Extracellular RNA profiles in non-small cell lung cancer plasma
Highlight box
Key findings
• The RNA biotypes of mitochondrial ribosomal RNAs and mitochondrial transfer RNAs were upregulated in the plasma. Hsa_circ_0000722 and hsa-miR-324-5p are potential biomarkers for non-small cell lung cancer (NSCLC).
What is known and what is new?
• Circulating extracellular RNAs are useful biomarkers for many diseases.
• Circulating hsa_circ_0000722 and hsa-miR-324-5p may be important molecular biomarkers for the diagnosis and prognosis of NSCLC.
What is the implication, and what should change now?
• Circulating hsa_circ_0000722 and hsa-miR-324-5p participated in the pathogenesis and progress of NSCLC; thus, circulating hsa_circ_0000722 and hsa-miR-324-5p may be potential therapeutic targets for NSCLC.
Introduction
Lung cancer is the most frequently diagnosed cancer and the leading cause of cancer-related death worldwide (1). The 2012 Global Cancer Statistics report (2) estimated that about 1.8 million new cases of lung cancer were diagnosed worldwide in 2012, which accounted for about 13% of the total cancer cases. Lung cancer can roughly be divided into non-small cell lung cancer (NSCLC) and small cell lung cancer (3). NSCLC accounts for 85% of newly diagnosed lung cancer cases, and lung adenocarcinoma is the most common type of NSCLC (3). The diagnosis and treatment of NSCLC is improving; however, the 5-year survival rate of NSCLC is still only about 15% (4). This is partly due to the lack of sensitive detection methods for NSCLC (5). Thus, it is crucial that the biomarkers of NSCLC be explored to improve the 5-year survival rate (6).
With the development of liquid biopsy, body fluids, including plasma, serum, urine, and saliva, as the carriers of tumor biomarkers, can be used to detect NSCLC (7,8). Plasma is an important part of the circulatory system, and circulating extracellular RNAs (exRNAs), which include microRNAs (miRNAs), long non-coding RNAs (lncRNAs), messenger RNAs (mRNAs), circular RNAs (circRNAs) and a series of non-coding RNAs (ncRNAs), were first identified in plasma in 1940s (9,10). The RNAs from the cells of whole-body tissues, and to some extent, the exRNAs of plasma, reflect the health of the body (11). Thus, circulating exRNAs are useful biomarkers for many diseases, including central nervous system disease, renal disease, and cancer (10,12,13). Many studies have reported possible NSCLC biomarkers, including miRNAs (e.g., mir-21 and mir-126) (14,15), and circRNAs (e.g., hsa_circ_0033155 and hsa_circ_0014130) (16,17). However, as the exRNAs in plasma are low in concentration, highly degraded, and fragmented, it is difficult to profile the total exRNA in the plasma (18,19).
CircRNAs are a new class of endogenous RNA and have a covalently closed continuous loop without 5' caps or 3' tails (20-22). Because of their unique closed-loop structure, circRNAs are highly stable and resistant to ribonuclease degradation (21). For a long time, circRNAs were thought to be the products of splicing errors, but there is increasing evidence that circRNAs play very important roles in the biogenesis process (23). Typically, circRNAs act as miRNA sponges to regulate gene expression by binding to miRNAs, which play an important role in the development and progression of cancers (24,25). Recent study has shown that circRNAs may be involved in the tumorigenesis and development of lung cancer, and relevant studies on circRNAs are of great significance for the diagnosis and treatment of lung cancer (26). However, the research on circRNAs is still in its infancy and requires researchers to explore more about the biological characteristics, biological functions and mechanisms of circRNAs in lung cancer through different research methods and approaches.
In this study, we conducted plasma exRNA sequencing to profile transcript expression in the plasma, assessed the association between these exRNAs and lung cancer and analyzed the possible mechanism to identify potential lung cancer biomarkers. We present this article in accordance with the MDAR reporting checklist (available at https://jtd.amegroups.com/article/view/10.21037/jtd-23-517/rc).
Methods
Patients and sample collection
This study was a double blind and random controlled trial. 12 peripheral blood samples (of approximately 2 mL), recruited from Jiangsu Province People’s Hospital, were collected, including 6 samples from NSCLC patients and 6 samples from normal controls (NCs) who were of a similar gender and age to the NSCLC patients. The clinical characteristics of the patients are presented in Wang et al.’s study (11). For the NSCLC samples, the eligible patients had to be aged ≥18 years and have histologically or cytologically confirmed locally advanced or metastatic NSCLC. We collected 2 mL of blood from another 34 NSCLC and 34 NC patients for the quantitative real-time polymerase chain reaction (qRT-PCR) validation (22 samples from the NSCLC patients and 22 samples from the NCs for the circRNA validation, and the others for the miRNA validation). The plasma samples were collected after the blood samples had been centrifugated at 3,000 rpm for 10 min at 4 ℃ and were then stored at −80 ℃ awaiting use. The plasma samples were collected from all the NSCLC patients before any treatment.
The study was conducted in accordance with the Declaration of Helsinki (as revised in 2013), and the protocol was approved by the Ethics Committee of Jiangsu Province People’s Hospital (No. 2019-SFRA-082). Written informed consent was obtained from each participant.
ExRNA-sequencing library preparation
The exRNA from 500 plasma samples was extracted using the miRNeasy Serum/Plasma Kit (QIAGEN, Mississauga, ON, Canada) according to the manufacturer’s protocol. Next, the NEBNext® Ultra™ Ⅱ Directional RNA Library Prep Kit for Illumina® (New England Biolabs, Ipswich, MA, USA) was used to prepare the exRNA-sequencing library and sequenced by Hiseq-PE150 (Illumina, San Diego, CA, USA). The sequencing data were submitted to the NCBI-SRA database (www.ncbi.nlm.nih.gov/sra), and the accession link is: https://dataview.ncbi.nlm.nih.gov/object/PRJNA589238.
Sequencing data analysis
FeatureCounts (v.1.5.0, San Diego, CA, USA) (27) was used to count the read numbers mapped to each transcript, and Homo_sapiens_Ensemble_94.gtf was used as the input file. Next, the annotation file in Ensembl biomart (https://www.ensembl.org/biomart/) was used to identify the biotypes of the expressed genes and classify the gene biotypes (28). For the statistical analysis, the t test was used to compare 2 groups, and a P value <0.05 was considered statistically significant.
In addition, DESeq2 (29) was used to analyze the differentially expressed transcript with NSCLC, and Metascape (30,31) was used to identify the enriched Gene Ontology (GO) and Kyoto Encyclopedia of Genes and Genomes (KEGG) terms.
Prediction of circRNAs
The circRNAs were identified using the clean sequencing data and CircRNA Identifier (CIRI) software (32). Subsequently, circBase (33) (http://www.circbase.org/) was used to annotate the circRNAs. For the circRNA analysis, the circRNAs that contained <3 reads in 12 individuals were excluded.
CircRNA and miRNA validation
For the circRNA validation, the total RNAs were isolated from 500 µL of human plasma using the miRNeasy Serum/Plasma Kit (Qiagen, Germany). For the miRNA validation, the total RNAs were isolated from 500 µL of human plasma using the RNAsimple Total RNA Kit (Tiangen Biotech, Beijing, China). The complementary DNA was synthesized using PrimeScript™ II reverse transcriptase (Takara, Japan) in accordance with the manufacturer’s instructions. Next, qRT-PCR was performed using SYBR Premix Ex Taq™ II (Takara, Japan) on the ABI 7500 system (ABI 7500, Alameda, CA, USA), the PCR conditions were: 30 cycles at 95 ℃; followed by 40 cycles at 95 ℃ for 5 s and 60 ℃ for 34 s. The cycle threshold (Ct) values were calculated using the automatic Ct setting of the 7500 system SDS software v1.4 (Applied Biosystems, Waltham, MA, USA). β-actin and U6 were used as the internal references for the circRNA and miRNA quantifications, respectively. All the primers used in this study are listed in Table 1.
Table 1
Primer name | Sequence (5’-3’) |
---|---|
hsa_circ_0000722-F | AGGAGCCCAGAGGCATGA |
hsa_circ_0000722-R | CGGTGGAAAGCATCCCTAGC |
hsa_circ_0006156-F | GAAGGGCCATAGTGGTGGAAG |
hsa_circ_0006156-R | CATGGCTGAGGGGTAGCTTG |
β-actin-F | GTGGCCGAGGACTTTGATTG |
β-actin-R | CCTGTAACAACGCATCTCATATT |
miR-324-5p RT primer | GAAAGAAGGCGAGGAGCAGATCGAGGAAGAAGACGGAAGAATGTGCGTCTCGCCTTCTTTCACACCA |
miR-326 RT primer | GAAAGAAGGCGAGGAGCAGATCGAGGAAGAAGACGGAAGAATGTGCGTCTCGCCTTCTTTCCTGGAG |
Universal miRNA Reverse Primer | CGAGGAAGAAGACGGAAGAAT |
miR-324-5p Forward Primer | AAACGCATCCCCTAGGGCAT |
miR-326 Forward Primer | AAACCTCTGGGCCCTTCCT |
U6 RT Primer | GCTTCACGAATTTGCGTGTCAT |
U6 Forward Primer | GCTTCGGCAGCACATATACTAAA |
U6 Reverse Primer | GCTTCACGAATTTGCGTGTCAT |
The miRNA and RBP binding site prediction of circRNAs
The circRNA-targeted miRNAs were predicted using 3 circRNA databases; that is, the Cancer-Specific CircRNA Database (CSCD) (34), circBank, and Circular RNA Interactome (35,36). The intersecting miRNAs were then selected for further analysis. The KEGG pathways of the miRNAs were predicted using DIANA-mirPath v.3 (37). The circRNA-miRNA-mRNA network was constructed using Cytoscape software (https://cytoscape.org/).
Statistical analysis
All the data were analyzed using the student’s t-test, and a P value <0.05 was considered statistically significant. GraphPad Prism 7.0 (GraphPad Software, Inc., La Jolla, CA, USA) and the R statistical programing language (3.3.3, R Development Core Team; https://cran.r-project.org/) were used to visualize the data.
Results
Summary of the sequencing data
The exRNAs were isolated from the plasma of the 6 NSCLC patients and 6 NCs, and next-generation RNA-sequencing (RNA-seq) was then performed to analyze the distribution of the exRNAs in the human circulatory system. There was an average clean read count of ~20 million for each sample, and about 50–80% reads aligned to the human genome v38 (hg38) using Hisat2 (http://ccb.jhu.edu/software/hisat2/index.shtml). There was an average of 10.89 thousand and 14.23 thousand transcripts in the NSCLC patients and NCs, respectively (with a P value of 0.51 between the NSCLC patients and NCs). There was an average of 0.80 million and 0.18 million aligned reads in the NSCLC patients and NCs, respectively (with a P value of 0.027 between the 2 groups).
In total, 4.82 and 1.12 million aligned reads were identified in the NSCLC and NCs, respectively, and the transcript reads were classified into 35 Ensembl transcript biotypes according to the annotation file of Ensembl biomart (the biotypes that had total reads of <10 were removed) (Figure 1A). The results showed that the read counts of the mitochondrial ribosomal RNAs (mt-rRNAs), mitochondrial transfer RNAs (mt-tRNAs), and protein coding were significantly increased in the plasma of the NSCLC patients compared to NC patients (Figure 1A,1B), but there were no significant differences between the NSCLC patients and NCs in the other transcript biotypes.
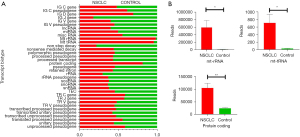
Data availability statement
The RNA-seq datasets are available at NCBI project PRJNA971084 (https://www.ncbi.nlm.nih.gov/, accessed on 16 May 2023).
ExRNA biotype distribution
To better show the transcript type of every individual, the reads of mt-rRNA, mt-tRNA, and other rRNAs were removed, and a donut graph was used to show the distribution of the transcript biotype of each individual. The results showed that the total expression of protein_coding and lncRNA accounted for 95% of the total transcripts after removing mt-rRNAs and mt-tRNAs (Figure 2A,2B). In addition, we also used a donut graph (Figure 2C) to characterize the proportion of each transcript type, and found that the transcript category of protein_coding and lncRNA accounted for >80% of the total transcripts, except mt-rRNA and mt-tRNA (Figure 2C).
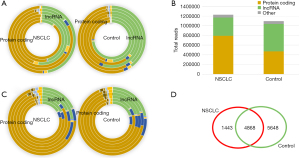
Among the protein_coding RNAs and lncRNAs, 1,324 transcript biotypes were expressed in more than 2/3 of the NSCLC samples, 2,684 transcript biotypes were expressed in more than 2/3 of the NC samples, and 726 transcript biotypes were shared among the 2 groups (Figure 2D).
GO and KEGG pathway analysis of the differentially expressed transcripts
We used DESeq2 package (https://bioconductor.org/packages/release/bioc/html/DESeq2.html) to identify the differentially expressed transcripts in the plasma of the NSCLC patients, Figure 3A showed the volcano plots of differential expression genes between NSCLC and normal controls, and we then used Metascape software (http://metascape.org/) to determine the enriched GO (Figure 3B) and KEGG terms (Figure 3C) of the differentially expressed transcripts. The results showed that the top significantly enriched GO terms included oxidative phosphorylation, proton transmembrane transport, and response to oxidative stress. The other GO terms, such as receptor tyrosine kinase binding and the regulation of the bone morphogenetic protein signaling pathway and notch signaling pathway, were related to cancer (Figure 3B). In addition, the significantly enriched KEGG terms included oxidative phosphorylation, and aminoacyl-transfer RNA biosynthesis (Figure 3C).
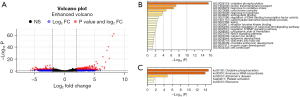
CircRNA distribution in plasma
We used CIRI software to predict the circRNAs from the RNA-seq data of 12 individuals, and 203 circRNAs with >3 reads were found in the 12 plasma samples (Figure 4A). However, most circRNAs were low in abundance, and only a few circRNAs were detected at a high number of reads (i.e., >50 reads), such as hsa_circ_0000722 and hsa_circ_0000437 (Figure 4B). Of these 203 circRNAs, 91% were annotated in the circBase (Figure 4C). In addition, most of the circRNAs were from chromosomes 1, 2, and 3, but the circRNAs with the most read counts were from chromosomes 1, 4, and 16 (Figure 4D).
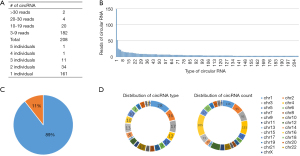
Hsa_circ_0000722 is upregulated in NSCLC plasma
According to the sequencing data, we validated the expression of 2 selected 2circRNAs in the plasma using qRT-PCR. According to the sequencing results, hsa_circ_0000722 was upregulated in the plasma of the NSCLC patients, and hsa_circ_0006156 was downregulated in the plasma of the NSCLC patients. The qRT-PCR results indicated that hsa_circ_0000722 was significantly more highly expressed in the NSCLC plasma than the control plasma (Figure 5A), but hsa_circ_0006156 did not differ significantly between the NSCLC plasma and the control plasma (Figure 5B).
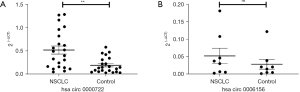
Hsa_circ_0000722- targeted miRNA pathway
We used 3 circRNA databases (i.e., CSCD, circBank, and Circular RNA Interactome) to predict the miRNAs targeted by hsa_circ_0000722, and the following 3 miRNAs were identified: miR-324-5p, miR-326, and miR-330-5p. According to the mirPath analysis (http://snf-515788.vm.okeanos.grnet.gr/), 14 KEGG pathways were identified, including fatty acid biosynthesis, proteoglycans in cancer, and central carbon metabolism in cancer (Figure 6A). The Cytoscape analysis (Figure 6B) showed the interaction of the genes related to the significant KEGG terms and miRNAs and circRNAs, and provided evidence that hsa_circ_0000722 and its predicted miRNAs were related to lung cancer.
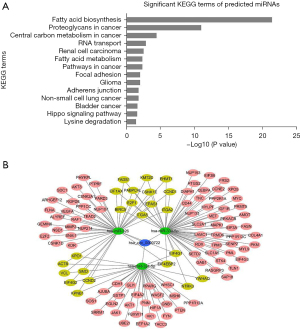
Hsa_miR_324-5p and hsa_miR_326 are upregulated in NSCLC plasma
Based on the circRNA-miRNA interaction analysis, we used qRT-PCR to validate the expression of miR-324-5p and miR-326 in the plasma of the lung cancer patients and NCs. The results showed that the expression of miR-324-5p (Figure 7A) and miR-326 (Figure 7B) were higher in the NSCLC plasma than the NC plasma.
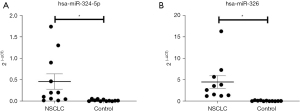
Discussion
In our study, we used RNA-seq to analyze the species of total RNAs in the plasma of NSCLC patients and NCs. According to previous studies, the amount of exRNA in 5–7 µL plasma is approximately 10 pg (12). Thus, there is <1 ng of RNA in 500 µL of plasma. Given the incompleteness and loss in RNA extraction, the actual obtained RNA was <0.4 ng, and we used the exRNA library preparation without rRNA depletion (38). The sequencing results showed that there was no significant difference in the number of transcript types between the NSCLC patients and NCs, but the transcript reads of the NSCLC plasma were significantly higher than those of the NCs, which indicates that some specific transcripts are highly expressed in NSCLC patients.
After the annotation of the Ensembl gene IDs, we found that the mt-rRNAs, mt-tRNAs, and protein_coding RNA transcripts were significantly upregulated in the plasma of the NSCLC patients. This phenomenon has also been reported in other studies, which was associated with mitochondria and diverse mechanism related to tumorigenesis (39,40), tumor anabolism, transcriptional regulation, and cell death (39). The mechanisms by which mitochondria participate in tumor formation include reactive oxygen species accumulation in mitochondria, the abnormal accumulation of some specific mitochondrial metabolites (e.g., fumarate and succinate), and the transition of mitochondrial membrane permeability (39,41). The high expression of mt-rRNA and mt-tRNA in the NSCLC patients was also the main reason for the significant difference in the aligned reads between the NSCLC patients and NCs. Additionally, the mt-RNAs, mt-tRNAs, and protein_coding RNAs differed significantly in terms of the number of reads and gene types between the NSCLC patients and NCs. According to the DESeq2 analysis, we found 103 differentially expressed protein-coding transcripts, and the top upregulated transcripts belonged to the mitochondria, which further proves that mitochondria play a key role in lung cancer.
To date, many studies have focused on the potential of circRNAs in the plasma as tumor biomarkers. However, only limited studies have examined the sequencing results of circRNAs in plasma. In this study, we used CIRI to identify 203 extracellular circRNAs, but the frequently predicted circRNAs were low in abundance, and the predicted circRNAs differed greatly between individuals. This is because exRNAs in plasma are low in abundance and fragmented (12,42), which makes it difficult to completely present an exRNA expression profile. Additionally, the source of exRNA in the plasma is complex, and the health status of individuals differ. Thus, the expression of exRNA between individuals differ, especially in those with a low abundance of these exRNAs.
In this study, we used qRT-PCR to validate the high expression of hsa_circ_0000722 in the plasma of NSCLC patients, and the silico analysis showed that hsa_circ_0000722 can function as sponges of miR-324-5p, miR-326, and miR-330-5p. These miRNAs may be involved in several pathways, including fatty acid biosynthesis, proteoglycans in cancer, central carbon metabolism in cancer, and focal adhesion. Previous studies have shown miR‑3245p is differentially expressed in various cancers (43,44), and miR‑3245p promotes cell proliferation and invasion in lung cancer cells (44). Further, previous studies have reported miR326 is downregulated in lung cancer (45); however, our research showed that miR‑326 was upregulated in the plasma of the NSCLC patients. The reason for this needs to be determined. In summary, we have identified NSCLC specific exRNA and preliminarily explained its possible mechanism, which not only provides further validation for previous studies, but also provides new translational targets for the development of these specific markers related to the diagnosis and treatment of NSCLC in the future.
Conclusions
We used RNA-seq technology to analyze the exRNA biotypes in the plasma of NSCLC patients and NCs. According to the bioinformatics analysis, we found mt-rRNAs, mt-tRNA, and multiple mitochondrial RNAs were upregulated in NSCLC. Additionally, hsa_circ_0000722 and hsa-miR-324-5p are potential biomarkers for NSCLC.
Acknowledgments
The authors would like to thank the Clinical Competency Enhancement Project and Top Talent Program of Jiangsu Province People’s Hospital for their support.
Funding: This work was supported by the Jiangsu Province Natural Science Foundation (No. BK20201492 to Jun Wang), the Key Medical Research Project of Jiangsu Provincial Health Commission (No. K2019002 to Jun Wang), the Clinical Capacity Improvement Project of Jiangsu Province People’s Hospital (No. JSPH-MA-2021-8 to Jun Wang) and the National Key Research and Development Program of China (No. 2022YFF0710800 to Qinyu Ge).
Footnote
Reporting Checklist: The authors have completed the MDAR reporting checklist. Available at https://jtd.amegroups.com/article/view/10.21037/jtd-23-517/rc
Data Sharing Statement: Available at https://jtd.amegroups.com/article/view/10.21037/jtd-23-517/dss
Peer Review File: Available at https://jtd.amegroups.com/article/view/10.21037/jtd-23-517/prf
Conflicts of Interest: All authors have completed the ICMJE uniform disclosure form (available at https://jtd.amegroups.com/article/view/10.21037/jtd-23-517/coif). JW reports that the study was supported by the Jiangsu Province Natural Science Foundation (No. BK20201492), the Key Medical Research Project of Jiangsu Provincial Health Commission (No. K2019002), and the Clinical Capacity Improvement Project of Jiangsu Province People’s Hospital (No. JSPH-MA-2021-8). QG reports that the study was supported by the National Key Research and Development Program of China (No. 2022YFF0710800). The other authors have no conflicts of interest to declare.
Ethical Statement: The authors are accountable for all aspects of the work in ensuring that questions related to the accuracy or integrity of any part of the work are appropriately investigated and resolved. The study was conducted in accordance with the Declaration of Helsinki (as revised in 2013). This protocol was approved by the Ethics Committee of Jiangsu Province People’s Hospital (No. 2019-SFRA-082), and written informed consent was obtained from each participant.
Open Access Statement: This is an Open Access article distributed in accordance with the Creative Commons Attribution-NonCommercial-NoDerivs 4.0 International License (CC BY-NC-ND 4.0), which permits the non-commercial replication and distribution of the article with the strict proviso that no changes or edits are made and the original work is properly cited (including links to both the formal publication through the relevant DOI and the license). See: https://creativecommons.org/licenses/by-nc-nd/4.0/.
References
- Mithoowani H, Febbraro M. Non-Small-Cell Lung Cancer in 2022: A Review for General Practitioners in Oncology. Curr Oncol 2022;29:1828-39. [Crossref] [PubMed]
- Di Maio M, Leighl NB, Gallo C, et al. Quality of life analysis of TORCH, a randomized trial testing first-line erlotinib followed by second-line cisplatin/gemcitabine chemotherapy in advanced non-small-cell lung cancer. J Thorac Oncol 2012;7:1830-44. [Crossref] [PubMed]
- Hong Y, Park S, Lee MK. The prognosis of non-small cell lung cancer patients according to endobronchial metastatic lesion. Sci Rep 2022;12:13588. [Crossref] [PubMed]
- Blandin Knight S, Crosbie PA, Balata H, et al. Progress and prospects of early detection in lung cancer. Open Biol 2017;7:170070. [Crossref] [PubMed]
- Song Z, Li Y, Chen S, et al. Efficacy and safety of pyrotinib in advanced lung adenocarcinoma with HER2 mutations: a multicenter, single-arm, phase II trial. BMC Med 2022;20:42. [Crossref] [PubMed]
- Rojas F, Parra ER, Wistuba II, et al. Pathological Response and Immune Biomarker Assessment in Non-Small-Cell Lung Carcinoma Receiving Neoadjuvant Immune Checkpoint Inhibitors. Cancers (Basel) 2022;14:2775. [Crossref] [PubMed]
- Tian F, Shen Y, Chen Z, et al. Aberrant miR-181b-5p and miR-486-5p expression in serum and tissue of non-small cell lung cancer. Gene 2016;591:338-43. [Crossref] [PubMed]
- de Rezende IM, Oliveira GFG, Costa TA, et al. Yellow Fever Molecular Diagnosis Using Urine Specimens during Acute and Convalescent Phases of the Disease. J Clin Microbiol 2022;60:e0025422. [Crossref] [PubMed]
- Chen D, Sun Q, Cheng X, et al. Genome-wide analysis of long noncoding RNA (lncRNA) expression in colorectal cancer tissues from patients with liver metastasis. Cancer Med 2016;5:1629-39. [Crossref] [PubMed]
- Li W, Wang H, Zheng Y. Circ_0001058 represses the progression of lung adenocarcinoma through governing of the miR-486-5p/TEK signaling axis. Anticancer Drugs 2022;33:710-9. [Crossref] [PubMed]
- Wang L, Wang J, Jia E, et al. Plasma RNA sequencing of extracellular RNAs reveals potential biomarkers for non-small cell lung cancer. Clin Biochem 2020;83:65-73. [Crossref] [PubMed]
- Bub A, Brenna S, Alawi M, et al. Multiplexed mRNA analysis of brain-derived extracellular vesicles upon experimental stroke in mice reveals increased mRNA content with potential relevance to inflammation and recovery processes. Cell Mol Life Sci 2022;79:329. [Crossref] [PubMed]
- Murthy VL, Yu B, Wang W, et al. Molecular Signature of Multisystem Cardiometabolic Stress and Its Association With Prognosis. JAMA Cardiol 2020;5:1144-53. [Crossref] [PubMed]
- Liu W, Zhang Y, Huang F, et al. The Polymorphism and Expression of EGFL7 and miR-126 Are Associated With NSCLC Susceptibility. Front Oncol 2022;12:772405. [Crossref] [PubMed]
- Mao Y, Sun Y, Xue J, et al. Ultra-sensitive and high efficiency detection of multiple non-small cell lung cancer-related miRNAs on a single test line in catalytic hairpin assembly-based SERS-LFA strip. Anal Chim Acta 2021;1178:338800. [Crossref] [PubMed]
- Gu X, Wang G, Shen H, et al. Hsa_circ_0033155: A potential novel biomarker for non-small cell lung cancer. Exp Ther Med 2018;16:3220-6. [Crossref] [PubMed]
- Du D, Cao X, Duan X, et al. Blocking circ_0014130 suppressed drug resistance and malignant behaviors of docetaxel resistance-acquired NSCLC cells via regulating miR-545-3p-YAP1 axis. Cytotechnology 2021;73:571-84. [Crossref] [PubMed]
- Max KEA, Wang VR, Chang MS, et al. Plasma microRNA Interindividual Variability in Healthy Individuals, Pregnant Women, and an Individual with a Stably Altered Neuroendocrine Phenotype. Clin Chem 2021;67:1676-88. [Crossref] [PubMed]
- Yan Z, Zhou Z, Wu Q, et al. Presymptomatic Increase of an Extracellular RNA in Blood Plasma Associates with the Development of Alzheimer's Disease. Curr Biol 2020;30:1771-1782.e3. [Crossref] [PubMed]
- Zhou WY, Cai ZR, Liu J, et al. Circular RNA: metabolism, functions and interactions with proteins. Mol Cancer 2020;19:172. [Crossref] [PubMed]
- Chen LL. The expanding regulatory mechanisms and cellular functions of circular RNAs. Nat Rev Mol Cell Biol 2020;21:475-90. [Crossref] [PubMed]
- Memczak S, Jens M, Elefsinioti A, et al. Circular RNAs are a large class of animal RNAs with regulatory potency. Nature 2013;495:333-8. [Crossref] [PubMed]
- Han B, Chao J, Yao H. Circular RNA and its mechanisms in disease: From the bench to the clinic. Pharmacol Ther 2018;187:31-44. [Crossref] [PubMed]
- Vakhshiteh F, Hassani S, Momenifar N, et al. Exosomal circRNAs: new players in colorectal cancer. Cancer Cell Int 2021;21:483. [Crossref] [PubMed]
- Zhuang ZG, Zhang JA, Luo HL, et al. The circular RNA of peripheral blood mononuclear cells: Hsa_circ_0005836 as a new diagnostic biomarker and therapeutic target of active pulmonary tuberculosis. Mol Immunol 2017;90:264-72. [Crossref] [PubMed]
- Guo W, Huai Q, Liu T, et al. Plasma extracellular vesicle long RNA profiling identifies a diagnostic signature for stage I lung adenocarcinoma. Transl Lung Cancer Res 2022;11:572-87. [Crossref] [PubMed]
- Liao Y, Smyth GK, Shi W. featureCounts: an efficient general purpose program for assigning sequence reads to genomic features. Bioinformatics 2014;30:923-30. [Crossref] [PubMed]
- Kinsella RJ, Kähäri A, Haider S, et al. Ensembl BioMarts: a hub for data retrieval across taxonomic space. Database (Oxford) 2011;2011:bar030. [Crossref] [PubMed]
- Love MI, Huber W, Anders S. Moderated estimation of fold change and dispersion for RNA-seq data with DESeq2. Genome Biol 2014;15:550. [Crossref] [PubMed]
- Kanehisa M, Furumichi M, Tanabe M, et al. KEGG: new perspectives on genomes, pathways, diseases and drugs. Nucleic Acids Res 2017;45:D353-61. [Crossref] [PubMed]
- Kanehisa M, Goto S. KEGG: kyoto encyclopedia of genes and genomes. Nucleic Acids Res 2000;28:27-30. [Crossref] [PubMed]
- Gao Y, Zhang J, Zhao F. Circular RNA identification based on multiple seed matching. Brief Bioinform 2018;19:803-10. [Crossref] [PubMed]
- Glažar P, Papavasileiou P, Rajewsky N. circBase: a database for circular RNAs. RNA 2014;20:1666-70. [Crossref] [PubMed]
- Xia S, Feng J, Chen K, et al. CSCD: a database for cancer-specific circular RNAs. Nucleic Acids Res 2018;46:D925-9. [Crossref] [PubMed]
- Liu M, Wang Q, Shen J, et al. Circbank: a comprehensive database for circRNA with standard nomenclature. RNA Biol 2019;16:899-905. [Crossref] [PubMed]
- Ali SA, Peffers MJ, Ormseth MJ, et al. The non-coding RNA interactome in joint health and disease. Nat Rev Rheumatol 2021;17:692-705. [Crossref] [PubMed]
- Vlachos IS, Zagganas K, Paraskevopoulou MD, et al. DIANA-miRPath v3.0: deciphering microRNA function with experimental support. Nucleic Acids Res 2015;43:W460-6. [Crossref] [PubMed]
- Phelps WA, Carlson AE, Lee MT. Optimized design of antisense oligomers for targeted rRNA depletion. Nucleic Acids Res 2021;49:e5. [Crossref] [PubMed]
- Zong WX, Rabinowitz JD, White E. Mitochondria and Cancer. Mol Cell 2016;61:667-76. [Crossref] [PubMed]
- Ribas de Pouplana L. The mitochondrial tRNA conundrum. Nat Rev Mol Cell Biol 2020;21:361. [Crossref] [PubMed]
- Liu Y, Shi Y. Mitochondria as a target in cancer treatment. MedComm (2020) 2020;1:129-39. [Crossref] [PubMed]
- Jia J, Yang S, Huang J, et al. Distinct Extracellular RNA Profiles in Different Plasma Components. Front Genet 2021;12:564780. [Crossref] [PubMed]
- Ghatak D, Datta A, Roychowdhury T, et al. MicroRNA-324-5p-CUEDC2 Axis Mediates Gain-of-Function Mutant p53-Driven Cancer Stemness. Mol Cancer Res 2021;19:1635-50. [Crossref] [PubMed]
- Wan Y, Luo H, Yang M, et al. miR-324-5p Contributes to Cell Proliferation and Apoptosis in Pancreatic Cancer by Targeting KLF3. Mol Ther Oncolytics 2020;18:432-42. [Crossref] [PubMed]
- Wang R, Xu J, Xu J, et al. MiR-326/Sp1/KLF3: A novel regulatory axis in lung cancer progression. Cell Prolif 2019;52:e12551. [Crossref] [PubMed]
(English Language Editor: L. Huleatt)