A mast cell-related prognostic model for non-small cell lung cancer
Highlight box
Key findings
• We constructed a mast cell-related prognostic model for NSCLC. This risk model may provide a theoretical basis for future investigations concerning NSCLC mechanisms, diagnosis, treatment, and prognosis.
What is known, and what is new?
• There have been reports of early mast cell infiltration in a variety of human and animal tumors, particularly malignant melanoma, breast cancer, and colorectal cancer.
• In the present study, we used a co-expression network to construct a risk-scoring model for predicting prognosis in NSCLC. We further explored the relationships between the risk-scoring model, immune infiltration cells, TMB, and immune checkpoint inhibitors.
What is the implication, and what should change now?
• Our findings may provide theoretical guidance for the clinical application of NSCLC therapy. Further cell and animal experiments and clinical analysis are required to verify the risk regression model we constructed and the function of risk genes.
Introduction
Lung cancer is the most frequent malignancy in China, and non-small cell lung cancer (NSCLC) is its most common pathological type (1). In recent years, although combination therapy (mainly chemotherapy) has improved the survival rate of patients with NSCLC, the overall 5-year prognosis for patients is low, and quality of life and the actual survival rate of patients have not changed significantly due to the additional damage caused by chemoradiotherapy (2-4). Recent advances in tumor immunology and molecular biology research have highlighted the importance of immunotherapy for lung cancer and provided a new direction for treating patients with NSCLC (5,6). However, further studies are needed to clarify these immune cell-mediated effects in NSCLC.
Although mast cells (MCs) have long been recognized as central players in allergy reactions, only recentlythe role of mast cells in tumors is multifaceted, including promoting tumor growth, angiogenesis, and immune escape. become apparent (7-9). First, mast cells can secrete a variety of growth factors and cytokines, such as vascular endothelial growth factor (VEGF), basic fibroblast growth factor (bFGF), etc., which can promote tumor growth and metastasis (10,11). Second, mast cells can also promote tumor angiogenesis. Tumors need to obtain nutrients and oxygen through new blood vessels, and mast cells can secrete factors such as VEGF to stimulate angiogenesis (12-14). Finally, mast cells can also contribute to tumor immune escape. Mast cells can secrete factors such as IL-10 to inhibit the activity of immune cells such as T cells, so that tumors can escape the attack of the immune system (15,16). However, no studies have reported the biological function of MCs in the carcinogenesis of lung cancer. Therefore, mast cells play an important role in the occurrence and development of tumors, and are one of the important targets for research and treatment of tumors.
In the present study, we compared mast cell infiltrates in tumor tissues versus normal tissues to identify differentially infiltrated resting MCs. Then, we used a co-expression network to construct a four-gene risk-scoring model for predicting prognosis in NSCLC. We further explored the relationships between the high- and low-risk groups, immune infiltration cells, tumor mutation burden (TMB), and immune checkpoint inhibitors (ICIs). In conclusion, these results may provide theoretical guidance for the clinical application of NSCLC therapy. We present the following article in accordance with the TRIPOD reporting checklist (available at https://jtd.amegroups.com/article/view/10.21037/jtd-23-362/rc).
Methods
Data acquisition
We acquired the gene expression data from the LUAD and LUSC datasets of The Cancer Genome Atlas (TCGA) (https://portal.gdc.cancer.gov/) and the Gene Expression Omnibus (GEO) database (GSE75037). The clinical and somatic mutation data were retrieved from TCGA database (LUAD and LUSC). The mutation data were analyzed by the R “maftools” package (17). Due to the use of publicly available surveillance data, no ethical approval was required for this study. The study was conducted in accordance with the Declaration of Helsinki (as revised in 2013).
Evaluation of infiltrating immune cells in NSCLC
CIBERSORT (18) was used to calculate tumor-infiltrating immune cells. The 22 types of immune cells in the two risk groups were determined using the Wilcoxon test. A Pearson correlation analysis was conducted to determine the co-expression relationship, with cutoffs of R>0.4 and P<0.001. Gene ontology (GO) was applied to disclose the biological function of resting mast cell-related genes (RMCRGs).
Establishment of RMCG signatures
To explore the prognostic value of RMCRGs, univariate Cox regression and least absolute shrinkage and selection operator (LASSO) regression analyses were performed using the expression levels of the 21 genes in the TCGA dataset. To calculate the risk score of the gene signature, we used the following formula: risk score = (coefficient gene1 × RMCG1) + (coefficient gene2 × RMCG2) +…+ (coefficient gene × expression gene). The NSCLC patients were divided into low- and high-risk groups using the cut-off value of the prognostic risk score from the receiver operating characteristic (ROC) curve. The patient survival rates in the high- and low-risk groups were estimated using the Kaplan-Meier survival curve and the “survival” R package. A nomogram was constructed based on each cohort’s risk score and other clinical parameters.
Gene set enrichment analysis (GSEA)
We analyzed the enrichment terms in the entire TCGA cohort using GSEA software version 4.1.1 to reveal the high- and low-risk group pathways. The GSEA analysis of the high- and low-risk groups was performed using the GSEA “clusterProfiler” package for the gene sets “c2.cp.kegg.symbols.gmt” (19).
Immune checkpoint inhibitor (ICI) analysis in the low- and high-risk groups
The correlations between known ICI targets and our signature were analyzed to explore the possible roles of RMCRGs and the risk signature in ICI efficacy in NSCLC. We used a Pearson correlation analysis to assess the associations between risk scores and ICIs.
Evaluation of the risk model’s significance in clinical treatment
To further assess the significance of the prognostic signature in predicting clinical NSCLC therapy response, the half-maximal inhibitory concentration (IC50) values for chemotherapeutic or targeted drugs in the high- and low-risk populations were evaluated via the R “oncoPredict” package (20).
Statistical analysis
We analyzed the relationship between RMCRGs and NSCLC clinical characteristics using Wilcoxon signed-rank tests. The relationship between risk scores, RMCRGs, immune cells, and ICIs was analyzed using Spearman’s correlation. Kaplan-Meier curves were drawn for the survival analysis.
Results
Mast cell-related genes in NSCLC
We performed a differential gene expression analysis in the GSE75037 dataset to identify genes whose expression differed between NSCLC and normal tissues. We used the “limma” R package to perform the differential gene analysis in two groups and create heatmaps and volcano plots (Figure 1A,1B). Then, the CIBERSORT algorithm was used to calculate the proportion of immune cells infiltrating the tumors, and a significant difference in resting mast cell infiltration was observed (Figure 1C). To further elaborate on the association between resting MCs and NSCLC, we analyzed the correlations of RMCRGs using the TCGA-LUAD and TCGA-LUSC datasets. We found 21 RMCRGs that were significantly associated with resting MCs (Figure 1D,1E). The GO term enrichment and pathway analysis of the 21 RMCRGs was carried out using the “clusterProfiler” R package. The GO analysis showed that the 21 RMCRGs were enriched in regulating angiotensin blood levels and angiotensin maturation (Figure 1F). These pathways control the flow of nutrients to the tumor and are crucial for tumor cell migration, growth, metastasis, and survival.
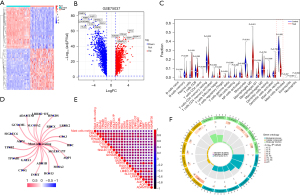
A prognostic model of NSCLC constructed with four RMCRGs
The role of MCs in tumorigenesis and development has received increasing attention, but the study of MCs in NSCLC only just beginning. A prognostic risk model was constructed based on RMCRGs to predict the prognosis of patients with NSCLC. An initial univariate Cox regression analysis was performed on the 21 RMCRGs, and four of these were identified as significantly related to prognosis in NSCLC (available online: https://cdn.amegroups.cn/static/public/jtd-23-362-1.xlsx). Then, LASSO regression was carried out to construct a prognostic model. Per the median risk score, the LASSO Cox regression analysis generated a four-gene risk signature that classified NSCLC patients into high- or low-risk groups. Overall survival was significantly better in the low- vs. high-risk group in early stage NSCLC (Figure 2A). An independent prognostic analysis showed that the risk scores could be used as independent prognostic factors for NSCLC (Figure 2B). The “rms” R package was used to construct a nomogram to identify risk based on the independent factors for clinical prognosis (Figure 2C). We also found that high-risk patients over 65 years and with stage III–IV disease were strongly associated with a poorer prognosis, but there was no association with gender (Figure 2D-2F). We further analyzed GSEA pathway enrichment in the high- and low-risk patient groups. We found that the cell cycle, DNA replication, Parkinson’s disease, ribosome, and spliceosome pathways were significantly enriched in the low-risk group. In contrast, the allograft rejection, asthma, cell adhesion molecules cams, and intestinal immune network for IGA production signaling pathways were significantly enriched in the high-risk group (Figure 3). GSEA results suggest that there are significant individual differences in enriched signaling pathways in patients with different risks. These results suggested that our prognostic risk model based on RMCRGs could predict the prognosis of patients with NSCLC.
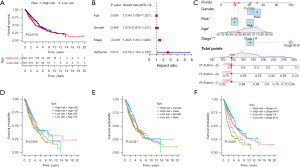
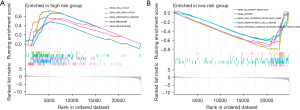
Infiltrating abundance of distinct immunocytes in the low- and high-risk NSCLC groups
We explored the immune infiltration levels of the diverse immune infiltrating cells between the high- and low-risk groups using CIBERSORT. The immune infiltration analysis showed that 14 immune cell infiltration levels were significantly different between the high and low-risk groups, with the low-risk group having the highest level of resting MCs (Figure 4A). These results indicate that the MCs were inhibited in the low-risk group. We also revealed a positive correlation between the expression of four RMCRGs and resting mast cell infiltration in NSCLC: the higher the risk score, the less resting mast cell infiltration (Figure 4B). This result was confirmed on the TISIDB web portal for tumor and immune system interactions (Figure 4C) (http://cis.hku.hk/TISIDB/index.php). The potential relationship between risk score and the efficacy of immunotherapy was evaluated by analyzing the expression of immune checkpoint molecules, an effective marker of immunotherapy, in different risk scores. We found that the risk score was negatively related to the expression of the immune checkpoint genes (ICGs) (Figure 4D). These results strongly suggest that patients in the low-risk group may benefit from immunotherapy.
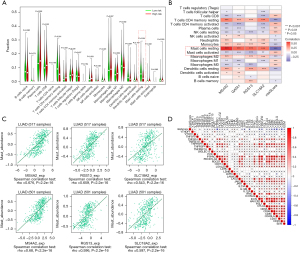
Association between risk scores and TMB
TMB, an emerging immunotherapy marker, is defined as the total number of somatic mutations in a specific region of the genome of tumor cells, which is precisely defined to vary with the size of the sequenced region and the mutability. In theory, the more somatic mutations in a tumor cell, the higher the TMB value and the greater the likelihood of neoantigens being formed, only some of which can be recognized by T cells and cause an antitumor immune response. To screen out those who truly benefit from immunotherapy and to distinguish reliable TMB values, clinicians need to develop a better understanding of the factors that contribute to the heterogeneity of TMB. We attempted to analyze the relationship between the risk score and TMB. Our TMB analysis showed that the risk scores between the wild types and the mutation types of TP53, TTN, MUC16, and CSMD were significantly different (Figure 5A,5B). Furthermore, the TMB in the high-risk group was higher than in the low-risk group (Figure 5C). These results suggest that the reliability of TMB as a biomarker of immunotherapy efficacy is critical.
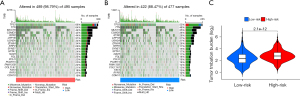
Drug sensitivity of NSCLC patients with high versus low risk
We further investigated the drug sensitivity between the different risk groups using the “oncoPredict” R package. The drug sensitivity analysis showed a significant difference in drug sensitivity between the high and low-risk groups. Specifically, a high-risk score was associated with lower IC50 values for drugs such as Acetalax (Figure 6A), Afatinib (Figure 6B), Alisertib (Figure 6C), AZD3759 (Figure 6D), AZD5991 (Figure 6E), and ERK_6604 (Figure 6F), whereas a low-risk score was associated with lower IC50 values for drugs such as AZD6482 (Figure 6G), AZD8055 (Figure 6H), BMS-754807 (Figure 6I), Doramapimod (Figure 6J), Ribociclib (Figure 6K), and SB216763 (Figure 6L). So, accurate prediction can potentially help to provide better treatment for patients.
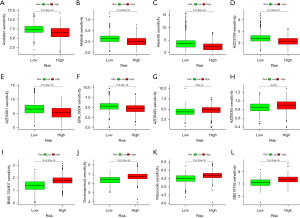
Discussion
Lung cancer has one of the highest fatality rates among malignant tumors in China (21,22). Compared with small cell lung cancer, NSCLC has a slow onset and growth rate but is generally found to be in an advanced stage at initial diagnosis and, therefore, very difficult to control and treat (23-25). A non-invasive, sensitive, and specific biomarker for NSCLC early detection is needed.
MCs are granulated-filled immune cells located primarily under the mucosal epithelium where the body is in contact with the external environment (26). MCs are known for their biological role in allergic reactions but are also now known to be involved in many other important physiological and pathological processes (27,28). MC infiltration has also been found in some human and animal tumor models; however, its function in the tumor microenvironment remains controversial (26,29). Further studies of the role of MCs in NSCLC are warranted.
In the present study, we found that resting MC infiltration in NSCLC is significantly inhibited. Li et al. also found that the ratio of eosinophils, resting MCs, and memory-activated CD4 T cells in the low-risk NSCLC group was higher than in the high-risk group (30). Several studies have shown that CD4 memory T cells can stimulate the activation of mast cells and release cytokines, thereby regulating immune and inflammatory responses (31,32). In addition, resting mast cells can also affect the activation and expansion of CD4 memory T cells, thereby affecting the strength and direction of the immune response (31,33). These findings provide an important reference for further understanding the regulatory mechanism of the immune system and the occurrence and development of related diseases. However, the specific role of resting MCs in early diagnosis and prognosis in NSCLC has not yet been elucidated.
First, we conducted a correlation analysis of 22 immune cells associated with genes using a Spearman rank correlation analysis. A total of 21 RMCRGs were identified, of which four were found to be prognosis-related RMCRGs. By using univariate Cox and LASSO regression analyses, we constructed an RMCG-associated risk model. Significantly, this study showed that the high-risk group had significantly lower resting mast cell infiltration and ICG expression compared with the low-risk group. In our study, we identified subgroups of patients with a significantly higher risk of having deleterious mutations. These results strongly suggest that patients in the high-risk group may not benefit from immunotherapy. We next evaluated the differences in drug susceptibility between the high-and low-risk groups. The results provide a basis for risk stratification and individualized treatment.
These results have clinical implications, as patients in high-risk groups may not benefit from immunotherapy. Therefore, this study provides a basis for risk stratification and individualized treatment. In addition, the study assessed differences in drug sensitivity between high-risk and low-risk groups, which could help in the development of more effective individualized treatment options.
However, the study also had some limitations. First, this is a database analysis that requires further in vitro experimental studies to validate. Second, the sample size used in this study was small, and further expansion of the study scale is required. In addition, the data used in this study are horizontal research data, and further longitudinal research is needed to verify the stability and reliability of its results.
Taken together, the study’s findings contribute to understanding the relationship between immune cells and risk stratification, and response to immunotherapy. This has important clinical implications for the development of more effective individualized treatment options, but further verification and exploration are needed.
Conclusions
In the present study, LASSO analysis was performed to construct a prediction model for estimating NSCLC prognosis. Immune cell infiltration, TMB, ICG expression, and drug sensitivity in different risk groups were analyzed to investigate the impact of risk scores on the tumor microenvironment. In summary, the present results provided novel insights into NSCLC immunotherapy and identified potential intervention targets for treating NSCLC patients.
Acknowledgments
The authors gratefully acknowledge each editor and reviewer for their profound insight into this study.
Funding: The study was supported by Sichuan Academy of Medical Sciences ‧ Sichuan Provincial People’s Hospital level project (No. 2020LY09).
Footnote
Reporting Checklist: The authors have completed the TRIPOD reporting checklist. Available at https://jtd.amegroups.com/article/view/10.21037/jtd-23-362/rc
Peer Review File: Available at https://jtd.amegroups.com/article/view/10.21037/jtd-23-362/prf
Conflicts of Interest: All authors have completed the ICMJE uniform disclosure form (available at https://jtd.amegroups.com/article/view/10.21037/jtd-23-362/coif). The authors have no conflicts of interest to declare.
Ethical Statement: The authors are accountable for all aspects of the work in ensuring that questions related to the accuracy or integrity of any part of the work are appropriately investigated and resolved. Due to the use of publicly available surveillance data, no ethical approval was required for this study. The study was conducted in accordance with the Declaration of Helsinki (as revised in 2013).
Open Access Statement: This is an Open Access article distributed in accordance with the Creative Commons Attribution-NonCommercial-NoDerivs 4.0 International License (CC BY-NC-ND 4.0), which permits the non-commercial replication and distribution of the article with the strict proviso that no changes or edits are made and the original work is properly cited (including links to both the formal publication through the relevant DOI and the license). See: https://creativecommons.org/licenses/by-nc-nd/4.0/.
References
- Schneider BJ, Ismaila N, Aerts J, et al. Lung Cancer Surveillance After Definitive Curative-Intent Therapy: ASCO Guideline. J Clin Oncol 2020;38:753-66. [Crossref] [PubMed]
- Lau SCM, Pan Y, Velcheti V, et al. Squamous cell lung cancer: Current landscape and future therapeutic options. Cancer Cell 2022;40:1279-93. [Crossref] [PubMed]
- Ryssel H, Egebjerg K, Nielsen SD, et al. Innate immune function during antineoplastic treatment is associated with 12-months survival in non-small cell lung cancer. Front Immunol 2022;13:1024224. [Crossref] [PubMed]
- Huang J, Li JX, Ma LR, et al. Traditional Herbal Medicine: A Potential Therapeutic Approach for Adjuvant Treatment of Non-small Cell Lung Cancer in the Future. Integr Cancer Ther 2022;21:15347354221144312. [Crossref] [PubMed]
- Passaro A, Brahmer J, Antonia S, et al. Managing Resistance to Immune Checkpoint Inhibitors in Lung Cancer: Treatment and Novel Strategies. J Clin Oncol 2022;40:598-610. [Crossref] [PubMed]
- Huang C, Yang X. Advances in Biomarkers for Immunotherapy of Non-small Cell Lung Cancer. Zhongguo Fei Ai Za Zhi 2021;24:777-83. [PubMed]
- Su Y, Zhang T, Lu J, et al. Identification and Validation of the Prognostic Panel in Clear Cell Renal Cell Carcinoma Based on Resting Mast Cells for Prediction of Distant Metastasis and Immunotherapy Response. Cells 2023;12:180. [Crossref] [PubMed]
- Grimes JA, Rajeev M, Wallace ML, et al. Scar revision for incompletely or narrowly excised cutaneous mast cell tumors in dogs. Can Vet J 2023;64:63-9. [PubMed]
- Zadvornyi T, Lukianova N, Borikun T, et al. Mast cells as a tumor microenvironment factor associated with the aggressiveness of prostate cancer. Neoplasma 2022;69:1490-8. [Crossref] [PubMed]
- Komi DEA, Redegeld FA. Role of Mast Cells in Shaping the Tumor Microenvironment. Clin Rev Allergy Immunol 2020;58:313-25. [Crossref] [PubMed]
- Nguyen SMT, Rupprecht CP, Haque A, et al. Mechanisms Governing Anaphylaxis: Inflammatory Cells, Mediators, Endothelial Gap Junctions and Beyond. Int J Mol Sci 2021;22:7785. [Crossref] [PubMed]
- De Palma M, Biziato D, Petrova TV. Microenvironmental regulation of tumour angiogenesis. Nat Rev Cancer 2017;17:457-74. [Crossref] [PubMed]
- Marone G, Varricchi G, Loffredo S, et al. Mast cells and basophils in inflammatory and tumor angiogenesis and lymphangiogenesis. Eur J Pharmacol 2016;778:146-51. [Crossref] [PubMed]
- Ribatti D, Tamma R, Crivellato E. Cross talk between natural killer cells and mast cells in tumor angiogenesis. Inflamm Res 2019;68:19-23. [Crossref] [PubMed]
- Moore KW, de Waal Malefyt R, Coffman RL, et al. Interleukin-10 and the interleukin-10 receptor. Annu Rev Immunol 2001;19:683-765. [Crossref] [PubMed]
- Nagata K, Nishiyama C. IL-10 in Mast Cell-Mediated Immune Responses: Anti-Inflammatory and Proinflammatory Roles. Int J Mol Sci 2021;22:4972. [Crossref] [PubMed]
- Mayakonda A, Lin DC, Assenov Y, et al. Maftools: efficient and comprehensive analysis of somatic variants in cancer. Genome Res 2018;28:1747-56. [Crossref] [PubMed]
- Chen B, Khodadoust MS, Liu CL, et al. Profiling Tumor Infiltrating Immune Cells with CIBERSORT. Methods Mol Biol 2018;1711:243-59. [Crossref] [PubMed]
- Yu G, Wang LG, Han Y, et al. clusterProfiler: an R package for comparing biological themes among gene clusters. OMICS 2012;16:284-7. [Crossref] [PubMed]
- Maeser D, Gruener RF, Huang RS. oncoPredict: an R package for predicting in vivo or cancer patient drug response and biomarkers from cell line screening data. Brief Bioinform 2021;22:bbab260. [Crossref] [PubMed]
- Zhou B, Zang R, Song P, et al. Association between radiotherapy and risk of second primary malignancies in patients with resectable lung cancer: a population-based study. J Transl Med 2023;21:10. [Crossref] [PubMed]
- Nie H, Han Z, Nicholas S, et al. Costs of traditional Chinese medicine treatment for inpatients with lung cancer in China: a national study. BMC Complement Med Ther 2023;23:5. [Crossref] [PubMed]
- Capriotti G, Piccardo A, Giovannelli E, et al. Targeting Copper in Cancer Imaging and Therapy: A New Theragnostic Agent. J Clin Med 2022;12:223. [Crossref] [PubMed]
- Chaft JE, Shyr Y, Sepesi B, et al. Preoperative and Postoperative Systemic Therapy for Operable Non-Small-Cell Lung Cancer. J Clin Oncol 2022;40:546-55. [Crossref] [PubMed]
- Donington J, Schumacher L, Yanagawa J. Surgical Issues for Operable Early-Stage Non-Small-Cell Lung Cancer. J Clin Oncol 2022;40:530-8. [Crossref] [PubMed]
- Shi S, Ye L, Yu X, et al. Focus on mast cells in the tumor microenvironment: Current knowledge and future directions. Biochim Biophys Acta Rev Cancer 2023;1878:188845. [Crossref] [PubMed]
- Schanin J, Korver W, Brock EC, et al. Discovery of an agonistic Siglec-6 antibody that inhibits and reduces human mast cells. Commun Biol 2022;5:1226. [Crossref] [PubMed]
- Zoabi Y, Levi-Schaffer F, Eliashar R. Allergic Rhinitis: Pathophysiology and Treatment Focusing on Mast Cells. Biomedicines 2022;10:2486. [Crossref] [PubMed]
- Peng J, Federman HG, Hernandez CM, et al. Communication is key: Innate immune cells regulate host protection to helminths. Front Immunol 2022;13:995432. [Crossref] [PubMed]
- Li J, Li X, Zhang C, et al. A signature of tumor immune microenvironment genes associated with the prognosis of non-small cell lung cancer. Oncol Rep 2020;43:795-806. [Crossref] [PubMed]
- Qiu P, Guo Q, Yao Q, et al. Characterization of Exosome-Related Gene Risk Model to Evaluate the Tumor Immune Microenvironment and Predict Prognosis in Triple-Negative Breast Cancer. Front Immunol 2021;12:736030. [Crossref] [PubMed]
- Xiong T, Lv XS, Wu GJ, et al. Single-Cell Sequencing Analysis and Multiple Machine Learning Methods Identified G0S2 and HPSE as Novel Biomarkers for Abdominal Aortic Aneurysm. Front Immunol 2022;13:907309. [Crossref] [PubMed]
- Li X, Li J, Wu P, et al. Smoker and non-smoker lung adenocarcinoma is characterized by distinct tumor immune microenvironments. Oncoimmunology 2018;7:e1494677. [Crossref] [PubMed]
(English Language Editor: D. Fitzgerald)