Construction of a prognostic model for lung adenocarcinoma based on membrane-tension-related genes
Highlight box
Key findings
• We constructed a prediction model with a superior integrated performance in terms of membrane tension and immunity.
What is known and what is new?
• Membrane tension regulates the growth, migration, and differentiation of malignant tumor cells while regulating cellular metabolism.
• In this study, we developed a novel prognostic model based on membrane tension and combined with immune status. We also combined single-cell sequencing to further explore the predictive ability of membrane tension on the prognosis of LUAD patients.
What are the implications, and what should change now?
• Our results suggest that the model based on membrane tension genes is an accurate prognostic tool for LUAD patients, and can thus be applied in the clinic and guide treatment. In the future, more in vivo and in vitro experimental studies based on this model are needed.
Introduction
According to global cancer statistics, lung cancer is the most frequently diagnosed cancer (11.6% of total cases) and the leading cause of cancer deaths (18.4% of total cancer deaths) (1). Lung cancer is divided into small cell lung cancer and non-small cell lung cancer (NSCLC). The most common type of NSCLC is lung adenocarcinoma (LUAD), accounting for 40% of lung cancer cases. LUAD is one of the most aggressive and lethal tumors, with an overall survival (OS) of less than 5 years (2), whereas the average survival of patients who do not receive any treatment is typically only 2–4 months (3). Great advances have been made in the treatment of NSCLC over the past decade, with tyrosine kinase inhibitors (TKIs) for patients with EGFR, ALK, ROS1, and NTRK mutations, immune checkpoint inhibitors (ICIs), and a revolution in molecular testing that has dramatically changed the landscape of NSCLC treatment (4). Despite significant changes in treatment strategy, including TKI-based therapies, the 5-year OS rate for LUAD remains only 16% (5,6). With the increasing number of new drugs for the treatment of NSCLC (7), the traditional prognostic prediction system, including tumor staging and histological grading, make it difficult to accurately cover the clinical features of LUAD. Moreover, LUAD is a complex process involving multiple factors and genes, and various factors lead to different prognoses. Therefore, it is important to further understand the molecular mechanisms of LUAD occurrence and development for the prognosis and treatment of LUAD patients.
Although cell membranes and related structures have long been understood, the understanding of their roles in cancer treatment is lacking. Tumor cells have been compared to normal cells to determine the differences in lipid composition and membrane function (8). Tumors are usually stiffer than normal tissues and have an abnormally rapid glucose metabolism. Cellular tension regulates processes including cell growth, migration, and differentiation; these processes require a high level of energy, highlighting the role of cell tension in regulating cell metabolism (9). It has long been thought that cell mechanics are related to invasion and metastasis; however, the disruption of steady-state cytoplasmic membrane tension has been shown to be a common mechanical property of malignant cells and may be associated with cancer progression, with metastatic cells exhibiting significantly lower cytoplasmic membrane tension than epithelial cells. Furthermore, it has also been shown that reduced tension based on membrane-to-cortex attachment (MCA) promotes cancer cell migration and invasion through the self-assembly of Bin/amphiphysin/Rvs domain proteins (BAR proteins) into Arp2/3 complex-dependent actin polymerization and that the deletion of ezrin, radixin, moesin (ERM) proteins in less invasive breast cancer cells leads to a dramatic increase in breast cancer cell invasion (10).
Considering these findings, the role of membrane tension in tumor development has become known; however, whether membrane tension genes are associated with the prognosis of LUAD patients is unclear. The present study aimed to clarify the prognostic value of membrane-tension-related genes (MRGs) in LUAD and explore the correlation between membrane tension genes and various factors. In recent years, single-cell sequencing by second-generation sequencing has developed rapidly and its efficacy is significantly better than previous sequencing counts, allowing us to explore the heterogeneity of the cellular and tumor microenvironments (TME) at the single-cell level. This greatly improved our ability to study the transcriptional, genetic, and metabolic characteristics of thousands of individual cells, thereby deepening our analysis of the cells within tumor lesions (11). Single-cell technology provides the tools needed to differentiate solid tumors by analyzing intra-tumor heterogeneity (ITH), revealing the mechanisms of tumor metastasis and epigenetic changes, and ultimately guiding individualized treatment strategies.
Tumor cells often consist of multiple clonal subpopulations, even in a single lesion, and single-cell technology provides the tools needed to differentiate ITH in solid tumors, which provides better diagnosis at the molecular level, superior disease prognosis prediction, and effective treatment planning (12). By integrating clinicopathological information with single-cell sequencing data, new diagnostic and prognostic biomarkers and potential treatment-related cell types or states can be identified (13).
In this study, the messenger RNA (mRNA) expression profiles of LUAD and the corresponding clinical data were extracted from public databases, membrane tension genes associated with prognosis were identified, prognostic models were constructed to predict the prognosis of LUAD patients, and other analyses were performed to explore other potential mechanisms. We extracted LUAD-associated single-cell sequencing data and annotated them into descending, cellular subpopulations, and finally projected the prognosis-associated membrane tension genes into cellular subpopulations to explore the role of cells in the development and progression of LUAD and further validate the significance of prognosis-associated membrane tension genes. We present the following article in accordance with the TRIPOD reporting checklist (available at https://jtd.amegroups.com/article/view/10.21037/jtd-23-396/rc).
Methods
Data source and processing
The RNA sequencing and corresponding clinical characteristics data of LUAD were obtained from The Cancer Genome Atlas (TCGA) database. Clinical parameters including age, sex, grade, stage, and survival status were also evaluated. The downloaded data were processed into matrix files using the Perl programming language, and clinical information was extracted using the “XML::Simple” package. In addition, genes related to cell membrane tension were obtained from previous reports and literature. Single-cell RNA sequencing data were downloaded from the Gene Expression Omnibus (GEO) database (GSE200972) and then two tumor and normal tissue samples were respectively selected for each analysis. The study was conducted in accordance with the Declaration of Helsinki (as revised in 2013).
Identification of prognosis-related membrane tension genes and construction of a prognostic model
First, LUAD patients in TCGA database were randomly divided into three groups: a trial group, a test group, and all groups. To identify the potential prognosis-associated membrane tension genes, we first performed univariate Cox regression analysis on the trial cohort using the “survival” R package of the R programming language to initially screen for membrane tension-associated genes. Next, we employed the “glmnet” R package to perform least absolute shrinkage and selection operator (LASSO) cox regression analysis to screen for the best prognosis-associated membrane tension genes. Finally, we performed a multivariate cox regression analysis to identify five prognosis-associated membrane tension genes.
In the trial, test, and all sets, we calculated the risk score of patients based on the regression coefficient of each membrane tension and the expression value of its genes. The risk score of genes involved in the construction of the prognostic model was calculated according to the following equation:
Patients were divided into low- and high-risk groups based on the median risk scores. The risk scores and survival status for each sample were displayed using risk curves and scatter plots, and Kaplan-Meier survival curves were applied to demonstrate prognostic differences between the three groups. P<0.05 was selected as the significant cut-off value. To assess the accuracy of the prognostic model, the all and test groups were used to validate the trial group results. To further explore the functional and prognostic value of membrane tension genes, we used the “ConsesusClusterPlus” package to consistently cluster data from LUAD patients based on membrane tension gene expression, and Kaplan-Meier curves to visualize the OS and progression-free survival (PFS) subgroups.
Prediction and analysis of independent prognostic factors
Clinical data, including gender, age, and stage, were extracted from TCGA cohort and analyzed using univariate and multivariate Cox regression models and by combining the risk scores of the variables in the regression models. The “regplot” R package was used to plot column line plots and calibration plots to provide further comprehensive clinicopathological factors for LUAD prognosis. Receiver operating characteristic (ROC) curves were plotted using the “survminer” and “timeROC” R packages, and the prognostic value of the risk model associated with membrane tension genes was assessed by ROC curves and the area under the curve (AUC) values.
Gene Ontology (GO) functional enrichment analysis and gene set enrichment analysis (GSEA) enrichment analysis
Firstly, the “limma” R package was used to analyze the correlation between high- and low-risk differential genes in TCGA cohort, and the “clusterProfiler” package was used to conduct GO enrichment analysis of the high- and low-risk differential genes. We then performed a correlation analysis of the prognosis-related membrane tension genes with patient risk scores. Finally, gene enrichment analysis was performed on all genes in the high- and low-risk groups of TCGA cohort to explore possible biological functions or pathways. c2.cp.kegg.v7.5.1.symbols.gmt from the MSigDB database was used in the GSEA for pathway enrichment analysis.
Analysis of membrane tension genes with related variables and classification
We downloaded LUAD gene copy data from the University of California Santa Cruz (UCSC) Xena (https://xenabrowser.net/datapages/) database, then extracted the gene copy data related to membrane tension genes to show their copy number variation (CNV) frequencies, and used the “RCircos” package to plot the distribution of gene copy number chromosomes. In addition, we also examined the correlation between membrane tension gene risk scores, tumor mutation burden (TMB) scores, and cancer stem cell (CSC) scores and performed a survival analysis of the TMB versus patient risk groups.
TME and related immunity
The level of infiltration of 16 immune cells and 13 immune-related pathways between the two risk groups was quantified by performing single-sample gene set enrichment analysis (ssGSEA) using the “GSVA” package. Using the inverse convolution CIBERSORT algorithm, the relative proportions of 22 infiltrating immune cells in the high- and low-risk groups of the membrane tension genes used to construct the prognostic model were determined, and differences in the expression of immune checkpoint (ICP) genes in the high- and low-risk groups were investigated by screening for differential ICP genes (using P<0.05 as the threshold).
Clustering and reduced dimensionality of single cell RNA Seq (scRNA-seq) data and distribution of the prognosis-related membrane tension genes
First, the selected four-sample data were merged, and the expression data was normalized with “Seurat” and “NormalizeData” R packages. We identified the top 1,000 highly variable genes using the “FindVariableFeatures” function, scaled all genes using the “ScaleData” function, and performed principal-component-analysis (PCA) downscaling on the screened 1,000 highly variable genes using the PCA function. The cells were clustered by the “FindNeighbors” and “FindClusters” functions, and the data were downscaled using uniform manifold approximation and projection (UMAP), which is an algorithm that maps high-dimensional space to low-dimensional space to achieve a final dimensionality reduction. The “FindAllMarkers” function was utilized to set the parameters. The Wilcoxon rank sum test was applied to determine the differentially expressed genes between different groups of cells, classify different groups of cells according to the gene expression values, and filter the genes with LogFC =0.25 (difference multiplicity). The cells were finally divided into nine subpopulations, and the CellMarker (http://biocc.hrbmu.edu.cn/CellMarker/) human cell marker genes were referenced and the cell subpopulations were annotated with reference to the relevant literature. P<0.05 was used to screen the marker genes and determine the prognosis-related membrane tension genes in the cell subpopulations’ distribution.
Statistical analysis
The R software (version 4.2.1) and various R packages were used for conducting the data analysis and visualizing the data. The results of the analysis were made statistically significant at P<0.05.
Results
Screening for prognosis-related membrane tension genes and classification of samples based on membrane tension genes
The flow chart of this study is shown in Figure 1. To construct the prognostic model, we performed univariate Cox regression analysis, LASSO cox regression analysis, and multivariate cox regression analysis on the experimental group (Figure 2A,2B). Finally, five prognosis-related membrane tension genes (FLG, SLK, CFL1, PECAM1, and ITGB1) were screened. To further explore the association between the membrane tension genes and the sample data from LUAD patients, we performed consensus cluster analysis on all samples from the TCGA cohort and found the highest intra-group correlation and low inter-group correlation when k=2, indicating that LUAD patients can be divided into two groups based on membrane tension genes. We also noted that the PFS (P<0.004) and OS (P<0.001) were longer in cluster 1 than in cluster 2 (Figure 2C-2E). We then predicted the prognosis of LUAD patients according to the high and low expression of prognostic genes and found that patients with low expression of SLK, ITGB1, and CFL1 had a better prognosis (Figures S1-S5).
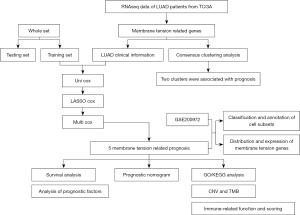

Construction and validation of the prognostic risk model
Patients were divided into high- and low-risk groups based on the median risk values. Heat maps were used to display the expression levels of five prognosis-related membrane tension genes in the high- and low-risk groups (Figure S6), and the prognostic risk scores for each LUAD patient in the three groups were calculated. The risk score and survival status of membrane tension-related gene expression levels in test, train, and all groups were visualized (Figure 3A-3F). The survival time of patients in the low-risk group was significantly higher than that in the high-risk group. To assess the prognostic impact of high and low risk scores, we evaluated the likelihood of survival of patients in the three groups using Kaplan-Meier curves (Figure 3G-3I), and the prognosis of low-risk patients was better than that of patients in the high-risk group. Overall, the results, validated by the test group and all groups, showed that the prognostic risk model based on prognosis-related membrane tension genes had good accuracy in predicting survival and prognosis in LUAD.
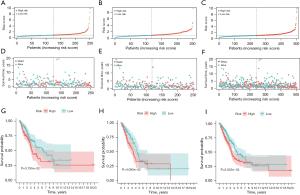
Independent prognostic value of risk scores
Univariate and multivariate cox regression analyses were performed in this study to explore whether the risk score was an independent prognostic factor. Multivariate cox regression analysis of the risk score (P<0.001, HR =1.054, 95% CI: 1.034–1.075) also showed that it could be used as an independent prognostic factor (Figure 4A). Furthermore, univariate analysis demonstrated that the risk score (P<0.001, HR =1.060, 95% CI: 1.040–1.080) showed a statistical difference (Figure 4B). Given the marked correlation between risk score and patient prognosis, we combined the relevant clinical parameters to construct a column line plot, which was used to assess LUAD patient survival rates at 1, 3, and 5 years (Figure 4C). Similarly, tumor staging can also serve as an independent prognostic factor for predicting the prognosis of LUAD patients. Therefore, we assessed the correlation between the five membrane-tension prognosis-related genes (5-MRG) risk score and TNM staging, and our results showed a significant correlation between the 5-MRG signature and TNM staging (P<0.05), indicating a statistical difference (Figure S7). As the risk score increased, the probability of progressing to an advanced tumor gradually increased, suggesting that our MRG signature may play a pivotal role in the progression of LUAD. The calibration curve of the column line plot showed high accuracy between the actual and predicted incidence (Figure 4D). Subsequently, ROC analysis was performed to evaluate the predictive value of the risk score model. The AUC for the risk score model was 0.699, which was relatively better than the other variables (Figure 4E). Finally, regarding the survival times at 1, 2, and 3 years, the AUC values of the risk score model were 0.699, 0.656, and 0.657, respectively (Figure 4F).
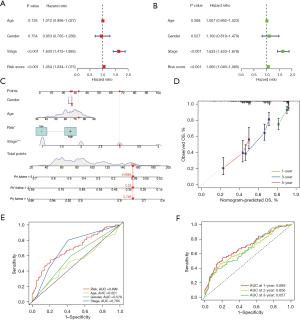
GO functional enrichment analysis and GSEA
To explore the biological functions and pathways leading to differences between the high- and low-risk groups, GO enrichment analysis was performed based on the differential genes between these two groups in the TCGA-LUAD cohort. We found that the differential genes between the high- and low-risk groups are significantly enriched in the biological process of humoral immune response (Figure 5A). Analysis of the correlation between prognosis-related membrane tension genes and patient risk scores showed a significant positive correlation between FLG and patient risk scores (Figure 5B).
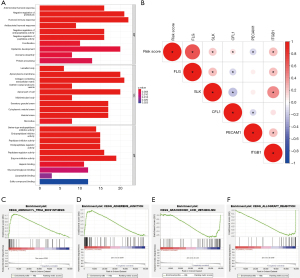
Subsequently, we conducted GSEA on all genes in the high- and low-risk groups of the TCGA cohort, and found two pathways enriched in the high-risk group (Figure 5C,5D): “AMINOACYL_TRNA_BIOSYNTHESIS” and “ADHERENS_JUNCTION”, and two pathways enriched in the low-risk group: “ARACHIDONIC_ACID_METABOLISM” and “ALLOGRAFT_REJECTION” (Figure 5E,5F).
Association between membrane tension genes and CNV, TMB, and CSC scores
First, we explored the incidence of CNV mutations, which showed that 44 MRGs exhibited significant CNV alterations (Figure 6A). The circle diagram displays the CNV alteration loci of 44 membrane tension-related genes on the chromosome (Figure 6B). Therefore, we concluded that CNVs may play a regulatory role in the expression of membrane tension genes. According to the risk score, the mutant genes were divided into high- and low-risk groups, and the top 20 mutant genes were selected. We found that the mutation frequency of the high-risk group was higher than that of the low-risk group (Figure 6C,6D).
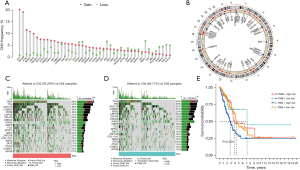
Previous study has shown that TMB is a valuable predictor of tumor immune response, and high TMB can benefit from ICIs (14). We analyzed the high and low TMB of patients in the high- and low-risk groups, and the results showed that patients with a high TMB in the low-risk group had better prognoses and longer survival times (Figure 6E), indicating that patients with a high TMB in the low-risk group may benefit from immunotherapy.
TME and immune activity between the subgroups
The risk and immune scores differed between the high- and low-risk groups (P<0.001), and immune cells were more abundant in the low-risk group (Figure 7A). The differences in the infiltration levels of 22 immune cells between the high- and low-risk groups were assessed by CIBERSORT. Among the LUAD patients, the levels of activated CD4 memory T cells (P<0.001), resting natural killer (NK) cells (P<0.01), and M0 macrophages (P<0.001) were higher in the high-risk group than in the low-risk group, while patients in the low-risk group exhibited higher levels of resting dendritic cells (P<0.001) and resting mast cells (P<0.001) than those in the high-risk group (Figure 7B).
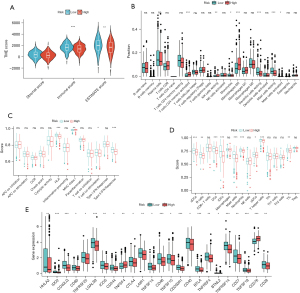
Next, we further compared the enrichment fraction of 16 immune cells and the activity of 13 immune-related pathways among subgroups in TCGA cohort by ssGSEA. Among the 13 immune-related pathways, the activity of human leukocyte antigen (HLA) and type II interferon (IFN) response pathways was higher in the low-risk group relative to that in the high-risk group (Figure 7C). In terms of immune cell infiltration, the high-risk group was mostly lower than the low-risk group (Figure 7D), especially in activated dendritic cells (ADCs), B cells, DCs, iDCs, mast cells, neutrophils, T helper cells, and tumor-infiltrating lymphocytes (TILs). We then performed a differential analysis of the ICP in the high- and low-risk groups (Figure 7E), which showed that 24 ICPs, such as LGALS9, TNFRSF14, CD276, etc., performed differently in both risk subgroups.
Cell subpopulation annotation and distribution of prognosis-related membrane tension genes
In this study, single-cell sequencing data from two lung cancer tissue samples and two normal tissue samples were analyzed, and the sample data were further normalized after quality control. A total of 1,000 highly variable genes were screened out and the top 10 most changed genes (Figure S8) were used for the follow-up study. By downscaling the cellular data of the four samples and selecting a principal component (PC) of 20 as the clustering parameter (Figure S9), nine cellular clusters were finally identified (Figure 8A). After annotation, we observed the distribution of prognosis-related membrane tension genes in these nine cell clusters (Figure 8B). It can be seen from that ITGB1 expression was higher in endothelial cells and fibroblasts, PECAM1 expression was higher in endothelial cells, CFL1 was expressed in all subpopulations of cells but was most highly expressed in NK cells, and SLK expression was relatively higher in endothelial cells. These findings were corroborated in the bubble plots (Figure 8C,8D).
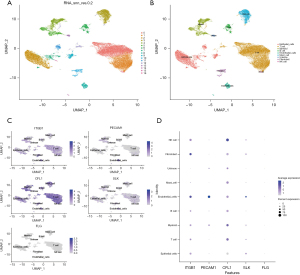
Discussion
At present, lung cancer still has high incidence and mortality rates (15). LUAD, the main form of lung cancer, is the deadliest cancer worldwide because of its late diagnosis and high heterogeneity (16). More accurate prognostic prediction and exploration of new therapeutic targets are urgently needed clinical treatment. Due to the heterogeneity of LUAD patients in terms of their clinical, molecular, and pathological aspects, it is difficult to customize individualized treatment and predict the outcomes of LUAD patients (17). Considering that the immune environment plays an important role in cancer progression, the search for new immune biomarkers is also important for the prognosis of LUAD patients. Previous study has found that ERM proteins, which maintain membrane-actin adhesion in cancer cells, can be dissociated from the cell membrane, thereby making the cancer cell membrane more flexible, while cancer cells with hard membrane structures lose their ability to spread and metastasize (18). In metastatic cells, the process of tumor invasion and metastasis is inhibited by manipulating the membrane cortex to increase plasma membrane tension and impair BAR protein-mediated signaling (19).
In this study, five membrane tension genes (FLG, SLK, CFL1, PECAM1, and ITGB1) associated with LUAD prognosis were screened, and a risk model was established to predict the prognosis of LUAD. Patients were divided into high- and low-risk groups according to the median risk score, and the prognosis of patients in the high-risk group was significantly worse. ROC curve analysis showed that the prognostic model had good predictive ability.
SLK, a member of the Ste20 family, is located in the nucleus, cytoplasmic lysosomes, microtubules, and centrosomes, and is associated with a variety of biological processes, such as growth, proliferation, survival, and cell migration (20). It has been shown that SLK is a novel adhesion dissociation signal that is activated and recruited downstream of FAK/Src, and its dependent signal is required to mediate microtubule-dependent focal adhesion channels (21). SLK negatively regulates RhoA-dependent function by phosphorylating RhoA at Ser188.15. These findings suggest that SLK is a novel relaxation signal involved in cytoskeleton remodeling and cell migration (22). In a breast cancer study, SLK was shown to be activated downstream of HRGb stimulation in 4T1 breast cancers, and negative SLK expression or siRNA-mediated knockdown of expression leads to impaired motility and invasion, suggesting SLK as a potential therapeutic target (23).
Members of the integrin beta superfamily (ITGB) have been documented to play important roles in a variety of biological processes, and there is growing evidence linking ITGB to the oncogenic effects of several malignancies (24). ITGB1 exerts a biological function in cell migration and invasion and is abnormally expressed in several cancer types, including breast, lung, stomach, prostate, pancreatic, colorectal, and laryngeal cancers, mediating cell migration, invasion, survival, and promoting the malignant phenotype of tumors. It was shown that ITGB1 knockdown significantly increased the expression of the epithelial marker, E-cadherin, and decreased the expression of the mesenchymal cell markers wave protein and fibronectin by polymerase chain reaction (PCR) and protein blotting assays, confirming the role of ITGB1 in promoting epithelial-mesenchymal transition (EMT) progression. Meanwhile, ITGB1 knockdown inhibited cell proliferation, invasion, EMT, and cancer stem cell (CSC)-like properties (25).
In a study of pancreatic ductal adenocarcinoma (PDAC), ITGB1 expression was significantly increased in PDAC compared to normal pancreatic tissue and was associated with transforming growth factor (TGF)-β, EMT, inflammation, stemness, and angiogenic pathways. It was concluded that PDAC had worse outcomes in tumors with high ITGB1 expression (26). Similarly, CFL1 plays an important role in cytoplasmic division, cell motility, and morphogenesis (27). It has been reported to be associated with the ability of cancer cells to metastasize and invade, which is an important feature of tumor cells in solid tumor tissues (27,28). In a study related to the role of CFL1 in vulvar squamous cell carcinoma (VSCC), it was noted that CFL1 silencing decreased the protein expression of MMP2 and MMP9, implying that CFL1 silencing inhibits tumor invasion and metastasis by suppressing the degradation of the extracellular matrix (29). In a study on LUAD, it was noted that enhanced expression of CFL1 was correlated with the severity of epithelial dysplasia and a relatively poor prognosis. The expression of CFL1 shRNA significantly inhibited tumor metastasis and prolonged survival in an LL/2 (Lewis lung carcinoma cell line) metastasis model (30). These studies suggest that CFL1 could be used as a potential diagnostic and prognostic biomarker.
To determine the role of membrane tension-related signaling pathways in different risk groups, GO and Kyoto Encyclopedia of Genes and Genomes (KEGG) analyses were performed on the differential genes in the high- and low-risk groups. The results showed that biological processes, cytological components, and molecular biological functions in GO enrichment analysis all played significant roles in the regulation of membrane tension, with humoral immune responses, collagen-containing extracellular matrix, and enzyme inhibitor activity being the most significant roles. In the KEGG enrichment analysis, pathways were observed to be enriched in high- and low-risk groups, with AMINOACYL_TRNA_BIOSYNTHESIS and ADHERENS_JUNCTION enriched in the high-risk group and arachidonic acid (AA) enriched in the low-risk group. In the “AMINOACYL_TRNA_BIOSYNTHESIS” pathway. Aminoacyl-transfer RNA (tRNA) synthetase (ARS) is an essential enzyme that participates in protein synthesis by catalyzing the activation of amino acids and linking them to their homologous tRNA, eight ARSs, and three ARS interacting multifunctional proteins (AIMPs) to form a multi-tRNA synthetase complex (MSC) (31,32). ARS is not only an essential enzyme for the covalent attachment of substrate amino acids to homologous tRNA for protein synthesis but also acts as a regulator of cellular processes by detecting different cellular conditions. Due to its catalytic role in protein synthesis and its regulatory role in homeostasis in vivo, altered or dysfunctional ARS may be pathologically relevant to tumorigenesis (33).
AIMP2 lacking exon 2 (AIMP2-DX2) could be a potential biomarker for lung cancer and may also be involved in tumorigenesis. A study has shown that shRNA downregulation of AIMP2-DX2 expression inhibits the EGFR/MAPK signaling pathway, thereby suppressing glucose uptake and cancer cell growth (34). Together, it appears that the ARS expression profile could be a useful prognostic tool for cancer. High ARS expression is usually associated with shorter patient survival. The activity of ARS associated with cancer is expected to provide new opportunities for the detection, prevention, and treatment of cancer (33,35). In the “ADHERENS_JUNCTION” pathway, Afadin is positioned at the adhesion junctions (36). A study evaluating the effect of Afadin reorientation on intercellular adhesion by immunofluorescence-measured E-cadherin staining showed that Afadin exhibited definite intercellular adhesion, and that Afadin knockout with a specific shRNA reduced cell migration (37). The removal of Afadin from the adhesion junctions is a system of cell migration that has a mechanism of promoting cancer progression (38). The AA pathway is a metabolic process in which prostaglandin G/H synthase (PGHS) metabolizes AA to prostaglandin H2 (PGH2), which are substrates for a series of downstream enzymes that produce specific prostaglandins (PGs), namely PGE2, PGI2, PGD2, PGF2, and TXA2 (39,40). PGE2 has the highest tumorigenic and metastatic potential, as it plays a key role in carcinogenesis by inhibiting apoptosis and increasing the invasiveness of cancer cells as well as promoting angiogenesis in tumors (41). Therefore, the AA pathway metabolizing enzymes, phospholipases A2s (PLA2s), cyclooxygenases (COXs), and lipoxygenases (LOXs), and their metabolites (such as prostaglandins and leukotrienes), have been identified as novel targets for the prevention and treatment of cancer (42).
ssGSEA showed significant differences in the infiltration scores of immune cells and immune-related pathways in the different risk groups. This study found that HLA, type II IFN response pathways, and DCs were significantly higher in the low-risk group, where HLA-I and HLA-II refer to the major histocompatibility complex (MHC) class I and II proteins found in humans, with HLA-I molecules being detectable on the surface of almost all nucleated cells and essential for the activation of CD8+ T cell responses (43). CD8+ T-cell-dependent killing of cancer cells requires HLA-I molecules for effective tumor antigen presentation, where the presence of HLA-1 heterogeneity and tumors carrying a high mutational load result in significantly increased survival times after checkpoint blockade therapy (44). Type II interferon only contains IFN-γ, which is the most important antitumor immune cytokine; IFN-γ deficiency leads to malignant lung epithelial tumors in mice (45). Meanwhile, IFN-γ induces the expression of PD-L1, and tumor patients expressing IFN-g and PD-L1 have a better prognosis. In LUAD cells, IFN-γ-induced activation of the JAK2-STAT1 pathway, which upon activation is responsible for the antiproliferative effect of IFN-γ, confirms the anti-cancer ability of IFN-γ (46). DCs are a rare heterogeneous population of leukocytes that play an important role in the induction and regulation of innate and adaptive immune responses. DCs capture tumor-associated antigens released from tumor cells and present them to CD8+ T cells for recognition, thereby killing cancer cells (47), which is consistent with the above findings.
Blocking the ICP pathway becomes a treatment against cancer (48). In this study, there were significant differences in many ICPs between the high- and low-risk groups. In the present study, we analyzed the high expression of LGALS9 in the low-risk group and the high expression of CD276 in the high-risk group. LGALS9 belongs to the galactose lectin family of proteins and contains a conserved carbohydrate recognition domain (49). It was first identified as an eosinophil chemoattractant and activator, regulating a variety of biological functions, including cell aggregation and adhesion as well as tumor cell apoptosis. Recombinant galactoglucan lectin-9 treatment prevents metastatic spread in various preclinical cancer models (50). A study on lung cancer showed that LGALS9 was expressed in all pathological types of NSCLC, and the level of LGALS9 was correlated with the level of other checkpoints, including PD-1 and PD-L1. The results showed that higher levels of LGALS9 in tumor cells resulted in a higher OS (51). Another study indicated that LGALS9 could improve the adhesion of cultured cancer cells and prevent the metastasis of tumor cells. Patients with LGALS9-positive tumors have a lower chance of metastasis compared to patients with LGALS9-negative tumors (52,53). CD276 is an ICP molecule and a co-stimulatory/co-inhibitory immunomodulatory protein that plays a dual role in the immune system (54); its expression in tumors is associated with poor prognosis. In addition to its role in immune regulation, it also promotes pro-tumor functions such as tumor migration, invasion, metastasis, resistance, and metabolism (55). CD276 is closely related to the invasion, metastasis, proliferation, and prognosis of NSCLC tumors. A study has reported that the expression of CD276 in NSCLC tissues is significantly up-regulated, and its expression is positively correlated with the tumor stage of NSCLC. Silencing CD276 inhibits cell invasion and migration by reducing integrin-related protein expression (56). This is consistent with the results of our ICP difference analysis in the high- and low-risk groups, which provides a new perspective on immunotherapy in LUAD.
Finally, in this study, the cells were divided into nine subpopulations by single-cell sequencing analysis, and the expression of five prognosis-related membrane tension genes was analyzed in each cell subpopulation. It was found that the expression level of PECAM-1 was higher in endothelial cells. A previous study showed that PECAM-1 not only delays endothelial cell migration but also inhibits junctional repair (57). PECAM-1-regulated paracrine factors drive the lethal progression of advanced tumor metastases, and anti-PECAM-1 mAb prevents this progression by altering the release of soluble factors that drive proliferation within these advanced metastases (58). These studies suggest a role in endothelial cells through PECAM-1 as a potential targeted therapeutic approach.
This study was based on the currently reported relationship between membrane tension genes and prognosis in LUAD patients. We analyzed several prognosis-related membrane tension genes and constructed a novel membrane tension-related prognosis model, and also confirmed the good predictive performance of the model through relevant data. We hypothesized that analysis of membrane tension in relation to LUAD at the single-cell level might more accurately verify its significance for the progression and prognosis of LUAD patients. However, our study still has some limitations. Firstly, we used retrospective data to establish and validate the prognostic signature. Secondly, the potential biological functions of prognosis-associated membrane tension genes with their cellular subpopulations in single-cell analysis and their molecular mechanisms need to be further investigated. In summary, we constructed a novel prognostic risk model with independent prognostic value to help clinicians provide a meaningful reference for the prognosis and treatment of LUAD patients.
Conclusions
After a series of analyses and validation, our study showed that the prognostic model based on the expression levels of five MRGs (FLG, SLK, CFL1, PECAM1, and ITGB1) could accurately predict the prognosis of LUAD patients. The relevant role of MRGs at the immune level was also explored and their significance was elucidated.
Acknowledgments
Thanks to TCGA and GEO databases for providing the platform and the contributors for uploading the meaningful data.
Funding: None.
Footnote
Reporting Checklist: The authors have completed the TRIPOD reporting checklist. Available at https://jtd.amegroups.com/article/view/10.21037/jtd-23-396/rc
Peer Review File: Available at https://jtd.amegroups.com/article/view/10.21037/jtd-23-396/prf
Conflicts of Interest: All authors have completed the ICMJE uniform disclosure form (available at https://jtd.amegroups.com/article/view/10.21037/jtd-23-396/coif). The authors have no conflicts of interest to declare.
Ethical Statement: The authors are accountable for all aspects of the work in ensuring that questions related to the accuracy or integrity of any part of the work are appropriately investigated and resolved. The study was conducted in accordance with the Declaration of Helsinki (as revised in 2013).
Open Access Statement: This is an Open Access article distributed in accordance with the Creative Commons Attribution-NonCommercial-NoDerivs 4.0 International License (CC BY-NC-ND 4.0), which permits the non-commercial replication and distribution of the article with the strict proviso that no changes or edits are made and the original work is properly cited (including links to both the formal publication through the relevant DOI and the license). See: https://creativecommons.org/licenses/by-nc-nd/4.0/.
References
- Bray F, Ferlay J, Soerjomataram I, et al. Global cancer statistics 2018: GLOBOCAN estimates of incidence and mortality worldwide for 36 cancers in 185 countries. CA Cancer J Clin 2018;68:394-424. [Crossref] [PubMed]
- Denisenko TV, Budkevich IN, Zhivotovsky B. Cell death-based treatment of lung adenocarcinoma. Cell Death Dis 2018;9:117. [Crossref] [PubMed]
- Demedts IK, Vermaelen KY, van Meerbeeck JP. Treatment of extensive-stage small cell lung carcinoma: current status and future prospects. Eur Respir J 2010;35:202-15. [Crossref] [PubMed]
- Hirsch FR, Scagliotti GV, Mulshine JL, et al. Lung cancer: current therapies and new targeted treatments. Lancet 2017;389:299-311. [Crossref] [PubMed]
- DeSantis CE, Lin CC, Mariotto AB, et al. Cancer treatment and survivorship statistics, 2014. CA Cancer J Clin 2014;64:252-71. [Crossref] [PubMed]
- Pao W, Girard N. New driver mutations in non-small-cell lung cancer. Lancet Oncol 2011;12:175-80. [Crossref] [PubMed]
- Akhurst T. Staging of Non-Small-Cell Lung Cancer. PET Clin 2018;13:1-10. [Crossref] [PubMed]
- Zalba S, Ten Hagen TL. Cell membrane modulation as adjuvant in cancer therapy. Cancer Treat Rev 2017;52:48-57. [Crossref] [PubMed]
- Park JS, Burckhardt CJ, Lazcano R, et al. Mechanical regulation of glycolysis via cytoskeleton architecture. Nature 2020;578:621-6. [Crossref] [PubMed]
- Singh V, Lamaze C. Membrane tension buffering by caveolae: a role in cancer? Cancer Metastasis Rev 2020;39:505-17. [Crossref] [PubMed]
- Lei Y, Tang R, Xu J, et al. Applications of single-cell sequencing in cancer research: progress and perspectives. J Hematol Oncol 2021;14:91. [Crossref] [PubMed]
- Liang SB, Fu LW. Application of single-cell technology in cancer research. Biotechnol Adv 2017;35:443-9. [Crossref] [PubMed]
- Rozenblatt-Rosen O, Regev A, Oberdoerffer P, et al. The Human Tumor Atlas Network: Charting Tumor Transitions across Space and Time at Single-Cell Resolution. Cell 2020;181:236-49. [Crossref] [PubMed]
- Addeo A, Friedlaender A, Banna GL, et al. TMB or not TMB as a biomarker: That is the question. Crit Rev Oncol Hematol 2021;163:103374. [Crossref] [PubMed]
- Torre LA, Siegel RL, Jemal A. Lung Cancer Statistics. Adv Exp Med Biol 2016;893:1-19. [Crossref] [PubMed]
- Seguin L, Durandy M, Feral CC. Lung Adenocarcinoma Tumor Origin: A Guide for Personalized Medicine. Cancers (Basel) 2022;14:1759. [Crossref] [PubMed]
- Nakamura H, Takagi M. Clinical impact of the new IASLC/ATS/ERS lung adenocarcinoma classification for chest surgeons. Surg Today 2015;45:1341-51. [Crossref] [PubMed]
- Ponuwei GA. A glimpse of the ERM proteins. J Biomed Sci 2016;23:35. [Crossref] [PubMed]
- Tsujita K, Satow R, Asada S, et al. Homeostatic membrane tension constrains cancer cell dissemination by counteracting BAR protein assembly. Nat Commun 2021;12:5930. [Crossref] [PubMed]
- Fanger GR, Gerwins P, Widmann C, et al. MEKKs, GCKs, MLKs, PAKs, TAKs, and tpls: upstream regulators of the c-Jun amino-terminal kinases? Curr Opin Genet Dev 1997;7:67-74. [Crossref] [PubMed]
- Wagner SM, Sabourin LA. A novel role for the Ste20 kinase SLK in adhesion signaling and cell migration. Cell Adh Migr 2009;3:182-4. [Crossref] [PubMed]
- Guilluy C, Rolli-Derkinderen M, Loufrani L, et al. Ste20-related kinase SLK phosphorylates Ser188 of RhoA to induce vasodilation in response to angiotensin II Type 2 receptor activation. Circ Res 2008;102:1265-74. [Crossref] [PubMed]
- Roovers K, Wagner S, Storbeck CJ, et al. The Ste20-like kinase SLK is required for ErbB2-driven breast cancer cell motility. Oncogene 2009;28:2839-48. [Crossref] [PubMed]
- Liu D, Liu S, Fang Y, et al. Comprehensive Analysis of the Expression and Prognosis for ITGBs: Identification of ITGB5 as a Biomarker of Poor Prognosis and Correlated with Immune Infiltrates in Gastric Cancer. Front Cell Dev Biol 2021;9:816230. [Crossref] [PubMed]
- Guo L, Sun C, Xu S, et al. Knockdown of long non-coding RNA linc-ITGB1 inhibits cancer stemness and epithelial-mesenchymal transition by reducing the expression of Snail in non-small cell lung cancer. Thorac Cancer 2019;10:128-36. [Crossref] [PubMed]
- Benesch MG, Wu R, Menon G, et al. High beta integrin expression is differentially associated with worsened pancreatic ductal adenocarcinoma outcomes. Am J Cancer Res 2022;12:5403-24. [PubMed]
- Hotulainen P, Paunola E, Vartiainen MK, et al. Actin-depolymerizing factor and cofilin-1 play overlapping roles in promoting rapid F-actin depolymerization in mammalian nonmuscle cells. Mol Biol Cell 2005;16:649-64. [Crossref] [PubMed]
- Wang W, Mouneimne G, Sidani M, et al. The activity status of cofilin is directly related to invasion, intravasation, and metastasis of mammary tumors. J Cell Biol 2006;173:395-404. [Crossref] [PubMed]
- Wu Q, Jiang Y, Cui S, et al. The role of cofilin-l in vulvar squamous cell carcinoma: A marker of carcinogenesis, progression and targeted therapy. Oncol Rep 2016;35:2743-54. [Crossref] [PubMed]
- Peng XC, Gong FM, Zhao YW, et al. Comparative proteomic approach identifies PKM2 and cofilin-1 as potential diagnostic, prognostic and therapeutic targets for pulmonary adenocarcinoma. PLoS One 2011;6:e27309. [Crossref] [PubMed]
- Zhou Z, Sun B, Huang S, et al. Roles of aminoacyl-tRNA synthetase-interacting multi-functional proteins in physiology and cancer. Cell Death Dis 2020;11:579. [Crossref] [PubMed]
- Zhou Z, Sun B, Nie A, et al. Roles of Aminoacyl-tRNA Synthetases in Cancer. Front Cell Dev Biol 2020;8:599765. [Crossref] [PubMed]
- Sung Y, Yoon I, Han JM, et al. Functional and pathologic association of aminoacyl-tRNA synthetases with cancer. Exp Mol Med 2022;54:553-66. [Crossref] [PubMed]
- Chang SH, Chung YS, Hwang SK, et al. Lentiviral vector-mediated shRNA against AIMP2-DX2 suppresses lung cancer cell growth through blocking glucose uptake. Mol Cells 2012;33:553-62. [Crossref] [PubMed]
- Kwon NH, Fox PL, Kim S. Aminoacyl-tRNA synthetases as therapeutic targets. Nat Rev Drug Discov 2019;18:629-50. [Crossref] [PubMed]
- Takai Y, Nakanishi H. Nectin and afadin: novel organizers of intercellular junctions. J Cell Sci 2003;116:17-27. [Crossref] [PubMed]
- Huxham J, Tabariès S, Siegel PM. Afadin (AF6) in cancer progression: A multidomain scaffold protein with complex and contradictory roles. Bioessays 2021;43:e2000221. [Crossref] [PubMed]
- Elloul S, Kedrin D, Knoblauch NW, et al. The adherens junction protein afadin is an AKT substrate that regulates breast cancer cell migration. Mol Cancer Res 2014;12:464-76. [Crossref] [PubMed]
- Smith WL, DeWitt DL, Garavito RM. Cyclooxygenases: structural, cellular, and molecular biology. Annu Rev Biochem 2000;69:145-82. [Crossref] [PubMed]
- Rich SA. The coxibs, selective inhibitors of cyclooxygenase-2. N Engl J Med 2001;345:1709. [PubMed]
- Dufour M, Faes S, Dormond-Meuwly A, et al. PGE2-induced colon cancer growth is mediated by mTORC1. Biochem Biophys Res Commun 2014;451:587-91. [Crossref] [PubMed]
- Yarla NS, Bishayee A, Sethi G, et al. Targeting arachidonic acid pathway by natural products for cancer prevention and therapy. Semin Cancer Biol 2016;40-41:48-81. [Crossref] [PubMed]
- Wieczorek M, Abualrous ET, Sticht J, et al. Major Histocompatibility Complex (MHC) Class I and MHC Class II Proteins: Conformational Plasticity in Antigen Presentation. Front Immunol 2017;8:292. [Crossref] [PubMed]
- Chowell D, Morris LGT, Grigg CM, et al. Patient HLA class I genotype influences cancer response to checkpoint blockade immunotherapy. Science 2018;359:582-7. [Crossref] [PubMed]
- Shankaran V, Ikeda H, Bruce AT, et al. IFNgamma and lymphocytes prevent primary tumour development and shape tumour immunogenicity. Nature 2001;410:1107-11. [Crossref] [PubMed]
- Gao Y, Yang J, Cai Y, et al. IFN-γ-mediated inhibition of lung cancer correlates with PD-L1 expression and is regulated by PI3K-AKT signaling. Int J Cancer 2018;143:931-43. [Crossref] [PubMed]
- Lee YS, Radford KJ. The role of dendritic cells in cancer. Int Rev Cell Mol Biol 2019;348:123-78. [Crossref] [PubMed]
- Li B, Chan HL, Chen P. Immune Checkpoint Inhibitors: Basics and Challenges. Curr Med Chem 2019;26:3009-25. [Crossref] [PubMed]
- Nagae M, Nishi N, Nakamura-Tsuruta S, et al. Structural analysis of the human galectin-9 N-terminal carbohydrate recognition domain reveals unexpected properties that differ from the mouse orthologue. J Mol Biol 2008;375:119-35. [Crossref] [PubMed]
- Fujihara S, Mori H, Kobara H, et al. Galectin-9 in cancer therapy. Recent Pat Endocr Metab Immune Drug Discov 2013;7:130-7. [Crossref] [PubMed]
- He Y, Jia K, Dziadziuszko R, et al. Galectin-9 in non-small cell lung cancer. Lung Cancer 2019;136:80-5. [Crossref] [PubMed]
- Irie A, Yamauchi A, Kontani K, et al. Galectin-9 as a prognostic factor with antimetastatic potential in breast cancer. Clin Cancer Res 2005;11:2962-8. [Crossref] [PubMed]
- Yamauchi A, Kontani K, Kihara M, et al. Galectin-9, a novel prognostic factor with antimetastatic potential in breast cancer. Breast J 2006;12:S196-200. [Crossref] [PubMed]
- Hofmeyer KA, Ray A, Zang X. The contrasting role of B7-H3. Proc Natl Acad Sci U S A 2008;105:10277-8. [Crossref] [PubMed]
- Malapelle U, Parente P, Pepe F, et al. B7-H3/CD276 Inhibitors: Is There Room for the Treatment of Metastatic Non-Small Cell Lung Cancer? Int J Mol Sci 2022;23:16077. [Crossref] [PubMed]
- Li F, Chen H, Wang D. Silencing of CD276 suppresses lung cancer progression by regulating integrin signaling. J Thorac Dis 2020;12:2137-45. [Crossref] [PubMed]
- Mei H, Campbell JM, Paddock CM, et al. Regulation of endothelial cell barrier function by antibody-driven affinity modulation of platelet endothelial cell adhesion molecule-1 (PECAM-1). J Biol Chem 2014;289:20836-44. [Crossref] [PubMed]
- DeLisser H, Liu Y, Desprez PY, et al. Vascular endothelial platelet endothelial cell adhesion molecule 1 (PECAM-1) regulates advanced metastatic progression. Proc Natl Acad Sci U S A 2010;107:18616-21. [Crossref] [PubMed]
(English Language Editor: A. Kassem)