Immune characteristics and genetic markers of esophageal cancer by single-cell analysis: implications for immunotherapy
Highlight box
Key findings
• Cell groups with homogenous cell surface markers exhibit intercellular variations that exert a considerable effect on cell function in esophageal cancer.
What is known and what is new?
• Esophageal cancer is one of the most common cancers worldwide. The prognoses of patients with the same stage of esophageal cancer can vary substantially. The progress of single-cell analysis technology has furthered the understanding of tumor heterogeneity.
• Specific cell subsets in the esophageal cancer and paracancerous samples were identified. Differences were detected between B cells and monocytes in stage II and III tumors, which may be related to RNA transcription and degradation. The CXCL8 protein was identified as a valid potential prognostic marker.
What is the implication, and what should change now?
• Our findings will contribute to the understanding of the tumor immune microenvironment and cellular heterogeneity in esophageal cancer and the identification of potential therapeutic targets in the future.
Introduction
Esophageal cancer (EC) is the 7th most common malignancy worldwide, with more than 500,000 new cases diagnosed annually (1,2). It is also the 6th leading cause of cancer death, with more than 500,000 cancer deaths globally each year, and the 5-year overall survival (OS) rate for EC patients is less than 20% (1,2). In recent years, the complexity of the processes involved in cancer formation has been recognized. Even patients with the same tumor pathological type and stage may have different prognoses; thus, it is necessary to decipher the complexity of the tumor and the tumor microenvironment (TME). In recent years, immunotherapy awareness has gradually increased. Immunotherapy has been proven advantageous in advanced or metastatic EC in both the first-line and second-line therapeutic settings (3-5) and has been established as standard care (6).
The TME consists of malignant cells, immune cells, stromal cells, blood cells, endothelial cells, and fat cells (7). Immune and stromal cells are essential components of the TME and have proven valuable for the survival of cancer patients (8). For example, checkpoint-blocking immunotherapy can benefit a limited subset of cancer patients for whom cancer interacts with immunity (9,10). The failure of several anti-angiogenic cancer therapies is thought to be due to the metabolic adaptation and reprogramming of cancer cells as well as endothelial cell abnormalities and their interaction with pericyte cells (11). At the same time, it has been reported that some immune-related parameters can be used to predict the prognosis of esophageal squamous cell carcinoma (ESCC) patients (12-15), which also suggests that these immune parameters have a profound impact on the treatment outcome of ESCC patients. Single-cell transcriptomics has developed rapidly since 2009 when the first single-cell transcriptome profile was described. Cell populations with homogenous cell surface markers harbor cell-to-cell variations, which have a considerable impact on cell function. This heterogeneity can be resolved using single-cell RNA sequencing (scRNA-seq) (16,17). The application of single-cell RNA sequencing brings important insights into the biology of tumor-infiltrating immune cells, including their heterogeneity, kinetics, and potential role in disease progression and response to immune checkpoint inhibitors and other immunotherapies, It remains an elusive goal to produce a complete and unbiased library of RNA transcripts from single cells by most or all methods so as to produce reduced-complexity libraries and missing transcripts that theoretically should have been present (18). EC transcriptomes have been based mostly on traditional RNA sequencing techniques in previous studies. The traditional RNA sequencing technology has led to more in-depth study of the transcription group characteristics of EC and a deeper understanding of the origin and development of EC. RNA-seq provides an effective high-throughput technique for reliably characterizing tumor immune microenvironments. Based on this technique, the key components of immunoinfiltration, expression phenotype and immune pool in anti-tumor immune response can be revealed (19). However, studies based on traditional RNA sequencing have focused on the overall level of the sample, and more research is needed to understand the heterogeneity of a cluster of cells and individual cells. Single-cell sequencing can provide clues about disease development at the individual cellular level. Researchers have applied scRNA-seq to dissect the complex immune responses of peripheral blood mononuclear cells in acute Kawasaki disease (20). In addition, single-cell sequencing analysis has also been applied in a variety of cancers, such as multiple myeloma, lung adenocarcinoma, and stomach adenocarcinoma.
In this study, we downloaded public data from the Gene Expression Omnibus (GEO) and The Cancer Genome Atlas (TCGA) databases to perform scRNA-seq on large-scale cells derived from EC tumor samples. We discovered some interesting facts that may help deepen our understanding of the immune microenvironment and immunotherapy in EC and provide some new inspiration for EC patients. We present the following article in accordance with the STREGA reporting checklist (available at https://jtd.amegroups.com/article/view/10.21037/jtd-23-317/rc).
Methods
Data download and preprocessing
We used TCGA Genomic Data Commons (GDC) Application Programming Interface (API) to download the latest gene expression data and clinical follow-up information for EC on the 2nd of February, 2022. The GSE145370 dataset was downloaded from the GEO database as GSE145370_RAW.tar. GSE145370 was derived from 28 samples, of which 14 were ESCC tumors and the other half were ESCC adjacent normal tissues. In processing TCGA data, only normal and primary tumor samples were retained. For GSE145370 data processing, we used R (version 3.6.4, https://www.r-project.org/) and the Seurat R software package (version 3.6, https://satijalab.org/seurat/) to combine the single-cell sequencing results for each sample from the GSE145370_RAW dataset to build a single-cell dataset. The study was conducted in accordance with the Declaration of Helsinki (as revised in 2013).
Quality control (QC) of single-cell data
As above, we used R and the Seurat R package for the analyses. The filter criteria of cells were determined after referencing previous studies (21,22). According to the median number of genes and the percentage of mitochondrial genes in the samples, cells with <200 and >4,000 genes (potential cell duplets) and a mitochondrial gene percentage of <20% were filtered. After QC, 103,223 high-quality cells were obtained. The relationship between the percentage of mitochondrial genes and the messenger RNA (mRNA) reads was detected and visualized as the relationship between the number of mRNAs and the mRNA reads. Following data normalization, all highly variable genes in single cells were identified after controlling for the relationship between average expression and dispersion. Subsequently, we performed principal component analysis (PCA) with variable genes as the input and identified significant principal components (PCs) based on the JackStraw function. A total of 20 PCs were selected as the input for uniform manifold approximation and projection (UMAP) and t-distributed stochastic neighbor embedding (t-SNE) when statistically significant. Using a resolution of 0.5, the cells were clustered by the FindClusters function and classified into 24 different cell types (clusters 0–23). Next, we used the FindAllMarkers function to find differentially expressed genes (DEGs) between each cell type.
Cell cycle bias analysis
Cell cycle analysis was performed using the Seurat program (CellCycleScoring function). We used a previously defined core set of 43 G1/S and 54 G2/M cell cycle genes. Cells were classified according to the maximal average expression (‘cycle score’) in these two gene sets. When the cycle scores of G1/S and G2/M were both less than 2, we considered these cells to be non-cycling; otherwise, we considered the cells to be proliferative. After the cell cycle analysis, no bias induced by the cell cycle genes was observed.
Notes for cell subgroups
Cell type assignment was performed based on the marker genes reported in a previous study (23), the CellMaker database (http://xteam.xbio.top/CellMarker/), and the SingleR package. Subclustering analysis of B cells, monocytes, effector cluster of differentiation (CD)8+ memory T (Tem) cells, CD8+ T cells, CD4+ T cells, and naive CD8+ T cells was performed using the SubsetData function in the Seurat program. Following data normalization and scaling, all highly variable genes in single cells were identified. Subsequently, 20 PCs were selected as the input for t-SNE when statistically significant.
The FindClusters function was used to classify cells into different clusters. Next, the FindAllMarkers function was used to find DEGs between each subtype. Then, to assign pathway activity estimates to individual cells, the R package gene set variation analysis (GSVA) with the standard settings was used for single-sample gene set enrichment analysis (ssGSEA), and a gene set containing all human pathways was downloaded from the Molecular Signatures Database (MsigDB), which included the Kyoto Encyclopedia of Genes and Genomes (KEGG) pathways. Significantly enriched pathways were identified according to the Benjamini-Hochberg-corrected P value <0.05.
Feature-rich analysis
The KEGG pathway enrichment analysis systems were applied to the clusterProfiler R package (24), to identify the over-represented KEGG pathway genes. In addition, we used the Signal Pathway Impact Analysis (SPIA) package (https://www.bioconductor.org/packages/release/bioc/html/SPIA.html) to activate and inhibit the KEGG pathway based on the different genes and performed GSEA to analyze the KEGG pathway enrichment of cells and samples from different sources using the gene expression spectrum. For both analyses, a q value of <0.05 denoted statistical significance.
Statistical analysis
Statistical analysis for comparison of group means was performed using Welch’s analysis of variance (ANOVA) and pairwise t-test. The association of the single-marker and multi-marker classified immune cells with clinical outcome was evaluated using both univariate and multivariate analyses with adjustment for clinico-pathological confounders in the multivariate Cox proportional hazards models. The Kaplan-Meier method was used to plot survival curves with the log-rank test used for comparisons. No adjustments were made for multiple comparisons. Hypothesis testing was performed at the 5% significance level.
Results
Single-cell data set QC
We downloaded the GSE145370 GEO dataset and analyzed it using the Seurat R package. The number of minimal information units (MIUs) was not significantly correlated with the mitochondrial gene percentage (Figure 1A) but positively correlated with the number of mRNAs (Figure 1B). We also analyzed the number of mRNAs, the mRNA readings, and the distribution of mitochondrial genes (Figure 1C), which revealed that most genes were distributed between 0 and 2,500 bp, mRNA readings were distributed between 0 and 1,000 bp, and mitochondrial percentages were below 15%. Further, we removed cells with more than 20% expression originating from mitochondrial genes and those with fewer than 200 genes. The expression spectrum of 33,538 genes from 103,223 cells was obtained, and the number of cells in each sample was counted (Table 1), including the number of filtered mRNAs, the mRNA readings, and the distribution of mitochondrial genes (Figure 1D). After QC, highly variable genes (top 2,000) were identified for downstream analysis (Figure 1E).
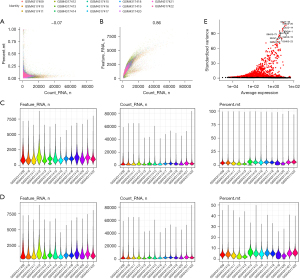
Table 1
Tag | Before cell count | After cell count | Percent (%) |
---|---|---|---|
GSM4317409 | 12,342 | 11,179 | 91 |
GSM4317410 | 8,338 | 7,025 | 84 |
GSM4317411 | 11,018 | 8,759 | 79 |
GSM4317412 | 10,727 | 9,955 | 93 |
GSM4317413 | 15,820 | 14,400 | 91 |
GSM4317414 | 4,986 | 3,944 | 79 |
GSM4317415 | 7,325 | 6,718 | 92 |
GSM4317416 | 6,134 | 5,241 | 85 |
GSM4317417 | 5,057 | 4,797 | 95 |
GSM4317418 | 10,680 | 10,200 | 96 |
GSM4317419 | 4,375 | 4,001 | 91 |
GSM4317420 | 3,502 | 3,039 | 87 |
GSM4317421 | 6,597 | 6,151 | 93 |
GSM4317422 | 8,256 | 7,814 | 95 |
Identification of cell subgroups by t-SNE analysis
Considering that cells of the same type may be classified into different clusters when clustering because of different cell cycles, we conducted cell cycle regression analysis to observe such effects. We found that in each region, three cycles of cells were randomly distributed, and there was no difference in cell cycle status, so there was no need to eliminate the effects of the cell cycles (Figure 2A). In addition, we conducted main component analysis to extract the cell characteristics and distance matrix to identify 24 cell groups (Figure 2B). There were different lymph node (LN) ratios in these cell groups, of which clusters 0, 1, 6, and 4 were mainly from tumor tissue, and clusters 5, 8, 2, and 3 were mainly from tissue peripheral to cancer (Figure 2C). Moreover, by applying rank and testing methods to identify differences in genes using the cell type annotation based on the CellMarker database (diff_genes_wilcox_fdr.txt per cell group), these 24 clusters were eventually annotated to 16 cells (all.marker.anno .txt), which we defined as: Cell Cluster 0, cell monocytes from tumor tissue; Cell Cluster 1, Paneth cells; Cell Cluster 2, B cells; Cell Cluster 3, monocytes 1; Cell Cluster 4, exhausted CD8+ T cells; Cell Cluster 5, CD8+ effector memory T (Tem) cells; Cell Cluster 6, natural killer (NK) cells; Cell Cluster 8, monocytes 2. In addition, we observed different proportions of tumor samples in different monocyte populations, with B cells derived mainly from normal samples and Paneth cells mainly from tumor samples (Figure 2D), and significant individual differences were observed in these cells in different patients.
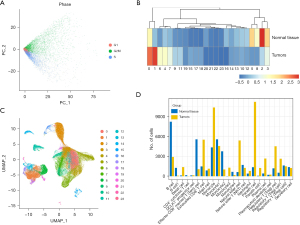
Analysis of the specific cell subgroups and functions in cancer and cancer side samples
We observed the concentration of Paneth cells, NK cells, and exhausted CD8+ T cells in the tumor samples, and the concentration of CD8+ Tem cells in the cancer side samples, which suggested the presence of specific cell subgroups in the cancer and cancer-side samples. We analyzed the differences in gene expression in each cell subgroup of cancer and the cancer side samples and also observed the differences in gene expression. Among the panel cell, NK cell, and exhausted CD8+ T cell, the NK cell has the most differential genes, and most of the differential genes in the exhausted CD8+ T cell are contained by the NK cell, as shown in Figure 3A. We then selected the genes common to the three types of cells to include in the clusterProfiler R software package for KEGG Functional enrichment analysis, which used a total of 29 pathways (step3_A_KEGG_enrich.txt), with the top 10 most significant shown in Figure 3B.
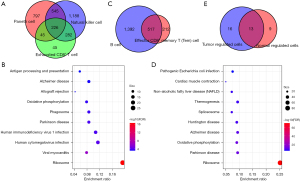
In addition to our analysis of the genetic differences between the CD8+ Tem cell, B cell cancer, and cancer side sample tissues, we observed two types of genetic differences with a large intersection (Figure 3C). Similarly, we identified common differences in genes using the clusterProfiler R package for the KEGG functional enrichment analysis, which used a total of 22 pathways (step3_B_KEGG_enrich.txt), with the top 10 shown in Figure 3D. We also observed a significant overlap between the different gene functions in cells in the cancer and cancer side sample tissues (Figure 3E). For example, genes with differences in the specific cancer cell subgroups and cancer side samples were highly concentrated in the Ribosome and Parkinson’s disease pathways, which showed that there were dysfunction similarities in the specific cell subgroups of cancer and the cancer side samples.
Cell subgroups and disorder pathways for different clinically phased patients
To identify the prognostically-related cell subgroups, we compared the differences among the cell types between stage II and stage III patients. We observed significant differences in cell sources in all cell groups (step4.stage.count.txt), suggesting significant differences in tumor microenvironmental characteristics between samples with different clinical stages, the most significant of which were among the B cell and monocyte groups. The B cell and monocyte counts were significantly higher in stage II than in stage III, which suggested unique immune cell immersion in the different stages. We also compared the differences in gene expression of the B cell and monocyte between the cancer and cancer side samples and observed that most of the degenerated genes in the monocyte intersected with 324 genes in the B cell (Figure 4A).
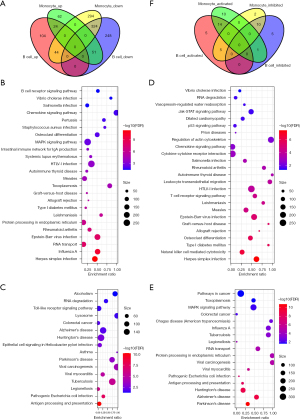
Further, using the SNP Panel Identification Assay (SPIA) R software package, we analyzed the differences in the gene participation pathways between the two types of cells. In B cells, we observed a total of 40 pathways with disorders (e.g., step4_spia.A.txt), of which 24 were activation pathways (Figure 4B) and 16 were inhibition pathways (Figure 4C). In monocytes, we observed a total of 42 offset pathways (e.g., step4_spia.B.txt), of which 25 were pathway activations (Figure 4D) and 17 were inhibition pathways (Figure 4E). Among them, 14 of the activated pathways were consistent, and 10 of the suppressed pathways were consistent, which indicates that the signaling pathways involved exhibit some overall consistency. However, there were six pathways to the contrary, five of which were activated in tumor samples in B cells but inhibited in monocytes (Figure 4F), including protein processing in the endoplasmic reticulum, RNA transport, influenza A, the mitogen-activated protein kinase (MAPK) signaling pathway, toxoplasmosis, and RNA degradation, which was mainly related to RNA transcription and degradation.
Transcription of classical mononucleosis
We also observed the presence of five different mononucleocytes in the monocyte1 marker-based cell subgroups due to CD302, SLC43A2, BACH1, QKI, monocyte1-based RIN3, ODF3B, H CK, LAT2, FGR, SFT2D1, IQGAP1, and CLEC7A; the monocyte2 marker-based cell groups due to IL1B, S100A12, SERPINB2, VCAN, EREG, F3, THBS1, PTGS2, GCA, PROK2, GK, EHD1, SLC25A37, and JMJD1C; the monocyte3 marker-based cell groups due to G0S2, VIM, SH3 BGRL3, FBP1, CTSB, DUSP1, IL1RN, LGALS3, and S100A11; and the monocyte4 marker-based cell groups due to IFI30, LST1, RNF144B, ACSL1, FPR2, C5AR1, CD55, CUX1, CD300E, C19orf38, IRAK3, and NLRP3. These monocytes exhibited different cancer sample ratios, with high proportions of tumor samples in the monoocyte3 group and high proportions of arranged samples in the monoocyte1, monocyte2, and monocyte3 groups, which showed different mononucleosis components in the cancer and cancer side samples.
Further, we obtained six cell subgroups in our subgroup clustering of these monocytes (Figure 5A), which included monocytes, CD8+ T cells, and microglial cells. We observed that microglial cells mainly originated from tumor samples, and monocyte2 originated mainly from cancer side samples (Figure 5B). Further comparison of the pathway enrichment levels between these cell clusters, such as by step5.spia.txt using the SPIA method showed that CD8+ T cell abnormalities had the least pathway impacts, and may represent a class of mononucleocytes that make up a small number of normal samples, in addition to several other classes that showed a large number of different path abnormalities (Figure 5C). We observed multiple inhibition pathways in microglial cells with significant activation pathways in other cells, such as RNA transport and T cell receptor signaling pathways, which suggested the presence of a unique class of dysfunctional monocyte clusters in the tumor samples. In addition, the clusters of microglial cells, CD8+ T cells, monocyte2 cells, and monocyte3 cells represented a higher level of major histocompatibility complexes (MHCs) (Figure 5D).
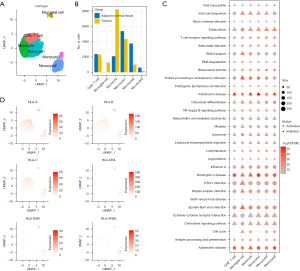
mRNA expression and differences between B-cell subclasses
B lymphocytic dysfunction, which is an important component of adaptive immunity, is thought to be significant in the pathogenesis of tumors, where two B cell groups were detected. There were 11,104 cells in the B cell group, labeled as CD69, FCER2, BANK1, FCMR, ADAM28, SIDT1, CXCR5, LY9, NGLY1, PARP15, BLK, and ARHGAP24. Of the cells in the B cell group, 1,312 were marker-based groups due to LRMP, TCL1A, BCAS4, CD22, VPREB3, SWAP70, and EZR. These B cells exhibited different proportions of tumor samples, and through t-SNE analysis, the B cells were divided into six clusters (Figure 6A), where the CD8+ T cells, NK cells, and microglial cells were mainly located in the tumor samples and the microglial cells and neutrophils were similarly located in the tumor and cancer side samples. This suggested that there was a similar group of cells (microglial cell, neutrophil) in the cancer and cancer side samples. To compare the functional differences between the tumor samples and the cancer side samples in the microglial cells and neutrophils, we calculated the difference in the KEGG pathway enrichment of the tumors in the two cell groups using the GSEA method. We observed that a total of 106 pathways were enriched in microglial cells. These pathways were enriched in the cancer side samples (e.g., step6.gsea.kegg.txt), the most significant of which were CARDIAC_MUSCLE_CONTRACTION, OOCYTE_MEIOSIS, and HUNTINGTONS_DISEASE (Figure 6B). Interestingly, the neutrophils had a total of 55 pathways (step6.gsea.kegg_B.txt), which were concentrated in the cancer side samples, the most significant of which were the first three pathways: ALZHEIMERS_DISEASE, SELENOAMINO_ACID_METABOLISM, and PARKINSONS_DISEASE (Figure 6C). All of these were associated with geriatric diseases. In addition, there was a significant intersection between the enriched pathways in the neutrophil and the rich pathways of microglial cells (Figure 6D). This suggested that there were some similarities in the disordered pathways of the tumor samples at the pathway level in the different types of cells.
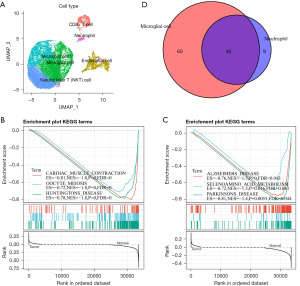
Identification of the genetic signature associated with prognosis
The prognosis of esophageal cancer is closely related to molecular genes (25). To identify prognostically-related genetic markers, we selected the cell group with the closest proportion of normal and tumor samples. We extracted the gene marker from the source cell subgroup, obtaining a total of 3,154 gene markers. First, we calculated that the 4,211 gene expression differences in stage III, stage II, and normal samples contained a total of 460 genes, (scRNAsub.exp.edgp.txt). We defined the sample feature vector as follows: normal 0, Stage II 2, and Stage III 3. We then calculated the Spearman’s rank correlation coefficient of the expression of these genes in each cell and sample feature vector, selecting a false discovery rate (FDR) <0.05 to obtain a total of 177 significantly related genes (scRNAsub.exp.edgp.cr.txt).
In the functional enrichment analysis of these genes using the clusterProfiler R software package, we observed that they were mainly concentrated in the KEGG pathways, such as the ribosome, leukocyte transendothelial migration, and viral myocarditis pathways (Figure 7A). In addition, they enriched the molecular functions of the structural constituents of the ribosome (Figure 7B), and we observed that these genes were enriched into a large number of biological processes and cellular components, such as the nuclear-transcribed mRNA catabolic processes and nonsense-mediated decay (Figure 7C). In cellular components, such as cell-substrate-adhesin junctions and focal adhesions (Figure 7D), these biological pathways were closely related to tumor proliferation and metastasis. We mapped these genes into the Search Tool for the Retrieval of Interacting Genes/Proteins (STRING) version 11.0b (www.string-db.org) database to obtain protein interoperability networks (ppi.network.txt) of these genes and analyzed the interrelationships of these genes to the network degree distribution (ppi.attr.table.txt) (Figure 7E), the closeness centrality distribution (Figure 7F), and the betweenness centrality distribution (Figure 7G). The proximity to centrality and the greater the amount of centrality indicate the importance of the network. We selected the genes of the top five degrees, closeness centrality, and betweenness centrality, and then selected the intersection of the three to identify two important genes, CXCL8 and CCL2. Finally, we observed that the CXCL8 gene had higher expressions in multiple cell groups (Figure 7H) and that the CCL2 gene expressed only weak signals in each cell population; hence, we considered CXCL8 an effective potential prognostic marker.
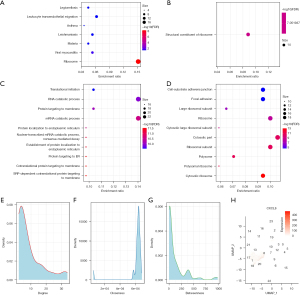
TCGA dataset validation
To verify the prognostic correlation of CXCL8, we downloaded the TCGA-Expression Spectrum Data Set (TCGA-ESDS; TCGA-ESCA.exp.txt) and the corresponding clinical follow-up data (TCGA-ESCA.exp.os.txt), from which the expression spectrum of CXCL8 was extracted and differences in expression between the cancer and cancer side tissue samples (Figure 8A) could be observed. In the tumor samples, the expression of CXCL8 was significantly higher than that in the cancer side samples. In addition, we compared the relationship between CXCL8 expression and prognosis using the maxstat R package to group CXCL8 expression and analyzed the prognosis by considering the differences in OS (Figure 8B), disease-free survival (DFS) (Figure 8C), and disease-specific survival (DSS) (Figure 8D) in patients with high and low expression. We observed significant differences between prognosis and the low expression group, which indicated that CXCL8 is a potential prognostic marker in EC.
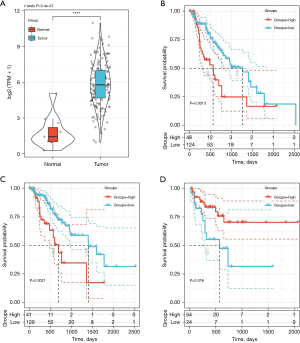
Discussion
Many patients are diagnosed with advanced EC at first diagnosis, having already surpassed the optimal treatment period, and thereby receive a relatively poor prognosis (26). Currently, the most common method of predicting the prognosis of patients with EC is the American Joint Committee on Cancer (AJCC) staging system (27). However, patients at the same clinical stage may still experience different survival periods, which may be related to the different molecular characteristics of the tumor. Therefore, it is important to develop a more sensitive prognostic/diagnostic approach based on the molecular characteristics of the patient’s tumor by identifying new prognostic markers. We identified cell subgroups using t-SNE analysis and found differences in cell cluster enrichment between tumor and normal tissues, where clusters 0, 1, 6, and 4 were mainly found in the tumor tissue and clusters 5, 8, 2, and 3 were mainly found in the paracancerous tissue. In addition, we observed different proportions of cell populations emerging in the tumor samples. These results indicate significant individual differences in these cells in different patients.
Tumor heterogeneity has long been considered an important obstacle in the clinical treatment of tumors. Different patients may experience different treatment responses due to complex immunogenicity (28,29). Existing immunotherapy is based on immune checkpoint-specific treatment options for T cell antigen receptors; however, not all patients benefit from immunotherapy due to the immune heterogeneity of tumors (30). Most patients exhibit significant differences in response and resistance, suggesting that further analysis of the individual tissues and individual cells in tumor patients is necessary to develop specific immunotherapy strategies (31). Based on recent reports and sequencing data, immune cells can more systematically describe the molecular signatures of the immune system (32-34).
Genes that differ from the specific cell subgroups of Paneth cell, NK cell, exhausted CD8+ T cell, effector CD8+ Tem cell, and B cell are highly concentrated in the Ribosome and Parkinson’s disease pathways. These results showed that there were some similarities in dysfunction between the cancer and paracancerous samples in specific cell subgroups. There were significantly different tumor microenvironmental characteristics in samples at different clinical phases, the most significant differences being in the phases of B cells and monocytes. The gene enrichment pathways in these two types of cells were generally the same, and the differences in the pathways were mainly related to RNA transcription and degradation.
Patients with advanced EC, whether untreated or after multiple treatments, may benefit from this treatment (3,5,35). However, the use of immune checkpoint inhibitors (ICIs) in the neoadjuvant treatment phase is still very limited. In the context of tumor immunotherapy, neoadjuvant immunotherapy may have a more prominent advantage, as antigen exposure will enhance the degree of the tumor-specific T-cell response and prolong the duration of action (4). In this study, it was found that there were significantly different tumor microenvironmental characteristics in samples with different clinical stages. B cells and monocytes exhibited different characteristics in stage II and stage III, and the MAPK signaling pathway exhibited activation and inhibition due to different gene participation in these two cell types, respectively. A preclinical study has shown that modulating the MAPK signaling pathway can improve the sensitivity of melanoma to immunotherapy. Inhibiting Mitogen-activated extracellular signal-regulated kinase (MEK) activity in melanoma cell lines can increase antigen levels, thereby enhancing the immunity of antitumor T cells (36). In addition, a study has shown that regulating MEK activity can affect the infiltration of immune cells in the TME, including T cells and B regulatory cells, thereby enhancing the response to anti-programmed death 1 (PD1) immunotherapy and anti-tumor immunity (37). Animal models have shown that regulating the MAPK pathway activity can affect the number of inter-tumor, effector-phenotype CD8 T cells, thereby inhibiting tumor growth. Moreover, a synergistic effect has been observed after the combined use of pathway regulators with programmed death ligand-1 (PD-L1) drugs (38). Another study found that metformin activated the JAK1/2/3/STAT5 and AKT/mTOR pathways in a p38 MAPK-dependent manner, significantly enhancing NK cytotoxicity, which increases the infiltration of NK cells and T cells into tumors (39).
By amalgamating the results of this study and previous studies, it is reasonable to assume that the MAPK signaling pathway has a regulatory effect on immune infiltration in the TME and the response to immunotherapy. The pre-trial results of nivolumab combined with neoadjuvant chemoradiotherapy included 16 patients with stage II–III EC or esophageal-gastric junction cancer, five patients with a pathological complete response (pCR), nine patients with a pathological hypothesis, and 15 patients with an R0 resection. The immune microenvironment in samples of different stages and the functional pathways enriched by different gene concentrations can affect immune efficacy to some extent, suggesting that the application of immunotherapy in the early stage of the clinical staging of cancer patients may be reasonable.
NK cells have a dual function of direct killing and immune regulation, performing important tasks in the complex immune processes, either by lysing tumor cells directly through antibody-dependent cell-mediated cytotoxicity and removing MHC-damaged cells in the primary immune response or by PD-L1 induction of tumors and the recruitment of dendritic cells and subsequent T cells to initiate the TME for the secondary adaptive immune response (40). In this study, we found that NK cells had the most differential genes among the three relatively enriched cell populations in paracrine signaling samples. It can be assumed that differences in NK cell distribution and gene expression in EC samples are closely related to the efficacy of immunotherapy, and this finding also provides information for subsequent studies to enhance the suicidal effect of NK cells and induce secondary adaptive immune responses.
We selected the marker gene in the secretory cell group that was closest to the normal sample and tumor sample ratio and found that the CXCL8 gene had a higher expression in multiple cell groups. The CXCL8 gene [also known as interleukin (IL-8)] is a versatile, pro-inflammatory chemokine that was originally recognized as a neutrophil chemical attractant, and has also been shown to play an important role in the development of tumorigenesis in recent years. CXCL8 is upregulated at the tumor invasion front in several human cancers. A study has also shown that the interaction of CXCL8 between tumors and the TME is achieved by influencing angiogenesis, genetic diversity, survival, proliferation, immune escape, metastasis, and multidrug resistance, thereby promoting tumor progression (41).
The role of CXCL8 in gastrointestinal tumors is increasingly being demonstrated. There is evidence that exosome release triggers the production of CXCL8, leading to increased neutrophil recruitment and neutrophil extracellular trap formation in the colon cancer TME, ultimately leading to the deterioration of colon cancer (42). A study has also confirmed that the high expression of IL-8 is associated with poor clinical prognosis in colorectal cancer patients (43). In addition, serum CXCL8 levels are considered an important risk factor for the development of gastric cancer (44). The CXCL8 gene plays a role in the association of epigenetic changes with the emergence of immunosuppressive microenvironments (45). Therefore, deciphering the regulatory/signaling cascade of CXCL8 and its downstream effects may have prognostic and clinical prospects for cancer therapy drugs directed by the TME.
Although significant progress has been made in the development of scRNA-seq technology, there are still some limitations. The scRNA-seq of eukaryotic cells is limited to the detection of large amounts of non-lipoprotein RNA [poly(A)-RNA] expression in mammalian cells with poly(A) tail [poly(A)-RNAs]. The advantages of reading rRNA sequencing are avoided by initiating oligonucleotides (dT). However, this approach inevitably excludes other RNA information that does not have poly(A), such as circRNA and microRNAs. In recent years, numerous studies have shown that non-coding RNAs (including circRNA and microRNAs) play an important role in the development of various tumors, which has become a hotspot for tumor mechanism research. Therefore, ongoing developments in this field are important for further applications of scRNA-seq with potential clinical significance (46,47).
In this study, we found specific cell subgroups in cancerous and paracancerous samples, such as the Paneth cell, NK cell, and exhausted CD8+ T cell in the tumor samples and the CD8+ Tem cell and B cell in normal tissues. Unlike the monocyte in stage II and stage III, these differences may be related to RNA transcription and degradation. We believe that CXCL8 is a valid potential prognostic marker. Several clinical samples and prospective studies are still needed to evaluate the value of the risk model for predicting the prognosis and immune response of EC patients and to determine the optimal cutoff value.
Conclusions
Cell groups with homogenous cell surface markers have intercellular variations that exert a considerable effect on cell function. Our findings will contribute to the comprehension of the TME and cellular heterogeneity in EC patients and serve as a valuable resource for in-depth exploration of the pathogenesis of EC and identification of potential therapeutic targets in the future.
Acknowledgments
This research was partly presented at the ESMO 24th World Congress on GI Cancer, Barcelona, Spain.
Funding: This work was supported by the National Natural Science Foundation of China (No. 82102827), the Chinese Society of Clinical Oncology (No. Y-Young2020-0003), Shanghai Sailing Program (No. 20YF1429200), and the Beijing Bethune Charitable Foundation (No. flzh202119).
Footnote
Reporting Checklist: The authors have completed the STREGA reporting checklist. Available at https://jtd.amegroups.com/article/view/10.21037/jtd-23-317/rc
Peer Review File: Available at https://jtd.amegroups.com/article/view/10.21037/jtd-23-317/prf
Conflicts of Interest: All authors have completed the ICMJE uniform disclosure form (available at https://jtd.amegroups.com/article/view/10.21037/jtd-23-317/coif). The authors have no conflicts of interest to declare.
Ethical Statement: The authors are accountable for all aspects of the work in ensuring that questions related to the accuracy or integrity of any part of the work are appropriately investigated and resolved. The study was conducted in accordance with the Declaration of Helsinki (as revised in 2013).
Open Access Statement: This is an Open Access article distributed in accordance with the Creative Commons Attribution-NonCommercial-NoDerivs 4.0 International License (CC BY-NC-ND 4.0), which permits the non-commercial replication and distribution of the article with the strict proviso that no changes or edits are made and the original work is properly cited (including links to both the formal publication through the relevant DOI and the license). See: https://creativecommons.org/licenses/by-nc-nd/4.0/.
References
- Sung H, Ferlay J, Siegel RL, et al. Global Cancer Statistics 2020: GLOBOCAN Estimates of Incidence and Mortality Worldwide for 36 Cancers in 185 Countries. CA Cancer J Clin 2021;71:209-49. [Crossref] [PubMed]
- Huang J, Koulaouzidis A, Marlicz W, et al. Global Burden, Risk Factors, and Trends of Esophageal Cancer: An Analysis of Cancer Registries from 48 Countries. Cancers (Basel) 2021;13:141. [Crossref] [PubMed]
- Kato K, Cho BC, Takahashi M, et al. Nivolumab versus chemotherapy in patients with advanced oesophageal squamous cell carcinoma refractory or intolerant to previous chemotherapy (ATTRACTION-3): a multicentre, randomised, open-label, phase 3 trial. Lancet Oncol 2019;20:1506-17. [Crossref] [PubMed]
- Huang J, Xu J, Chen Y, et al. Camrelizumab versus investigator's choice of chemotherapy as second-line therapy for advanced or metastatic oesophageal squamous cell carcinoma (ESCORT): a multicentre, randomised, open-label, phase 3 study. Lancet Oncol 2020;21:832-42. [Crossref] [PubMed]
- Kojima T, Shah MA, Muro K, et al. Randomized Phase III KEYNOTE-181 Study of Pembrolizumab Versus Chemotherapy in Advanced Esophageal Cancer. J Clin Oncol 2020;38:4138-48. [Crossref] [PubMed]
- Shah MA, Kennedy EB, Catenacci DV, et al. Treatment of Locally Advanced Esophageal Carcinoma: ASCO Guideline. J Clin Oncol 2020;38:2677-94. [Crossref] [PubMed]
- Hui L, Chen Y. Tumor microenvironment: Sanctuary of the devil. Cancer Lett 2015;368:7-13. [Crossref] [PubMed]
- Hirata E, Sahai E. Tumor Microenvironment and Differential Responses to Therapy. Cold Spring Harb Perspect Med 2017;7:a026781. [Crossref] [PubMed]
- Sharma P, Hu-Lieskovan S, Wargo JA, et al. Primary, Adaptive, and Acquired Resistance to Cancer Immunotherapy. Cell 2017;168:707-23. [Crossref] [PubMed]
- Su S, Zhao J, Xing Y, et al. Immune Checkpoint Inhibition Overcomes ADCP-Induced Immunosuppression by Macrophages. Cell 2018;175:442-457.e23. [Crossref] [PubMed]
- Hugo W, Zaretsky JM, Sun L, et al. Genomic and Transcriptomic Features of Response to Anti-PD-1 Therapy in Metastatic Melanoma. Cell 2016;165:35-44. [Crossref] [PubMed]
- Jiang D, Liu Y, Wang H, et al. Tumour infiltrating lymphocytes correlate with improved survival in patients with esophageal squamous cell carcinoma. Sci Rep 2017;7:44823. [Crossref] [PubMed]
- Huang ZL, Lin ZR, Xiao YR, et al. High expression of TACC3 in esophageal squamous cell carcinoma correlates with poor prognosis. Oncotarget 2015;6:6850-61. [Crossref] [PubMed]
- Li Y, Lu Z, Che Y, et al. Immune signature profiling identified predictive and prognostic factors for esophageal squamous cell carcinoma. Oncoimmunology 2017;6:e1356147. [Crossref] [PubMed]
- Cao K, Ma T, Ling X, et al. Development of immune gene pair-based signature predictive of prognosis and immunotherapy in esophageal cancer. Ann Transl Med 2021;9:1591. [Crossref] [PubMed]
- Stein CM, Weiskirchen R, Damm F, et al. Single-cell omics: Overview, analysis, and application in biomedical science. J Cell Biochem 2021;122:1571-8. [Crossref] [PubMed]
- Potter SS. Single-cell RNA sequencing for the study of development, physiology and disease. Nat Rev Nephrol 2018;14:479-92. [Crossref] [PubMed]
- Ren X, Zhang L, Zhang Y, et al. Insights Gained from Single-Cell Analysis of Immune Cells in the Tumor Microenvironment. Annu Rev Immunol 2021;39:583-609. [Crossref] [PubMed]
- Lau D, Bobe AM, Khan AA. RNA Sequencing of the Tumor Microenvironment in Precision Cancer Immunotherapy. Trends Cancer 2019;5:149-56. [Crossref] [PubMed]
- Wang Z, Xie L, Ding G, et al. Single-cell RNA sequencing of peripheral blood mononuclear cells from acute Kawasaki disease patients. Nat Commun 2021;12:5444. [Crossref] [PubMed]
- Korsunsky I, Millard N, Fan J, et al. Fast, sensitive and accurate integration of single-cell data with Harmony. Nat Methods 2019;16:1289-96. [Crossref] [PubMed]
- Young MD, Mitchell TJ, Vieira Braga FA, et al. Single-cell transcriptomes from human kidneys reveal the cellular identity of renal tumors. Science 2018;361:594-9. [Crossref] [PubMed]
- Liao J, Yu Z, Chen Y, et al. Single-cell RNA sequencing of human kidney. Sci Data 2020;7:4. [Crossref] [PubMed]
- Yu G, Wang LG, Han Y, et al. clusterProfiler: an R package for comparing biological themes among gene clusters. OMICS 2012;16:284-7. [Crossref] [PubMed]
- Zhang J, Zhou Y, Zhang B, et al. Bioinformatics analysis identifying FBXO45 gene as a potential oncogene in esophageal cancer. J Gastrointest Oncol 2021;12:2653-64. [Crossref] [PubMed]
- Ko HH, Cheng SL, Lee JJ, et al. Factors influencing the incidence and prognosis of second primary tumors in patients with oral squamous cell carcinoma. Head Neck 2016;38:1459-66. [Crossref] [PubMed]
- Hsu PK, Chen HS, Liu CC, et al. Application of the Eighth AJCC TNM Staging System in Patients With Esophageal Squamous Cell Carcinoma. Ann Thorac Surg 2018;105:1516-22. [Crossref] [PubMed]
- Seth S, Li CY, Ho IL, et al. Pre-existing Functional Heterogeneity of Tumorigenic Compartment as the Origin of Chemoresistance in Pancreatic Tumors. Cell Rep 2019;26:1518-1532.e9. [Crossref] [PubMed]
- Shibata M, Hoque MO. Targeting Cancer Stem Cells: A Strategy for Effective Eradication of Cancer. Cancers (Basel) 2019;11:732. [Crossref] [PubMed]
- Smith A, Roy A, Karapetis CS, et al. Immunotherapy use in oesophagogastric cancers-a review of the literature. Br J Cancer 2022;127:21-9. [Crossref] [PubMed]
- Servetto A, Salomone F, Di Costanzo F, et al. Inadequate health-related quality of life assessment and reporting in phase III clinical trials of immune checkpoint inhibitors in solid cancers: A systematic review. Crit Rev Oncol Hematol 2022;172:103649. [Crossref] [PubMed]
- Li C, Liu F, Sun L, et al. Natural killer cell-related gene signature predicts malignancy of glioma and the survival of patients. BMC Cancer 2022;22:230. [Crossref] [PubMed]
- Liao P, Wang W, Wang W, et al. CD8(+) T cells and fatty acids orchestrate tumor ferroptosis and immunity via ACSL4. Cancer Cell 2022;40:365-378.e6. [Crossref] [PubMed]
- Masuda K, Kornberg A, Miller J, et al. Multiplexed single-cell analysis reveals prognostic and nonprognostic T cell types in human colorectal cancer. JCI Insight 2022;7:e154646. [Crossref] [PubMed]
- Owen D, Chaft JE. Immunotherapy in surgically resectable non-small cell lung cancer. J Thorac Dis 2018;10:S404-11. [Crossref] [PubMed]
- Kono M, Dunn IS, Durda PJ, et al. Role of the mitogen-activated protein kinase signaling pathway in the regulation of human melanocytic antigen expression. Mol Cancer Res 2006;4:779-92. [Crossref] [PubMed]
- Yarchoan M, Mohan AA, Dennison L, et al. MEK inhibition suppresses B regulatory cells and augments anti-tumor immunity. PLoS One 2019;14:e0224600. [Crossref] [PubMed]
- Ebert PJR, Cheung J, Yang Y, et al. MAP Kinase Inhibition Promotes T Cell and Anti-tumor Activity in Combination with PD-L1 Checkpoint Blockade. Immunity 2016;44:609-21. [Crossref] [PubMed]
- Xia W, Qi X, Li M, et al. Metformin promotes anticancer activity of NK cells in a p38 MAPK dependent manner. Oncoimmunology 2021;10:1995999. [Crossref] [PubMed]
- Guillerey C, Huntington ND, Smyth MJ. Targeting natural killer cells in cancer immunotherapy. Nat Immunol 2016;17:1025-36. [Crossref] [PubMed]
- Asokan S, Bandapalli OR. CXCL8 Signaling in the Tumor Microenvironment. Adv Exp Med Biol 2021;1302:25-39. [Crossref] [PubMed]
- Shang A, Gu C, Zhou C, et al. Exosomal KRAS mutation promotes the formation of tumor-associated neutrophil extracellular traps and causes deterioration of colorectal cancer by inducing IL-8 expression. Cell Commun Signal 2020;18:52. [Crossref] [PubMed]
- Wang Y, Zhao M, He S, et al. Necroptosis regulates tumor repopulation after radiotherapy via RIP1/RIP3/MLKL/JNK/IL8 pathway. J Exp Clin Cancer Res 2019;38:461. [Crossref] [PubMed]
- Pawluczuk E, Łukaszewicz-Zając M, Gryko M, et al. Serum CXCL8 and Its Specific Receptor (CXCR2) in Gastric Cancer. Cancers (Basel) 2021;13:5186. [Crossref] [PubMed]
- Dong F, Qin X, Wang B, et al. ALKBH5 Facilitates Hypoxia-Induced Paraspeckle Assembly and IL8 Secretion to Generate an Immunosuppressive Tumor Microenvironment. Cancer Res 2021;81:5876-88. [Crossref] [PubMed]
- Kharchenko PV. The triumphs and limitations of computational methods for scRNA-seq. Nat Methods 2021;18:723-32. [Crossref] [PubMed]
- González-Silva L, Quevedo L, Varela I. Tumor Functional Heterogeneity Unraveled by scRNA-seq Technologies. Trends Cancer 2020;6:13-9. [Crossref] [PubMed]
(English Language Editor: A. Kaseem)