa00980 | Metabolism of xenobiotics by cytochrome P450 | 7 | 2.48E-04 |
Hsa00830 | Retinol metabolism | 6 | 9.89E-04 |
Hsa04913 | Ovarian steroidogenesis | 5 | 0.00276348 |
Hsa00982 | Drug metabolism-cytochrome P450 | 5 | 0.008937351 |
Hsa04975 | Fat digestion and absorption | 4 | 0.011324964 |
Hsa05204 | Chemical carcinogenesis | 5 | 0.015572222 |
Hsa03320 | PPAR signaling pathway | 4 | 0.046733944 |
Hsa05202 | Transcriptional misregulation in cancer | 6 | 0.050691025 |
DEGs, differentially expressed genes; PPAR, peroxisome proliferator activated receptor.
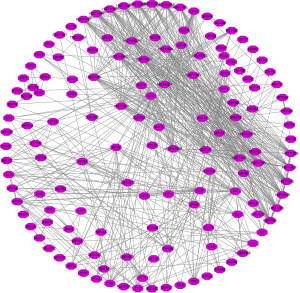
Metastasis genes related to prognosis of LUAD
The results of K-M survival analysis revealed that the expression levels of PI3, CALB1, STC1, STAR, HIST1H4B, CYP17A1, HIST2H2AB, RHOV, GUCA2B, TM4SF20, KRT20, HIST1H4A, PI15, GLP2R, KRT78, DEFB1, CRHR2, ABCC2, HIST1H2BO, CYP4B1, LIPF, S100G, CPB1, OTX2, KRT16, CYP2A6, and NTS were related to the OS of LUAD patients (Table 2).
Table 2
Gene | P value |
---|---|
ABCC2 | 2.504e-02 |
CALB1 | 4.097e-02 |
CPB1 | 1.504e-02 |
CRHR2 | 2.322e-02 |
CYP2A6 | 7.923e-03 |
CYP4B1 | 2.655e-03 |
CYP17A1 | 9.490e-05 |
DEFB1 | 1.287e-02 |
GLP2R | 2.748e-02 |
GUCA2B | 2.236e-03 |
HIST1H2BO | 2.663e-04 |
HIST1H4A | 3.322e-02 |
HIST1H4B | 4.416e-02 |
HIST2H2AB | 1.243e-02 |
KRT16 | 6.119e-04 |
KRT20 | 4.358e-02 |
KRT78 | 1.235e-02 |
LIPF | 3.390e-02 |
NTS | 4.799e-02 |
OTX2 | 1.315e-02 |
PI15 | 2.613e-02 |
PI3 | 3.250e-02 |
RHOV | 7.444e-03 |
STAR | 4.710e-03 |
STC1 | 3.702e-03 |
TM4SF20 | 4.258e-02 |
S100G | 1.731e-03 |
LUAD, lung adenocarcinoma; K-M, Kaplan-Meier.
Construction of the prognostic model
Univariate Cox regression analysis revealed that the expression levels of STC1, RHOV, GUCA2B, ABCC2, CYP4B1, KRT16, and NTS might be risk factors for the OS of LUAD patients (Figure S2). Multivariate Cox regression analysis and the AIC method revealed that RHOV, ABCC2, and CYP4B1 were independent risk factors for a poor prognosis in patients with LUAD, and a prognostic model based on the expression levels of RHOV, ABCC2, and CYP4B1 was constructed. In addition, a nomogram based on the expression levels of RHOV, ABCC2, and CYP4B1 was constructed (Figure 4).
Risk score of lymph node metastasis-related genes was associated with poor prognosis in LUAD patients
Figure 5A-5C depict the relationship between the risk score and the prognosis of cancer patients. K-M survival analysis illustrated that OS in the low-risk group was significantly higher than in the high-risk group in LUAD patients (Figure 5D). Univariate Cox regression analysis demonstrated that the clinical stage, T stage, lymph node metastasis, and risk score were the influencing factors of a poor prognosis in LUAD patients (Figure S3A). Multivariate Cox regression analysis depicted that the clinical stage and prognostic model score were independent risk factors for a poor prognosis in LUAD patients (Figure S3B). A nomogram based on the results of the Cox regression analysis was constructed to assess the prognosis of cancer patients (Figure 6).
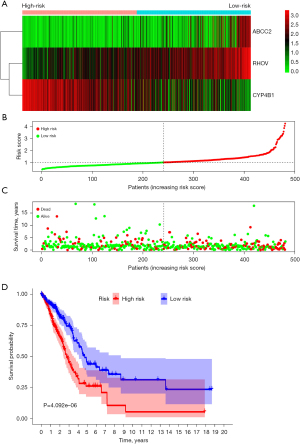
Prognostic model participation in the signaling mechanisms of LUAD
In the signaling mechanism module, DNA replication, cell cycle, homologous recombination, mismatch repair, proteasome, pyrimidine metabolism, base excision repair, pentose phosphate pathway, spliceosome, P53 signaling pathway, oocyte meiosis, ubiquitin-mediated proteolysis, basal transcription factors, and other pathways were significantly enriched in the high-risk group (Table 3).
Table 3
Name | Size | NES | NOM P value |
---|---|---|---|
DNA replication | 36 | 2.188415 | 0 |
Cell cycle | 124 | 2.101134 | 0 |
Homologous recombination | 28 | 2.0641458 | 0 |
Mismatch repair | 23 | 2.0362506 | 0 |
Proteasome | 44 | 2.0210252 | 0 |
Pyrimidine metabolism | 97 | 2.0000293 | 0 |
Base excision repair | 33 | 1.98535 | 0.002083333 |
Pentose phosphate pathway | 27 | 1.9286441 | 0.004024145 |
Nucleotide excision repair | 44 | 1.8649865 | 0.005725191 |
P53 signaling pathway | 68 | 1.7573924 | 0.007905139 |
Riboflavin metabolism | 15 | 1.6678965 | 0.011494253 |
Spliceosome | 126 | 1.8515968 | 0.014084507 |
Pathogenic escherichia coli infection | 55 | 1.7213273 | 0.015717093 |
Fructose and mannose metabolism | 32 | 1.6948974 | 0.015904572 |
N glycan biosynthesis | 46 | 1.7191821 | 0.018181818 |
Protein export | 23 | 1.7690526 | 0.019646365 |
Oocyte meiosis | 112 | 1.5964646 | 0.023715414 |
RNA degradation | 57 | 1.628982 | 0.028957529 |
Glycolysis gluconeogenesis | 61 | 1.6014928 | 0.029166667 |
Ubiquitin mediated proteolysis | 133 | 1.5566719 | 0.037328094 |
Purine metabolism | 156 | 1.4549121 | 0.042769857 |
Glyoxylate and dicarboxylate metabolism | 16 | 1.6099515 | 0.042857144 |
Basal transcription factors | 35 | 1.4993141 | 0.04828974 |
Galactose metabolism | 25 | 1.5636381 | 0.05 |
NES, normalized enrichment score; NOM, nominal.
Prognostic model in LUAD immune microenvironment
Based on the results of estimation methods concerning LUAD tissues, the risk score was significantly correlated with tumor purity, stromal score, immune score, and estimated score expression levels using correlation analysis (Figure 7A-7D). In the high-risk group, the expression of tumor purity was increased, and the expression levels of the stromal score, immune score, and estimate score were decreased (Figure 7E-7H). Based on the results of MCP-counter methods concerning LUAD tissues, the risk score was significantly associated with T cells, endothelial cells, neutrophils, and other immune cells (Table 4). Based on the results of CIBERSORT methods concerning LUAD tissues, the risk score was significantly associated with the levels of B cell memory, T cell CD8, T cell follicular helper, and other immune cells (Figure 8 and Table 5). In the LUAD tissues, the risk score was significantly associated with the levels of immune cell markers (Table 6). More specifically, the risk score was significantly correlated with the expression levels of BCL6, CCR7, CCR8, and other markers of immune cells.
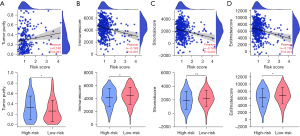
Table 4
Immune cells | Correlation coefficient | P value |
---|---|---|
T cells | −0.149 | 0.001 |
CD8 T cells | 0.024 | 0.597 |
Cytotoxic lymphocytes | 0.03 | 0.505 |
B lineage | −0.126 | 0.006 |
NK cells | 0.007 | 0.886 |
Monocytic lineage | −0.068 | 0.137 |
Myeloid dendritic cells | −0.290 | <0.001 |
Neutrophils | −0.109 | 0.017 |
Endothelial cells | −0.321 | <0.001 |
Fibroblasts | 0.057 | 0.214 |
MCP, microenvironment cell populations; NK, natural killer.
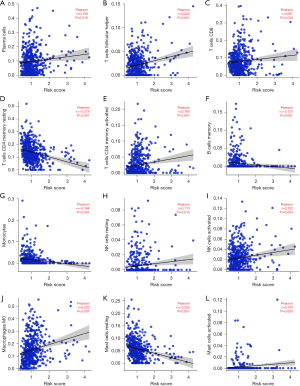
Table 5
Immune cells | Correlation coefficient | P value |
---|---|---|
B cells memory | −0.123 | 0.007 |
B cells naive | 0.055 | 0.227 |
Plasma cells | 0.108 | 0.018 |
T cells CD8 | 0.097 | 0.034 |
T cells CD4 memory resting | −0.278 | <0.001 |
T cells CD4 memory activated | 0.160 | <0.001 |
T cells follicular helper | 0.220 | <0.001 |
T cells regulatory | −0.021 | 0.652 |
T cells gamma delta | 0.012 | 0.786 |
NK cells resting | 0.113 | 0.013 |
NK cells activated | 0.132 | 0.004 |
Monocytes | −0.184 | <0.001 |
Macrophages M0 | 0.220 | <0.001 |
Macrophages M1 | 0.077 | 0.091 |
Macrophages M2 | −0.164 | <0.001 |
Dendritic cells resting | −0.220 | <0.001 |
Dendritic cells activated | 0.093 | 0.043 |
Mast cells resting | −0.252 | <0.001 |
Mast cells activated | 0.134 | 0.003 |
Eosinophils | 0.019 | 0.672 |
Neutrophils | 0.095 | 0.037 |
NK, natural killer.
Table 6
Cell markers | Correlation coefficient | P value |
---|---|---|
BCL6 | −0.102 | 0.025 |
CCR8 | −0.106 | 0.020 |
CD2 | −0.100 | 0.028 |
CD3E | −0.101 | 0.027 |
CD8B | 0.024 | 0.597 |
CD79A | −0.081 | 0.076 |
CEACAM8 | −0.156 | <0.001 |
FOXP3 | −0.091 | 0.046 |
GZMB | 0.170 | <0.001 |
HLA-DPA1 | −0.307 | <0.001 |
HLA-DQB1 | −0.256 | <0.001 |
IFNG | 0.094 | 0.040 |
IL17A | −0.007 | 0.877 |
IRF5 | −0.046 | 0.311 |
ITGAX | −0.154 | <0.001 |
MS4A4A | −0.165 | <0.001 |
NRP1 | −0.167 | <0.001 |
PTGS2 | 0.039 | 0.392 |
STAT3 | −0.072 | 0.114 |
STAT5B | −0.239 | <0.001 |
TBX21 | 0.014 | 0.755 |
TNF | −0.002 | 0.971 |
CCR7 | −0.180 | <0.001 |
CD1C | −0.268 | <0.001 |
CD3D | −0.058 | 0.208 |
CD8A | 0.022 | 0.626 |
CD19 | −0.113 | 0.013 |
CD163 | −0.092 | 0.044 |
CTLA4 | −0.052 | 0.251 |
GATA3 | 0.185 | <0.001 |
HAVCR2 | −0.114 | 0.012 |
HLA-DPB1 | −0.326 | <0.001 |
HLA-DRA | −0.273 | <0.001 |
IL13 | −0.100 | 0.029 |
IL21 | −0.012 | 0.785 |
ITGAM | −0.201 | <0.001 |
LAG3 | 0.023 | 0.613 |
NOS2 | −0.009 | 0.845 |
PDCD1 | 0.018 | 0.701 |
STAT1 | 0.185 | <0.001 |
STAT5A | −0.182 | <0.001 |
STAT6 | −0.180 | <0.001 |
TGFB1 | −0.112 | 0.014 |
VSIG4 | −0.142 | 0.002 |
Discussion
Lung cancer is the most common malignant tumor worldwide and has the highest mortality rate. LUAD accounts for about 40% of lung cancer (1,3,4). Currently, the annual mortality rate of LUAD patients remains high. In the past few decades, the application and mining of big data have constituted one of the important means by which to diagnose, treat, and evaluate the prognosis of patients with cancer. The TCGA database contains high-quality tumor genome data and clinical information of patients. In our study, we found that PI3, CALB1, STC1, STAR, HIST1H4B, CYP17A1, HIST2H2AB, RHOV, GUCA2B, TM4SF20, KRT20, HIST1H4A, PI15, GLP2R, KRT78, DEFB1, CRHR2, ABCC2, HIST1H2BO, CYP4B1, LIPF, S100G, CPB1, OTX2, KRT16, CYP2A6, and NTS were unusually expressed, and related to the OS of LUAD using the TCGA database. RHOV, ABCC2, and CYP4B1 were independent risk factors for a poor prognosis in LUAD patients and were correlated with the prognostic model. Currently, RHOV, ABCC2, and CYP4B1 have important biological roles in cancer (16-21). For example, Shepelev et al. found that the expression of RHOV was increased in lung cancer tissues and cells, and the increased expression of RHOV was associated with a poor prognosis of patients (16). Chen et al. reported that ABCC2 was overexpressed in various human cancers (18). The expression of ABCC2 was upregulated in cisplatin-resistant A549 cells (A549/DDP). Interfering with the expression of ABCC2 could reverse the resistance of A549/DDP cells to cisplatin in vitro, promote G1 phase arrest, and activate the expression of PARP and caspase-3 proteins. The knockout of ABCC2 expression in vivo could enhance the cytotoxicity of cisplatin to subcutaneous transplanted tumors (18). The expression of CYP4B1 in LUAD decreased, which was related to the history of drug treatment, radiotherapy, and the survival status of cancer patients (21). In addition, we established prognostic model for RHOV, ABCC2, and CYP4B1 and demonstrated that RHOV and ABCC2 were overexpressed and CYP4B1 was underexpressed in the high-risk group, and the OS of LUAD patients in the low-risk group was significantly higher than that in the high-risk group. Univariate Cox regression analysis showed that clinical stage, T stage, lymph node metastasis, and risk score were influencing factors of a poor prognosis in patients with LUAD. Multivariate Cox regression analysis demonstrated that clinical stage and prognostic model score were independent risk factors for a poor prognosis in LUAD patients. This demonstrated that RHOV, ABCC2, and CYP4B1 played an important role in lung cancer and indicated that the prognostic model and the nomogram based on the genes RHOV, ABCC2, and CYP4B1 of LUAD metastasis have important predictive value.
The process of lung cancer metastasis involves a variety of biological processes and changes of molecular markers (22-27). The expression of long-chain noncoding RNA NSCLCAT1 was upregulated in the NSCLC tissues. NSCLCAT1 could increase the viability, migration, and invasion of NSCLC cells and reduce apoptosis by inhibiting the expression of CDH1 and mediating the hippo signaling pathway (22). In NSCLC cells, the inhibition of PLK1 expression could change the expression of genes related to DNA damage, replication, and repair (23). Rig-G was frequently downregulated in lung cancer tissues and cell lines and associated with a poor prognosis in lung cancer patients. The overexpression of Rig-G has been shown to result in a significant reduction in cell growth and migration inhibition in A549 and NCI-H1944 cells, along with a reduced epithelial-to-mesenchymal transition. Rig-G acted as a tumor suppressor through the p53 signaling mechanism (27). The metastasis genes and the prognostic model had important biological value in the cell cycle, DNA replication, p53 signaling pathway, and other mechanisms using GO, KEGG, and GSEA, whereby proving that our prognostic model had good predictive value in the progression of LUAD.
Recently, immunotherapy has become a dominant therapeutic theme. Immunotherapy could improve long-term survival and the chances of surgery in patients with LUAD (28,29). For example, the CDK5 inhibitor resulted in decreased PD-L1 protein expression in human lung adenocarcinoma (LLC) cells. PD-L1 protein degradation was mediated using the E3 ligase TRIM21 ubiquitination-proteasome pathway (29). In vitro, the deletion of CDK5 in the LLC of mice has not been shown to affect cell proliferation. However, the attenuation of CDK5 or binding to anti-PD-L1 was shown to greatly inhibit tumor growth in vivo mouse model of LLC implantation. CDK5 disruption caused higher levels of CD3, CD4, and CD8 T cells in the spleen and decreased PD-1 expression in CD4 and CD8 T cells, which provided a potential therapeutic target for LUAD combination immunotherapy (29). In our research, the prognostic model score based on the RHOV, ABCC2, and CYP4B1 was significantly correlated with tumor purity, stromal score, immune score, and estimate score expression levels. The risk score was significantly associated with T cells, endothelial cells, neutrophils, CD8, T cell follicular helper cells, and other immune cells. In LUAD tissues, the risk score was significantly associated with the levels of immune cell markers. In addition, study has confirmed that RHOV is related to the regulation of immune cells (30). Specifically, RHOV expression increased during the differentiation of macrophages into osteoclasts, while a large number of macrophages showed apoptosis. When osteoprotegerin (OPG) inhibits the differentiation of macrophages into osteoclasts, and then OPG can inhibit apoptosis, which is related to the down-regulation of RHOV expression level (30). However, the relationship between ABCC2, and CYP4B1 and immune cell regulation has not been reported in the literature, which will be our research direction in the future.
The molecular mechanisms of lymph node metastasis have not been fully understood in LUAD. Lymph node metastasis-related genes RHOV, ABCC2, and CYP4B1 were found to be associated with the prognosis of LUAD patients. In addition, this study used big data samples to provide new candidate biomarkers related to LUAD metastasis for the prognosis of LUAD patients, which has the advantage of high reliability. However, our study also had some limitations. First, the expression levels of RHOV, ABCC2, and CYP4B1 in clinical LUAD samples need to be verified. Moreover, the expression levels of RHOV, ABCC2, and CYP4B1 and the values of their constructed prognostic model in the prognosis of LUAD patients need to be explored. The roles and the underlying signaling mechanisms of our constructed prognostic model were explored in LUAD using basic research in the future.
Conclusions
There are many molecular DEGs in the process of LUAD metastasis. RHOV, ABCC2, and CYP4B1 are influencing factors of a poor prognosis, and clinical stage and risk score are independent risk factors for a poor prognosis in patients with LUAD. The prognostic model might be involved in the progression of LUAD through the cell cycle, DNA replication, p53 signaling pathway, and others. A prognostic model based on RHOV, ABCC2, and CYP4B1 might predict the prognosis of LUAD patients and be associated with immune infiltration.
Acknowledgments
Thanks to the TCGA database for providing the data used for analysis.
Funding: None.
Footnote
Reporting Checklist: The authors have completed the TRIPOD reporting checklist. Available at https://jtd.amegroups.com/article/view/10.21037/jtd-23-265/rc
Conflicts of Interest: All authors have completed the ICMJE uniform disclosure form (available at https://jtd.amegroups.com/article/view/10.21037/jtd-23-265/coif). The authors have no conflicts of interest to declare.
Ethical Statement: The authors are accountable for all aspects of the work in ensuring that questions related to the accuracy or integrity of any part of the work are appropriately investigated and resolved. The study was conducted in accordance with the Declaration of Helsinki (as revised in 2013).
Open Access Statement: This is an Open Access article distributed in accordance with the Creative Commons Attribution-NonCommercial-NoDerivs 4.0 International License (CC BY-NC-ND 4.0), which permits the non-commercial replication and distribution of the article with the strict proviso that no changes or edits are made and the original work is properly cited (including links to both the formal publication through the relevant DOI and the license). See: https://creativecommons.org/licenses/by-nc-nd/4.0/.
References
- Chen PW, Huang SK, Chou WC, et al. Severinia buxifolia-isolated acridones inhibit lung cancer invasion and decrease HIFα protein synthesis involving 5'UTR-mediated translation inhibition. Phytomedicine 2023;109:154570. [Crossref] [PubMed]
- Ding DX, Li Q, Shi K, et al. LncRNA NEAT1-miR-101-3p/miR-335-5p/miR-374a-3p/miR-628-5p-TRIM6 axis identified as the prognostic biomarker for lung adenocarcinoma via bioinformatics and meta-analysis. Transl Cancer Res 2021;10:4870-83. [Crossref] [PubMed]
- Feng RM, Zong YN, Cao SM, et al. Current cancer situation in China: good or bad news from the 2018 Global Cancer Statistics? Cancer Commun (Lond) 2019;39:22. [Crossref] [PubMed]
- Kleczko EK, Kwak JW, Schenk EL, et al. Targeting the Complement Pathway as a Therapeutic Strategy in Lung Cancer. Front Immunol 2019;10:954. [Crossref] [PubMed]
- Yang J, Peng A, Wang B, et al. The prognostic impact of lymph node metastasis in patients with non-small cell lung cancer and distant organ metastasis. Clin Exp Metastasis 2019;36:457-66. [Crossref] [PubMed]
- Luo T, Chen Q, Zeng J. Analysis of lymph node metastasis in 200 patients with non-small cell lung cancer. Transl Cancer Res 2020;9:1577-83. [Crossref] [PubMed]
- Dai C, Xie H, Kadeer X, et al. Relationship of Lymph Node Micrometastasis and Micropapillary Component and Their Joint Influence on Prognosis of Patients With Stage I Lung Adenocarcinoma. Am J Surg Pathol 2017;41:1212-20. [Crossref] [PubMed]
- Guo Q, Wu CY, Jiang N, et al. Downregulation of T-cell cytotoxic marker IL18R1 promotes cancer proliferation and migration and is associated with dismal prognosis and immunity in lung squamous cell carcinoma. Front Immunol 2022;13:986447. [Crossref] [PubMed]
- Gong WJ, Zhou T, Wu SL, et al. A novel immune-related ceRNA network that predicts prognosis and immunotherapy response in lung adenocarcinoma. Ann Transl Med 2021;9:1484. [Crossref] [PubMed]
- Du J, Zheng L, Chen S, et al. NFIL3 and its immunoregulatory role in rheumatoid arthritis patients. Front Immunol 2022;13:950144. [Crossref] [PubMed]
- Guo Q, Liu XL, Liu HS, et al. The Risk Model Based on the Three Oxidative Stress-Related Genes Evaluates the Prognosis of LAC Patients. Oxid Med Cell Longev 2022;2022:4022896. [Crossref] [PubMed]
- Huang H, Wu W, Lu Y, et al. The development and validation of a m6A-lncRNAs based prognostic model for overall survival in lung squamous cell carcinoma. J Thorac Dis 2022;14:4055-72. [Crossref] [PubMed]
- Zhang YQ, Li K, Guo Q, et al. A New Risk Model Based on 7 Quercetin-Related Target Genes for Predicting the Prognosis of Patients With Lung Adenocarcinoma. Front Genet 2022;13:890079. [Crossref] [PubMed]
- Guo Q, Xiao XY, Wu CY, et al. Clinical Roles of Risk Model Based on Differentially Expressed Genes in Mesenchymal Stem Cells in Prognosis and Immunity of Non-small Cell Lung Cancer. Front Genet 2022;13:823075. [Crossref] [PubMed]
- Shen A, Ye Y, Chen F, et al. Integrated multi-omics analysis identifies CD73 as a prognostic biomarker and immunotherapy response predictor in head and neck squamous cell carcinoma. Front Immunol 2022;13:969034. [Crossref] [PubMed]
- Shepelev MV, Korobko IV. The RHOV gene is overexpressed in human non-small cell lung cancer. Cancer Genet 2013;206:393-7. [Crossref] [PubMed]
- Zhang D, Jiang Q, Ge X, et al. RHOV promotes lung adenocarcinoma cell growth and metastasis through JNK/c-Jun pathway. Int J Biol Sci 2021;17:2622-32. [Crossref] [PubMed]
- Chen Y, Zhou H, Yang S, et al. Increased ABCC2 expression predicts cisplatin resistance in non-small cell lung cancer. Cell Biochem Funct 2021;39:277-86. [Crossref] [PubMed]
- Qian CY, Zheng Y, Wang Y, et al. Associations of genetic polymorphisms of the transporters organic cation transporter 2 (OCT2), multidrug and toxin extrusion 1 (MATE1), and ATP-binding cassette subfamily C member 2 (ABCC2) with platinum-based chemotherapy response and toxicity in non-small cell lung cancer patients. Chin J Cancer 2016;35:85. [Crossref] [PubMed]
- Lim S, Alshagga M, Ong CE, et al. Cytochrome P450 4B1 (CYP4B1) as a target in cancer treatment. Hum Exp Toxicol 2020;39:785-96. [Crossref] [PubMed]
- Liu X, Jia Y, Shi C, et al. CYP4B1 is a prognostic biomarker and potential therapeutic target in lung adenocarcinoma. PLoS One 2021;16:e0247020. [Crossref] [PubMed]
- Zhao W, Zhang LN, Wang XL, et al. Long noncoding RNA NSCLCAT1 increases non-small cell lung cancer cell invasion and migration through the Hippo signaling pathway by interacting with CDH1. FASEB J 2019;33:1151-66. [Crossref] [PubMed]
- Yao D, Gu P, Wang Y, et al. Inhibiting polo-like kinase 1 enhances radiosensitization via modulating DNA repair proteins in non-small-cell lung cancer. Biochem Cell Biol 2018;96:317-25. [Crossref] [PubMed]
- Wang Y, Zhang J, Li YJ, et al. MEST promotes lung cancer invasion and metastasis by interacting with VCP to activate NF-κB signaling. J Exp Clin Cancer Res 2021;40:301. [Crossref] [PubMed]
- Zhang Q, Liu H, Zhang J, et al. MiR-142-5p Suppresses Lung Cancer Cell Metastasis by Targeting Yin Yang 1 to Regulate Epithelial-Mesenchymal Transition. Cell Reprogram 2020;22:328-36. [Crossref] [PubMed]
- Chao CC, Lee WF, Wang SW, et al. CXC chemokine ligand-13 promotes metastasis via CXCR5-dependent signaling pathway in non-small cell lung cancer. J Cell Mol Med 2021;25:9128-40. [Crossref] [PubMed]
- Sun J, Wang X, Liu W, et al. Novel evidence for retinoic acid-induced G (Rig-G) as a tumor suppressor by activating p53 signaling pathway in lung cancer. FASEB J 2020;34:11900-12. [Crossref] [PubMed]
- Zhang YQ, Yuan Y, Zhang J, et al. Evaluation of the roles and regulatory mechanisms of PD-1 target molecules in NSCLC progression. Ann Transl Med 2021;9:1168. [Crossref] [PubMed]
- Gao L, Xia L, Ji W, et al. Knockdown of CDK5 down-regulates PD-L1 via the ubiquitination-proteasome pathway and improves antitumor immunity in lung adenocarcinoma. Transl Oncol 2021;14:101148. [Crossref] [PubMed]
- Song R, Liu X, Zhu J, et al. RhoV mediates apoptosis of RAW264.7 macrophages caused by osteoclast differentiation. Mol Med Rep 2015;11:1153-9. [Crossref] [PubMed]
(English Language Editor: J. Jones)