Identification of the potential ferroptosis key genes in lung cancer with bone metastasis
Highlight box
Key findings
• The differentially expressed ferroptosis-related genes and predicted microRNAs (miRNAs) regulatory network in lung cancer bone metastasis, as well as the related functional enrichment analysis, provide new targets for the treatment of lung cancer bone metastasis.
What is known and what is new?
• Ferroptosis has been linked to tumor formation and progression.
• Using data mining and analytic tools, we screened DEGs for differences in gene expression between lung cancer and normal lung tissues in the presence of bone metastases.
What are the implications, and what should change now?
• Our results aided in elucidating the status of ferroptosis after lung cancer bone metastases and provided novel insights into the clinical diagnosis and treatment of lung cancer bone metastases.
Introduction
At present, most deaths worldwide are caused by non-communicable diseases, and cancer is predicted to be the only disease that hinders the increase of human life expectancy in the 21st century (1). The onset of lung cancer has no obvious symptoms; its progression is fast and the prognosis is poor. The 5-year survival rate for lung cancer patients in the United States is around 16% and less than 10% in the United Kingdom. Lung cancer has become one of the deadliest malignant tumors globally (2,3). In China, the incidence (21.9%) and mortality (26.4%) rates of lung cancer rank first among all malignancies in male patients, while among female cancer patients, its incidence rate (13.3%) ranks second only to breast cancer, and its mortality rate (11.1%) ranks first (4). Thus, lung cancer has become a major public health problem facing the development of our society. It is prone to distant metastasis in the advanced stage, and bone is one of the common target organs of hematogenous metastasis (5,6). Among the types of lung cancer, lung adenocarcinoma has the highest incidence of bone metastasis, mainly osteolytic destruction, and is prone to secondary bone-related events, which seriously affect the daily life and survival time of patients (7). The treatment of lung cancer with bone metastasis (LCBM) still faces serious challenges. Searching for differential genes of this disease and molecular markers for its diagnosis will help elucidate the relevant molecular mechanisms of LCBM and promote gene-targeted therapy.
Previous studies have also demonstrated the involvement of programmed cell death in the occurrence of LCBM (8,9). Ferroptosis resembles programmed cell death and is characterized by the generation and accumulation of iron-dependent lipid reactive oxygen species (10). It has also been linked to tumor formation and progression in several studies; for instance, Levistilide A causes ferroptosis in breast cancer cells by stimulating the Nrf2/HO-1 (nuclear factor erythroid 2-related factor 2/heme oxygenase-1) signaling pathway (11); iron oxide nanoparticles loaded with paclitaxel through the enhanced autophagy-dependent ferroptosis pathway to inhibit glioblastoma (12); ropivacaine inhibits ovarian cancer cell stem cells and promotes cell death by inactivating PI3K/AKT (phosphatidylinositol 3'-kinase/AKT serine/threonine kinase) signaling pathway (13); TIGAR (TP53 induced glycolysis regulatory phosphatase) inhibits ovarian cancer cell stem cells via the ROS/AMPK/SCD1 (ROS proto-oncogene/activated protein kinase/stearyl coenzyme A desaturated enzyme 1) pathway, which drives ferroptosis resistance in colorectal cancer (14). TXNIP as a potential biomarker for predicting the prognosis and efficacy of chemotherapy combined with immunotherapy in SCLC patients (15).
At present, the mechanism of iron death in bone metastasis of lung cancer by gluconeogenesis is not clear, and there is also a lack of relevant bioinformatics research. We use data mining and analysis techniques to screen differentially expressed genes (DEGs) in lung cancer tissue with bone metastasis and normal lung tissue. Then intersect these DEGs with the iron death dataset to obtain the DEGs related to iron death. In addition, in order to identify key biomarkers and establish the pathogenesis of lung cancer bone metastasis at the molecular level, we investigated key miRNAs that may play a major role in lung cancer bone metastasis. Our research results will help to understand the iron death state after bone metastasis in lung cancer, and provide new ideas for the clinical diagnosis and treatment of bone metastasis in lung cancer. We present this article in accordance with the STREGA reporting checklist (available at https://jtd.amegroups.com/article/view/10.21037/jtd-23-539/rc).
Methods
Gene Expression Omnibus (GEO) data analysis
We downloaded the GSE10799 RNA expression dataset (contain tumor tissues and normal tissues with LCBM) from the GEO database (https://www.ncbi.nlm.nih.gov/geo/query/acc.cgi) using the GEO query package. Probes corresponding to more than one molecule were discarded, while probes corresponding to the same molecule were collected; only the probe with the largest signal value was retained.
Ferroptosis data analysis
The relevant ferroptosis dataset was downloaded from the database of ferroptosis (http://www.zhounan.org/ferrdb), which contains 259 genes. The annotation of these genes revealed 111 gene markers, 69 repressor genes and 108 driver genes (16).
Differential expression analysis
GEO2R is an online analytical tool for differential expression analysis. P values and adjusted P values in the DEGs analyses were determined using t-tests. The following criteria were used to choose genes conserved across tissues: |log2(fold-change)| >0.5 and an adjusted P value <0.05. To further our understanding of the DEGs related to ferroptosis, we collected a dataset that intersected GSE10799 with a ferroptosis database. A Venn diagram and heat map of the DEGs were created using the Venny2.1 online tool and the Heml program (https://bioinfogp.cnb.csic.es/tools/venny/), respectively.
Gene Ontology (GO) and Kyoto Encyclopedia of Genes and Genomes (KEGG) pathway enrichment analyses and gene set enrichment analysis (GSEA)
GO and KEGG analyses were used to explore the biological functions of DEGs in LCBM. GO analysis is a powerful bioinformatics tool for identifying biological processes (BPs), cellular components (CCs), and molecular functions (MFs) associated with DEGs. In addition, the biological pathways of miRNAs were analyzed using the Funrich enrichment analysis tool. Differences were considered statistically significant when P<0.05.
Protein-protein interaction (PPI) network analysis
The interactions between these proteins were collected from the STRING database to predict the PPI. In addition, Cytoscape v3.6.0 (https://cytoscape.org/release_notes_3_6_0.html) was used to generate and display the PPI network. A cluster analysis of gene networks was performed using Molecular Combinatorial Detection (MCODE) to identify significant PPI network components. The purpose of MCODE is to select the essential sub-networks or modules. In a PPI network, a module indicates a function. Various genes in a module have different module scores, and key genes may be selected based on the scores. When identifying the key modules, P<0.05 was considered to indicate significant variations.
Functional enrichment analysis of targeted miRNAs for genes in key modules
We used the miEAA database (https://ccb-compute2.cs.uni-saarland.de/mieaa2/) to perform GO and KEGG enrichment analyses on the previously predicted micro RNAs (miRNAs) and used the enriched results as functional annotations of miRNAs.
Clinical data of lung cancer patients
A retrospective analysis was conducted from January 2018 to October 2021 on histopathologically confirmed lung cancer cases (bronchoscopy biopsy, percutaneous lung biopsy, and thoracoscopic biopsy) at the Affiliated Cancer Hospital of Nanjing Medical University. A total of 105 patients received standardized comprehensive treatment, including 69 males and 36 females [aged 45–69 (57.96±3.21) years old]. The patients’ Eastern Cooperative Oncology Group (ECOG) scores were 0 points in 34 cases, 1 point in 50 cases, and 2 points in 21 cases. Among these patients, there were 59 cases of adenocarcinoma, 30 cases of squamous cell carcinoma, and 16 cases of small cell lung cancer (SCLC). We reviewed the patients’ case histories and excluded the following situations: (I) patients who received glucocorticoid, bisphosphonate, and calcium therapy within 3 months before diagnosis; (II) combined with endocrine and metabolic diseases, such as diabetes, gout, osteoporosis, thyroid disease, etc.; (III) combined with other serious lung diseases, such as uncontrolled asthma, severe chronic obstructive pulmonary disease, active tuberculosis, etc.; (IV) rheumatoid arthritis, ankylosing spondylitis, and other rheumatoid arthritis cases or a history of traumatic fracture within 5 years; (V) severe liver, kidney, or heart dysfunction; (VI) incomplete clinical data.
The study was conducted in accordance with the Declaration of Helsinki (as revised in 2013). The study was approved by ethics board of the Affiliated Cancer Hospital of Nanjing Medical University (No. 2020-129) and informed consent was taken from all the patients.
Statistical analysis
GraphPad Prism 7.0 software (https://www.graphpad-prism.cn/)was used to draw graphs and conduct statistical analysis. All data are shown as mean ± standard deviation (SD). In the DEG analysis, t-tests were used to determine P values and adjusted P values, where P values were adjusted by the false discovery rate (FDR). P<0.05 indicated that the difference was statistically significant. Logistic regression analysis was performed to assess the relationship between serum alkaline phosphatase (ALP), neuron-specific enolase (NSE), and bone metastasis in lung cancer patients. A receiver operating characteristic (ROC) curve was drawn to test the value of serum ALP and NSE in evaluating bone metastasis in lung cancer patients, and in the area under the curve (AUC) evaluation, an AUC >0.90 denoted a higher evaluation value, 0.70< AUC ≤0.90 signified medium evaluation value, 0.50< AUC ≤0.70 reflected a lower evaluation value, and AUC ≤0.50 represented no evaluation value. The inspection level was set as α=0.05.
Results
Gene-miRNA interaction network
We used miRWalk 2.0 (http://mirwalk.umm.uni-heidelberg.de/)to predict the target key miRNAs and construct related gene-miRNA interaction networks. We intersected the predictions from the MiRTarBase and miRWalk databases to ensure the accuracy of the results. Moreover, we also screened for miRNAs targeting more than two genes.
GO and KEGG enrichment analyses
Next, we performed functional enrichment analysis to assess the underlying molecular mechanisms of DEGs in the occurrence and development of lung cancer bone metastasis (Figure 1). The GO and KEGG enrichment analyses suggested that these genes may affect the oxidative stress response, hypoxia response, rough endoplasmic reticulum, mitochondrial outer membrane, iron-sulfur cluster binding, virus receptor activity, cancer central carbon metabolism, the interleukin-17 (IL-17) signaling pathway, and other aspects involved in the occurrence and development of LCBM (Figure 2). The detailed results are shown in Table 1.
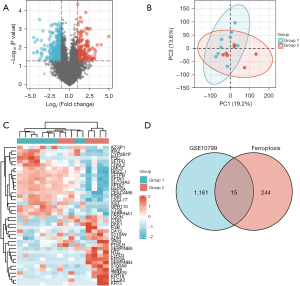
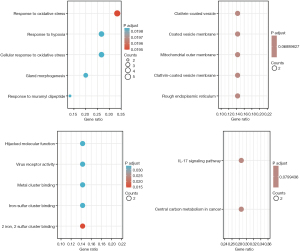
Table 1
Ontology | ID | Description | Gene ratio | Bg ratio | P value | Adjust P value | q value |
---|---|---|---|---|---|---|---|
BP | GO:0006979 | Response to oxidative stress | 5/15 | 451/18,670 | 1.98e-05 | 0.019 | 0.011 |
BP | GO:0034599 | Cellular response to oxidative stress | 4/15 | 302/18,670 | 7.96e-05 | 0.020 | 0.011 |
BP | GO:0022612 | Gland morphogenesis | 3/15 | 120/18,670 | 1.11e-04 | 0.020 | 0.011 |
BP | GO:0032495 | Response to muramyl dipeptide | 2/15 | 20/18,670 | 1.14e-04 | 0.020 | 0.011 |
BP | GO:0001666 | Response to hypoxia | 4/15 | 359/18,670 | 1.55e-04 | 0.020 | 0.011 |
CC | GO:0005791 | Rough endoplasmic reticulum | 2/14 | 85/19,717 | 0.002 | 0.069 | 0.033 |
CC | GO:0030665 | Clathrin-coated vesicle membrane | 2/14 | 115/19,717 | 0.003 | 0.069 | 0.033 |
CC | GO:0005741 | Mitochondrial outer membrane | 2/14 | 178/19,717 | 0.007 | 0.069 | 0.033 |
CC | GO:0030662 | Coated vesicle membrane | 2/14 | 182/19,717 | 0.007 | 0.069 | 0.033 |
CC | GO:0030136 | Clathrin-coated vesicle | 2/14 | 188/19,717 | 0.008 | 0.069 | 0.033 |
MF | GO:0051537 | 2 iron, 2 sulfur cluster binding | 2/14 | 22/17,697 | 1.33e-04 | 0.015 | 0.008 |
MF | GO:0051536 | Iron-sulfur cluster binding | 2/14 | 63/17,697 | 0.001 | 0.033 | 0.017 |
MF | GO:0051540 | Metal cluster binding | 2/14 | 63/17,697 | 0.001 | 0.033 | 0.017 |
MF | GO:0001618 | Virus receptor activity | 2/14 | 74/17,697 | 0.002 | 0.033 | 0.017 |
MF | GO:0104005 | Hijacked molecular function | 2/14 | 74/17,697 | 0.002 | 0.033 | 0.017 |
KEGG | hsa05230 | Central carbon metabolism in cancer | 2/7 | 70/8,076 | 0.002 | 0.080 | 0.070 |
KEGG | hsa04657 | IL-17 signaling pathway | 2/7 | 94/8,076 | 0.003 | 0.080 | 0.070 |
P<0.05, statistically significant difference. GO, Gene Ontology; KEGG, Kyoto Encyclopedia of Genes and Genomes; DEGs, differentially expressed genes; BP, biological processes; CC, cellular components; MF, molecular functions.
PPI network analysis of ferroptosis-associated DEGs in LCBM
We used the STRING online database’s default cut-off (interaction score >0.4) to generate a network graph with 13 nodes. The nodes in this network were represented by genes, and the connections between the genes were denoted by the edges. Upregulated genes were marked in red, while downregulated genes were marked in blue. Cytoscape’s MCODE is an application for essential module mapping through cluster analysis of the gene networks. A key module was established, and a total of four key genes were screened, namely phosphatidylethanolamine binding protein 1 (PEBP1), isocitrate dehydrogenase [NADP(+)] 1 (IDH1), ELAV like RNA binding protein 1 (ELAVL1), and epidermal growth factor receptor (EGFR) (Figure 3).
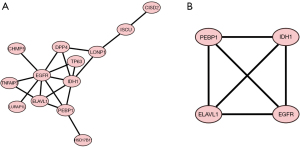
miRNA interactions and mining
A gene-miRNA analysis was performed using miRWalk 2.0 software on the four previously screened key genes. To ensure the accuracy and consistency of our findings, the miRWalk and miRTarBase databases were employed to select cross-linked miRNAs. The following criteria were used to filter the results: P<0.05; seed sequence length >7; and 3' UTR (untranslated region) as a binding region for the target gene. These regulatory networks may contribute to the occurrence and progression of LCBM (Figure 4).
Functional enrichment analysis of genes targeting miRNAs in key modules
We conducted functional enrichment analysis on the predicted miRNA in the miEAA database, and constructed word cloud maps and enrichment heat maps to demonstrate the possible pathways involved. We found that these miRNAs may affect the Hippo signaling pathway, the AMPK (adenosine 5’-monophosphate (AMP)-activated protein kinase)signaling pathway, arginine biosynthesis, the IL-17 signaling pathway, pyruvate metabolism, the GnRH (gonadotrophin-releasing hormone) signaling pathway, autophagy, cytokine-cytokine receptor interaction, the ErbB (erythroblastic leukemia viral oncogene homolog) signaling pathway, RNA degradation, pyrimidine metabolism, apoptosis, the JAK-STAT (Janus kinase-signal transducer and activator of transcription)signaling pathway, the Toll-like receptor signaling pathway, the mitogen-activated protein kinase (MAPK) signaling pathway, the p53 signaling pathway, and other aspects, and are responsible for the occurrence and development of LCBM (Figure 5).
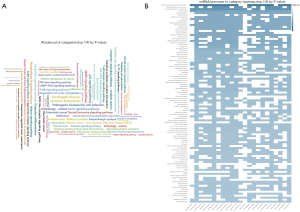
General status of lung cancer patients
After evaluation, the results showed that 39 of the 105 included lung cancer patients had bone metastases, with an incidence rate of 37.14%. The proportion of patients with an ECOG score of 2 in the bone metastasis group was higher than that in the non-bone metastasis group, and the serum ALP and NSE levels in this group were higher than those in the non-bone metastasis group (P<0.05). Also, there were no significant differences between the two groups in terms of gender, age, and pathological type (P>0.05, Table 2 for details).
Table 2
Clinical feature | Bone metastasis (n=39) | Non-bone metastasis (n=66) | χ2 | P |
---|---|---|---|---|
Gender | 1.018 | 0.313 | ||
Male | 28 (71.79) | 41 (62.12) | ||
Female | 11 (28.21) | 25 (37.88) | ||
Age (years) | 57.85±3.48 | 58.03±3.29 | 0.265 | 0.791 |
Pathological feature | 0.486 | 0.784 | ||
Adenocarcinoma | 22 (56.41) | 37 (56.06) | ||
Squamous cell carcinoma | 10 (25.64) | 20 (30.30) | ||
Small cell lung cancer | 7 (17.95) | 9 (13.64) | ||
ECOG score | 4.171 | <0.001 | ||
0 | 5 (12.82) | 26 (39.39) | ||
1 | 16 (41.03) | 33 (50.00) | ||
2 | 18 (20.51) | 7 (10.61) | ||
Serum ALP (U/L) | 254.73±15.24 | 235.50±12.45 | 7.029 | <0.001 |
Serum NSE (μg/L) | 16.62±3.18 | 13.26±2.83 | 5.599 | <0.001 |
Data are shown as mean ± SD or n (%). ECOG, Eastern Cooperative Oncology Group; ALP, alkaline phosphatase; NSE, neuron-specific enolase.
Logistic regression analysis of serum ALP, NSE, and bone metastasis in patients with lung cancer
The variables with statistically significant differences in Table 2 were included as independent variables (ECOG score is a categorical variable, assigned as 0=1, 1=2, 2=3; the remaining variables were continuous variables). The occurrence of bone metastasis in patients with lung cancer was used as the dependent variable (bone metastasis =1, non-bone metastasis =0). After logistic regression analysis, the results showed that a high ECOG score and serum ALP and NSE overexpression were related to bone metastasis in patients with lung cancer (P<0.05, Table 3).
Table 3
Index | β | SE | Wald χ2 | P | OR | 95% CI |
---|---|---|---|---|---|---|
ECOG score | ||||||
1 | 0.546 | 0.706 | 0.598 | 0.439 | 1.725 | 0.433–6.879 |
2 | 1.869 | 0.866 | 4.654 | 0.031 | 6.483 | 1.187–35.417 |
Serum ALP | 0.092 | 0.024 | 15.007 | <0.001 | 1.096 | 1.046–1.148 |
Serum NSE | 0.349 | 0.108 | 10.397 | 0.001 | 1.418 | 1.147–1.754 |
Constant | −28.838 | 6.243 | 21.341 | <0.001 |
ECOG, Eastern Cooperative Oncology Group; ALP, alkaline phosphatase; NSE, neuron-specific enolase; SE, standard error; OR, odd ratio; CI, confidence interval.
Efficiency analysis of serum ALP and NSE for evaluating bone metastasis in patients with lung cancer
The levels of serum ALP and NSE in patients with lung cancer before treatment were used as the test variables, the occurrence of bone metastasis was used as a state variable (bone metastasis =1, non-bone metastasis =0), and a ROC curve was drawn. The AUCs of the bone metastasis risk in lung cancer patients were all >0.70, the evaluation value was ideal, and the joint evaluation value was optimal. Specificity and sensitivity were measured when the cut-off values of serum ALP and NSE were 237.790 U/L and 13.425 µg/L, respectively (Table 4 for details).
Table 4
Variable | AUC | 95% CI | P | Cut off | Specificity | Sensitivity | Youden index |
---|---|---|---|---|---|---|---|
ALP | 0.834 | 0.758–0.910 | <0.001 | 242.290 | 0.697 | 0.744 | 0.441 |
NSE | 0.769 | 0.677–0.861 | <0.001 | 14.640 | 0.667 | 0.718 | 0.385 |
Combined | 0.894 | 0.831–0.956 | <0.001 | 0.382 | 0.848 | 0.821 | 0.669 |
ALP, alkaline phosphatase; NSE, neuron-specific enolase; AUC, area under the curve; CI, confidence interval.
Discussion
The morbidity and mortality rates of lung cancer, the most frequent malignant tumor, continue to rise. Distant metastasis is the leading cause of mortality in people with lung cancer and is present in a high percentage of patients at the time of diagnosis (17). The skeletal system is a predilection site for distant lung cancer metastasis (18). A study has shown that the incidence of LCBM is about 10–15%, while the incidence of bone metastasis in advanced lung cancer is as high as 30–40% (19). After lung cancer cells reach the bone marrow through the blood and lymphatic system, they begin to release soluble mediators to activate osteoblasts and osteoclasts. Among these, osteoclasts will release cytokines, which in turn promote cancer cells to secrete soluble mediators, forming a vicious circle that further allows cancer cells to continue to proliferate in bone tissue, and eventually form metastases (20). Tumor markers have an important reference value for the cancer patients’ early diagnosis, formulation of a treatment plan, prognostic assessment, recurrence monitoring, etc. Therefore, finding effective tumor markers is an important way of improving the survival rates of lung cancer patients with bone metastases.
Numerous physiological and pathological processes, including development, aging, immunity, and cancer, depend on ferroptosis, which is a unique kind of programmed cell death reliant on iron ions and triggered by lipid peroxidation. Iron, lipids, and reactive oxygen species all play crucial roles in cell survival (21); these three work together to maintain regular physiological processes while metabolism is functioning normally but deliver a lethal blow to cells when metabolic abnormalities arise. Antioxidant, iron, and lipid abnormalities are at the root of the complex biological processes that induce ferroptosis (22). Recent study has examined the biological events of ferroptosis in tumor metastasis. For instance, cell ferroptosis is suppressed in osteosarcoma by recombinant lysine specific demethylase 4A (KDM4A)-mediated demethylation of the solute carrier family 7 member 11 (SLC7A11) histone, which deepens the response to osteosarcoma, chemoresistance, and understanding of epigenetic regulatory mechanisms in metastasis (23). Also, inhibiting ferroptosis and promoting distant metastasis of nasopharyngeal cancer by upregulating integrin subunit beta 3 (ITGB3) are two effects of platelet-derived extracellular vesicles (24). Moreover, dysregulation of cholesterol homeostasis leads to resistance to ferroptosis, increasing cancer tumorigenicity and metastasis (25). In clear cell renal cell carcinoma, KLF transcription factor 2 (KLF2) regulates ferroptosis through glutathione peroxidase 4 (GPX4) to prevent cancer cell invasion and migration (26). At the same time, there are many related studies on ferroptosis in lung cancer. For example, the sensitivity of human non-small cell lung carcinoma (NSCLC) cells to cystine deprivation-induced ferroptosis is controlled by NFE2 like BZIP transcription factor 2 (NRF2) through the FOCAD-focal adhesion kinase (FAK) signaling pathway (27). Ferroptosis is also inhibited and cancer invasion is facilitated by the tumor suppressor, ACSL4 (acyl-CoA synthetase long chain family member 4) (28).
Genes involved in ferroptosis have been shown to play an important role in LCBM, and some of these were discovered for the first time in the present research. These novel genes provide a fresh perspective on ferroptosis in LCBM and reveal novel treatment targets for this condition. Finally, we analyzed the clinical data of lung cancer patients. This study showed that bone metastases occurred in 39 of the 105 included lung cancer patients, with an incidence rate of 37.14%. We found that the bone metastasis risk in patients with lung cancer is high, and early assessment of the risk of bone metastasis is crucial. At present, imaging methods such as emission computed tomography (ECT), computed tomography (CT), and nuclear magnetic resonance imaging (MRI) are mainly used for the clinical evaluation of LCBM. A bone biopsy can be utilized to further clarify only isolated bone destruction lesions. However, the signs and symptoms of LCBM in most patients appear in the late stage of bone metastases. Imaging examinations are expensive and cannot be effectively identified in the early stage. Bone biopsy is traumatic and cannot dynamically monitor changes in bone metastases, so its application is limited. Therefore, to assess the risk of bone metastasis early and dynamically, it is necessary to explore the indicators related to bone metastasis in patients with lung cancer.
A study has shown that under normal physiological conditions, bone formation and bone resorption are balanced processes, which are mediated by osteoblasts and osteoclasts, respectively. When a malignant tumor metastasizes to bone, it can accelerate the bone remodeling process and lead to bone metabolism. While the bone metabolism rate increases, the normal bone metabolism mechanism will be affected, which then induces the generation of abnormal bone metabolism markers (6). ALP, mainly derived from tissues such as the liver and bone, is an important indicator reflecting bone metabolism. The overexpression of serum ALP indicates that the activity of osteoblasts increases and the mechanism of bone metabolism is abnormal (29). NSE is a kind of enolase that is involved in the glycolysis pathway, and its expression is significantly increased in tumors with neuroendocrine function or those derived from neuroectodermal tissue (30). According to reports, the level of NSE in the peripheral blood of patients with advanced lung cancer was significantly increased, and gradually decreased after treatment (31). Therefore, it is speculated that the abnormal expression of serum ALP and NSE may be related to bone metastasis in patients with lung cancer.
The logistic regression analysis results in this study showed that overexpression of serum ALP and NSE was related to LCBM. It was also preliminarily confirmed that the abnormal expression of serum ALP and NSE might be related to bone metastasis in patients with lung cancer. This may be because, in the process of osteogenesis, ALP can provide the necessary phosphoric acid and enter the blood circulation under the action of polysaccharide-inositol phosphate-specific hydrolase. When the expression of serum ALP in patients with lung cancer is significantly increased, bone formation is accelerated, the bone metabolism mechanism is abnormal, and the risk of bone metastasis is high. NSE mainly exists in nerve tissue and neuroendocrine cells. The tumor cells of patients with SCLC bone metastases exhibit the characteristics of neuroendocrine cells and can produce NSE. A study (32) has reported that some non-small cell lung cancers also have neuroendocrine functions, often accompanied by NSE secretion. In addition, in the process of LCBM, tumor cell proliferation is accelerated and glycolysis is enhanced, and NSE participates in the glycolysis pathway. Therefore, when bone metastasis occurs, NSE levels in the tumor cells of lung cancer patients increase, and the release into peripheral blood also increases. Furthermore, serum testing shows overexpression.
To verify our hypothesis, a ROC curve was drawn upon completion of our study. The results showed that the AUCs of serum ALP and NSE (alone and combined) in assessing the bone metastasis risk of patients with lung cancer were >0.70, and the evaluation value was the highest when the cut-off values of serum ALP and NSE were 237.790 U/L, 13.425 µg/L, further confirming that the abnormal expression of serum ALP and NSE is related to LCBM and can be used as an effective indicator for evaluating bone metastasis. However, whether serum ALP and NSE can be used as specific markers for LCBM still needs to be confirmed by further research in the future. In summary, the overexpression of serum ALP and NSE is related to LCBM, indicating a higher risk of bone metastasis in lung cancer patients.
Conclusions
In summary, this study utilizes bioinformatics analysis to identify potential key genes involved in ferroptosis in the pathogenesis of LCBM, thereby providing new targets for the treatment of LCBM. At the same time, based on the analysis of clinical data of cancer patients, further explore the relevant evaluation indicators of LCBM from a serological perspective, providing some reference value for clinical treatment.
Acknowledgments
Funding: This work was supported by the Program of Jiangsu Cancer Hospital (Nos. ZM201917, ZH202216)), the Open Project of Jiangsu Key Laboratory of Anesthesiology (No. XZSYSKF2022037), the National Natural Science Foundation of China (No. 82002434), the Talents Program of Jiangsu Cancer Hospital (No. YC201805), and the Jiangsu Province Science and Technology Coordination Research Project (No. JSKXKT2023040).
Footnote
Reporting Checklist: The authors have completed the STREGA reporting checklist. Available at https://jtd.amegroups.com/article/view/10.21037/jtd-23-539/rc
Data Sharing Statement: Available at https://jtd.amegroups.com/article/view/10.21037/jtd-23-539/dss
Peer Review File: Available at https://jtd.amegroups.com/article/view/10.21037/jtd-23-539/prf
Conflicts of Interest: All authors have completed the ICMJE uniform disclosure form (available at https://jtd.amegroups.com/article/view/10.21037/jtd-23-539/coif). The authors have no conflicts of interest to declare.
Ethical Statement: The authors are accountable for all aspects of the work in ensuring that questions related to the accuracy or integrity of any part of the work are appropriately investigated and resolved. The study was conducted in accordance with the Declaration of Helsinki (as revised in 2013). The study was approved by ethics board of the Affiliated Cancer Hospital of Nanjing Medical University (No. 2020-129) and informed consent was taken from all the patients.
Open Access Statement: This is an Open Access article distributed in accordance with the Creative Commons Attribution-NonCommercial-NoDerivs 4.0 International License (CC BY-NC-ND 4.0), which permits the non-commercial replication and distribution of the article with the strict proviso that no changes or edits are made and the original work is properly cited (including links to both the formal publication through the relevant DOI and the license). See: https://creativecommons.org/licenses/by-nc-nd/4.0/.
References
- Siegel RL, Miller KD, Jemal A. Cancer statistics, 2018. CA Cancer J Clin 2018;68:7-30. [Crossref] [PubMed]
- Liu X, Yu Y, Wang M, et al. The mortality of lung cancer attributable to smoking among adults in China and the United States during 1990-2017. Cancer Commun (Lond) 2020;40:611-9. [Crossref] [PubMed]
- Kim D, Lee JW. Current Status of Lung Cancer and Surgery Based on Studies Using a Nationwide Database. J Chest Surg 2022;55:1-9. [Crossref] [PubMed]
- Feng RM, Zong YN, Cao SM, et al. Current cancer situation in China: good or bad news from the 2018 Global Cancer Statistics? Cancer Commun (Lond) 2019;39:22. [Crossref] [PubMed]
- Yao Y, Zhou Y, Yang Z, et al. Risk Factors of Non-small Cell Lung Cancer with Bone Metastasis after Therapy. Zhongguo Fei Ai Za Zhi 2018;21:476-80. [PubMed]
- Teng X, Wei L, Han L, et al. Establishment of a serological molecular model for the early diagnosis and progression monitoring of bone metastasis in lung cancer. BMC Cancer 2020;20:562. [Crossref] [PubMed]
- Xu Y, Wu H, Wang C, et al. Case report: A balance of survival and quality of life in long-term survival case of lung adenocarcinoma with synchronous bone metastasis. Front Oncol 2022;12:1045458. [Crossref] [PubMed]
- Han L, Huang Z, Liu Y, et al. MicroRNA-106a regulates autophagy-related cell death and EMT by targeting TP53INP1 in lung cancer with bone metastasis. Cell Death Dis 2021;12:1037. [Crossref] [PubMed]
- Muguruma H, Yano S, Kakiuchi S, et al. Reveromycin A inhibits osteolytic bone metastasis of small-cell lung cancer cells, SBC-5, through an antiosteoclastic activity. Clin Cancer Res 2005;11:8822-8. [Crossref] [PubMed]
- Dixon SJ, Lemberg KM, Lamprecht MR, et al. Ferroptosis: an iron-dependent form of nonapoptotic cell death. Cell 2012;149:1060-72. [Crossref] [PubMed]
- Jing S, Lu Y, Zhang J, et al. Levistilide a Induces Ferroptosis by Activating the Nrf2/HO-1 Signaling Pathway in Breast Cancer Cells. Drug Des Devel Ther 2022;16:2981-93. [Crossref] [PubMed]
- Chen H, Wen J. Iron oxide nanoparticles loaded with paclitaxel inhibits glioblastoma by enhancing autophagy-dependent ferroptosis pathway. Eur J Pharmacol 2022;921:174860. [Crossref] [PubMed]
- Lu Y, Mao J, Xu Y, et al. Ropivacaine represses the ovarian cancer cell stemness and facilitates cell ferroptosis through inactivating the PI3K/AKT signaling pathway. Hum Exp Toxicol 2022;41:9603271221120652. [Crossref] [PubMed]
- Liu MY, Li HM, Wang XY, et al. TIGAR drives colorectal cancer ferroptosis resistance through ROS/AMPK/SCD1 pathway. Free Radic Biol Med 2022;182:219-31. [Crossref] [PubMed]
- Li S, Qiu G, Wu J, et al. Identification and validation of a ferroptosis-related prognostic risk-scoring model and key genes in small cell lung cancer. Transl Lung Cancer Res 2022;11:1380-93. [Crossref] [PubMed]
- Zhou N, Bao J. FerrDb: a manually curated resource for regulators and markers of ferroptosis and ferroptosis-disease associations. Database (Oxford) 2020;2020:baaa021. [Crossref] [PubMed]
- Altorki NK, Markowitz GJ, Gao D, et al. The lung microenvironment: an important regulator of tumour growth and metastasis. Nat Rev Cancer 2019;19:9-31. [Crossref] [PubMed]
- Akoury E, Ramirez Garcia Luna AS, Ahangar P, et al. Anti-Tumor Effects of Low Dose Zoledronate on Lung Cancer-Induced Spine Metastasis. J Clin Med 2019;8:1212. [Crossref] [PubMed]
- Hernandez RK, Wade SW, Reich A, et al. Incidence of bone metastases in patients with solid tumors: analysis of oncology electronic medical records in the United States. BMC Cancer 2018;18:44. [Crossref] [PubMed]
- Sathiakumar N, Delzell E, Yun H, et al. Accuracy of Medicare Claim-based Algorithm to Detect Breast, Prostate, or Lung Cancer Bone Metastases. Med Care 2017;55:e144-9. [Crossref] [PubMed]
- Shan X, Li S, Sun B, et al. Ferroptosis-driven nanotherapeutics for cancer treatment. J Control Release 2020;319:322-32. [Crossref] [PubMed]
- Zhao L, Zhou X, Xie F, et al. Ferroptosis in cancer and cancer immunotherapy. Cancer Commun (Lond) 2022;42:88-116. [Crossref] [PubMed]
- Chen M, Jiang Y, Sun Y. KDM4A-mediated histone demethylation of SLC7A11 inhibits cell ferroptosis in osteosarcoma. Biochem Biophys Res Commun 2021;550:77-83. [Crossref] [PubMed]
- Li F, Xu T, Chen P, et al. Platelet-derived extracellular vesicles inhibit ferroptosis and promote distant metastasis of nasopharyngeal carcinoma by upregulating ITGB3. Int J Biol Sci 2022;18:5858-72. [Crossref] [PubMed]
- Liu W, Chakraborty B, Safi R, et al. Dysregulated cholesterol homeostasis results in resistance to ferroptosis increasing tumorigenicity and metastasis in cancer. Nat Commun 2021;12:5103. [Crossref] [PubMed]
- Lu Y, Qin H, Jiang B, et al. KLF2 inhibits cancer cell migration and invasion by regulating ferroptosis through GPX4 in clear cell renal cell carcinoma. Cancer Lett 2021;522:1-13. [Crossref] [PubMed]
- Liu P, Wu D, Duan J, et al. NRF2 regulates the sensitivity of human NSCLC cells to cystine deprivation-induced ferroptosis via FOCAD-FAK signaling pathway. Redox Biol 2020;37:101702. [Crossref] [PubMed]
- Zhang Y, Li S, Li F, et al. High-fat diet impairs ferroptosis and promotes cancer invasiveness via downregulating tumor suppressor ACSL4 in lung adenocarcinoma. Biol Direct 2021;16:10. [Crossref] [PubMed]
- Yao NS, Wu YY, Janckila AJ, et al. Serum tartrate-resistant acid phosphatase 5b (TRACP5b) activity as a biomarker for bone metastasis in non-small cell lung cancer patients. Clin Chim Acta 2011;412:181-5. [Crossref] [PubMed]
- Chung JH, Park MS, Kim YS, et al. Usefulness of bone metabolic markers in the diagnosis of bone metastasis from lung cancer. Yonsei Med J 2005;46:388-93. [Crossref] [PubMed]
- Xia X, Li K, Wu R, et al. Predictive value of neuron-specific enolase, neutrophil-to-lymphocyte-ratio and lymph node metastasis for distant metastasis in small cell lung cancer. Clin Respir J 2020;14:1060-6. [Crossref] [PubMed]
- Yan HJ, Tan Y, Gu W. Neuron specific enolase and prognosis of non-small cell lung cancer: a systematic review and meta-analysis. J BUON 2014;19:153-6. [PubMed]
(English Language Editor: A. Kassem)