Exploration of the shared genes and signaling pathways between lung adenocarcinoma and idiopathic pulmonary fibrosis
Highlight box
Key findings
• Extracellular matrix–related mechanisms may be the underlying link between lung cancer and idiopathic pulmonary fibrosis (IPF). Seven shared genes were identified as potential diagnostic markers and therapeutic targets for lung adenocarcinoma (LUAD) and IPF.
What is known and what is new?
• IPF-activated fibroblasts could be a source of carcinoma-associated fibroblasts in IPF–lung cancer. Importantly, the production of stromal cell proteins by activated fibroblasts in the lungs of patients with IPF may cause lung cancer.
• The pathogenesis driving these malignant transformations remains unclear, so this study aimed to identify shared genes and functional pathways associated with both disease conditions.
What are the implications, and what should change now?
• This study facilitates further insight into the interplay between LUAD and IPF. However, gaining a greater understanding of the specific underlying mechanisms and 7 shared genes requires further investigation.
Introduction
Idiopathic pulmonary fibrosis (IPF) is a chronic, progressive, fibrotic interstitial lung disease (ILD) of unknown cause (1). The median survival time of patients with IPF after diagnosis is 3–5 years (2). One of the main features of IPF is the overdeposition of extracellular matrix (ECM) proteins by activated lung fibroblasts and myofibroblasts, which reduces gaseous exchange and eventually leads to respiratory failure (3-5). An epidemiological research study reported incidence and prevalence rates for IPF of 0.09 and 1.30 per 10,000 individuals, respectively (6). In addition to acute exacerbation (AE) and chronic respiratory failure caused by further progression of disease in patients with IPF, the occurrence of lung cancer (LC) is also one of the main causes of death in these patients (7). Studies have reported that the majority of tumors are typically found in the lower lobe and lung periphery, and 70% of cancers are observed in the fibrotic area of the chest (8). Furthermore, research suggests that activated mesenchymal cells play a vital role in cancer and fibrosis (9). Other studies also indicate that the pathophysiology of IPF is linked to an increased malignancy of adenocarcinoma in patients with IPF. However, much information about the underlying processes remains unknown.
Clinically, individuals with LC-IPF have few options for therapy. Pirfenidone and nintedanib are 2 medications currently approved to treat people with this condition (10,11). Therefore, understanding the connection between IPF and lung adenocarcinoma (LUAD) is vital for the discovery of novel biomarkers and targeted therapies for treatment.
Advancements in gene chip technology have made it possible to assess the expression of hundreds of genes in various illnesses, thereby allowing for a more in-depth exploration of disease pathogenesis and the discovery of novel biomarkers. To the best of our knowledge, this study is the first attempt to identify gene modules of shared genes in LUAD and IPF using weighted gene coexpression network analysis (WGCNA). Gene enrichment analysis was also used to investigate the common mechanism in LUAD and IPF. We identified the common differential genes and coexpression modules in LUAD and IPF using gene expression data from The Cancer Genome Atlas (TCGA) and Gene Expression Omnibus (GEO). The association between acquired modules and differentially expressed genes (DEGs) was then investigated, and the shared genes of LUAD and IPF were identified. Finally, diagnostic biomarkers were obtained using receiver operating characteristic (ROC) curve analysis. The study aimed to further clarify the molecular mechanism of LUAD–IPF and may contribute to the efficient diagnosis and treatment of LUAD–IPF. We present this article in accordance with the STARD reporting checklist (available at https://jtd.amegroups.com/article/view/10.21037/jtd-22-1522/rc).
Methods
Datasets from TCGA and GEO Databases
The study was conducted in accordance with the Declaration of Helsinki (as revised in 2013). LUAD-related data were downloaded from TCGA and GEO databases. A total of 59 normal and 535 tumor samples were used for TCGA-LUAD transcriptomic data, whereas 58 normal and 58 tumor samples were used in the GSE32863 dataset cohort. The IPF gene expression data were obtained from the GEO database. The IPF dataset GSE10667 includes 31 samples from patients with IPF and 15 healthy control samples, whereas the IPF dataset GSE24206 includes 17 IPF samples and 6 healthy control samples.
Weighted gene coexpression network analysis
The WGCNA is a tool for identifying prospective biomarkers and therapeutic targets using network-based gene screening approaches (12). With the R package (The R Foundation for Statistical Computing, Vienna, Austria), WGCNA was used to identify strongly correlated gene modules, and the gene expression data profiles of GSE32863 and GSE24206 were created as weighted gene coexpression modules (13). The adjacency matrix was then created, which was then transformed into the topological overlap matrix (TOM) and the corresponding dissimilarity matrix (1-TOM). Afterward, a cluster formation treemap was generated to divide similarly expressed genes into multiple gene coexpression modules. The link between module eigengene (ME) and clinical features was assessed using the Spearman test. In this study, the soft threshold in the WGCNA analysis of LUAD and IPF was 11 and 6, respectively.
Validation of shared genes through DEG analysis
Differential gene expression analysis was performed on the LUAD and IPF datasets (TCGA-LUAD and GSE10667). The DEGs were assessed with the R package “limma”, a library for the analysis of gene expression microarray data. The cutoff value was absolute log2 fold change ≥1 and a P value <0.05. The R package “pheatmap” was used to plot the heat map while the package “ggplot2” was used to draw volcano charts. To generate a heat map, the top 20 upregulated and the top 20 downregulated DEGs were ranked using adjusted values. A Venn diagram of the overlapping DEGs in LUAD and IPF was generated using the R package “VennDiagram”.
Function enrichment analysis
Gene Ontology (GO) analysis includes biological processes (BP), cellular components (CC), and molecular functions (MF). The R packages “clusterProfiler” and “org.Hs.eg.db” were used, and the thresholds of adjusted P<0.05 and q value <1 were considered to indicate statistical significance. Metascape (https://metascape.org), a web-based portal designed to provide a comprehensive GO analysis of annotated regions, was used. In terms of design functions, Metascape combines functional enrichment, gene annotation, interactome analysis, and member search (14). The pathway and process enrichment analysis for the gene list was performed using the ontology sources Kyoto Encyclopedia of Genes and Genomes (KEGG) pathway, Canonical Pathways, and WikiPathways.
Protein-protein interaction (PPI) network construction
PPI networks were constructed using the biological database Search Tool for the Retrieval of Interacting Genes (STRING; http://string-db.org). The confidence score threshold was kept at 0.7.
ROC curve analysis
The R package “pROC” was used for ROC curve analysis, which was carried out to determine the sensitivity and specificity of the risk score.
Drug sensitivity
RNA-sequencing (RNA-seq) expression profiles and the US National Cancer Institute 60 human tumor cell line anticancer drug screen (NCI-60) compound activity data were retrieved from the tool CellMiner (https://discover.nci.nih.gov/cellminer/home.do). For further analysis, only the drugs approved by the US Food and Drug Administration (FDA) were used. The effect of the genes on drug sensitivity was analyzed using the R packages “impute”, “limma”, “ggplot2”, and “ggpubr”. Drugs with a P<0.05 were considered to be significantly correlated with shared genes. A correlation coefficient greater than 0.7 indicated a positive correlation of the shared gene with drug sensitivity.
Statistical analysis
The data were processed using the Perl programming language (version 5.32.0, The Perl Foundation, Walnut, CA, USA; http://www.perl.org). All statistical analyses were performed using R software (version 4.1.2; https://www.r-project.org/). A P value <0.05 was considered statistically significant.
Results
Discovery cohort: construction of weighted coexpression networks
The coexpression network of both the GSE32863 and GSE24206 datasets had 7 similar modules (available online https://cdn.amegroups.cn/static/public/jtd-22-1522-1.xlsx). Significant correlations (P<0.05) were found in the LUAD-GSE32863 dataset among the black module (P=6e−05), blue module (P=1e−11), yellow module (P=2e−24), brown module (P=9e–71), red module (P=9e–09), and gray module (P=3e−39). LUAD was negatively correlated with genes in the brown and red modules, whereas it was positively correlated with genes in the black, blue, yellow, and gray modules (Figure 1A,1B). A significant correlation was observed for the IPF-GSE24206 dataset for the black module (P=0.01), blue module (P=2e–07), green module (P=0.03), and pink module (P=0.001) (P<0.05). Genetic modules in black and blue had negative correlations with IPF, whereas those in green and pink were positively correlated. From the WGCNA modules of IPF, the blue module and the green-yellow module were selected as the most important modules (Figure 1C,1D). Furthermore, the gray module related to LUAD and the pink and green modules related to IPF were selected as the essential critical modules. In addition, the gray LUAD module and the pink and green IPF modules were used to obtain the overlapping genes. A total of 148 genes were found to be overlapping in the LUAD and IPF-related modules, which were referred to as gene set 1 (GS1) (Figure 2 and available online https://cdn.amegroups.cn/static/public/jtd-22-1522-1.xlsx).
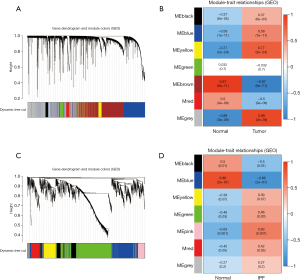
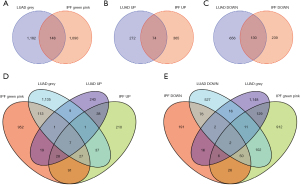
Validated cohort: identification of DEGs
Differential gene analysis was performed using the TCGA-LUAD and GSE10667 datasets to validate our results further. A total of 1132 DEGs were discovered in TCGA-LUAD, with 346 upregulated genes and 786 downregulated genes (Figure 3A,3B and available online https://cdn.amegroups.cn/static/public/jtd-22-1522-1.xlsx). The GSE10667 dataset included 808 DEGs, with 439 upregulated genes and 369 downregulated genes (Figure 3C,3D and available online https://cdn.amegroups.cn/static/public/jtd-22-1522-1.xlsx). Furthermore, the intersection of upregulated genes obtained by TCGA-LUAD (n=346) and GSE10667 (n=439), and downregulated genes obtained by TCGA-LUAD (n=786) and GSE10667 (n=369) was determined. Finally, we obtained 74 upregulated and 130 downregulated overlapping genes, defined as gene set 2 (GS2) (Figure 2B,2C and available online https://cdn.amegroups.cn/static/public/jtd-22-1522-1.xlsx). To further explore the genes related to both diseases, 74 upregulated and 130 downregulated genes in GS2 were crossed with 148 genes in GS1, respectively, and 9 shared genes were obtained, including 7 upregulated shared genes and 2 downregulated shared genes (Figure 2D,2E and available online https://cdn.amegroups.cn/static/public/jtd-22-1522-1.xlsx).
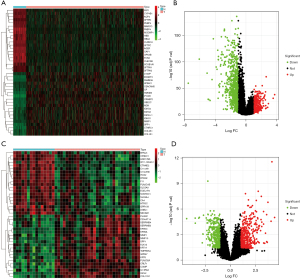
Enrichment analysis of coexpressed genes
We used R packages to analyze the GO enrichment of GS1 and GS2 to explore their functions (available online https://cdn.amegroups.cn/static/public/jtd-22-1522-1.xlsx). “Extracellular matrix organization”, “Extracellular structure organization”, and “External encapsulating structure organization” were the top 3 significantly enriched GO terms in GS1 and GS2 (Figure 4A,4B). https://cdn.amegroups.cn/static/public/jtd-22-1522-1.xlsx show the enrichment analysis results of GS1 and GS2 output by Metascape. The results of functional enrichment analysis showed that GS1 was most closely correlated with “NABA CORE MATRISOME”, and “NABA MATRISOME-ASSOCIATED” gene sets, while GS2 was most closely associated with “NABA CORE MATRISOME” and “NABA SECRETED FACTORS” (Figure 5A,5B). In addition, we also performed functional enrichment analysis on 9 shared genes and found that the 9 shared genes were associated with “NABA CORE MATRISOME” and “NABA SECRETED FACTORS” (Figure 5C and available online https://cdn.amegroups.cn/static/public/jtd-22-1522-1.xlsx). “NABA CORE MATRISOME” was the most significant pathway. The results showed that these GO terms and “NABA CORE MATRISOME” pathway may be essential both in IPF and LUAD.
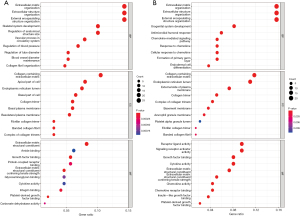
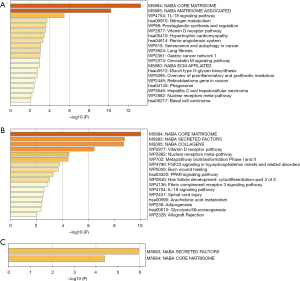
PPI network construction
An interaction network between proteins encoded by the GS1 and GS2 datasets was constructed using the web-based tool STRING and visualized with Cytoscape (https://cytoscape.org/; available online https://cdn.amegroups.cn/static/public/jtd-22-1522-1.xlsx). MCODE plugin was used to identify gene cluster modules. A total of 71 nodes and 125 edges were identified in the GS1 network while 53 nodes and 52 edges were identified in the GS2 network (Figure 6A,6B).
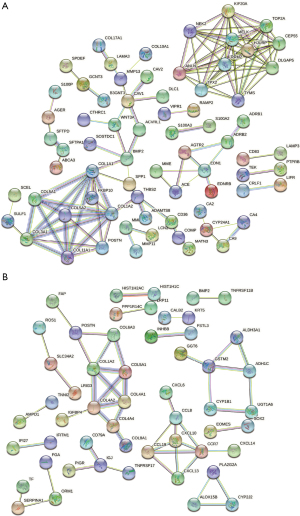
Evaluation of the diagnostic value of shared genes
The ROC curves of the LUAD-TCGA and GSE10667 data sets were drawn to evaluate the diagnostic value of shared genes by computing the area under the curve (AUC) values to test if they could differentiate diseased samples from normal samples. The AUC values for COL1A2, COL5A1, CRLF1, CXCL13, CXCL14, CYP24A1, POSTN, BMP2, and SLCO4C1 were 0.787, 0.868, 0.648, 0.857, 0.760, 0.886, 0.832, 0.799, and 0.858, respectively, in the LUAD-TCGA dataset (Figure 7A-7I). The AUC values for COL1A2, COL5A1, CRLF1, CXCL13, CXCL14, CYP24A1, POSTN, BMP2, and SLCO4C1 were 0.880, 0.837, 0.951, 0.770, 0.882, 0.884, 0.897, 0.766, and 0.652, respectively, in the GSE10667 dataset (Figure 8A-8I). The ROC analysis showed that only the AUC of CRLF1 was less than 0.7 in the TCGA-LUAD dataset, and the AUC of other genes was greater than 0.7. In addition, the ROC analysis showed that only the AUC of SLCO4C1 was less than 0.7 in the GSE10667 dataset, and the AUC of other genes was greater than 0.7. Finally, the results showed that AUC values of the 7 shared genes were >0.7, including COL1A2, COL5A1, CXCL13, CXCL14, CYP24A1, POSTN, and BMP2, indicating that these genes had a high diagnostic value for LUAD and IPF.
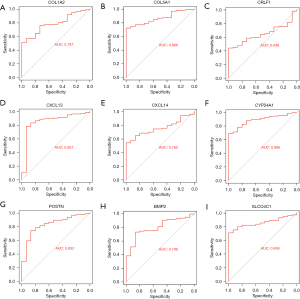
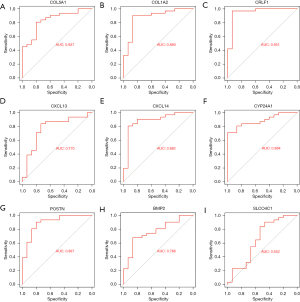
Drug sensitivity analysis
We investigated the potential correlation between drug sensitivity and the expression of 7 genes using the CellMiner database. The top 16 drugs with the most significant statistical differences were compiled: POSTN expression was positively correlated with the sensitivity of zoledronate; CXCL14 expression was negatively correlated with 6-mecraptopurine sensitivity; COL1A2 expression was positively correlated with zoledronate and abiraterone sensitivity but negatively correlated with allopurinol and CUDC by-product sensitivity; COL5A1 expression was positively correlated with rapamycin, abiraterone, lenvatinib, midostaurin, everolimus, and staurosporine sensitivity but negatively correlated with CUDC by-product sensitivity; CXCL13 expression was positively correlated with elesclomol sensitivity; BMP2 expression was positively correlated with rebimastat sensitivity; and CYP24A1 expression was negatively associated with vincristine sensitivity (Figure S1 and available online https://cdn.amegroups.cn/static/public/jtd-22-1522-1.xlsx).
Discussion
Lung cancer is one of the most common complications in patients with IPF and is associated with the highest mortality rates (15). The prognosis of patients with LC and IPF is even worse than that of patients without IPF (16). More research is needed to elucidate the potential mechanisms related to the malignant transition of IPF to LC, which would promote a better understanding of lung disease transformation mechanisms and provide drug targets for treatment. In this study, using WGCNA, we identified the gray module related to LUAD and the pink and green modules related to IPF as the most relevant modules. There are nine overlapped genes in GS1 and GS2. This study was the first to identify the shared genes and common mechanisms of LUAD and IPF using WGCNA. WGCNA and differential expression analysis was carried out to examine 9 common genes from 4 datasets to evaluate the relationship between LUAD and IPF. Finally, ROC curve analysis revealed that 7 genes, including COL1A2, COL5A1, POSTN, CXCL13, CXCL14, CYP24A1, and BMP2 (AUC >0.7), had good diagnostic value.
“Extracellular matrix organization”, “extracellular structure organization”, and “external encapsulating structure organization” were the top 3 significantly enriched GO terms in GS1 and GS2. Moreover, the Metascape database was used to perform functional enrichment analysis to investigate the genes associated with these pathways. The results of the analysis showed that genes of the GS1 dataset most closely correlated with genes of “NABA CORE MATRISOME” and “NABA MATRISOME-ASSOCIATED”, whereas the genes of the GS2 dataset are most closely associated with that of “NABA CORE MATRISOME” and “NABA SECRETED FACTORS”. Functional enrichment analysis results show that 9 shared genes are associated with “NABA CORE MATRISOME” and “NABA SECRETED FACTORS”. These results suggest that “NABA CORE MATRISOME” may have a significant impact on gene function enrichment analysis, that these GO terms and this pathway may be important in LUAD and IPF, and finally, that the ECM-related process could be the common pathogenic mechanism linking both disease conditions.
Periostin (POSTN) is an ECM protein that affects cell adhesion, proliferation, migration, and tissue angiogenesis by binding with integrin (17). When combined with chemokines, POSTN can attract neutrophils and macrophages, promoting the establishment of IPF (18). In addition, high expression of POSTN was found in many cancers, including in non-small cell lung cancer (NSCLC), as compared with normal tissues. POSTN may also interact directly with other ECM proteins including fibronectin and tenascin C to create a favorable metastatic niche for tumor formation (19). Interestingly, POSTN secreted by IPF fibroblasts can promote the proliferation of NSCLC cells. In addition, inhibiting the interaction of the POSTN receptor weakens the invasiveness of IPF to NSCLC (20). Therefore, it can be concluded that POSTN provides a potential therapeutic target for patients with NSCLC and IPF. In this study, 2 collagen family members including collagen type I alpha 2 chain (COL1A2) and collagen type V alpha 2 chain (COL5A2) were found to be upregulated. Airway ECM is mainly composed of 28 different collagen subtypes (21). The products of the COL1A1 and COL1A2 genes constitute type I collagen. Homeostatic type I collagen plays an important role in the pathogenesis of IPF. Although collagen V is a minor component of ECM, its absence can result in a condition called fibrotic stroma. The increase of collagen deposition in the alveolar wall leads to continuous destruction of the alveolar structure and increases the stiffness of ECM (22). Fibroblasts and cancer cells both produce type I collagen. In LC cell lines, type I collagen can also induce epithelial–mesenchymal transition. In NSCLC and esophageal squamous cell carcinoma (ESCC), COL1A2 is highly expressed. Studies have found that COL5A2 is upregulated in pulmonary neuroendocrine tumors (PNETs) (23). Cytokine receptor-like factor 1 (CRLF1) is a member of the interleukin-6 (IL-6) cytokine family (24). In IPF, CRLF1 enhances T-helper 1 and T-regulatory cells in the lung, increases inflammatory response, and reduces pulmonary fibrosis (25). By stimulating the MAPK/ERK and PI3K/AKT pathways, CRLF1 enhances the malignant phenotype of papillary thyroid cancer (26). However, a low expression of CRLF1 has been observed in those with colorectal cancer. Furthermore, CRLF1 inhibits tumorigenesis and metastasis, whereas miR-3065-3p promotes stemness and metastasis by targeting CRLF1 in colorectal cancer (27). Nevertheless, CRLF1 has not been reported in LC. Cytochrome P450 family 24, subfamily A, member 1 (CYP24A1) plays a key role in vitamin D catabolism and calcium homeostasis. Compared with normal lung tissue, CYP24A1 is highly expressed in LUAD (28). High CYP24A1 expression enhances LC cell growth and migration and is associated with low survival rates in patients with LC (29). Chloride channels have a crucial function in the transition of fibroblasts to myofibroblasts, with myofibroblasts gaining the ability to migrate and secrete ECM proteins. Studies have shown that bone morphogenetic protein 2 (BMP2) inhibits chloride channel activity (30-32). Through signaling, BMP2 stimulation of lung fibroblasts affects the expression of genes in the BMP pathway and BMP target genes. The activation of POSTN is particularly intriguing, as it suggests that lung fibroblasts have a gene expression profile similar to that of carcinoma-associated fibroblasts (CAFs) (33). BMP2 research is conflicting and complicated. Studies have reported that BMP2 is downregulated in LUAD relative to normal tissues (34,35).
Pulmonary fibrosis and LC are linked through genetic, molecular, and cellular processes, such as myofibroblast/interstitial transformation and myofibroblast proliferation. After being stimulated by IPF, fibroblasts release transforming growth factor-β (TGF-β) and cytokines to promote their proliferation. Furthermore, several cytokines play a role in the development of NSCLC (16,36). The Wnt/-catenin pathway and the TGF-a pathway are both active in IPF-activated fibroblasts and have a role in NSCLC development (37,38). IPF produces excess myofibroblasts, and these activated myofibroblasts secrete excessive ECM (39). Research suggests that fibroblasts and/or myofibroblasts are important regulators of migration and proliferation of malignant epithelial cells in tumours (9). Similar to IPF, CAFs are also involved in lung carcinogenesis. Both myofibroblasts and CAF exhibit mesenchymal-like features and have a heterogeneous phenotype (40). Both tumor cells and stromal cells can generate the proteins that constitute the tumor ECM (41). The tumor microenvironment consists of tumor cells, stromal cells, ECM, and immune cells (42). Tumorigenesis and metastasis are influenced by the intricate interactions between tumor cells, stromal cells, ECM, and other components of the tumor microenvironment (43). The ECM acts as a reservoir for growth factors and cytokines, ECM-remodeling enzymes, and essential ECM components (fibronectins, collagen, laminins, proteoglycans, etc.). The progression of the tumor will be influenced by changes in the ECM structure and biophysical characteristics. Excessive ECM deposition is a characteristic of malignancies with a bad prognosis. PF-activated fibroblasts have been discovered to release POSTN, promoting the growth of LC cells. Furthermore, POSTN, which is produced by CAFs, plays a significant role in the development of cancer (44). Although there are many possible origins of CAFs, they are largely thought to arise from resident tissue fibroblasts (42). Therefore, IPF-activated fibroblasts could be one source of CAFs in IPF-LC. Thus, the production of stromal cell proteins by activated fibroblasts in the lungs of patients with IPF may cause LC. In addition, we speculate that ECM-related mechanisms play an important role in this process.
Pirfenidone and nintedanib are 2 drugs approved for IPF treatment and may prolong the survival time and reduce the incidence rate of those with LC (10). Nintedanib was approved as a second-line therapeutic drug for NSCLC, and pirfenidone has demonstrated antitumor activity in preclinical studies of NSCLC (45,46). Nintedanib is a tyrosine kinase inhibitor which inhibits angiogenesis by blocking various growth factors and has been used to treat both NSCLC and IPF (47). Pirfenidone inhibits TGF-β1–induced type I collagen overexpression in LC (48). Given the mechanistic similarities between LC and IPF and the coexistence of NSCLC and IPF, specific drugs need to be investigated. The CellMiner database analysis in this study indicated that the shared genes were associated with many drugs that have already been approved by the FDA for the treatment of cancer. These drugs may offer new possibilities for the treatment of patients with LC-IPF.
Conclusions
We determined that ECM-related mechanisms may be the underlying link between LC and IPF. Moreover, 7 shared genes were identified as potential diagnostic markers and therapeutic targets for LUAD and IPF. Our findings provide further insight into the interplay between LUAD and IPF and highlight potential drug targets for treatment.
Acknowledgments
We acknowledge the contributions to the study of all the doctors from the Department of Thoracic Surgery, Affiliated Hospital of Nantong University, who also provided written permission for the publication of data and conclusions.
Funding: This work was supported by the National Natural Science Foundation of China (No. 81770266), the Clinical Medical Research Center of Cardiothoracic Diseases in Nantong (No. HS2019001), the Innovation Team of Cardiothoracic Disease in Affiliated Hospital of Nantong University (No. TECT-A04), and Nantong Key Laboratory of Translational Medicine of Cardiothoracic Diseases.
Footnote
Reporting Checklist: The authors have completed the STARD reporting checklist. Available at https://jtd.amegroups.com/article/view/10.21037/jtd-22-1522/rc
Conflicts of Interest: All authors have completed the ICMJE uniform disclosure form (available at https://jtd.amegroups.com/article/view/10.21037/jtd-22-1522/coif). All authors report that this work was supported by the National Natural Science Foundation of China (No. 81770266), the Clinical Medical Research Center of Cardiothoracic Diseases in Nantong (No. HS2019001), the Innovation Team of Cardiothoracic Disease in Affiliated Hospital of Nantong University (No. TECT-A04), and Nantong Key Laboratory of Translational Medicine of Cardiothoracic Diseases. The authors have no other conflicts of interest to declare.
Ethical Statement: The authors are accountable for all aspects of the work in ensuring that questions related to the accuracy or integrity of any part of the work are appropriately investigated and resolved. The study was in accordance with the Helsinki Declaration (as revised in 2013).
Open Access Statement: This is an Open Access article distributed in accordance with the Creative Commons Attribution-NonCommercial-NoDerivs 4.0 International License (CC BY-NC-ND 4.0), which permits the non-commercial replication and distribution of the article with the strict proviso that no changes or edits are made and the original work is properly cited (including links to both the formal publication through the relevant DOI and the license). See: https://creativecommons.org/licenses/by-nc-nd/4.0/.
References
- Hadjicharalambous MR, Lindsay MA. Idiopathic Pulmonary Fibrosis: Pathogenesis and the Emerging Role of Long Non-Coding RNAs. Int J Mol Sci 2020;21:524. [Crossref] [PubMed]
- Raghu G, Remy-Jardin M, Myers JL, et al. Diagnosis of Idiopathic Pulmonary Fibrosis. An Official ATS/ERS/JRS/ALAT Clinical Practice Guideline. Am J Respir Crit Care Med 2018;198:e44-68. [Crossref] [PubMed]
- Lederer DJ, Martinez FJ. Idiopathic Pulmonary Fibrosis. N Engl J Med 2018;379:797-8. [PubMed]
- Chanda D, Otoupalova E, Smith SR, et al. Developmental pathways in the pathogenesis of lung fibrosis. Mol Aspects Med 2019;65:56-69. [Crossref] [PubMed]
- Raghu G, Chang J. Idiopathic pulmonary fibrosis: current trends in management. Clin Chest Med 2004;25:621-36. v. [Crossref] [PubMed]
- Maher TM, Bendstrup E, Dron L, et al. Global incidence and prevalence of idiopathic pulmonary fibrosis. Respir Res 2021;22:197. [Crossref] [PubMed]
- Natsuizaka M, Chiba H, Kuronuma K, et al. Epidemiologic survey of Japanese patients with idiopathic pulmonary fibrosis and investigation of ethnic differences. Am J Respir Crit Care Med 2014;190:773-9. [Crossref] [PubMed]
- Antoniou KM, Tomassetti S, Tsitoura E, et al. Idiopathic pulmonary fibrosis and lung cancer: a clinical and pathogenesis update. Curr Opin Pulm Med 2015;21:626-33. [Crossref] [PubMed]
- Schäfer M, Werner S. Cancer as an overhealing wound: an old hypothesis revisited. Nat Rev Mol Cell Biol 2008;9:628-38. [Crossref] [PubMed]
- Miura Y, Saito T, Tanaka T, et al. Reduced incidence of lung cancer in patients with idiopathic pulmonary fibrosis treated with pirfenidone. Respir Investig 2018;56:72-9. [Crossref] [PubMed]
- McCormack PL. Nintedanib: first global approval. Drugs 2015;75:129-39. [Crossref] [PubMed]
- Liu J, Zhao J, Xu J, et al. SPINK5 is a Prognostic Biomarker Associated With the Progression and Prognosis of Laryngeal Squamous Cell Carcinoma Identified by Weighted Gene Co-Expression Network Analysis. Evol Bioinform Online 2022;18:11769343221077118. [Crossref] [PubMed]
- Langfelder P, Horvath S. WGCNA: an R package for weighted correlation network analysis. BMC Bioinformatics 2008;9:559. [Crossref] [PubMed]
- Zhou Y, Zhou B, Pache L, et al. Metascape provides a biologist-oriented resource for the analysis of systems-level datasets. Nat Commun 2019;10:1523. [Crossref] [PubMed]
- Murohashi K, Hara Y, Saigusa Y, et al. Clinical significance of Charlson comorbidity index as a prognostic parameter for patients with acute or subacute idiopathic interstitial pneumonias and acute exacerbation of collagen vascular diseases-related interstitial pneumonia. J Thorac Dis 2019;11:2448-57. [Crossref] [PubMed]
- Ballester B, Milara J, Cortijo J. Idiopathic Pulmonary Fibrosis and Lung Cancer: Mechanisms and Molecular Targets. Int J Mol Sci 2019;20:593. [Crossref] [PubMed]
- Norris RA, Moreno-Rodriguez R, Hoffman S, et al. The many facets of the matricelluar protein periostin during cardiac development, remodeling, and pathophysiology. J Cell Commun Signal 2009;3:275-86. [Crossref] [PubMed]
- Uchida M, Shiraishi H, Ohta S, et al. Periostin, a matricellular protein, plays a role in the induction of chemokines in pulmonary fibrosis. Am J Respir Cell Mol Biol 2012;46:677-86. [Crossref] [PubMed]
- Liu AY, Zheng H, Ouyang G. Periostin, a multifunctional matricellular protein in inflammatory and tumor microenvironments. Matrix Biol 2014;37:150-6. [Crossref] [PubMed]
- Yamato H, Kimura K, Fukui E, et al. Periostin secreted by activated fibroblasts in idiopathic pulmonary fibrosis promotes tumorigenesis of non-small cell lung cancer. Sci Rep 2021;11:21114. [Crossref] [PubMed]
- Zhang Z, Bai M, Barbosa GO, et al. Broadly conserved roles of TMEM131 family proteins in intracellular collagen assembly and secretory cargo trafficking. Sci Adv 2020;6:eaay7667. [Crossref] [PubMed]
- Tjin G, White ES, Faiz A, et al. Lysyl oxidases regulate fibrillar collagen remodelling in idiopathic pulmonary fibrosis. Dis Model Mech 2017;10:1301-12. [Crossref] [PubMed]
- Prieto TG, Machado-Rugolo J, Baldavira CM, et al. The Fibrosis-Targeted Collagen/Integrins Gene Profile Predicts Risk of Metastasis in Pulmonary Neuroendocrine Neoplasms. Front Oncol 2021;11:706141. [Crossref] [PubMed]
- Elson GC, Lelièvre E, Guillet C, et al. CLF associates with CLC to form a functional heteromeric ligand for the CNTF receptor complex. Nat Neurosci 2000;3:867-72. [Crossref] [PubMed]
- Kass DJ, Yu G, Loh KS, et al. Cytokine-like factor 1 gene expression is enriched in idiopathic pulmonary fibrosis and drives the accumulation of CD4+ T cells in murine lungs: evidence for an antifibrotic role in bleomycin injury. Am J Pathol 2012;180:1963-78. [Crossref] [PubMed]
- Yu ST, Zhong Q, Chen RH, et al. CRLF1 promotes malignant phenotypes of papillary thyroid carcinoma by activating the MAPK/ERK and PI3K/AKT pathways. Cell Death Dis 2018;9:371. [Crossref] [PubMed]
- Li Y, Xun J, Wang B, et al. miR-3065-3p promotes stemness and metastasis by targeting CRLF1 in colorectal cancer. J Transl Med 2021;19:429. [Crossref] [PubMed]
- Shiratsuchi H, Wang Z, Chen G, et al. Oncogenic Potential of CYP24A1 in Lung Adenocarcinoma. J Thorac Oncol 2017;12:269-80. [Crossref] [PubMed]
- Chen G, Kim SH, King AN, et al. CYP24A1 is an independent prognostic marker of survival in patients with lung adenocarcinoma. Clin Cancer Res 2011;17:817-26. [Crossref] [PubMed]
- Yin Z, Watsky MA. Chloride channel activity in human lung fibroblasts and myofibroblasts. Am J Physiol Lung Cell Mol Physiol 2005;288:L1110-6. [Crossref] [PubMed]
- Shlyonsky V, Soussia IB, Naeije R, et al. Opposing effects of bone morphogenetic protein-2 and endothelin-1 on lung fibroblast chloride currents. Am J Respir Cell Mol Biol 2011;45:1154-60. [Crossref] [PubMed]
- Yin Z, Tong Y, Zhu H, et al. ClC-3 is required for LPA-activated Cl- current activity and fibroblast-to-myofibroblast differentiation. Am J Physiol Cell Physiol 2008;294:C535-42. [Crossref] [PubMed]
- Rajski M, Saaf A, Buess M. BMP2 response pattern in human lung fibroblasts predicts outcome in lung adenocarcinomas. BMC Med Genomics 2015;8:16. [Crossref] [PubMed]
- Xu Z, Chen C. Abnormal Expression and Prognostic Significance of Bone Morphogenetic Proteins and Their Receptors in Lung Adenocarcinoma. Biomed Res Int 2021;2021:6663990. [Crossref] [PubMed]
- Meng W, Xiao H, Zhao R, et al. The Prognostic Value of Bone Morphogenetic Proteins and Their Receptors in Lung Adenocarcinoma. Front Oncol 2021;11:608239. [Crossref] [PubMed]
- Vancheri C. Idiopathic pulmonary fibrosis and cancer: do they really look similar. BMC Med 2015;13:220. [Crossref] [PubMed]
- Chen X, Shi C, Cao H, et al. The hedgehog and Wnt/β-catenin system machinery mediate myofibroblast differentiation of LR-MSCs in pulmonary fibrogenesis. Cell Death Dis 2018;9:639. [Crossref] [PubMed]
- Epstein Shochet G, Brook E, Bardenstein-Wald B, et al. TGF-β pathway activation by idiopathic pulmonary fibrosis (IPF) fibroblast derived soluble factors is mediated by IL-6 trans-signaling. Respir Res 2020;21:56. [Crossref] [PubMed]
- Thannickal VJ, Henke CA, Horowitz JC, et al. Matrix biology of idiopathic pulmonary fibrosis: a workshop report of the national heart, lung, and blood institute. Am J Pathol 2014;184:1643-51. [Crossref] [PubMed]
- Horowitz JC, Osterholzer JJ, Marazioti A, et al. “Scar-cinoma”: viewing the fibrotic lung mesenchymal cell in the context of cancer biology. Eur Respir J 2016;47:1842-54. [Crossref] [PubMed]
- Naba A, Clauser KR, Hoersch S, et al. The matrisome: in silico definition and in vivo characterization by proteomics of normal and tumor extracellular matrices. Mol Cell Proteomics 2012;11:M111.014647.
- Kalluri R. The biology and function of fibroblasts in cancer. Nat Rev Cancer 2016;16:582-98. [Crossref] [PubMed]
- Peinado H, Zhang H, Matei IR, et al. Pre-metastatic niches: organ-specific homes for metastases. Nat Rev Cancer 2017;17:302-17. [Crossref] [PubMed]
- Oskarsson T, Massagué J. Extracellular matrix players in metastatic niches. EMBO J 2012;31:254-6. [Crossref] [PubMed]
- Reck M, Kaiser R, Mellemgaard A, et al. Docetaxel plus nintedanib versus docetaxel plus placebo in patients with previously treated non-small-cell lung cancer (LUME-Lung 1): a phase 3, double-blind, randomised controlled trial. Lancet Oncol 2014;15:143-55. [Crossref] [PubMed]
- Mediavilla-Varela M, Boateng K, Noyes D, et al. The anti-fibrotic agent pirfenidone synergizes with cisplatin in killing tumor cells and cancer-associated fibroblasts. BMC Cancer 2016;16:176. [Crossref] [PubMed]
- Paliogiannis P, Fois SS, Fois AG, et al. Repurposing Anticancer Drugs for the Treatment of Idiopathic Pulmonary Fibrosis and Antifibrotic Drugs for the Treatment of Cancer: State of the Art. Curr Med Chem 2021;28:2234-47. [Crossref] [PubMed]
- Hisatomi K, Mukae H, Sakamoto N, et al. Pirfenidone inhibits TGF-β1-induced over-expression of collagen type I and heat shock protein 47 in A549 cells. BMC Pulm Med 2012;12:24. [Crossref] [PubMed]
(English Language Editor: J. Jones and J. Gray)