Analysis of the sensitivity and specificity of compressed sensing magnetic resonance imaging in the diagnosis of heart failure
Highlight box
Key findings
• MRI based on the compressed sensing algorithm had better overall quality and displayed the cardiac structure.
• The PFR2 and the FV2 had good classification ability and could be used as effective indicators for evaluating heart failure.
What is known and what is new?
• Ultrasound is still the mainstream method used to diagnose left ventricular function in patients with heart failure, but few studies have examined the use of cardiac MRI.
• We performed cardiac MRI scanning in patients with heart failure and constructed a MRI image reconstruction algorithm based on compressed sensing.
What is the implication, and what should change now?
• MRI based on the compressed sensing imaging algorithm has diagnostic value for heart failure.
Introduction
Heart failure (HF) refers to a group of syndromes with the clinical manifestations of pulmonary circulation and/or systemic circulation congestion and insufficient organ and tissue blood perfusion caused by various cardiac structural or functional diseases, resulting in impaired ventricular filling and/or ejection function and the failure of cardiac output to meet the metabolic needs of body tissues. The main manifestations are dyspnea, limited physical activity, and fluid retention (1-3). The common predisposing factors of HF include infection, serious arrhythmia, anemia, pregnancy, emotional excitement, and pulmonary embolism (4). Left HF is caused by left ventricular compensatory insufficiency and is the most common in clinical settings. The main manifestations of left HF are pulmonary circulatory venous congestion and low cardiac output. The symptoms of left HF include dyspnea, cough, expectoration, hemoptysis, fatigue, and renal impairment (5,6). Simple right HF is rare, and mainly in pulmonary heart disease and some congenital heart diseases. Clinical manifestations of right HF include systemic circulation venous congestion, gastrointestinal, liver congestion, dyspnea, and malignant vomiting (7,8).
With the development of imaging technology, there are more and more ways to clinically diagnose HF, including radionuclide, multi-spiral computerized tomography (CT), left ventricular angiography, echocardiography, and cardiac magnetic resonance imaging (MRI) (9). Among these, radionuclide obtains information about both cardiac function and myocardial perfusion, and has high reliability but low spatial resolution. The perfusion imaging defect area has a certain influence on the boundary of the heart (10,11). CT can obtain cardiac images in an anatomic direction, but its temporal resolution is low. Left ventricular angiography was previously considered the gold standard; however, it is invasive and radioactive (12). Ultrasound has been widely used in the field of cardiac detection, and has a number of advantages, including that it does not use radiation, has low costs, and is simple to operate; however, it also has low resolution and the accuracy of evaluations of cardiac structural changes in patients with HF is not reliable (13). With its high spatial and temporal resolution, cardiac MRI displays the cardiac anatomical structure, myocardial activity, and myocardial ischemia well. Its clinical use has attracted wide attention.
Compressed sensing refers to a signal reconstruction technology based on sparse signal characteristics that enable the signal sampling and compression process to proceed simultaneously, thus greatly reducing the sampling rate (14). Sun et al. [2019] (15) combined analysis-based blind compression sensing with other non-local low-rank constraints to obtain better MRI images with fewer measurements, and simulation results showed that the performance of the proposed method was superior to previous algorithms. Imaging speed has always been the main factor limiting the clinical use of magnetic resonance (16,17). As the down-sampling reconstruction method can be used to address the imaging speed issue, the use of the compressed sensing algorithm is of great research value (18).
Presently, ultrasound is still the mainstream method used to diagnose left ventricular function in patients with HF. To date, few studies have examined the use of cardiac MRI. Therefore, the research idea of this work is as follows. (I) A total of 66 patients with acute ischemic stroke were selected as the experimental group, and 20 patients with normal cardiac function were selected as the control group; (II) cardiac MRI scans were performed on the patients and evaluated by several senior physicians; (III) based on compressed sensing, an MRI image reconstruction algorithm was constructed to reconstruct and process the patients’ heart MRI images, and the traditional sensitivity encoding (SENSE) algorithm and orthogonal matching tracking (OMP) algorithm were introduced to conduct simulation experiments for comparison; (IV) the clinical data of the experimental group and the control group were compared; (V) left ventricular function parameters of patients were obtained, and the diagnostic efficacy of each parameter on HF was analyzed by receiver operating characteristic (ROC) curves. Left ventricular functional parameters include left ventricular ejection fraction (LVEF), endothelium-dependent vasodilation (EDV), ecosystem service value (ESV), peak filling rate (PFR), and filling volume (FV), which can be used to evaluate cardiac hypertrophy or atrophy, assess cardiac contractile and pumping function, cardiac diastolic function, and blood reflux, etc. A comprehensive analysis of these cardiac MRI indicators can provide a comprehensive assessment of the function and structure of the heart, providing important references and guidance for the diagnosis and treatment of heart disease. ROC curve has important applications in medical diagnosis. When the accuracy of medical examinations needs to be evaluated, ROC curve can help physicians to evaluate the accuracy of medical examinations and improve the credibility of medical examinations. ROC is an important tool that can be applied to model comparison, model adjustment, and medical diagnosis. In this study, it was assumed that the diagnostic sensitivity and specificity of MRI images based on compressed sensing algorithm were better than those of traditional detection methods. Therefore, patients with HF were selected as the experimental group, and those with normal cardiac function were selected as the control group. All the patients were subjected to cardiac MRI scanning, and the compressed sensing reconstruction algorithm was applied to process MRI images. The objective of this study was to investigate the diagnostic value of cardiac MRI based on compressive sensing imaging algorithm for HF by analyzing cardiac MRI image parameters. We present the following article in accordance with the STARD reporting checklist (available at https://jtd.amegroups.com/article/view/10.21037/jtd-23-190/rc).
Methods
Study objects
Patients diagnosed with HF in Wangjing Hospital of CACMS from December 1, 2019 to October 15, 2020 were selected as the experimental group, aged between 36 and 75 years old. Meanwhile, 20 patients with normal cardiac function who underwent physical examination in this hospital at the same period were selected as the control group. The study was conducted in accordance with the Declaration of Helsinki (as revised in 2013). The study was approved by ethics committee of Wangjing Hospital of CACMS (No. 20201578) and informed consent was taken from all the patients.
Inclusion criteria of experimental group: (I) patients having typical HF symptoms; (II) patients with no claustrophobia, and MRI examination was allowed; (III) New York Heart Association Functional Score ≥ level I; (IV) patients with normal left ventricular systolic function; (V) ultrasound results showed diastolic dysfunction (ie, e/e’ ratio ≥15).
Inclusion criteria of normal group: (I) the medical test results were normal, and there was no disease affecting the experimental results; (II) patients with LVEF >50%; (III) the ultrasound index E/e’ ratio was ≤8.
Exclusion criteria for all subjects: (I) patients suffering from conditions including coronary heart disease, atrial fibrillation, and dilated cardiomyopathy; (II) patients suffering from chronic obstructive pulmonary disease; (III) patients having symptoms of renal failure; (IV) patients with secondary hypertension and hyperlipidemia; (V) patients suffering from combined endocrine diseases.
Ultrasonic testing
The scan was performed with the Netherlands Philips high-end heart Department iE33 four-dimensional Doppler color ultrasonic diagnostic instrument. The patient was placed in the lateral decubitus position in the resting state. The probe was placed at the apex of the heart to obtain the standard 4-chamber view of the apex. The peak diastolic blood flow velocity E and peak diastolic blood flow velocity in the late diastolic period were measured A. Tissue doppler imaging was used to record the motion velocity of the septum, lateral wall, inferior wall, and anterior wall of the mitral annulus. The e’ values of the 4 regions were measured. The average value was obtained to calculate the E/e’.
MRI testing
Netherlands Philips Ingenia 3.0T full digital magnetic resonance system was employed. The patient was placed in the supine position and instructed to hold their breath, so that the heart was in a relatively fixed position during each scan, and the image collection was completed at the end of the breath holding. The scanning range was from the bottom of the heart to the apex of the heart, and multi-layer images were successively collected to obtain a cross-sectional black blood sequence, long axis 2, 3, and 4 chamber heart of the left ventricle, and a series of short axis movie sequences. The scanning parameters were as follows: excitation frequency: 1; layer thickness: 7 mm; time of repetition: 4.1 ms; time of echo: 1.5 ms, turn angle: 45 degrees; and field of vision: 256×256.
The obtained images were transferred to the workstation, and the left ventricular section from the apex to the bottom of the heart was the semi-automatic contour delineation of the endocardial membrane. The program automatically generated the time-volume curve of the left ventricle and collected the cardiac function parameters of the left ventricle, including LVEF, left ventricular end-diastolic volume (LVEDV), left ventricular end-systolic volume (LVESV), the early peak filling rate (PFR1), the late peak filling rate (PFR2), the early filling volume (FV1), and the late filling volume (FV2).
MRI algorithm based on compressed sensing
Because of the sparsity of the image in the sparse domain constructed on the sparse basis, the image could be reconstructed by solving a minimum optimization issue, which was written as follows:
where represents the Euclidean norm, represents the number of non-zero elements in the matrix, f represents the image, and represents the sparse basis.
Due to the influence of environment, noise, and measurement error, the signal acquired in the process of signal acquisition always had a certain error. Under the error condition, Eq. [1] was optimized as follows:
where θ represents the upper bound of error. We decided to transpose the objective function and constraint term in Eq. [2], which was illustrated as follows:
where Z represents a constant related to sparsity. By adding the constraint term to the above equation as a penalty term to the objective function, it was transformed into the following unconstrained optimization problem:
where represents the regularization term, α represents the regularization parameter, and represents the data fidelity term. Better reconstruction results can be obtained by balancing data fidelity terms and regularization terms. Thus, the objective function was defined as:
If p(f) was a convex function, Eq. [5] was a convex optimization problem. f* represents the estimated value of the image after solution. The gradient descent method was used to solve the minimum objective function. The gradient descent iteration equation was written as follows:
where C represents the iteration ordinal, fz represents the image data of the cth, represents the gradient value of the objective function at the point fz, and lc represents the step size of the cth iteration. The gradient descent method in Eq. [6] showed that the search direction of each iteration had a negative gradient direction, which was the direction with the fastest decline of function value. From the direction of the negative gradient, the optimal point was gradually approached until the minimum point of the objective function was found. In the solving process, the step size was obtained by backtracking the line search method. The process of the MRI imaging oral contraceptives (OCS) algorithm based on optimized compressed sensing is shown in Figure 1.
Image quality evaluation methods
Mean square error (MSE), peak signal to noise ratio (PSNR), and structural similarity (SSIM) were used as indexes to evaluate the quality of the reconstructed images (19). MSE was calculated as follows:
where p*q represents the original image size, E0 represents the image, and E1 represents the reconstructed image.
PSNR represents the relative size of the maximum possible signal power and the noise power represented by MSE, and was written as:
where B represents the number of image pixel gray value bits. SSIM represents image structure by combining brightness, contrast, and the attributed reflecting object structure in the image, and was expressed as:
where β0 and β1 represent the brightness of the E0 and E1 images, respectively, δ0 and δ1 represent the variance of the E0 and E1 images, respectively, and n0 and n1 represent the constant terms.
Observation indexes
The following information from patients was collected: age, gender, body mass index (BMI), heart rate (HR), body surface area (BSA), ratio (E/e’) of early diastolic blood flow velocity of mitral valve (E) to early diastolic peak velocity of mitral annulus (e’), time interval from onset of symptoms to follow-up MRI examination, medical history (related diseases such as heart disease, stroke, transient ischemic attack, atherosclerosis, etc.), National Institutes of Health Stroke Scale (NIHSS) score, and modified Rankin Scale (mRS) score. The cardiac MRI parameters and image quality indicators were collected from two groups of participants and compared.
Evaluation parameters
To rule out the influence caused by inconsistent data volumes of various types of images, MRI images were evaluated from three aspects of sensitivity, specificity, and the area under the curve (AUC). The calculations are shown in Equations 1 and 2. AUC is the area under the curve of subject curve (ROC), and ROC is a comprehensive index reflecting the relationship between sensitivity and specificity. The larger AUC indicated that the diagnosis was correct.
True positive (TP): the number of samples where the model predicts positive and the true value is positive.
False negative (FN): the number of samples where the model predicts negative but the true value is positive.
False positive (FP): the number of samples where the model predicts positive but the true value is negative.
True negative (TN): the number of samples where the model predicts negative and the true value is negative.
The AUC was calculated using the trapezoidal rule. The area of the trapezoid was calculated as (upper base + lower base) * height / 2, which is equal to (x2 − x1) * (y1 + y2) / 2.
Statistical methods
SPSS 19.0 statistical software was used for the data processing in this study. The mean ± standard deviation was used for the measurement data, and the percentage (%) was used for the counting data. Pairwise comparisons were performed by a one-way analysis of variance. The diagnostic effect of diffusion-weighted imaging, SWItch, 3D TOF MRA, and MR scale-PWI sequences on the ischemic penumbra was analyzed by ROC curves. The differences were considered statistically significant if the P values were <0.05.
Results
Comparison of the clinical data between the 2 groups
Figure 2 shows the results of comparisons of the clinical data between the 2 groups. There were no statistically significant differences in terms of the proportion of males, average age, BMI, bovine serum albumin level level, and E between the experimental group and the control group (P>0.05). The e’ and HR levels of the experimental group were significantly higher than those of the control group (P<0.05). The E/e’ ratio of the experimental group was significantly lower than that of the control group (P<0.05).
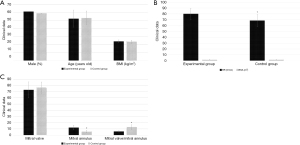
Comparison of cardiac MRI parameters between the 2 groups
Figure 3 shows the results of the comparison of the cardiac MRI parameters between the 2 groups. There were no statistically significant differences in LVEF, EDV, ESV, and FV between the experimental group and the control group (P>0.05). The PFR1, PFR1/PFR2, FV1, and FV1/FV of the experimental group were significantly higher than those of the control group (P<0.05). The PFR2 and FV2 of the experimental group were significantly lower than those of the control group (P<0.05).
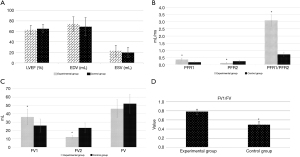
Analysis of cardiac MRI images
Figure 4 shows the MRI images of the heart of a 65-year-old man with symptoms of progressive HF, who later underwent an endocardial biopsy that revealed thyroxin-carrying protein amyloidosis. Figure 5 shows MRI images of the heart of a 57-year-old man with progressive exertive dyspnea and lower limb edema.
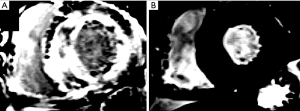
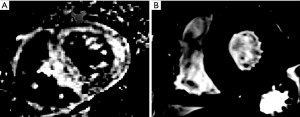
Diagnostic performance of MRI parameters in left ventricular function
Figure 6 shows the diagnostic performance of the MRI parameters on left ventricular function. The diagnostic indexes of the PFR2 and FV2 were relatively high. The diagnostic sensitivity, specificity, and AUC for the concentration-time of the PFR2 were 0.891, 0.788, and 0.904, respectively. The diagnostic sensitivity, specificity, and AUC for the concentration-time of the FV2 were 0.902, 0.878, and 0.925, respectively. The diagnostic indexes of the other parameters (i.e., the PFR1, PFR1/PFR2, FV1, and FV1/FV) were all low.
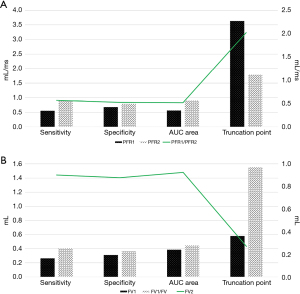
Quality evaluation of image reconstruction algorithm
In this study, the traditional SENSE algorithm (20) and OMP algorithm (21) were used to analyze the OCS algorithm. SENSE sensitivity coding reconstruction is a parallel imaging reconstruction technology based on image domain. Its core is recovering the complete image without artifact from the image with artifact acquired by multiple coils from the under-sampled image with artifact, and realizing the reconstruction based on artifact image by using sensitivity coding. OMP is one of the classical algorithms in the field of Compressed Sensing, which is the basis of many commonly used efficient algorithms and has the characteristics of simple and efficient. As the results in Figure 7 show, the MSE of the images reconstructed using the OCS algorithm was significantly smaller than those reconstructed using the SENSE algorithm and the OMP algorithm (P<0.05). The PSNR and SSIM of the images reconstructed using the OCS algorithm were significantly higher than those reconstructed using the SENSE algorithm and OMP algorithm (P<0.05). Figure 8 shows the MRI images reconstructed by each algorithm. The MRI image reconstructed using the OCS algorithm had better display clarity and better overall quality than those reconstructed using the SENSE algorithm and OMP algorithm.
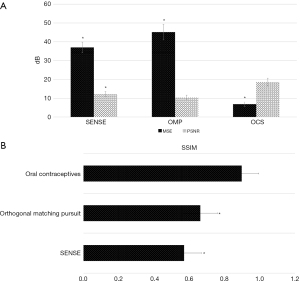
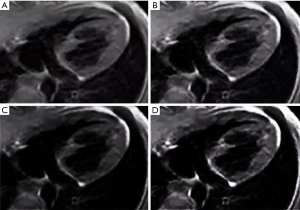
Discussion
Preserved LVEF is defined as a symptom and sign of HF. A syndrome with roughly normal LVEF and diastolic dysfunction. It is characterized by high morbidity and high mortality, and it is difficult to treat (22). Cardiac magnetic resonance is a unique technique that can measure ventricular volume and function with high accuracy and good repetition and is considered the gold standard for quantifying cardiac function (23).
In this study, 66 patients with acute ischemic stroke were selected for the experimental group and 20 patients with normal cardiac function who underwent physical examinations during the same period were selected for the control group. All of the patients underwent cardiac MRI scanning. An MRI image reconstruction algorithm based on compressed sensing was developed and used in the cardiac MRI image processing. First, the clinical data of the 2 groups of patients were compared, and we found that the e’ and HR levels of the experimental group were significantly higher than those of the control group, and the E/e’ ratio was significantly lower than that of the control group (P<0.05). This is similar to the research results of Lundorff et al. [2021] (24), which indicated that the diastolic blood flow and HR of the left atrioventricular valve orificium in patients with HF in the experimental group differed to those in the normal group. The PFR1, PFR1/PFR2, FV1, and FV1/FV of the experimental group were significantly higher than those of the control group. Consistent with the findings of previous study (25), the PFR2 and FV2 of the experimental group were significantly lower than those of the control group (P<0.05), which indicates that the left ventricular diastolic function of patients might have been impaired.
Ventricular diastolic dysfunction often precedes systolic dysfunction. Thus, even in asymptomatic patients, the evaluation of diastolic function is of great significance for patient prognosis and treatment strategies (26). There were no significant differences in the LVEF, EDV, ESV, and FV between the experimental group and the control group (P>0.05), indicating that there were no differences in left ventricular systolic function between the patients with HF and the normal controls.
The diagnostic performance of each MRI parameter on left ventricular function was calculated, and the diagnostic indexes of the PFR2 and FV2 were high. The diagnostic sensitivity, specificity, and AUC for the concentration-time of the of the PFR2 were 0.891, 0.788, and 0.904, respectively. The diagnostic sensitivity, specificity, and AUC for the concentration-time of the of the FV2 were 0.902, 0.878, and 0.925, respectively. The cardiac MRI image parameters PFR2 and FV2 have been shown to be effective indicators in evaluating HF (27).
The traditional SENSE algorithm and OMP algorithm were compared to the OCS algorithm. The MSE of the images reconstructed using the OCS algorithm was significantly lower than those reconstructed using the SENSE algorithm and OMP algorithm. The PSNR and SSIM of the images reconstructed using the OCS algorithm were significantly higher than those reconstructed using the SENSE algorithm and OMP algorithm (P<0.05). Cardiac MRI image reconstruction using the OCS algorithm had the best effect, and thus may be used to assist doctors to make objective evaluations (28). The MRI image structure reconstructed using the OCS algorithm was clearer than those reconstructed using the SENSE algorithm and OMP algorithm, and the overall quality was better, which was consistent with the results of the quantitative data. This study still has several limitations. First, some clinical data on our patient, including hypertension, hyperlipidemia, and smoking history, were not collected. These data could have been used to assess the risk of cardiovascular disease. Second, since traditional invasive hemodynamics was not practical, ultrasonic diastolic function indices were used for grouping rather than invasive hemodynamic data. In the future, the experimental method will be further improved and a deeper exploration will be carried out in the direction of this subject.
Conclusions
In this study, 66 patients with acute ischemic stroke were selected for the experimental group, and 20 patients with normal cardiac function were selected for the control group. All the patients underwent cardiac MRI scanning. An MRI image reconstruction algorithm based on compressed sensing was then developed and used in the cardiac MRI image processing. The imaging algorithm based on compressed sensing had excellent processing effect on cardiac MRI and improves the image quality. Cardiac MRI imaging had good diagnostic performance for HF and had the value of clinical popularization.
The findings of this study provide theoretical support for the use of MRI imaging technology in evaluating HF.
Acknowledgments
Funding: None.
Footnote
Reporting Checklist: The authors have completed the STARD reporting checklist. Available at https://jtd.amegroups.com/article/view/10.21037/jtd-23-190/rc
Data Sharing Statement: Available at https://jtd.amegroups.com/article/view/10.21037/jtd-23-190/dss
Peer Review File: Available at https://jtd.amegroups.com/article/view/10.21037/jtd-23-190/prf
Conflicts of Interest: All authors have completed the ICMJE uniform disclosure form (available at https://jtd.amegroups.com/article/view/10.21037/jtd-23-190/coif). The authors have no conflicts of interest to declare.
Ethical Statement: The authors are accountable for all aspects of the work in ensuring that questions related to the accuracy or integrity of any part of the work are appropriately investigated and resolved. The study was conducted in accordance with the Declaration of Helsinki (as revised in 2013). The study was approved by ethics committee of Wangjing Hospital of CACMS (No.20201578) and informed consent was taken from all the patients.
Open Access Statement: This is an Open Access article distributed in accordance with the Creative Commons Attribution-NonCommercial-NoDerivs 4.0 International License (CC BY-NC-ND 4.0), which permits the non-commercial replication and distribution of the article with the strict proviso that no changes or edits are made and the original work is properly cited (including links to both the formal publication through the relevant DOI and the license). See: https://creativecommons.org/licenses/by-nc-nd/4.0/.
References
- Santos É, Menezes Falcão L. Chagas cardiomyopathy and heart failure: From epidemiology to treatment. Rev Port Cardiol 2020;39:279-89. (Engl Ed). [Crossref] [PubMed]
- Farooqi KM, Cooper C, Chelliah A, et al. 3D Printing and Heart Failure: The Present and the Future. JACC Heart Fail 2019;7:132-42. [Crossref] [PubMed]
- Huber AT, Razakamanantsoa L, Lamy J, et al. Multiparametric Differentiation of Idiopathic Dilated Cardiomyopathy With and Without Congestive Heart Failure by Means of Cardiac and Hepatic T1-Weighted MRI Mapping. AJR Am J Roentgenol 2020;215:79-86. [Crossref] [PubMed]
- Pibarot P, Messika-Zeitoun D, Ben-Yehuda O, et al. Moderate Aortic Stenosis and Heart Failure With Reduced Ejection Fraction: Can Imaging Guide Us to Therapy? JACC Cardiovasc Imaging 2019;12:172-84. [Crossref] [PubMed]
- Kanagala P, Arnold JR, Singh A, et al. Characterizing heart failure with preserved and reduced ejection fraction: An imaging and plasma biomarker approach. PLoS One 2020;15:e0232280. [Crossref] [PubMed]
- Bhardwaj H, Tomar P, Sakalle A, et al. EEG-Based Personality Prediction Using Fast Fourier Transform and DeepLSTM Model. Computational Intelligence and Neuroscience 2021;2021:1-10. [Crossref] [PubMed]
- Kammerlander AA, Donà C, Nitsche C, et al. Feature Tracking of Global Longitudinal Strain by Using Cardiovascular MRI Improves Risk Stratification in Heart Failure with Preserved Ejection Fraction. Radiology 2020;296:290-8. [Crossref] [PubMed]
- Kumar A, Sinha N, Bhardwaj A, et al. Clinical risk assessment of chronic kidney disease patients using genetic programming. Comput Methods Biomech Biomed Engin 2022;25:887-95. [Crossref] [PubMed]
- Wongchai A, Jenjeti DR, Priyadarsini AI, et al. Farm monitoring and disease prediction by classification based on deep learning architectures in sustainable agriculture. Ecological Modelling 2022;474:110167. [Crossref]
- Marra AM, Benjamin N, Cittadini A, et al. When Pulmonary Hyper-tension Complicates Heart Failure. Heart Fail Clin 2020;16:53-60. [Crossref] [PubMed]
- Aung N, Vargas JD, Yang C, et al. Genome-Wide Analysis of Left Ventricular Image-Derived Phenotypes Identifies Fourteen Loci Associated With Cardiac Morphogenesis and Heart Failure Development. Circulation 2019;140:1318-30. [Crossref] [PubMed]
- van Woerden G, Gorter TM, Westenbrink BD, et al. Epicardial fat in heart failure patients with mid-range and preserved ejection fraction. Eur J Heart Fail 2018;20:1559-66. [Crossref] [PubMed]
- Elming MB, Hammer-Hansen S, Voges I, et al. Myocardial fibrosis and the effect of primary prophylactic defibrillator implantation in patients with non-ischemic systolic heart failure-DANISH-MRI. Am Heart J 2020;221:165-76. [Crossref] [PubMed]
- Carlsson M, Ugander M, Kanski M, et al. Heart fill-ing exceeds emptying during late ventricular systole in patients with systolic heart failure and healthy subjects - a cardiac MRI study. Clin Physiol Funct Imaging 2019;39:192-200. [Crossref] [PubMed]
- Sun M, Tao J, Ye Z, et al. An Algorithm Combining Analysis-based Blind Compressed Sensing and Nonlocal Low-rank Constraints for MRI Reconstruction. Curr Med Imaging Rev 2019;15:281-91. [Crossref] [PubMed]
- Aljizeeri A, Sulaiman A, Alhulaimi N, et al. Cardiac magnetic resonance imaging in heart failure: where the alphabet begins! Heart Fail Rev 2017;22:385-99. [Crossref] [PubMed]
- Yang SJ, Zhao SH. State of the art for value of cardiac MRI in the assessment of heart failure with preserved ejection fraction. Zhonghua Xin Xue Guan Bing Za Zhi 2022;50:717-22. [PubMed]
- Carerj S, Zito C, Di Bella G, et al. Heart failure diagnosis: the role of echocardiography and magnetic resonance imaging. Front Biosci (Landmark Ed) 2009;14:2688-703. [Crossref] [PubMed]
- Xin JF, Zhang LY, Liu XF, et al. A retrospective study on the clinical significance of cardiac computed tomography in heart failure patients with preserved ejection fraction. Ann Transl Med 2022;10:1319. [Crossref] [PubMed]
- Ohta Y, Kishimoto J, Kitao S, et al. Investigation of myocardial extracellular volume fraction in heart fail-ure patients using iodine map with rapid-kV switching dual-energy CT: Segmental com-parison with MRI T1 mapping. J Cardiovasc Comput Tomogr 2020;14:349-55. [Crossref] [PubMed]
- Baessler B, Luecke C, Lurz J, et al. Cardiac MRI and Texture Analysis of Myocardial T1 and T2 Maps in Myocarditis with Acute versus Chronic Symp-toms of Heart Failure. Radiology 2019;292:608-17. [Crossref] [PubMed]
- Vega-Adauy J, Tok OO, Celik A, et al. Comprehensive Assess-ment of Heart Failure with Preserved Ejection Fraction Using Cardiac MRI. Heart Fail Clin 2021;17:447-62. [Crossref] [PubMed]
- Chamsi-Pasha MA, Zhan Y, Debs D, et al. CMR in the Evaluation of Diastolic Dysfunction and Phenotyping of HFpEF: Current Role and Future Perspectives. JACC Cardiovasc Imaging 2020;13:283-96. [Crossref] [PubMed]
- Lundorff I, Modin D, Mogelvang R, et al. Echocardiographic predictors of cardiovascular morbidity and mortality in women from the general population. Eur Heart J Cardiovasc Imaging 2021;22:1026-34. [Crossref] [PubMed]
- Schmeißer A, Rauwolf T, Groscheck T, et al. Predictors and prognosis of right ventricular function in pulmonary hypertension due to heart failure with reduced ejection fraction. ESC Heart Fail 2021;8:2968-81. [Crossref] [PubMed]
- Malek H, Samiei N, Yaghoobi N, et al. Assessment of LV diastolic dysfunction in myocardial perfusion imaging: a correlative study with transthoracic echocardiography. Nucl Med Commun 2021;42:979-83. [Crossref] [PubMed]
- Aziz W, Claridge S, Ntalas I, et al. Emerging role of cardiac com-puted tomography in heart failure. ESC Heart Fail 2019;6:909-20. [Crossref] [PubMed]
- Ohta Y, Kitao S, Yunaga H, et al. Myocardial Delayed Enhancement CT for the Evaluation of Heart Failure: Comparison to MRI. Radiology 2018;288:682-91. [Crossref] [PubMed]
(English Language Editor: L. Huleatt)