Automated deep neural network-based identification, localization, and tracking of cardiac structures for ultrasound-guided interventional surgery
Highlight box
Key findings
• We developed a deep learning–based model that can identify, localize, and track the key structures and devices in ultrasound-guided cardiac interventions in pathological states.
• This model outperformed most human experts and was comparable to the optimal performance of all human experts in cardiac structure identification and localization.
What is known and what is new?
• The increase in the use of ultrasound-guided interventional therapy for cardiovascular diseases has increased the importance of intraoperative real-time cardiac ultrasound image interpretation, which is a challenging task that is prone to errors and requires expert readers.
• Deep learning–based algorithms may help improve the identification and localization of cardiac structures and surgical instruments in real-time ultrasound images, thereby increasing the efficiency of the workflow.
What is the implication, and what should change now?
• This model was applicable in external data sets, which will help to promote the generalization of ultrasound-guided techniques and improve the quality and efficiency of the clinical workflow of ultrasound diagnosis.
Introduction
With the development of cardiac interventional therapy, the risk of radiation exposure faced by patients and medical workers, as well as the accompanying adverse effects on health, such as cataracts (1,2), arteriosclerosis (3), and tumor formation (4), during traditional radiation-guided interventional therapy has attracted increasing attention (5). In contrast, ultrasound-guided cardiac interventional therapy avoids radiation-associated risks. The reliability of this method was proven in our previous studies of the ultrasound-guided interventional treatment of congenital heart disease (6,7) and valvular heart disease (8,9). It is now possible to perform percutaneous balloon mitral valvuloplasty under pure ultrasound guidance without radiation exposure and contrast agents. These include pregnant patients, patients with chronic renal failure, and patients with contrast allergies. These patients are difficult to treat by conventional means (8). Similarly, a technique for percutaneous closure of unclosed foramen ovale under echocardiographic guidance only has been achieved (6). The success rate was 90.4%, and the median length of stay was 3.0 days, with no severe complications such as peripheral vascular injury or cardiac perforation at the time of discharge. And no complications such as death, stroke, transient ischemic attack, and residual shunt occurred at more than one year of follow-up. And as technology advances and innovates, more diseases will be treated with ultrasound guidance. Cardiac ultrasound plays an important role not only in the diagnosis of cardiovascular diseases but also in guiding interventional surgery. However, real-time and accurate echocardiographic interpretation remains challenging and requires ample experience, expertise, and long-term training. The following challenges render ultrasound interpretation prone to errors: relevant information may be needed at any time during the operation in accordance with the operation conditions, various physiological and pathological changes may appear similar, and a single pathology may exhibit various features (10,11). Compounding this difficulty is the discrepancy between the increased rate of various examinations and surgeries and the number of qualified sonographers (12), which has led to an increased workload for sonographers and hindered the rapid generalization of ultrasound-guided interventional surgery.
Deep learning techniques have led to several promising achievements in medical imaging analysis, such as the use of skin photographs to diagnose skin cancer (13) and the use of echocardiography videos to evaluate ejection fraction (14). However, to our knowledge, no study has reported on the use of artificial intelligence methods to localize and track cardiac structures, especially in the disease state. The localization and tracking of cardiac structures are the basis of artificial intelligence-assisted ultrasound-guided interventional surgery. Therefore, we aimed to develop a deep learning-based model to accurately identify, localize, and track important cardiac structures and lesions to enable streamlining of the clinical workflow and promote the generalization of ultrasound-guided interventional techniques.
The performance of physicians with different levels of experience from the departments of cardiology and ultrasound in the China National Center for Cardiovascular Diseases was compared with that of the model to validate the performance of artificial intelligence interpretation of the ultrasound images. To ensure that the model was applicable to other data, we used 2 data sets of populations from different countries for independent validation. This model will be of great significance for the generalization of single ultrasound-guided cardiac interventional therapy and for improving the level of echocardiographic interpretation in medical units at all levels. We present the following article in accordance with the TRIPOD reporting checklist (available at https://jtd.amegroups.com/article/view/10.21037/jtd-23-470/rc).
Methods
Data sets
The Ethics Committee of Fuwai Hospital, Chinese Academy of Medical Sciences, approved this study (Approval No. 2022-1672) and waived the requirement for patient consent. The study was conducted in accordance with the Declaration of Helsinki (as revised in 2013).
We used the surgical videos of 79 patients who had undergone single ultrasound-guided or ultrasound-assisted interventional cardiac surgeries for structural heart disease at Fuwai hospital. We cut the videos into 3,856 ultrasound views (static ultrasound views) categorized as transthoracic and transesophageal ultrasound views (including parasternal long-axis, parasternal short-axis, subcostal 4-chamber, and apical 4-chamber views) and midesophageal aortic short-axis views (including nonstandard views). The disease categories were atrial septal defect (n=25), ventricular septal defect (n=8), patent ductus arteriosus (n=11), mitral stenosis (n=5), mitral insufficiency (n=6), coarctation of the aorta (n=2), aortic valve stenosis (n=7), pulmonary valve stenosis (n=8), and left auricular occlusion (n=7).
The data sets were labeled by 3 physicians (2 cardiologists 1 one sonographer) with more than 5 years of specialized experience, and confirmation of each label required the agreement of at least 2 of the physicians. Forty views were randomly selected from the data sets to form the test data set, which was not involved in model training and was used for subsequent comparison with the findings of human experts. In total, 17,114 labels were used in the modeling, including 1,929 for interventricular septum (IVS), 2,126 for interatrial septum (IAS), 3,114 for mitral valve (MV), 1,411 for tricuspid valve (TV), 439 for atrial and ventricular septal defects (nidus), 4,108 for sealing installation (SI), 2,214 for the aorta (AO), 1,151 for the aortic valve (AV), and 622 for the pulmonary artery (PA). The sealing installation here refers to the sealer and delivery system visible under ultrasound.
The independent validation data set comprised the EchoNet-Dynamic data set (15) and Cardiac Acquisitions for Multi-structure Ultrasound Segmentation (CAMUS) data set, (14) both of which are publicly available online and were approved by the relevant ethics committees. The EchoNet-Dynamic dataset contains 10,030 videos of apical four-chamber echocardiograms. These individuals underwent imaging examinations at Stanford University Hospital between 2016 and 2018 as part of routine clinical care. The CAMUS dataset was composed of imaging data from clinical tests of 500 patients, and was included in this study after complete anonymization according to the University Hospital of Saint-Etienne (France) ethics committee. This study used 450 of these cases, which is the officially published training set data.
Cardiac structure identification (multiple classifications)
We used the ResNet18 model pretrained using the modified ImageNet as the backbone network (16) for feature extraction and removed the last layer of the 1000-category fully connected layer, containing 1 convolutional layer and 4 residual blocks. After 40 images were extracted as the test set, the remaining images were randomly divided into a training data set and a validation data set in the ratio of 8:2. All images were preprocessed by random cropping (227×227), image flipping, and image normalization, and then input into the network. After training, we applied the model to the test data set of 40 views and evaluated the performance. For ultrasonography, which tends to detect a small proportion of the whole frame, we added a spatial attention module for calculating the spatial attention map and a channel attention module for calculating the channel attention (17). Global maximum pooling, average pooling, and random pooling of space were used to obtain three 1×1×C channel descriptions that were then summed. That is, spatial attention represented the significance of spatial location, while channel attention represented the significance of feature channels; these were then multiplied by the original features separately to obtain 2 attention features. The spatial attention, channel attention, and original features were then fused additively to obtain the optimal features for classification. Figure 1 shows the fusion process. The fusion features were globally pooled on average and then sent to the fully connected layer. Finally, the judgment of the 9 labels was outputted by the softmax activation function. The complete model structure is presented in Figure S1.
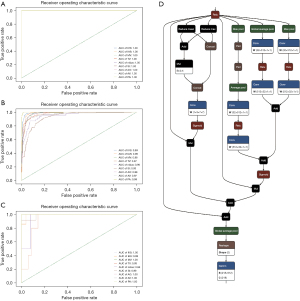
Performance of the model versus specialized physicians
A total of 15 specialized physicians participated in the study, all of whom were specialized cardiologists and sonographers from the China National Cardiovascular Center (Beijing) and its subcenters (Zhengzhou and Kunming). The physicians were divided into 6 groups. There were 3 sonographers with work experience of less than 1 year, 3 with 1–3 years of experience, and 3 with more than 3 years of experience. There were 2 cardiologists with 2 years, 2 with 3 years, and 2 with 1 year of experience, respectively.
The test data set was independently judged by each participant and the neural network model. The interpretation results of the participants and of the neural network model were compared. The evaluation index was accuracy (ACC).
Cardiac structure localization and tracking
To enhance the robustness of the model, the background of the detected objects was enriched and adapted to the task of small target detection, the data were enhanced with mosaic data augmentation (18) (assembled with random scaling, random cropping, and random arrangement) and randomly flipped up and down and left and right, and 300 views were randomly selected to form the validation data set. The Yolov5 model and DeepSORT algorithm were used for target identification and tracking (19) (Figure 2). The area under the precision-recall curve (AP value) was used as the evaluation index, and the average AP value for all categories was mapped (mean average precision) (19). After training, we applied the model to the test data set of 40 views and evaluated the performance.
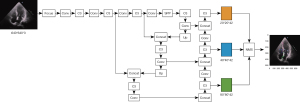
Validation of external data sets
The neural network model was used to dynamically identify 10,030 videos in the EchoNet-Dynamic data set (15) in a frame-by-frame manner, and 5,168,055 labels were detected in total, including 1,970,266 for IVS, 1,101,465 for IAS, 1,711,586 for MV, and 384,738 for TV. From 200 randomly selected videos, 200 frames of images were randomly selected for the human experts to validate and judge the performance of the model. For the CAMUS data set (14), 450 videos were first divided into 8,705 images, and then 10 images were randomly selected in a ratio of 20:1 with the EchoNet-Dynamic data set for human experts to validate and judge the model performance. This eliminated the possible bias caused by the sampling method. In total, 27,938 labels were detected in the CAMUS data set, including 3,740 for IVS, 11,413 for IAS, 10,075 for MV, and 2,710 for TV. The randomly selected views in both the data sets after application of the model were judged individually by 3 physicians with more than 5 years of specialized work experience to determine whether the artificial intelligence annotation results were accurate; each label was confirmed with the agreement of at least 2 physicians. Views were drawn in equal proportions according to the sample size for validation, and the ratio of drawn views was 20:1 for both data sets. In addition, as both data sets had 4-chamber views, there were only 4 validation labels, namely IVS, IAS, MV, and TV. The generalization performance of the model was evaluated using the results for each label in the 2 data sets. The evaluation index was ACC.
Statistical methods
The deep learning neural network model was built using Python software (Python Foundation Software). Comparisons between consecutive values were performed using the t test or Mann-Whitney test. All comparisons were bidirectional, with statistical significance defined as P<0.05. Random sampling of the EchoNet-Dynamic data set was implemented using the random function of the NumPy package in Python, while random sampling of the CAMUS data set was implemented using the RAND function of Excel software (Microsoft Corp.). ACC= (TP + TN)/(TP + FP + FN + TN) where TP (true positive) is the number of samples correctly classified as positive, TN (true negative) is the number of samples correctly classified as negative, FP (false positive) is the number of samples incorrectly classified as positive, FN (false negative) is the number of samples misclassified as negative samples.
Results
Cardiac structure identification (multiple classifications)
The performance of the neural network model in the training data set revealed an area under the receiver operating characteristic (ROC) curve (AUC) of 1 (95% CI: 1–1) for all labels. In the validation set, the AUC of each label was 0.99 (95% CI: 0.98554228–0.99657772) for IVS, 0.99 (95% CI: 0.98073132–0.99390029) for IAS, 0.98 (95% CI: 0.97096391–0.98816888) for MV, 0.97 (95% CI: 0.96262785–0.98319048) for TV, 0.95 (95% CI: 0.92245692–0.97870795) for nidus, 0.93 (95% CI: 0.90918763–0.95377301) for SI, 0.98 (95% CI: 0.97280951–0.99030731) for AO, 0.97 (95% CI: 0.94874406–0.98268206) for AV, and 0.99 (95% CI: 0.99766502–1) for PA. In the test data set, the AUC of each label was 1 (95% CI: 1–1) for IVS, 0.99 (95% CI: 0.98148637–1) for IAS, 1 (95% CI: 1–1) for MV, 0.93 (95% CI: 0.84205691–1) for TV, 0.96 (95% CI: 0.87179627–1) for nidus, 0.99 (95% CI: 0.96973719–1) for SI, 1 (95% CI: 1–1) for AO, 1 (95% CI: 1–1) for AV, and 1 (95% CI: 1–1) for PA. The ROC curves are shown in Figure 1A-1C.
Comparison with the performance of multicenter physicians
In the test data set, the highest ACC of each label of the 15 physicians was 0.769 for AO, 0.923 for AV, 0.974 for IAS, 0.949 for IVS, 0.974 for MV, 0.923 for nidus, 0.923 for PA, 0.923 for SI, and 0.974 for TV. The median ACC of each label of the 15 physicians was 0.744 for AO, 0.795 for AV, 0.846 for IAS, 0.846 for IVS, 0.923 for MV, 0.692 for nidus, 0.872 for PA, 0.872 for SI, and 0.744 for TV. In comparison, the ACC of each label of the artificial intelligence model was 1.000 for AO, 1.000 for AV, 0.925 for IAS, 0.975 for IVS, 1.000 for MV, 0.925 for nidus, 1.000 for PA, 0.950 for SI, and 0.850 for TV. According to the t test, the identification ACC of each label in the artificial intelligence group was comparable to the highest ACC (P=0.204) but significantly higher than the median ACC (P<0.001) in the physician group (Table 1). The ROC curves for each label and the distribution of the interpretation results of the 15 physicians are separately shown in Figure 3.
Table 1
AO (ACC) | AV (ACC) | IAS (ACC) | IVS (ACC) | MV (ACC) | Nidus (ACC) | PA (ACC) | SI (ACC) | TV (ACC) | |
---|---|---|---|---|---|---|---|---|---|
H1_1 | 0.744 | 0.744 | 0.821 | 0.923 | 0.897 | 0.769 | 0.872 | 0.487 | 0.795 |
H1_2 | 0.718 | 0.641 | 0.718 | 0.769 | 0.769 | 0.744 | 0.821 | 0.641 | 0.590 |
H1-3_1 | 0.641 | 0.923 | 0.949 | 0.846 | 0.923 | 0.923 | 0.692 | 0.513 | 0.795 |
H1-3_2 | 0.769 | 0.897 | 0.744 | 0.821 | 0.846 | 0.564 | 0.769 | 0.718 | 0.692 |
H1-3_3 | 0.744 | 0.821 | 0.974 | 0.769 | 0.872 | 0.692 | 0.872 | 0.641 | 0.947 |
H3+_1 | 0.718 | 0.744 | 0.846 | 0.897 | 0.974 | 0.615 | 0.872 | 0.923 | 0.744 |
U1_1 | 0.744 | 0.795 | 0.846 | 0.821 | 0.974 | 0.462 | 0.923 | 0.872 | 0.718 |
U1_2 | 0.718 | 0.769 | 0.846 | 0.949 | 0.974 | 0.846 | 0.923 | 0.821 | 0.692 |
U1_3 | 0.744 | 0.846 | 0.769 | 0.949 | 0.923 | 0.538 | 0.923 | 0.897 | 0.718 |
U1-3_1 | 0.769 | 0.718 | 0.846 | 0.949 | 0.974 | 0.692 | 0.872 | 0.897 | 0.692 |
U1-3_2 | 0.769 | 0.795 | 0.872 | 0.846 | 0.923 | 0.385 | 0.897 | 0.923 | 0.821 |
U1-3_3 | 0.744 | 0.795 | 0.846 | 0.795 | 0.949 | 0.718 | 0.895 | 0.923 | 0.744 |
U3+_1 | 0.769 | 0.744 | 0.872 | 0.923 | 0.872 | 0.513 | 0.897 | 0.769 | 0.795 |
U3+_2 | 0.769 | 0.795 | 0.872 | 0.897 | 0.923 | 0.359 | 0.872 | 0.923 | 0.821 |
U3+_3 | 0.769 | 0.821 | 0.872 | 0.821 | 0.974 | 0.821 | 0.897 | 0.897 | 0.769 |
AI | 1.000 | 1.000 | 0.925 | 0.975 | 1.000 | 0.925 | 1.000 | 0.950 | 0.850 |
“1”, “1-3”, and “3+” represent up to 1 year, 1–3 years, and more than 3 years of experience in the specialty, respectively. H, cardiologist; U, ultrasonographer; AI, artificial intelligence model; AO, aorta; AV, aortic valve; IAS, interatrial septum; IVS: interventricular septum; MV, mitral valve; PA, pulmonary artery; SI, sealing installation; TV, tricuspid valve; ACC, accuracy.
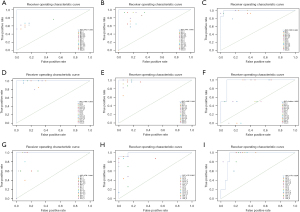
Cardiac structure localization and tracking
The Yolov5 model and DeepSORT algorithm were used for target identification and tracking (19) (Figure 2). To comprehensively evaluate the performance of the model, the AP values were used to compare the ability of the model to localize each structure. In the validation data set, the AP values of each structure were 0.83 for IVS, 0.57 for IAS, 0.69 for MV, 0.52 for TV, 0.09 for nidus, 0.75 for SI, 0.76 for AO, 0.64 for AV, and 0.73 for PA (Figure 4). The performance of the model in the test data set is shown in Figure 5. Figure 5A shows an apical 4-chamber view of an atrial septal defect occluded under single ultrasound guidance, allowing easy tracing of the moving path of the occluder. Figure 5B,5C show the parasternal long-axis views of a ventricular septal defect occluded under single ultrasound guidance, allowing more structures to be seen and for the moving path of the occluder to be traced. Figure 5D shows the apical 4-chamber view of the atrial septal defect, suggesting the presence of the defect site where the echo is interrupted. Figure 5E shows midesophageal aortic short-axis views used for the evaluation of aortic valve stenosis with transesophageal ultrasound. Figure 5F,5G show the application performance in the apical 4-chamber views in the EchoNet-Dynamic and CAMUS data sets.
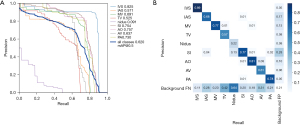
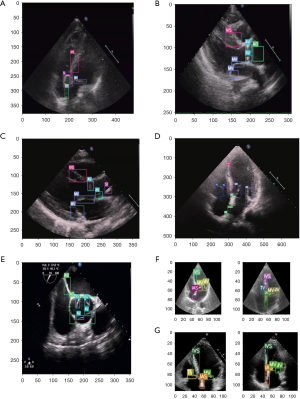
Validation in external data sets
The localization ACC of each label in the 200 views drawn from the EchoNet-Dynamic data set was 77% for IVS, 79% for IAS, 88% for MV, and 89.5% for TV. The localization ACC of each label in the 10 views drawn from the CAMUS data set was 70% for IVS, 70% for IAS, 90% for MV, and 90% for TV. t tests showed no significant difference between the 2 groups in the performance of the model in different external data sets (P=0.63).
Discussion
Because of the health hazards associated with radiation exposure (1-5), there is an urgent need for a surgical option to reduce radiation exposure to both patients and physicians in cardiac interventional procedures. Moreover, the high cost of conventional radiation-guided interventional operating rooms prevents such therapy from being widely applied in most primary hospitals (20). This increases the cost of patient care and the pressure of diagnosis and treatment in regional medical centers and may even lead to missed opportunities for surgical intervention. However, ultrasound-guided interventions can solve these problems. The use of ultrasound-guided interventions avoids intraoperative exposure to large amounts of radiation; furthermore, ultrasound equipment is relatively portable and inexpensive and does not require a special application environment such as a dedicated lead-lined operating room, which makes it easy to implement in a greater number of medical institutions. However, ultrasound-guided interventions place higher demands on the operator‘s interpretation of echocardiography, and the medical team needs to process image information quickly in complex pathological states and real-time changing operating environments, which often requires a long training period. The artificial intelligence-assisted ultrasound image interpretation and subsequent positioning and tracking will improve the ability of surgeons in ultrasound-guided surgery to obtain relevant information on time according to the surgical operation, which will help promote this technology. It will also shorten the learning cycle of surgeons and ultrasonographers for this technology and help alleviate the pressure of insufficient medical resources.
To resolve these issues, we developed a new deep learning-based model integrating spatial attention, channel attention, and original features for cardiac ultrasound images (17), which enables the identification, localization, and tracking of all major cardiac structures, pathological defects, and interventional devices in normal and disease states. Our algorithm consistently demonstrated high performance across independent data sets, even outperforming senior cardiologists and sonographers in hospitals specializing in cardiovascular diseases.
Our study has 5 main strengths. First, to our knowledge, this is the first ultrasound-based artificial intelligence study to identify, localize, and track key structures and devices during cardiac interventions and is thus unlike the classical models that focus mainly on cardiac function (14,15). Our model improves surgical safety by localizing and tracking devices (sealing installation) and key structures to prevent iatrogenic intraoperative injuries and improve the response capacity of the surgical team. This will facilitate the generalization of new techniques, reduce learning costs, and provide a reference for subsequent study and device development.
Second, to our knowledge, this is the first artificial intelligence model trained based on the data of pathological states during cardiac interventions; in contrast, the recently reported ultrasound artificial intelligence models have been trained on the data of normal individuals or physical examination screening (14,15,21-23). Our research is conducive to the implementation of intelligent ultrasound models in clinical practice.
Third, the performance of deep learning–based algorithms has been shown to depend not only on the quantity of the training data set but also on the quality of the data labels (24). The data sets used in our study were jointly labeled by cardiologists and sonographers with more than 5 years of specialized experience working in the China National Cardiovascular Center. All the physicians involved in the data labeling were from the surgical teams that performed single ultrasound-guided cardiac interventions. The abundant experience of the labeling team guarantees the high accuracy of the data labels.
Fourth, the data used in our study were obtained from real-world surgical videos obtained during clinical diagnosis and treatment. The use of real-world data is conducive to improving the robustness of the model and better meeting the practical needs of clinical application.
Fifth, our 2 independent external validation data sets were from different countries (the EchoNet-Dynamic data set is from the United States, while the CAMUS data set is from France), and both are recognized as high-quality data sets. The excellent performance of the model in these data sets ensures the excellent generalization of the model performance, which is beneficial for the generalization to a wider range of data sets and practical situations.
The present study has several limitations. First, the retrospective collection of ultrasound video data resulted in the missing of basic data for some patients, such as height, weight, and ethnicity, which prevented a more detailed presentation of information of the included cases. However, high-quality data annotation ensured the high quality of the data used, and the excellent, nondifferential performance in the validation data set proved that the missing information was not critical for this study.
Second, our study covered only 9 disease categories, and thus the findings may not be applicable to some rare or less clinically relevant abnormalities. However, as ultrasound-guided interventions for more diseases are still in the research stage, we believe that our model can be applied to a substantial proportion of clinically relevant diseases in actual clinical practice. Third, the ultrasound views are freely designed by the operator during the operation according to actual needs (including nonstandard angles and nonstandard cuts). Therefore, although our data sets contained some nonstandard views, the model may not be applicable to images obtained from some rare probing angles. However, the excellent performance of the model in the training and multiple validation data sets demonstrated that it was applicable to the most commonly used views. Fourth, our model will benefit from application in larger training data sets covering a wider range of populations and diseases. Because ultrasound-guided intervention for structural heart disease is a very new technology, we could not collect enough data to construct an independent external validation dataset. However, this model performs equally well on public datasets, which demonstrates the excellent performance of this model. Fifth, as with various AI-assisted medical research and applications, AI can assist people but not replace them, which is ethically impermissible. Ultimately, the quality of medical care still needs to be controlled by human experts. Sixth, blinding was not used in the external validation process; the interpretation of a human expert panel was used as the gold standard. Therefore, this study would benefit from further prospective, blinded, multicenter studies in the future.
Conclusions
We developed a deep learning-based model that can identify, localize, and track the key structures and devices in ultrasound-guided cardiac interventions in pathological states. This model outperformed most human experts and was comparable to the optimal performance of all human experts in cardiac structure identification and localization. This model was applicable in external data sets and will thus help to promote the generalization of ultrasound-guided techniques and improve the quality and efficiency of the clinical workflow of ultrasound diagnosis.
Acknowledgments
This study benefited from the high-quality data of previous studies of which the true generosity has advanced cardiovascular medicine.
Funding: This evaluation study was supported by funding from the Fundamental Research Funds for the Central Universities (No. 2019PT350005), the National Natural Science Foundation of China (No. 81970444), the Beijing Municipal Science and Technology Project (No. Z201100005420030), the National High Level Talents Special Support Plan (No. 2020-RSW02), the CAMS Innovation Fund for Medical Sciences (No. 2021-I2M-1-065), and the Sanming Project of Medicine in Shenzhen (No. SZSM202011013).
Footnote
Reporting Checklist: The authors have completed the TRIPOD reporting checklist. Available at https://jtd.amegroups.com/article/view/10.21037/jtd-23-470/rc
Data Sharing Statement: Available at https://jtd.amegroups.com/article/view/10.21037/jtd-23-470/dss
Peer Review File: Available at https://jtd.amegroups.com/article/view/10.21037/jtd-23-470/prf
Conflicts of Interest: All authors have completed the ICMJE uniform disclosure form (available at https://jtd.amegroups.com/article/view/10.21037/jtd-23-470/coif). The authors have no conflicts of interest to declare.
Ethical Statement: The authors are accountable for all aspects of the work in ensuring that questions related to the accuracy or integrity of any part of the work are appropriately investigated and resolved. The Ethics Committee of Fuwai Hospital, Chinese Academy of Medical Sciences, approved this study (approval no. 2022-1672) and waived the requirement for patient consent. The study was conducted in accordance with the Declaration of Helsinki (as revised in 2013).
Open Access Statement: This is an Open Access article distributed in accordance with the Creative Commons Attribution-NonCommercial-NoDerivs 4.0 International License (CC BY-NC-ND 4.0), which permits the non-commercial replication and distribution of the article with the strict proviso that no changes or edits are made and the original work is properly cited (including links to both the formal publication through the relevant DOI and the license). See: https://creativecommons.org/licenses/by-nc-nd/4.0/.
References
- Elmaraezy A, Ebraheem Morra M, Tarek Mohammed A, et al. Risk of cataract among interventional cardiologists and catheterization lab staff: A systematic review and meta-analysis. Catheter Cardiovasc Interv 2017;90:1-9. [Crossref] [PubMed]
- Ciraj-Bjelac O, Rehani MM, Sim KH, et al. Risk for radiation-induced cataract for staff in interventional cardiology: is there reason for concern? Catheter Cardiovasc Interv 2010;76:826-34. [Crossref] [PubMed]
- Andreassi MG, Piccaluga E, Gargani L, et al. Subclinical carotid atherosclerosis and early vascular aging from long-term low-dose ionizing radiation exposure: a genetic, telomere, and vascular ultrasound study in cardiac catheterization laboratory staff. JACC Cardiovasc Interv 2015;8:616-27. [Crossref] [PubMed]
- Roguin A, Goldstein J, Bar O, et al. Brain and neck tumors among physicians performing interventional procedures. Am J Cardiol 2013;111:1368-72. [Crossref] [PubMed]
- McNamara DA, Chopra R, Decker JM, et al. Comparison of Radiation Exposure Among Interventional Echocardiographers, Interventional Cardiologists, and Sonographers During Percutaneous Structural Heart Interventions. JAMA Netw Open 2022;5:e2220597. [Crossref] [PubMed]
- Yang T, Butera G, Ou-Yang WB, et al. Percutaneous closure of patent foramen ovale under transthoracic echocardiography guidance-midterm results. J Thorac Dis 2019;11:2297-304. [Crossref] [PubMed]
- Ou-Yang WB, Li SJ, Wang SZ, et al. Echocardiographic Guided Closure of Perimembranous Ventricular Septal Defects. Ann Thorac Surg 2015;100:1398-402. [Crossref] [PubMed]
- Liu Y, Guo GL, Wen B, et al. Feasibility and effectiveness of percutaneous balloon mitral valvuloplasty under echocardiographic guidance only. Echocardiography 2018;35:1507-11. [Crossref] [PubMed]
- Wang SZ, Ou-Yang WB, Hu SS, et al. First-in-Human Percutaneous Balloon Pulmonary Valvuloplasty Under Echocardiographic Guidance Only. Congenit Heart Dis 2016;11:716-20. [Crossref] [PubMed]
- Baumgartner H, Falk V, Bax JJ, et al. 2017 ESC/EACTS Guidelines for the Management of Valvular Heart Disease. Rev Esp Cardiol (Engl Ed) 2018;71:110. [Crossref] [PubMed]
- Nishimura RA, Otto CM, Bonow RO, et al. 2017 AHA/ACC Focused Update of the 2014 AHA/ACC Guideline for the Management of Patients With Valvular Heart Disease: A Report of the American College of Cardiology/American Heart Association Task Force on Clinical Practice Guidelines. J Am Coll Cardiol 2017;70:252-89. [Crossref] [PubMed]
- Holmlund S, Lan PT, Edvardsson K, et al. Health professionals' experiences and views on obstetric ultrasound in Vietnam: a regional, cross-sectional study. BMJ Open 2019;9:e031761. [Crossref] [PubMed]
- Esteva A, Kuprel B, Novoa RA, et al. Dermatologist-level classification of skin cancer with deep neural networks. Nature 2017;542:115-8. [Crossref] [PubMed]
- Ouyang D, He B, Ghorbani A, et al. Video-based AI for beat-to-beat assessment of cardiac function. Nature 2020;580:252-6. [Crossref] [PubMed]
- Leclerc S, Smistad E, Pedrosa J, et al. Deep Learning for Segmentation Using an Open Large-Scale Dataset in 2D Echocardiography. IEEE Trans Med Imaging 2019;38:2198-210. [Crossref] [PubMed]
- He K, Zhang X, Ren S, et al. Deep residual learning for image recognition. Proceedings of the IEEE conference on computer vision and pattern recognition 2016; 770-778.
- Woo S, Park J, Lee J Y, et al. Cbam: Convolutional block attention module. Proceedings of the European conference on computer vision (ECCV). 2018; 3-19.
- Bochkovskiy A, Wang C Y, Liao H Y M. Yolov4: Optimal speed and accuracy of object detection. arXiv preprint .2004.10934, 2020.
- Zhu X, Lyu S, Wang X, et al. TPH-YOLOv5: Improved YOLOv5 based on transformer prediction head for object detection on drone-captured scenarios. Proceedings of the IEEE/CVF International Conference on Computer Vision 2021; 2778-2788.
- Deng RD, Zhang FW, Zhao GZ, et al. A novel double-balloon catheter for percutaneous balloon pulmonary valvuloplasty under echocardiographic guidance only. J Cardiol 2020;76:236-43. [Crossref] [PubMed]
- Pandey A, Kagiyama N, Yanamala N, et al. Deep-Learning Models for the Echocardiographic Assessment of Diastolic Dysfunction. JACC Cardiovasc Imaging 2021;14:1887-900. [Crossref] [PubMed]
- Narang A, Bae R, Hong H, et al. Utility of a Deep-Learning Algorithm to Guide Novices to Acquire Echocardiograms for Limited Diagnostic Use. JAMA Cardiol 2021;6:624-32. [Crossref] [PubMed]
- Moal O, Roger E, Lamouroux A, et al. Explicit and automatic ejection fraction assessment on 2D cardiac ultrasound with a deep learning-based approach. Comput Biol Med 2022;146:105637. [Crossref] [PubMed]
- Deng J, Dong W, Socher R, et al. Imagenet: A large-scale hierarchical image database. 2009 IEEE conference on computer vision and pattern recognition 2009;248-255.
(English Language Editor: J. Gray)