The predictive value of chest computed tomography images, tumor markers, and metabolomics in the identification of benign and malignant pulmonary nodules
Highlight box
Key findings
• Chest CT images and TMs, combined with metabolomics, have potential applicability in the diagnosis of BPNs and MPNs.
What is known and what is new?
• Individual roles of chest CT images, TMs, and metabolomics are known.
• Research on combined identification value was newly performed.
What is the implication, and what should change now?
• Non-invasive diagnostic methods need further development.
Introduction
Pulmonary nodule (PN) is a common clinical sign, referring to circular or irregular lesions with a diameter less than or equal to 3 cm (30 mm) in the lungs. Imaging manifestations of PNs include shadows with increased density, which can either be single or multiple, with clear or unclear boundaries (1,2). They are usually found accidentally during physical examination or diagnosis and treatment of other diseases. Most patients do not have obvious symptoms, and those with symptoms are mostly manifested as dyspnea, chest pain, cough, hemoptysis, and others (3). PNs can be classified in many ways, for example, they can be sorted into solitary and multiple PNs according to the number of PNs. They can also be divided into PNs, small nodules, and micro nodules according to the diameter of nodules. According to the density of nodules, they can be classed as solid and subsolid PNs. Finally, PN can also be classified into benign PNs (BPNs) and malignant PNs (MPNs) (4,5).
From a clinical perspective, tissue biopsy is used to determine whether a nodule is benign or malignant. Special treatment is not required if the benign nodule is small in size (typically less than 5 mm in diameter) and does not cause any significant clinical symptoms. Chest computed tomography (CT) scans should be performed at the hospital every six months to monitor any changes in the nodules (6,7). Myeloproliferative tumors usually occur in the early stages of lung cancer and may not have particularly typical symptoms. However, common symptoms include clubbed fingers, hemoptysis, cough, expectoration, and arthritis (8). In clinical practice, physicians need to make a comprehensive diagnosis based on chest CT scans, blood and biochemical tests, and even tumor markers (TMs) to determine the nature of the condition and provide treatment as soon as possible. In the early stage of MPNs, surgical resection is the preferred treatment, supplemented by radiotherapy, chemotherapy, biological therapy, interventional therapy, and other comprehensive treatments, to achieve the purpose of curing lung cancer and preventing recurrence (9-11).
Currently, there are many examination and diagnostic methods for PNs in clinical practice, including physical examination, laboratory examination, imaging examination, and pathological examination (12). Laboratory examination mainly comprises TM detection and tuberculin skin testing. Imaging examinations mainly include chest X-ray examination, chest CT scan, and positron emission tomography (PET)-CT (13,14). Among them, chest X-ray examination has certain value for the diagnosis of PNs and the identification of lesions, but the significance is small. Compared with chest X-ray, chest CT scan can provide more information about the location, size, morphology, density, margin, and internal characteristics of PNs. Thus, it becomes the major examination method for diagnosis of PNs (15,16). PET-CT is of certain value for the determination of active lesions in patients, helping to determine the benign and malignant nodules. It also provides an important basis for the selection of sites for puncture biopsy (17).
Currently, commonly used pulmonary TMs predominantly include carbohydrate antigen 125 (CA125), carbohydrate antigen 199 (CA199), carbohydrate antigen 153 (CA153), and squamous cell carcinoma antigen (SCC-Ag) (18,19). Carcinoembryonic antigen (CEA), protein antigen, cytokeratin-19 fragment antigen (CYFRA 21-1), tissue polypeptide specific antigen, neuron-specific enolase (NSE), neuron-specific enzyme antigens, and pro-gastrin-releasing peptide are also included (20). The examination of TMs is of reference value for pulmonary malignancies. Usually, elevated levels of TMs can indicate the possible existence of malignant tumors, which should be further diagnosed in time. For example, biopsy should be conducted to make a final determination of space-occupying lesions (21).
In recent years, the idea of systems biology has been frequently applied to the innovation of etiologic tracing and diagnosis of various diseases. Metabolomics, as a new subject after genomics and proteomics, is developing vigorously. Metabolomics seeks the relative relationship between metabolites and physiological and pathological changes through the quantitative analysis of all metabolites in the organisms within a certain period (22,23). The basis of metabolomics is group indicator analysis, which relies on high-throughput detection and data processing. It aims to analyze the physiological state of organisms through information modelling and system integration (24). Currently, common metabolomic techniques consist of nuclear magnetic resonance, mass spectrometry, and chromatography [high performance liquid chromatography (HPLC) and gas chromatography] (25). In clinical studies, nuclear magnetic resonance spectra of a series of samples are first detected when studying the metabolomics of an organism. Then, the physiological and pathological states of the organism are judged by model recognition method. Finally, biomarkers related to its pathology are identified as its warning signals (26,27).
In general, the related technologies of metabolomics have been applied to the diagnosis and prediction of various diseases. However, there are relatively few metabolomics studies on the differentiation of BPNs and MPNs, especially the metabolites of fatty acids (FAs) in metabolomics, such as free FA spectrum and 31 kinds of free FA indexes. Current studies have confirmed some correlations between FA metabolism and lung diseases. Some studies have shown that FA metabolism disorders may be associated with the occurrence and development of lung diseases such as asthma and chronic obstructive pulmonary disease. In addition, FA metabolism in patients with lung cancer may also be affected. Moreover, there are no relevant studies of the simultaneous application of chest CT images, TMs, and metabolomic technologies to the diagnosis of clinical BPNs and MPNs in patients. Therefore, this work aimed to conduct corresponding studies about the above topics, and compare and to analyze the effectiveness and accuracy of the above 3 diagnostic methods in diagnosing BPNs and MPNs clinically. We hoped to yield some reference values for the diagnosis of benign and MPNs. We present this article in accordance with the STARD reporting checklist (available at https://jtd.amegroups.com/article/view/10.21037/jtd-23-250/rc).
Methods
Participants
A total of 110 patients with PNs diagnosed in Dongtai Hospital of Traditional Chinese Medicine from March 2021 to March 2022 were selected as the study cohort for a retrospective analysis. All patients underwent chest CT and pathological biopsy.
The inclusion criteria were as follows: (I) the pulmonary space-occupying lesion was round or quasi-round, and the maximum diameter was less than 3 cm. (II) The patient was not accompanied by hilar abnormalities of the lungs, atelectasis, or abnormality of surrounding lung tissues. (III) All the patients had definite pathological diagnostic results. (IV) The patients had no prior history of chemoradiotherapy. (V) The patients had not previously received relevant treatment for small PNs. The exclusion criteria were as follows: (I) incomplete patient clinical medical records; (II) the patients were not diagnosed by pathological examination after admission; (III) the patients had a history of chemoradiotherapy; (IV) the patients experienced severe complications, like tuberculosis, lung injury, COVID-19 infection, etc.
This study was approved by the Ethics Committee of Dongtai Hospital of Traditional Chinese Medicine (No. DY2021008) and informed consent was provided by all participants. The study was conducted in accordance with the Declaration of Helsinki (as revised in 2013).
Chest CT imaging examination
Chest CT examination was performed on all participants using a 64-slice spiral CT machine. The scanning range of CT was the whole-lung scan from the starting point of the thoracic cage to the lower margin of the costophrenic angle on both sides, with a slice thickness of 5 mm.
According to the results of chest CT scan, the relevant 3-dimensional (3D) image features of PN lesions in each patient were recorded. These included the location, diameter, density, marginal features, calcification, vascular signs, pleural tracheal signs, and tracheal signs of the patients’ nodules. All these imaging data were judged independently by 2 senior radiologists. In case of inconsistency in the assessment of patients’ imaging, the unified diagnosis results were finalized through consultation of the 2 radiologists.
Then, according to the chest CT imaging signs of patients, a mathematical model for the prediction of benign and malignant solitary PNs was constructed. The model version was based on that of the Thoracic Surgery Department in Peking University People’s Hospital. The mathematical equation of benign and malignant predictive value P of solitary PNs was expression as Eq. [1]. The mathematical equation for K value of Eq. [1] was shown as Eq. [2].
In the equations, a, b, c, d, e, and f represent patients’ age, diameter of PN, spicule of PN, family history of tumor, calcification of PN, and boundary of PN, respectively. However, the collection standard of PN diameter referred to the maximum cross-sectional diameter (cm) of a solitary PN detected by preoperative chest CT. For other PN signs, if a sign occurred, the value of this sign was 1. If a sign did not occur, the value of this sign was 0. The mathematical model of benign and malignant prediction of solitary PNs is displayed in Figure 1.
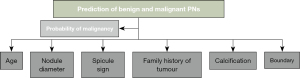
Before the benign and malignant prediction model of solitary PNs was applied, the data of 10 patients were randomly selected for accuracy verification experiment. After the accuracy verification was deemed successful, the data of all the PN patients included in the study were brought into the mathematical model. The benign and malignant conditions of PNs in each patient were predicted in accordance with the calculation results by the model. After the prediction, the outcomes were compared with the actual pathological results.
Detection and analysis of serum TMs in patients
Before test, patients should take care of their diet and avoid seafood, alcohol, spicy and high-oil food intake at least 3 days. Fasting vein blood collection was performed on all participants in the morning of the day after admission. The blood collection volume was 10 mL, which was divided into 2 parts. After being placed aside, 5 mL of the blood sample was centrifuged at 3,000 rpm/min and the upper serum was removed. After that, the Roche electrochemical immunoassay kit (Roche, Basel, Switzerland) and the matching analyzer were utilized to detect TMs in the collected upper serum according to the procedure in the instructions. The detected TMs in this study included CEA, NSE, CYFRA 21-1, and SCC-Ag. After the detections of serum TMs in all patients, the detection results were compared with the actual pathological results.
Analysis of plasma samples from patients
Metabolomic analysis was performed on the other 5 mL of blood samples collected from fasting vein in the morning after admission. Plasma samples were utilized to detect the free FA spectrum of these patients, and 31 kinds of free FA indicators were obtained. The total ω-3 polyunsaturated FA (W3), total ω-6 polyunsaturated FA (W6), palmitic acid, palmitoleic acid, lauric acid, nervonic acid, and so on, were included.
Clinical data collection
The basic data of the patients were collected, and the chest CT imaging features of the patients’ PNs, the detection results of TMs, metabolomic results, and clinicopathological results were recorded. The basic data collected consisted of age, gender, weight, height, body mass index (BMI), smoking history, daily smoking amount, emphysema or not, family history of tumors or not, and symptoms. Chest CT imaging features of patients with PNs included the location, diameter, density, margin features, calcification, vascular signs, pleural tracheal signs, tracheal signs, and vacuole signs of PNs. Pulmonary TMs were examined by kits of enzyme-linked immunosorbent assay (ELISA). Detected indicators in serum included NSE, CEA, CYFRA 21-1, and so on. The metabolomic data of patients included free FA profiles and 31 free FA indicators.
Accuracy evaluation indicators of different diagnostic prediction methods
When the benign and malignant diagnosis of solitary PNs was studied by chest CT, the malignant probability of patients’ PNs worked out after the mathematical model prediction was compared with the actual pathological results of patients. The consistency was determined by chi-square (χ2) test. The Kappa value evaluated the consistency rate of the mathematical model, and the receiver operating characteristic (ROC) curve was drawn according to the prediction probability. The area under ROC curve (AUC) was calculated, and the accuracy of the mathematical model for benign and malignant prediction of solitary PNs was evaluated.
The horizontal axis of ROC curve represented the false positive rate (FPR), whereas the vertical axis represented the true positive rate (TPR). Their calculations were expressed as Eq. [3] and Eq. [4]; the calculation of AUC followed Eq. [5].
FP, TP, TN, and FN stood for false positives, true positives, true negatives, and false negatives, respectively. The Youden index was also taken as the cut-off point on AUC images, and the outcomes above the cut-off point were judged as high malignant risk of PNs, whereas those below the cut-off point were judged as low malignant risk. The calculation formula of Youden index Y was computed as Eq. [6].
Se and Sp represented sensitivity and specificity, respectively, of which the calculation equations were described as Eq. [7] and Eq. [8]. The misdiagnosis rate W and missed diagnosis rate L were calculated as Eq. [9] and Eq. [10], respectively.
a represented TP, that was, patients with MPNs were diagnosed as malignant. b represented FP, that was, patients with non-MPNs were diagnosed as malignant. c was TN, that was, patients with non-MPNs were diagnosed as benign. d was FN, that was, patients with MPNs were diagnosed as benign. In addition, Eq. [11] and Eq. [12] show the calculations of positive predictive value (PPV) and negative predictive value (NPV), respectively.
Technical route
Figure 2 displays the technical route of this study.
Statistics
The software SPSS 19.0 (IBM Corp., Armonk, NY, USA) was applied to process the experimental data. The mean ± standard deviation indicated the measurement data, whereas the comparisons of mean values between/among groups conducted made by t-test. The enumeration data were represented by percentage (%), and the χ2 test was adopted. P<0.05 was utilized to indicate statistically significant differences.
Results
Summary of pathological results of PNs
Figure 3 shows the distribution of pathological results of patients. Among the patients with PNs, there were 72 patients with MPNs and 38 patients with BPNs. The number of patients with adenocarcinoma was the largest in MPNs, followed by squamous cell carcinoma, metastatic carcinoma, small cell carcinoma, neuroendocrine tumor, and adenosquamous carcinoma. Among the patients with BPNs, chronic inflammation was the most common, followed by tuberculosis, granulomatous inflammation, hamartoma, fungal infection, mechanical pneumonia, atypical adenomatous hyperplasia, inflammatory pseudotumor, bronchogenic cyst, sclerosing pulmonary cell tumor, and clear vascular Castleman’s disease.
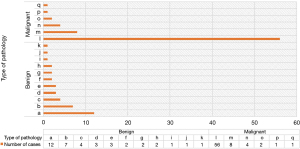
Comparison of basic data of BPN and MPN patients
Figure 4 presents the comparison of gender and average age of BPN and MPN patients. As shown in Figure 4, there was no significant difference in the gender ratio between the patients with BPNs and those with MPNs included in the work (P>0.05). The average age of patients with MPNs was 62 years old, remarkably higher than the 56 years old of patients with BPNs, with a statistically significant difference (P<0.05).
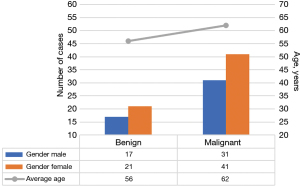
Figure 5 displays a comparison of the medical history and symptoms of BPN and MPN patients. No significant differences were discovered in tumor history, smoking history, lung disease history, and clinical symptoms between the included patients with BPNs and MPNs (P>0.05).
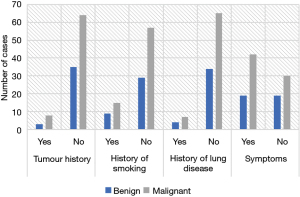
Comparison of chest CT morphologic signs of BPN and MPN patients
Figure 6 presents the comparison of the location and margin of PNs between the 2 groups. Figure 7 compares lobules and spicule sign of PNs between groups. Figure 8 shows the comparison of density of PNs between groups. From Figures 6-8, in CT morphological signs of patients, notable differences of statistical significance were found in the location of PNs, the number of patients with lobules, spicule sign, and vessel convergence sign between MPN and BPN groups (P<0.05). There was no significant difference between groups in the number of patients with pleural traction, calcification, vacuole sign, air bronchial sign, solid or ground glass nodules, and mixed ground glass nodules or not in CT images (P>0.05).
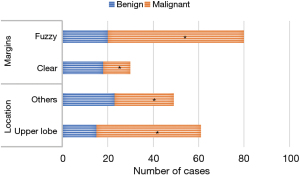
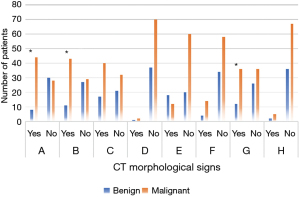
Detection results of TMs in BPN and MPN patients
Figure 9 compares the positive rates of TMs detected of PN patients in the 2 groups. No significant differences were detected in serum CEA, CYFRA 21-1, NSE, and SCC-Ag levels between groups (P>0.05).
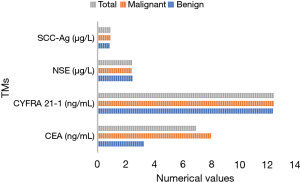
Figure 10 shows the comparison of positive TMs between groups. The serum contents of CEA and CYFRA 21-1 in the MPN group were remarkably higher than those in the BPN group with statistical significance (P<0.05). The contents of NSE and SCC-Ag were not significantly different between groups (P>0.05).
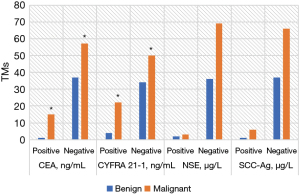
Metabolomic results of two groups of patients
Figures 11-15 display the metabolomic detection results of the 2 groups of patients. According to Figures 11-15, there was no significant difference in the levels of plasma triene/tetraene, W3/W6, erucic acid, arachidonic acid, lauric acid, eicosadienoic acid, arachidic acid, epoxyeicosatrienoic acid, lignoceric acid, montanic acid, myristic acid, docosapentaenoic acid, docosatrienoic acid, palmitoleic acid, docosahexaenoic acid, oleic acid, total monounsaturated FAs, linoleic acid, total W6, and total polyunsaturated FAs between the MPN group and the BPN group, and the differences were not statistically significant (P>0.05).
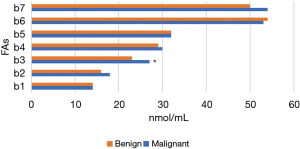
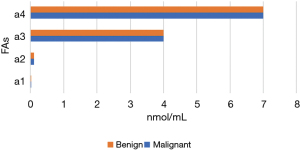
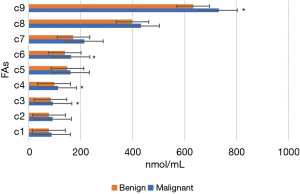
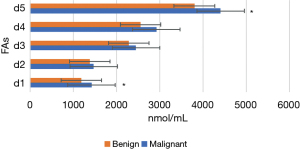
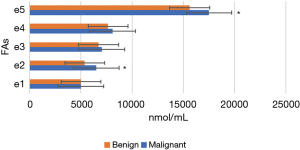
However, the levels of plasma palmitic acid, total W3, nervonic acid, stearic acid, docosatetraenoic acid, alpha-linolenic acid, eicosapentaenoic acid, total saturated FAs, and total FAs in the MPN group were significantly higher than those in the BPN group, and the differences were statistically significant (P<0.05).
Discussion
In the clinical CT diagnosis of patients with PNs, the BPN and MPN are often assessed from the following aspects: (I) their location: PNs in the anterior upper lobe of lungs are more likely to be malignant. The posterior apical segment of upper lobe or the dorsal segment of lower lobe of both lungs is the most common site of tuberculosis. PNs in this site may be benign lesions, especially tuberculosis, judged from its location (28). (II) Their size: small nodules with a diameter of less than 5 mm have a relatively high proportion of BPNs, reaching about 80%; there is a relatively low probability of malignancy. If the diameter is over 1 cm, its malignant probability is as high as more than 50%, and the total proportion is about 50–80% (29). (III) From the density, if there is uneven density in the middle, the boundary is not very clear, and there are shallow lobules, small/short spicules, and pleural shrinkage features, the probability of malignancy is relatively great. On the contrary, if the boundaries are clear with thick spicules, the probability to be benign is relatively high (30). (IV) The surrounding structure: in the surrounding manifestations, such as inflammatory manifestations, emphysema, obstructive pneumonia, obstructive local atelectasis, or local infiltration and vascular penetration, the probability of malignancy is relatively high (31). Contrastingly, for example, for PNs with nothing around them, such as satellite lesions, these manifestations may suggest small calcification, and if the boundaries are clear, the probability is relatively high to be benign (32). (5) From enhanced CT of the lungs, the uneven enhancement, such as the accumulation of nodules, small vacuoles, and eccentric voids in the middle, can be observed. These are all malignant features, so the probability is high to be malignant (33). In contrast, without significant enhancement, the probability to be benign increases. (6) Whether there is calcification: calcification around PNs often indicates a high probability of it being benign, and the probability of it being malignant is relatively low. The BPNs as well as MPNs are mainly identified from the above aspects.
In this study, chest CT images were utilized for benign and malignant diagnoses of patients with PNs. In CT morphological signs of patients with MPNs, the number of patients with upper lung lobe location, lobulation, spicule sign, and vessel convergence sign were considerably different from those in the BPN group (P<0.05), with statistical significance. There was not any significant difference between groups in the number of patients with pleural traction, calcification, vacuole sign, air bronchial sign, solid or ground glass nodules, and mixed ground glass nodules in CT images (P<0.05). The experimental results confirmed that the location, lobulation, spicule sign, and vessel convergence sign of PNs in CT morphological signs could indeed be taken as evaluation indicators to differentiate BPNs and MPNs. CT images also had a high practical value in the process of distinguishing BPNs and MPNs in clinical practice.
In this study, the related contents of FA metabolism in metabonomics were used, and metabonomics is one of the hot research fields in recent years. In the detection of pulmonary TMs in this study, there were no significant differences in serum CEA, CYFRA 21-1, NSE, and SCC-Ag levels in patients with MPNs (P>0.05). The serum contents of CEA and CYFRA 21-1 in the MPN group were observably higher than those in the BPN group with statistical significance (P<0.05). The contents of NSE and SCC-Ag were not significantly different between groups (P>0.05). Therefore, serum TMs CEA and CYFRA 21-1 had high diagnostic accuracy in the diagnosis of BPNs and MPNs. However, if a patient has elevated pulmonary TMs, the occurrence of MPNs or pulmonary tumors should be highly suspected clinically. Thereout, a clear diagnosis is often required under the patient’s clinical manifestations and further examination. The common clinical manifestations of pulmonary tumors consist of cough, expectoration, fever, chest pain, hemoptysis, dyspnea, and so on. Chest radiographs or pulmonary CT often indicate mass shadows. Sputum detection for cancer cells, bronchoscopy, and puncture biopsy can be performed to make a definite diagnosis.
In addition, the metabolomic results of the 2 groups showed no significant differences in the levels of plasma triene/tetraterpene, W3/W6, erucic acid, myristic oleic acid, lauric acid, eicosenoic acid, arachidic acid, eicosadienoic acid, lignoceric acid, behenic acid, docosapentaenoic acid, epoxyeicosatrienoic acid, palmitoleic acid, docosahexaenoic acid, arachidonic acid, oleic acid, total monounsaturated FA, linoleic acid, total W6, and total polyunsaturated FA between BPN and MPN groups (P>0.05). The plasma levels of palmitic acid, total W3, nervonic acid, stearic acid, docosatetraenoic acid, linolenic acid, eicosapentaenoic acid, total saturated FA, and total FA in the MPN group were notably higher than those in the BPN group, with statistically significant differences (P<0.05). In conclusion, the levels of plasma palmitic acid, total W3, nervonic acid, stearic acid, docosatetraenoic acid, linolenic acid, eicosapentaenoic acid, total saturated FA, and total FA in the FA metabolomics could be taken as great predictors in the differentiation of BPNs and MPNs. This was in line with the findings of Widłak et al. [2021] (34), which confirmed that FA metabolism indicators are of great clinical value in the diagnosis of BPNs and MPNs. In the future, it would be helpful to further investigate and explore the metabolomic features of different types of MPNs.
In addition, in this study, chest CT was used to detect the BPNs and MPNs, which is one of the commonly used non-invasive detection methods for PNs. Compared with invasive detection methods (such as bronchoscopy and puncture biopsy), it has the advantages of high detection accuracy, simple operation, and low cost, providing patients with the advantages of a safer, faster and more economic detection option. Moreover, the risk of continuous monitoring using these models may outweigh the risk of biopsy where minimally invasive biopsies can be performed by endobronchial ultrasound-guided transbronchial needle aspiration (EBUS-TBNA) or robotic bronchoscopy. In conclusion, this study has a certain reference value in the promotion of non-invasive clinical detection of PNs.
Conclusions
The chest CT images and TMs, combined with metabolomics have a good application effect in the diagnosis of BPNs and MPNs, and are worthy of further promotion. More non-invasive co-diagnostic models need to be constructed and fully clinically validated.
Acknowledgments
Funding: None.
Footnote
Reporting Checklist: The authors have completed the STARD reporting checklist. Available at https://jtd.amegroups.com/article/view/10.21037/jtd-23-250/rc
Data Sharing Statement: Available at https://jtd.amegroups.com/article/view/10.21037/jtd-23-250/dss
Peer Review File: Available at https://jtd.amegroups.com/article/view/10.21037/jtd-23-250/prf
Conflicts of Interest: All authors have completed the ICMJE uniform disclosure form (available at https://jtd.amegroups.com/article/view/10.21037/jtd-23-250/coif). The authors have no conflicts of interest to declare.
Ethical Statement: The authors are accountable for all aspects of the work in ensuring that questions related to the accuracy or integrity of any part of the work are appropriately investigated and resolved. The study was conducted in accordance with the Declaration of Helsinki (as revised in 2013). This study was approved by the Ethics Committee of Dongtai Hospital of Traditional Chinese Medicine (No. DY2021008) and informed consent was provided by all participants.
Open Access Statement: This is an Open Access article distributed in accordance with the Creative Commons Attribution-NonCommercial-NoDerivs 4.0 International License (CC BY-NC-ND 4.0), which permits the non-commercial replication and distribution of the article with the strict proviso that no changes or edits are made and the original work is properly cited (including links to both the formal publication through the relevant DOI and the license). See: https://creativecommons.org/licenses/by-nc-nd/4.0/.
References
- Wu Q, Cao B, Zheng Y, et al. Feasibility and safety of fine positioning needle-mediated breathing control in CT-guided percutaneous puncture of small lung/liver nodules adjacent to diaphragm. Sci Rep 2021;11:3411. [Crossref] [PubMed]
- Nishino M. CT Volumetry for Lung-RADS Classification of Solid Nodules. Radiology 2020;297:685-6. [Crossref] [PubMed]
- Gu Y, Chi J, Liu J, et al. A survey of computer-aided diagnosis of lung nodules from CT scans using deep learning. Comput Biol Med 2021;137:104806. [Crossref] [PubMed]
- Li JS. International Clinical Practice Guideline of Chinese Medicine: Chronic Obstructive Pulmonary Disease. World J Tradit Chin Med 2020;6:39-50. [Crossref]
- Holbrook MD, Clark DP, Patel R, et al. Detection of Lung Nodules in Micro-CT Imaging Using Deep Learning. Tomography 2021;7:358-72. [Crossref] [PubMed]
- Iraqi Houssaini Z. Lung nodules. Rev Med Interne 2021;42:885-6. [PubMed]
- Mastouri R, Khlifa N, Neji H, et al. Deep learning-based CAD schemes for the detection and classification of lung nodules from CT images: A survey. J Xray Sci Technol 2020;28:591-617. [Crossref] [PubMed]
- Wan C, Ma L, Liu X, et al. Computer-aided Classification of Lung Nodules on CT Images with Expert Knowledge. Proc SPIE Int Soc Opt Eng 2021;11598:115982K.
- Iguchi T, Hiraki T, Matsui Y, et al. CT-guided biopsy of lung nodules with pleural contact: Comparison of two puncture routes. Diagn Interv Imaging 2021;102:539-44. [Crossref] [PubMed]
- Vieites Branco I, Martins S, Monteiro JP, et al. Fluoro-CT guided biopsy of lung nodules: a step by step revision. Port J Card Thorac Vasc Surg 2022;28:43-6. [PubMed]
- Zhang G, Yang Z, Gong L, et al. Classification of lung nodules based on CT images using squeeze-and-excitation network and aggregated residual transformations. Radiol Med 2020;125:374-83. [Crossref] [PubMed]
- Ghosh S, Mehta AC, Abuquyyas S, et al. Primary lung neoplasms presenting as multiple synchronous lung nodules. Eur Respir Rev 2020;29:190142. [Crossref] [PubMed]
- Heuvelmans MA, van Ooijen PMA, Ather S, et al. Lung cancer prediction by Deep Learning to identify benign lung nodules. Lung Cancer 2021;154:1-4. [Crossref] [PubMed]
- Thapaliya S, Gilligan LA, Brady SL, et al. Comparison of 0.3-mSv CT to Standard-Dose CT for Detection of Lung Nodules in Children and Young Adults With Cancer. AJR Am J Roentgenol 2021;217:1444-51. [Crossref] [PubMed]
- Zhang X, Liu X, Zhang B, et al. Accurate segmentation for different types of lung nodules on CT images using improved U-Net convolutional network. Medicine (Baltimore) 2021;100:e27491. [Crossref] [PubMed]
- Hwang EJ, Goo JM, Kim HY, et al. Optimum diameter threshold for lung nodules at baseline lung cancer screening with low-dose chest CT: exploration of results from the Korean Lung Cancer Screening Project. Eur Radiol 2021;31:7202-12. [Crossref] [PubMed]
- Kammer MN, Lakhani DA, Balar AB, et al. Integrated Biomarkers for the Management of Indeterminate Pulmonary Nodules. Am J Respir Crit Care Med 2021;204:1306-16. [Crossref] [PubMed]
- Zhou J, Cheng T, Li X, et al. Epigenetic imprinting alterations as effective diagnostic biomarkers for early-stage lung cancer and small pulmonary nodules. Clin Epigenetics 2021;13:220. [Crossref] [PubMed]
- Ostrin EJ, Sidransky D, Spira A, et al. Biomarkers for Lung Cancer Screening and Detection. Cancer Epidemiol Biomarkers Prev 2020;29:2411-5. [Crossref] [PubMed]
- Xu L, Su Z, Xie B. Diagnostic value of conventional tumor markers in young patients with pulmonary nodules. J Clin Lab Anal 2021;35:e23912. [Crossref] [PubMed]
- Liu Z, Wang L, Du M, et al. Plasm Metabolomics Study in Pulmonary Metastatic Carcinoma. J Oncol 2022;2022:9460019. [Crossref] [PubMed]
- Zhou W, Lin L, Jiang LY, et al. Comprehensive plasma metabolomics and lipidomics of benign and malignant solitary pulmonary nodules. Metabolomics 2022;18:71. [Crossref] [PubMed]
- Wang L, Zhang M, Pan X, et al. Integrative Serum Metabolic Fingerprints Based Multi-Modal Platforms for Lung Adenocarcinoma Early Detection and Pulmonary Nodule Classification. Adv Sci (Weinh) 2022;9:e2203786. [Crossref] [PubMed]
- Chen K, Lai YC, Vanniarajan B, et al. Clinical impact of a deep learning system for automated detection of missed pulmonary nodules on routine body computed tomography including the chest region. Eur Radiol 2022;32:2891-900. [Crossref] [PubMed]
- Mastouri R, Khlifa N, Neji H, et al. A bilinear convolutional neural network for lung nodules classification on CT images. Int J Comput Assist Radiol Surg 2021;16:91-101. [Crossref] [PubMed]
- Lee HJ, Son HJ, Yun M, et al. Prone position [18F]FDG PET/CT to reduce respiratory motion artefacts in the evaluation of lung nodules. Eur Radiol 2021;31:4606-14. [Crossref] [PubMed]
- Miki S, Nomura Y, Hayashi N, et al. Prospective Study of Spatial Distribution of Missed Lung Nodules by Readers in CT Lung Screening Using Computer-assisted Detection. Acad Radiol 2021;28:647-54. [Crossref] [PubMed]
- Jacob M, Romano J, Ara Jo D, et al. Predicting lung nodules malignancy. Pulmonology 2022;28:454-60. [Crossref] [PubMed]
- Galperin-Aizenberg M, Katz S, Shankla V, et al. Preliminary Assessment of an Optical Flow Method (OFM) for Nonrigid Registration and Temporal Subtraction (TS) of Serial CT Examinations to Facilitate Evaluation of Interval Change in Metastatic Lung Nodules. Curr Probl Diagn Radiol 2021;50:344-50. [Crossref] [PubMed]
- Lancaster HL, Heuvelmans MA, Pelgrim GJ, et al. Seasonal prevalence and characteristics of low-dose CT detected lung nodules in a general Dutch population. Sci Rep 2021;11:9139. [Crossref] [PubMed]
- Takaishi T, Ozawa Y, Bando Y, et al. Incorporation of a computer-aided vessel-suppression system to detect lung nodules in CT images: effect on sensitivity and reading time in routine clinical settings. Jpn J Radiol 2021;39:159-64. [Crossref] [PubMed]
- Hammer MM, Byrne SC. Cancer Risk in Nodules Detected at Follow-Up Lung Cancer Screening CT. AJR Am J Roentgenol 2022;218:634-41. [Crossref] [PubMed]
- Byrne SC, Hammer MM. Malignant Nodules Detected on Lung Cancer Screening CT: Yield of Short-Term Follow-Up CT in Showing Nodule Growth. AJR Am J Roentgenol 2022;219:735-41. [Crossref] [PubMed]
- Widłak P, Jelonek K, Kurczyk A, et al. Serum Metabolite Profiles in Participants of Lung Cancer Screening Study; Comparison of Two Independent Cohorts. Cancers (Basel) 2021;13:2714. [Crossref] [PubMed]
(English Language Editor: J. Jones)