Bioinformatics analysis based on DNA methylation data identified in lung adenocarcinoma subgroups with different immune characteristics and clinical outcomes
Highlight box
Key findings
• Three DNA methylated subgroups were identified; subgroup C3 had the best prognosis, while subgroup C2 exhibited the lowest immune cell infiltration and the highest mRNAsi, mDNAsi, and TMB, which may contribute to the development of personalized therapy for new specific subtypes of LUAD.
What is known and what is new?
• DNA methylation can be used as a predictor for clinical outcomes and tumor subtypes.
• Three DNA-methylated subgroups provide a supplement for the LUAD typing system.
What is the implication, and what should change now?
• This study offers novel insights into the underlying mechanism of DNA methylation in LUAD and provides directions for the personalized treatment of specific LUAD subtypes.
Introduction
Lung cancer is the most common cause of malignancy-related death. Its prognosis is poor, with 75% of patients diagnosed at an advanced stage (1). Lung cancer is the result of multi-stage carcinogenesis, and the underlying molecular basis involves the complex interaction between genetic and epigenetic factors of significant diversity. These factors induce the activation of key oncogenes and the inactivation of tumor suppressor genes in a cancer tissue-specific way (2). In recent years, advances in molecular strategies and analysis platforms, including genomics, epigenomics, and proteomics, have been observed, which make it possible to accurately classify lung cancer subtypes and analyze their detailed mechanisms (3).
An enormous field of lung cancer molecular landscape is determined by epigenetic changes (1). Epigenetic changes, such as DNA methylation, histone modification, and small non-coding RNA, are heritable and reversible (4). DNA methylation is a particular focus of human cancer research, which leads to chromosome instability through global hypomethylation and abnormal gene expression by changing the methylation level of the promoter cytosine-guanine (CpG) island (5). Scientific research has been conducted on the existence of significant biomarkers of DNA methylation and their clinical association with lung cancer (6). Although research on single-gene methylation and the methylation profile of lung cancer has progressed (7), only 14 of these biomarkers have been converted into commercially available clinical products, including adenomatous polyposis coli protein (APC), glutathione S-transferase P (GSTP1), methylated-DNA-protein-cysteine methyltransferase (MGMT), ras association domain-containing protein 1 (RASSF1), septin-9 (SEPT9), short stature homeobox protein 2 (SHOX2), N-myc downregulated gene 4 (NDRG4), bone morphogenetic protein 3 (BMP3), orthodenticle homeobox 1 (OTX1), twist-related protein 1 (TWIST1), one cut domain family member 2 (ONECUT2), branched-chain-amino-acid aminotransferase, cytosolic (BCAT1), and DNA-binding protein Ikaros (IKZF1) (8). It is reported that the expression and function of methyltransferases are responsible for immune regulation to some extent and may have an impact on DNA methylation modification patterns in human cancers (9). Choi et al. showed that a DNA methyltransferase inhibitor (DNMTi) attenuated graft-versus-host disease without compromising the graft-versus-leukaemia effect by converting effector T cells into regulatory T cells (10).
For the treatment of lung cancer, previous clinical studies have shown that the DNMTis targeting DNMT also represents an epigenetic drug for lung cancer. DNMTis 5-Azacytidine (5-AzaC) and its analogue, 5-Aza-2'-deoxycytidine (5-Aza-CdR), act by demethylating tumor suppressor genes to inhibit tumors. However, 5-Aza-CdR also has a risk of poor specificity and improper dosing, which may lead to tumor metastasis (11). In addition to predicting clinical outcomes, differential DNA methylation can also be used to distinguish tumor subtypes, indicate treatment responsiveness, and determine treatment strategies (12). Therefore, it is necessary to study DNA methylation in lung cancer.
In this study, we proposed a novel lung adenocarcinoma (LUAD) typing system based on 782 immune cell marker gene-related methylation sites and revealed the survival outcomes, clinical features, immune cell infiltration, stem cell characteristics, and genomic variations of each molecular subgroup. Our work demonstrated the different states of molecular subgroups defined by the immune-related methylation sites, which may be helpful for the personalized management and monitoring of LUAD in practice. We present the following article in accordance with the STREGA reporting checklist (available at https://jtd.amegroups.com/article/view/10.21037/jtd-23-494/rc).
Methods
Acquisition and preprocessing of research data
RNA-sequencing (RNA-seq) data, DNA methylation data generated by Illumina Infinium HumanMethylation 450 BeadChip array, copy number variation (CNV) data (Masked Copy Number Segment, affymetrix snp 6.0), single nucleotide variant (SNV) data (MuTect2. Variant0. Maf), and LUAD clinical follow-up pathological data were downloaded from The Cancer Genome Atlas (TCGA) (https://portal.gdc.cancer.gov/). The expression profile data was changed from fragments per kilobase million (FPKM) to transcripts per million (TPM) and transformed into log2. The processing of DNA methylation data involved the use of the k-nearest neighbors (KNN) algorithm to complete the missing values and retrograde, the removal of cytosine-guanine (CpG) sites with cross-reflection, and the exclusion of methylation sites on X and Y chromosomes. The stemness index was derived from Malta et al. (13). messenger RNA (mRNA) expression-based stemness indices (mRNAsi) was calculated based on the expression profile data, while DNA methylation-based stemness index (mDNAsi) was calculated based on the methylation data. The immune cell biomarkers gene set was obtained from the article of Charoentong et al. (14), which includes 782 genes. The workflow of this study is summarized in Figure S1. The study was conducted in accordance with the Declaration of Helsinki (as revised in 2013).
Screening of the differential methylation sites of immune markers
For the methylation data after preprocessing, only the methylation sites related to the 782 immune cell marker genes were retained. The differential methylation sites (DMS) of normal and LUAD samples were screened using the dmpFinder function of Minfi R package (V1.34.0) (15). P<0.05 and | deltabeta | >0.15 was used as the threshold for filtering. A deltabeta methylation site >0.15 was considered hypermethylation, while that <−0.15 was considered hypomethylation.
Consensus clustering analysis
The coxph function of R packet survival was used to analyze the DMS by univariate cox analysis based on the survival data. P<0.05 was used as the critical value to screen the DMS related to the prognosis of LUAD. The D2 clustering algorithm in R packet ConsensusClusterPlus was used to consistently cluster the TCGA-LUAD samples according to the prognosis-related DMS, in which the parameters were set as follows: reps =100, pItem =0.8, pFeature =1, and distance = “pearson”. The stability of the classification was verified by principal component analysis (PCA).
Single-sample gene set enrichment analysis (ssGSEA)
The immune infiltration of 28 immune cells was evaluated by R package “GSVA” and GSEABase. The 28 immune cells included two types: innate immune cells [myeloid-derived suppressor cells (MDSCs), natural killer T cells, natural killer cells, monocytes, eosinophils, plasmacytoid dendritic cells, immature dendritic cells, CD56 dim natural killer cells, CD56 bright natural killer cells, macrophages, activated dendritic cells, neutrophils, and mast cells], and adaptive immune cells (memory B cells, type 1 T helper cells, gamma delta T cells, activated B cells, type 2 T helper cells, regulatory T cells, central memory CD4 T cells, effector memory CD4 T cells, immature B cells, type 17 T helper cells, activated CD4 T cells, central memory CD8 T cells, effector memory CD8 T cells, activated cluster of differentiation (CD)8 T cells, and T follicular helper cells). The relative abundance of each immune cell in each cluster was expressed by the enrichment score calculated by ssGSEA analysis and compared between clusters by the Kruskal-Wallis test.
Infiltration analysis of immune cells provided by CIBERSORT
CIBERSORT is a tool used for the deconvolution of the expression matrix of human immune cells based on the principle of linear support vector regression, which provides a gene expression signature set for 22 immune cells (16). Using CIBERSORT, the immune infiltration of 22 immune cell species was quantified based on a known reference set. Then, the Kruskal-Wallis test was used to evaluate the immune infiltration scores between the clusters.
Extrapolation of the tumor microenvironment (TME) by ESTIMATE
ESTIMATE provides an algorithm to evaluate the presence of stromal cells and immune cell infiltration in tumor samples based on the expression characteristics of specific genes associated with stromal cells and immune cell infiltration (17). Using this method, the stromal and immune scores of each tissue sample were calculated using the public network station SourceForge software repositor (https://sourceforge.net/projects/estimateproject/).
SNV and CNV analysis
The most common mutation genes in LUAD were selected, and the mafTools (V2.4.05) software package (18) was applied to analyze and visualize the mutation pattern and frequency of the selected genes in the clusters, and the Chi-square test was used to test the mutation distribution in the cluster. Genomic Identification of Significant Targets in Cancer (GISTIC) was also used to determine the copy number amplification and deletion of genes in LUAD. Regions with a log2 ratio >0.2 were considered to be amplified, while those with a log2 ratio <0.2 were considered to be missing. The CNV patterns between clusters were compared by the chi-square test.
Statistical analysis
Statistical analysis in this study was performed in R software (version 3.6.3, https://www.r-project.org/ ver. 3.6.3). The Kaplan-Meier method and logarithmic rank test were used to examine the overall survival (OS) of the subtypes defined by DNA methylation sites using the “survival” and “survminer” R packets. The clinical sample information of clusters based on the DNA methylation site was analyzed by the chi-square test. For all statistical tests, significance was defined as P<0.05.
Results
Identification of three DNA methylated molecular subgroups by consensus clustering
A total of 6,199 methylation sites related to immunocyte marker genes were obtained. By analyzing the difference in methylation sites between the normal and LUAD samples, 336 DMS were identified, including 159 low DMS and 177 high DMS (Figure 1A). Next, univariate Cox regression analysis of DMS was performed to obtain 40 DMS associated with LUAD prognosis (Table S1). According to the consensus matrix obtained by consensus clustering of the 40 DMS, the LUAD samples in TCGA were divided into three subgroups: cluster 1 (C1), cluster 2 (C2), and cluster 3 (C3) (Figure 1B).
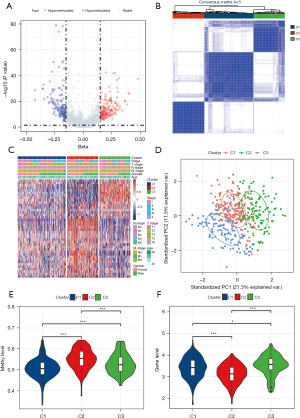
The heat map showed 40 DMS methylation levels per subgroup. Most of the methylation sites were not abundant in each sample of C1. The methylation level of C3 was higher than that of C1 but less than C2 (Figure 1C). PCA was performed to verify the results of the consensus clustering analysis. The two-dimensional score scatterplot also showed that the classification result of the consensus clustering analysis was reasonable (Figure 1D). The methylation level of the immune gene methylation site of each subgroup was quantified and compared between groups. It was observed that the methylation level of the immune gene of C2 was significantly higher than that of the other two subgroups, meanwhile the methylation level of C3 was significantly higher than that of C1 (Figure 1E). In terms of immune gene expression, C2 was obviously the lowest, and the expression of C3 was significantly higher than that of C2 (Figure 1F).
Survival and clinical characteristics of three subgroups
We analyzed the clinical features of each subgroup. The most obvious differences observed between C2 and the other two subtypes were a high proportion of death samples, a high proportion of current smokers (3), a higher T stage (T2–T4), a later N stage (N1–N2), and a relatively high proportion of late samples. For C3, the proportion of surviving patients was the highest, most of the samples were female, in the early stage of N stage and American Joint Committee on Cancer (AJCC) stage, and the proportion of current reformed smokers for more than 15 years was higher (Figure 2A). The prognostic results of the three subgroups were also estimated. Kaplan-Meier curve showed that the OS of C3 was significantly higher than that of C1 and C2, but there was no significant OS difference between C1 and C2 (Figure 2B-2E).
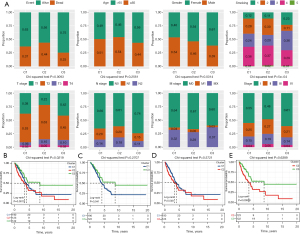
Immune cell infiltration in the different DNA methylation molecular subgroups
The infiltration of 28 immune cells in the different subgroups was studied by ssGSEA. By generating the immune cell enrichment score of each sample to draw the heat map, we found that among the three subgroups, C2 had the lowest immune cell infiltration score (Figure 3A). Meanwhile, the Kruskal-Wallis test was used to compare the immune cell infiltration scores of 28 immune cells in the three subgroups, and the results showed that all of the immune cell infiltration scores were significantly different among the three subgroups, and the most congenital and adaptive immune cells had the lowest scores in C2 (Figure 3B).
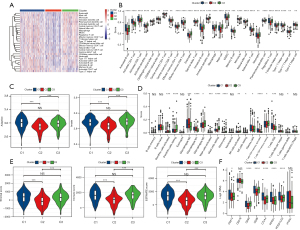
The innate and adaptive immunity scores of the three subgroups were separately considered, both of which had the lowest level in C2 (Figure 3C). The scores of 16 of the 22 immune cells calculated by CIBERSORT showed significant differences between the three subgroups (Figure 3D). In addition, ESTIMATE was applied to calculate stromal, immune, and ESTIMATE scores to evaluate the level of infiltrating matrix, immune cells, and tumor purity in the three subgroups. The C2 cluster had the lowest stromal, immune, and ESTIMATE scores among the three subgroups, indicating that this subgroup may contain the least infiltrating matrix or immune cell components (Figure 3E).
Immune checkpoint expression is often required before the treatment of tumors with immune checkpoint inhibitors, which is also an important immune indicator. The expression analysis of immune checkpoints in the three subgroups showed that six of the eight immune checkpoints exhibited significantly different expression levels among the three subgroups, including CD274 [programmed death ligand 1 (PD-L1)], cytotoxic T-lymphocyte-associated protein 4 (CTLA4), and programmed cell death 1 [PDCD1 (PD1)], which are often used as targets of immunotherapy, and they all had the lowest expression level in C2 (Figure 3F). These results indicated that C2 has weak antitumor immunity.
To provide evidence for this conclusion, the enrichment scores of nine antitumor immune reaction-related indicators in three subgroups [antigen-presenting cell (APC) co-inhibition, APC co-stimulation, endothelial cells, fibroblasts, human leukocyte antigen (HLA), inflammation-promoting, MHC class I, Type I interferon response, and Type II interferon response] (19,20) were analyzed. The results were consistent with our inference that all nine indexes related to the anti-tumor immune response of C2 were the lowest among the three subgroups (Figure 4).
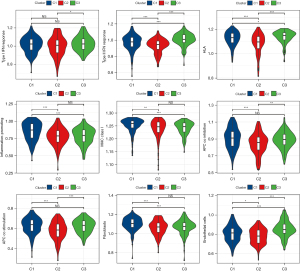
Stemness indices of different DNA-methylated molecular subgroups
In the process of tumorigenesis and development, various differentiation phenotypes disappear rapidly and acquire progenitor and stem-cell-like characteristics. Considering the close relationship between tumor stem cells and tumor progression, the performances of mRNAsi and mDNAsi in three subgroups were analyzed. The mRNAsi of the three subgroups was significantly different, and the mRNAsi of C2 was significantly higher relative to C1 and C3 (Figure 5A). In addition, the mDNAsi of C2 was also the highest among the three subgroups (Figure 5B). Both mRNAsi and mDNAsi were lowest among C3 samples with the best prognosis.
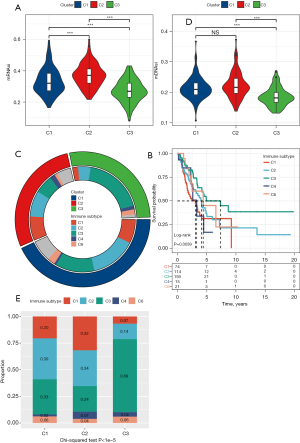
Relationship between the DNA methylation molecular subgroups and six pan-cancerous immune subtypes
We explored the associations between six pan-cancerous immune subtypes [C1: wound healing, C2: interferon (IFN)-γ dominant, C3: inflammatory, C4: lymphocyte depleted, C5: immunologically quiet, C6: transforming growth factor (TGF)-β dominant] (21) and the three immune subtypes identified in this study. The majority of LUAD patients in TCGA belonged to the C1, C2, and C3 immune subtypes (about 90.6%), and the C5 immune subtype was not present (Figure 5C).
The prediction of pan-cancer immune subtypes to OS in patients with LUAD showed that there were significant differences in survival trends among the five immune subtypes. The prognosis of pan-cancer immune subtypes C1 and C6 was poor, while that of C3 was the best (Figure 5D). There were also significant differences in the composition of five immune subtypes among the three DNA-methylated molecular subgroups. The DNA-methylated molecular subgroup C2 contains more C1 and C2 immune subtypes, and C3 immune subtypes account for the largest proportion of molecular subgroup C3 samples with the best prognosis (Figure 5E). Therefore, our LUAD classification based on DMS was consistent with the pan-cancer immune classification.
Genomic characteristics in the different DNA methylation molecular subgroups
The mutation patterns and rates of 23 common mutant genes were analyzed in different DNA-methylated molecular subgroups samples. The genes with the highest mutations in C1 and C2 were TP53, CSMD3, and RYR2, but their mutation patterns in the two DNA-methylated molecular subgroups were different. Although the mutation rate of TP53 in C1 was higher than that in C2, TP53 had more missense mutations in C2. The main mutation mode of CSMD3 in C1 was missense mutation, while that in C2 included both missense and multi-hit mutations. The most frequently mutated gene in C3 was KRAS, and the overall mutation rate of the 23 genes in the first two DNA-methylated molecular subgroups was higher than that in C3 (Figure 6A). The tumor mutational burden (TMB) of C2 was also significantly higher than that of C1 and C3 (Figure 6B).
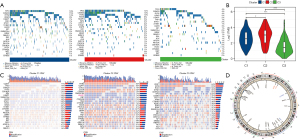
CNV analysis of three DNA-methylated molecular subgroups showed that CNV was most common in C2, and different genes had different CNV frequencies in the three DNA-methylated molecular subgroups. The main CNV of RYR2, EGFG, CSMD3, RPAF, NF1, MET, ERBB2, and ALK in C2 was amplification, while that of STK11, SMARCA4, SMARCA4, KEAP1, RB1, CDKN2A, TP53, CTNNB1, and ROS1 in C2 was deletion. CNV deletion was the main change in C3 (Figure 6C). According to the distribution of the aforementioned genes on all chromosomes, the CNV of three genes was observed on chromosomes 7, 17, and 19, and the high-frequency deletion region was found on chromosome 18 (Figure 6D).
Discussion
Differences in methylation patterns have been observed in the subtypes of lung cancer, mutation status of cancer-driven genes, and various epidemiological factors (22). Therefore, the identification of methylation sites of cancer-specific genes may be helpful to molecular classification and disease stratification (23). The field of DNA methylation analysis is rapidly shifting toward genome-wide representation, rather than studying the methylation of individual genes in tumors (24). TCGA includes a large number of Infinium Human Methylation 450 BeadChip arrays of tumor samples, which provides available bioinformatics data for the study of tumor DNA methylation. Based on the DNA methylation data of cancers in TCGA, several studies have classified a variety of malignancies, such as biliary tract (25), breast (26), cervical (27), and esophageal (28) cancers. In this study, the transcriptome and DNA methylation data of LUAD were downloaded from TCGA. Three molecular subgroups of LUAD were defined based on 782 immune cell-related DMS. The clinical differences, immunocyte infiltration, and molecular differences between them confirmed the necessity of the detailed classification of LUAD.
Among the three DNA methylated molecular subgroups, C3 had the best prognosis, and the most striking difference between this subgroup and the two subgroups was that the proportion of surviving patients was the highest, most of the samples were female, in the early stage of N stage and AJCC stage, and the proportion of current reformed smokers for more than 15 years was higher, which was consistent with a better prognosis. Unlike C3, C2 had a high proportion of death samples, a higher proportion of current smokers (3), a higher T stage, a later N stage, and a relatively high proportion of late samples, which are external indicators of poor prognosis.
The control of DNA methylation is not only essential for regulating gene transcription; its broader effects include maintaining genome integrity and immune response regulation (12). In our work, we found heterogeneity in the pattern of immune cell infiltration and the anti-tumor immune response of molecular subgroups, as defined by DMS. According to a previous study, hypermethylated subgroups had lower immune cell infiltration rates in cutaneous melanoma and breast cancer (29). Our results showed that most of the innate and adaptive immune cells had the lowest infiltration score in C2, and the infiltrating stromal and immune scores representing stromal and immune cells in this subgroup were also the lowest. The immune checkpoints related to anti-tumor immune response, especially PD-L1, CTLA4, and PDCD1, also exhibited the lowest expression level in C2. Furthermore, nine indexes related to the anti-tumor immune response of C2 showed the lowest level. Therefore, the anti-tumor immunity of C2 was weak. C2 also exhibited the most common TMB and CNV frequencies, indicating that the C2 genome was the least stable. According to a previous study, high stemness is associated with a tumor progression phenotype, poor prognosis, and genomic instability in LUAD (30). In this study, C2 showed the highest levels of mRNAsi and mDNAsi, which has been confirmed as the worst prognostic and genomically unstable phenotype.
The most advantages and disadvantages of the LUAD typing system is could predict prognosis, and immune status, and lack of experimental verification, respectively. Thus, in future, we considered the basic experiment and clinical validation.
Conclusions
In short, according to the 40 DMS, LUAD was divided into three molecular subgroups with specific DNA mutation and CNV patterns, exhibiting different clinical features, immune cell infiltration, immune and matrix scores, immune checkpoint expression, anti-tumor immune response, and stemness. Our results may provide potential strategies for the scientific study of different epigenetic subtypes.
Contribution to the field statement
In this study, a total of 40 DMS were obtained by difference and univariate COX analyses, and the LUAD samples of TCGA were divided into three subgroups: cluster 1 (C1), cluster 2 (C2), and cluster 3 (C3). Among these subgroups, the overall survival (OS) of C3 was significantly higher than that of C1 and C2. Compared with C1 and C3, C2 had the lowest innate immune cell and adaptive immune cell infiltration scores; the lowest stromal score, immune score, and iconic immune checkpoint expression; and the highest expression of mRNA expression-based stemness indices (mRNAsi), DNA methylation-based stemness index (mDNAsi), and tumor mutational burden (TMB). We proposed a LUAD typing system for DMS, which was closely related to the survival, clinical features, immune characteristics, and genomic variations of LUAD, and may contribute to the development of personalized treatment for new specific subtypes.
Acknowledgments
Funding: The research was supported by the Scientific Research Projects of Medical and Health Institutions of Longhua District, Shenzhen (Nos. 2022011, 2022105, 2020096); the Basic and Applied Basic Research Fund of Guangdong Province (2021A1515110967).
Footnote
Reporting Checklist: The authors have completed the STREGA reporting checklist. Available at https://jtd.amegroups.com/article/view/10.21037/jtd-23-494/rc
Peer Review File: Available at https://jtd.amegroups.com/article/view/10.21037/jtd-23-494/prf
Conflicts of Interest: All authors have completed the ICMJE uniform disclosure form (available at https://jtd.amegroups.com/article/view/10.21037/jtd-23-494/coif). The authors have no conflicts of interest to declare.
Ethical Statement: The authors are accountable for all aspects of the work in ensuring that questions related to the accuracy or integrity of any part of the work are appropriately investigated and resolved. The study was conducted in accordance with the Declaration of Helsinki (as revised in 2013).
Open Access Statement: This is an Open Access article distributed in accordance with the Creative Commons Attribution-NonCommercial-NoDerivs 4.0 International License (CC BY-NC-ND 4.0), which permits the non-commercial replication and distribution of the article with the strict proviso that no changes or edits are made and the original work is properly cited (including links to both the formal publication through the relevant DOI and the license). See: https://creativecommons.org/licenses/by-nc-nd/4.0/.
References
- Wadowska K, Bil-Lula I, Trembecki Ł, et al. Genetic Markers in Lung Cancer Diagnosis: A Review. Int J Mol Sci 2020;21:4569. [Crossref] [PubMed]
- Yang Z, Xu F, Wang H, et al. Pan-cancer characterization of long non-coding RNA and DNA methylation mediated transcriptional dysregulation. EBioMedicine 2021;68:103399. [Crossref] [PubMed]
- Deng S, Lu X, Zhang Z, et al. Identification and assessment of PLK1/2/3/4 in lung adenocarcinoma and lung squamous cell carcinoma: Evidence from methylation profile. J Cell Mol Med 2021;25:6652-63. [Crossref] [PubMed]
- Brait M, Sidransky D. Cancer epigenetics: above and beyond. Toxicol Mech Methods 2011;21:275-88. [Crossref] [PubMed]
- Kerr KM, Galler JS, Hagen JA, et al. The role of DNA methylation in the development and progression of lung adenocarcinoma. Dis Markers 2007;23:5-30. [Crossref] [PubMed]
- Kang C, Wang D, Zhang X, et al. Construction and Validation of a Lung Cancer Diagnostic Model Based on 6-Gene Methylation Frequency in Blood, Clinical Features, and Serum Tumor Markers. Comput Math Methods Med 2021;2021:9987067. [Crossref] [PubMed]
- Heller G, Zielinski CC, Zöchbauer-Müller S. Lung cancer: from single-gene methylation to methylome profiling. Cancer Metastasis Rev 2010;29:95-107. [Crossref] [PubMed]
- Koch A, Joosten SC, Feng Z, et al. Analysis of DNA methylation in cancer: location revisited. Nat Rev Clin Oncol 2018;15:459-66. [Crossref] [PubMed]
- Yuan D, Wei Z, Wang Y, et al. DNA Methylation Regulator-Meditated Modification Patterns Define the Distinct Tumor Microenvironment in Lung Adenocarcinoma. Front Oncol 2021;11:734873. [Crossref] [PubMed]
- Choi J, Ritchey J, Prior JL, et al. In vivo administration of hypomethylating agents mitigate graft-versus-host disease without sacrificing graft-versus-leukemia. Blood 2010;116:129-39. [Crossref] [PubMed]
- Liang R, Li X, Li W, et al. DNA methylation in lung cancer patients: Opening a "window of life" under precision medicine. Biomed Pharmacother 2021;144:112202. [Crossref] [PubMed]
- Lakshminarasimhan R, Liang G. The Role of DNA Methylation in Cancer. Adv Exp Med Biol 2016;945:151-72. [Crossref] [PubMed]
- Malta TM, Sokolov A, Gentles AJ, et al. Machine Learning Identifies Stemness Features Associated with Oncogenic Dedifferentiation. Cell 2018;173:338-354.e15. [Crossref] [PubMed]
- Charoentong P, Finotello F, Angelova M, et al. Pan-cancer Immunogenomic Analyses Reveal Genotype-Immunophenotype Relationships and Predictors of Response to Checkpoint Blockade. Cell Rep 2017;18:248-62. [Crossref] [PubMed]
- Aryee MJ, Jaffe AE, Corrada-Bravo H, et al. Minfi: a flexible and comprehensive Bioconductor package for the analysis of Infinium DNA methylation microarrays. Bioinformatics 2014;30:1363-9. [Crossref] [PubMed]
- Newman AM, Liu CL, Green MR, et al. Robust enumeration of cell subsets from tissue expression profiles. Nat Methods 2015;12:453-7. [Crossref] [PubMed]
- Yoshihara K, Shahmoradgoli M, Martínez E, et al. Inferring tumour purity and stromal and immune cell admixture from expression data. Nat Commun 2013;4:2612. [Crossref] [PubMed]
- Mayakonda A, Lin DC, Assenov Y, et al. Maftools: efficient and comprehensive analysis of somatic variants in cancer. Genome Res 2018;28:1747-56. [Crossref] [PubMed]
- Sui X, Lei L, Chen L, et al. Inflammatory microenvironment in the initiation and progression of bladder cancer. Oncotarget 2017;8:93279-94. [Crossref] [PubMed]
- Xiao Y, Ma D, Zhao S, et al. Multi-Omics Profiling Reveals Distinct Microenvironment Characterization and Suggests Immune Escape Mechanisms of Triple-Negative Breast Cancer. Clin Cancer Res 2019;25:5002-14. [Crossref] [PubMed]
- Thorsson V, Gibbs DL, Brown SD, et al. The Immune Landscape of Cancer. Immunity 2018;48:812-830.e14. [Crossref] [PubMed]
- Hoang PH, Landi MT. DNA Methylation in Lung Cancer: Mechanisms and Associations with Histological Subtypes, Molecular Alterations, and Major Epidemiological Factors. Cancers (Basel) 2022;14:961. [Crossref] [PubMed]
- Suzuki M, Yoshino I. Aberrant methylation in non-small cell lung cancer. Surg Today 2010;40:602-7. [Crossref] [PubMed]
- Pfeifer GP, Rauch TA. DNA methylation patterns in lung carcinomas. Semin Cancer Biol 2009;19:181-7. [Crossref] [PubMed]
- Qiu Z, Ji J, Xu Y, et al. Common DNA methylation changes in biliary tract cancers identify subtypes with different immune characteristics and clinical outcomes. BMC Med 2022;20:64. [Crossref] [PubMed]
- Zhang S, Wang Y, Gu Y, et al. Specific breast cancer prognosis-subtype distinctions based on DNA methylation patterns. Mol Oncol 2018;12:1047-60. [Crossref] [PubMed]
- Li C, Ke J, Liu J, et al. DNA methylation data-based molecular subtype classification related to the prognosis of patients with cervical cancer. J Cell Biochem 2020;121:2713-24. [Crossref] [PubMed]
- Chen H, Qin Q, Xu Z, et al. DNA methylation data-based prognosis-subtype distinctions in patients with esophageal carcinoma by bioinformatic studies. J Cell Physiol 2021;236:2126-38. [Crossref] [PubMed]
- Jeschke J, Bizet M, Desmedt C, et al. DNA methylation-based immune response signature improves patient diagnosis in multiple cancers. J Clin Invest 2017;127:3090-102. [Crossref] [PubMed]
- Liu Q, Lei J, Zhang X, et al. Classification of lung adenocarcinoma based on stemness scores in bulk and single cell transcriptomes. Comput Struct Biotechnol J 2022;20:1691-701. [Crossref] [PubMed]
(English Language Editor: A. Kassem)