Value of radiomics in differentiating synchronous double primary lung adenocarcinomas from intrapulmonary metastasis
Highlight box
Key findings
• Differences of radiomics features showed excellent diagnostic performance for preoperative differentiation between SDPLA from IPM.
What is known and what is new?
• Certain CT characteristics are valuable in differentiating between SDPLA and IPM, which proves that CT possesses the potential to differentiate between the two conditions.
• This study is the first to identify double lung cancer with the difference of radiomics and achieve satisfactory diagnostic efficacy.
What is the implication, and what should change now?
• The radiomics features can serve as a vital decision support tool in identifying SDPLA and IPM. Prospective multicentric studies need to conducted to verify the present results.
Introduction
With the widespread use of cancers screening programs and high-resolution imaging facilities, multiple lung cancers are increasingly detected (1,2). Whether double lung cancer should be diagnosed as synchronous double primary lung adenocarcinoma (SDPLA) or single lung cancer with intrapulmonary metastases (IPM) is a critical point directly affecting tumor node metastasis (TNM) staging and thus the therapeutic plans (2-5). If double lesions are diagnosed as SDPLA, surgical resections would be recommended. Contrarily, patients are recommended to undergo systemic treatment modalities such as chemotherapy or targeted therapy if IPM is diagnosed (2). Moreover, according to the International Association for the Study of Lung Cancer (IASLC) 8th TNM Staging System (5), when the primary lung cancer metastasizes as intrapulmonary metastasis, it would be classified as advanced (IIIb-IV) as per TNM staging System. In contrast, a separate T, N, and M stage should be assigned to each tumor in patients with SDPLAs, and the TNM stage is determined by the higher stage of the lesions. Nevertheless, for distinguishment between the two largely rely on pathological features, which are generally obtained after surgical excision (6). To date, there is still no definitive method to identify SDPLAs before surgery.
High-resolution CT (HRCT) is an examination technique for thin slice (1.0–1.5 mm) scanning and high-resolution algorithm reconstruction of images, which is the best method for the detection of multiple lung cancers. A wide range of imaging findings has shown to present clear correlation with adenocarcinoma subtypes, histological patterns, and prognosis (7-9), stressing the key role of imaging in the diagnosis and management of the disease. Our previous study (10) and other studies (3,11,12) have found that certain CT characteristics are significantly different between SDPLA and IPM, which prove that CT possesses the potential to differentiate between the two, such as with the presentation of ground-glass opacities or air bronchograms. The research on PET/CT also showed that the relative difference between the standard uptake value of these tumors (ΔSUV) was significantly different between second primary cancer and metastatic disease (3). However, previous studies only proposed some differences in clinical and imaging features between the two groups of patients; without quantitative analysis, none of them had constructed prediction models to differentiate metastatic disease from second primary tumors.
Radiomics is an expression algorithm for extracting and analyzing quantitative image features from medical images (13). Many studies have identified the promising potential of radiomics in predicting histologic subtypes (14,15), therapeutic response (16), and clinical prognosis (17) in lung cancer. However, to our knowledge, no studies have applied radiomics to differentiate between SDPLA and IPM. This may be because radiomics usually focuses on the evaluation of single lesion. Recently, a novel radiomics concept has markedly attracted clinicians’ attention, namely delta-radiomics features, which can compare the radiomics differences of lesions obtained from two CT images. These features have been shown to be effective in improving the performance of diagnostic models in lung cancer screening (18), differentiation of ground-glass nodules, and prediction of treatment response (19). Thus, the differences of radiomics may have the potential to differentiate SDPLA from IPM.
On this basis, the present study aimed to investigate the value of differences of radiomics to discriminate SDPLA from IPM. We also compared the diagnostic value of the differences of radiomics signature and the combination of clinical and CT features. We present this article in accordance with the TRIPOD reporting checklist (available at https://jtd.amegroups.com/article/view/10.21037/jtd-23-133/rc).
Methods
The study was conducted in accordance with the Declaration of Helsinki (as revised in 2013). This retrospective study was approved by the Ethics Committee of Wuhan Union Hospital (code: 0648-01, date of approval: 08/20/2021), and all patients have given verbal consent via telephone.
Patients and inclusion criteria
Data from a total of 6,270 patients with lung adenocarcinomas confirmed by surgery and histopathology at our institution between January 2013 and December 2021 were retrospectively analyzed. 6,117 patients were excluded according to the following exclusion criteria: (I) the number of lung lesions <2 or ≥3 (n=4,567); (II) only a satellite lesion in a single lobe (n=1,074); (III) metachronous multiple lung cancers (The interval between occurrences >6 months, n=53); (IV) no thin-section CT available before surgery (n=402); or inadequate CT image quality (n=21, Figure 1). A satellite lesion was defined based on the guideline of American College of Chest Physicians as the lesion located in the same lung lobe as the tumor, having the same histological pattern and with no distant metastasis (20). Then, 153 patients with double lung adenocarcinoma were screened. Of these, 96 cases that met the comprehensive histologic assessment (CHA) criteria were assigned to the SDPLA group, while 60 cases that showed an identical clonal origin by histology were categorized as IPM. In total, 153 patients were included in building the model and then were randomly assigned to the training dataset (122 patients) and testing dataset (31 patients), with a ratio of 4:1 (Figure 1).
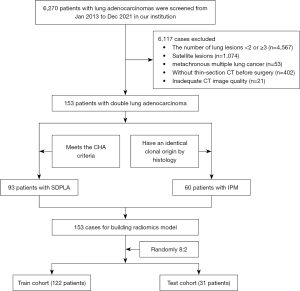
The patient’s clinical characteristics, surgical approach, and pathological findings, including age, gender, smoking history, TNM stage, and histological subtype were recorded. The TNM stage was determined based on the IASLC 8th TNM Lung Cancer Staging System (5). When the primary lung cancer metastasizes to the same lobe, it is classified as stage IIIb; when it metastasizes to the ipsilateral lung on different lobe, it is classified as stage T4s; and when it metastasizes to the contralateral lung, it is classified as stage M1. In contrast, a separate T, N, and M stage should be assigned to each tumor in patients with SDPLA, and the TNM stage is determined by the higher stage of the two lesions.
CHA criteria
The definitions of SDPLA and IPM were defined as CHA standard (21), which was recommended by the IASLC/American Thoracic Society/European Respiratory Society for distinguishing multiple lung primary cancers from lung metastases (22): (I) at least one of the multiple cancers was adenocarcinoma in situ (AIS); (II) multiple lesions with different major histopathologic subtypes; (III) the pathological subtypes of the main tissues are similar, but there are differences in histological subtypes/cytology/stroma, and (IV) the interval of tumor-free between cancers was less than six months In addition, IPM was defined as primary adenocarcinoma with intrapulmonary metastasis, and the two synchronous lesions have (I) both similar major histopathologic subtypes and other histological subtypes; or (II) both similar major histopathologic subtypes and growth pattern/architecture. Information on genetic mutations was also collected in cases including epidermal growth factor receptor (EGFR) inhibitor and Kirsten rat sarcoma viral oncogene homolog (KRAS). Tumor pairs with different mutations were diagnosed as SDPLA, and those with similar mutations as IPM (23).
CT image acquisition and reconstruction
CT scan was performed with one of two multislice spiral CT scanners (SOMATOM Definition, SOMATOM Definition AS+, Siemens Healthineers, Germany) with the following parameters: detector collimation width, 64 mm × 0.6 mm and 128 mm × 0.6 mm; tube voltage, 120 kV. An automatic exposure control system (CARE Dose 4D) was used to modulate the tube current. Images with a slice thickness of 1.5 mm or 1 mm and an interval of 1.5 mm or 1 mm were reconstructed. No contrast medium was used.
Image evaluation
Two senior radiologists (HSS and HWR, with 31- and 15-year experience in thoracic radiology, respectively) evaluated the CT images to determine tumor locations (same lobe or different lobe), tumor size [longest diameter on multiplanar reconstruction (MPR) images], the difference (Δd) in the largest diameter between two tumors, tumor density types (solid or ground-glass opacities) and other important morphological features with consensus on the picture archiving and communication system (PACS). To note, pairs of nodules that either showing or ones with no certain CT sign were grouped into the (−) group, while pairs of nodules that showed one nodule with that CT sign were grouped into the (+) group. The two radiologists assessed the CT features on both axial CT and MPR images while they were blinded to the clinical and histologic findings.
Three radiologists with different degrees of experience (HWR, XJ, and YTZ, and with 15, 5, and 2 years of experience in radiology, respectively) were invited to perform independently all image analysis from the testing set without knowing to the clinical or pathological findings. In the task, radiologists needed to decide whether the cases were SDPLA or IPM (19 SDPLA and 13 IPM) independently.
Radiomics feature extraction
The regions of interest (ROIs) on CT images were semi-automatically delineated layer-by-layer by two junior radiologists (XYH, YTZ with 6 and 3 years of experience in chest imaging, respectively). The two radiologists were aware of the tumor’s presence and location but without knowledge of the clinical and pathological report. According to the characteristics of CT, the 3D Slicer software was used to segment the CT images semi-automatically and independently (Figure 2). Two senior radiologists (HSS and HWR with 31 and 13 years of experience in thoracic imaging, respectively) were responsible for checking the segmentation of tumors, and any deviation was addressed with additional correction. In addition, intra-observer and interobserver variability were analyzed in 35 randomly selected CT images (25 SDPLA and 10 IPM cases) using intraclass correlation coefficients (ICCs). One radiologist (XYH) repeated the segmentation after one week to analyze intra-observer variability. The two radiologists (XYH and YTZ) performed the segmentation on the same image set using the same method for inter-observer variability analysis. An ICC cutoff value of >0.75 was utilized to identify the stable and reproducible features.
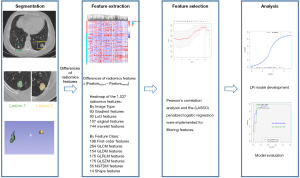
All the radiomics features in the feature extraction module were extracted using a third-party Python library called Pyradiomics (24). The settings for the pyradiomics feature extraction were as followings: normalized with the mean standard deviation as the center, resampled to a voxel size of 1×1×1 mm3 using B-spline interpolation, and a fixed bin width of 25 used in the histogram for gray dispersion (25). Moreover, all images were resampled with a slice thickness of 0.625–1.50 mm with an increment of 1.5 or 1 mm. For each ROI, 1,037 radiomic features, including original image, wavelet transformed, loG features, and gradient filtered features, were extracted (Figure 2, and further details can be found at pyradiomics.readthedocs.io/en/latest/features.html). The heatmap of the 1,037 radiomics feature could be categorized based on image type and feature class. By image type, there were 93 gradient features, 93 LoG features, 107 original features, and 744 wavelet features. By feature class, there were 198 first-order features, 264 GLCM features, 154 GLDM features, 176 GLRLM features, 176 GLSZM features, 55 NGTDM features, and 14 Shape features.
Differences of radiomics features
For patients who received CT scans, these radiomics features (RFs) were extracted from these two coexisting lesions in the same scans, respectively. The differences of-RFs were defined as the relative net difference of RFs between two lesions (26):
Differences of radiomics features = |Featurelesion1 – Featurelesion2|.
Statistical analysis
The 21.0 SPSS software (IBM, Armonk, NY, USA) and R (version 4.0.2; http://www.Rproject.org) were used for all statistical analyses. Lasso binary logistic regression was carried out with the ‘glmnet’ package. Multivariate binary logistic regression was performed with the ‘rms’ package. Decision curve analysis was conducted with the ‘rmda’ package. Receiver operating characteristic (ROC) analysis was performed with the ‘pROC’ package. Continuous and categorical variables were expressed as median [interquartile range (IQR)] and frequency (percentage), respectively. The student’s t-test was applied to compare differences between the two groups, and Fisher’s exact or Chi-Square tests were used to compare categorical variables. All features of the training cohort were normalized to the z-score, and Pearson’s correlation analysis and inter-observer variability analysis were employed to exclude redundant variables (r>0.8). The five-fold cross-validation least absolute shrinkage and selection operator (LASSO) analysis was performed on the training data set to determine features for further assessment (20). The model with 'training data' coefficients was used evaluating on the testing data.
Multivariate logistic regression (LR) algorithm was used to establish the classification model according to the selected features. Three models were built based on three sets of features: a radiomics model based on the four most contributing differences of radiomics features from the temporary model, a clinical-CT model based on selected clinical and CT findings from significant characteristics between two groups, and a mixed model (MixModel) combined the Rad-score with clinical-CT characteristics. The performances of these models for distinguishing SDPLA from IPM were evaluated in the training and testing sets by plotting ROC curves and calculating the areas under the curves (AUCs). The DeLong test was conducted to compare the diagnostic performance among the different models. In addition, decision curve analysis was performed to determine the clinical usefulness of the three models by quantifying the net benefits at different threshold probabilities in the data set. Two-tailed P<0.05 was considered statistically significant.
Results
Clinicopathological characteristics and CT features
Totally, 153 eligible patients (306 tumors) with synchronous cancers (93 SDPLA and 60 IPM) were included. As shown in Table 1, no significant differences were found between the two groups in terms of age, or smoking history in both training and testing sets. IPM occurred more often in men than SDPLA in the testing sets (75% vs. 21.1%, P=0.008). Regarding tumor histology, 54 of 74 patients (73%) with SDPLA had at least one tumor being minimally invasive adenocarcinoma (MIA), whereas all tumors of IPM were invasive adenocarcinoma in the training cohort. Only 14.9% of SDPLA patients presented with stage III or IV disease, whereas all patients with IPM (100%) showed as III-IV TNM stage. Furthermore, lymph node metastasis and distant metastasis were more common in the IPM group than SDPLA in the training set, while no significant differences were found between the two groups in the testing set. Compared with IPM, SDPLA was more frequently located in different lobes (36.5% vs. 60.4%, P=0.015) and different tumor type (41.9% vs. 10.4%, P<0.001). On CT, compared with IPM, SDPLA more frequently showed a smaller difference in the largest diameter (Δd) between tumors (8.9±7.0 vs. 15.5±11.9 mm, P=0.001), less commonly presented as solid nodules (42.9% vs. 93.6%, P<0.001, Figure 3). No significant differences were found in other CT signs such as spiculate, lobulated, and air bronchogram, between groups. Therefore, gender, Δd, and tumor type were included in the combined clinical and CT model (Clinical-CT Model) for differentiating SDPLA from IPM by the LR algorithm. Based on the selected clinical and CT features, the AUC of the model was 0.793 and 0.735 in the training cohort and in the testing cohort (Figure 4).
Table 1
Variable | Train cohort | Test cohort | |||||
---|---|---|---|---|---|---|---|
SDPLA (n=74) | IPM (n=48) | P value | SDPLA (n=19) | IPM (n=12) | P value | ||
Age (years) | 61.6±7.2 | 59.8±11.6 | 0.295 | 61.8±8.6 | 62.9±9.3 | 0.732 | |
Sex | 0.063 | 0.008* | |||||
Male | 38 (51.4) | 33 (68.8) | 4 (21.1) | 9 (75.0) | |||
Female | 36 (48.6) | 15 (31.3) | 15 (78.9) | 3 (25.0) | |||
Smoking history | 1.0 | 0.219 | |||||
Yes | 15 (20.3) | 10 (20.8) | 4 (21.1) | 3 (25.0) | |||
No | 59 (79.7) | 38 (79.2) | 15 (78.9) | 9 (75.0) | |||
Histological subtype | <0.001 | <0.001* | |||||
Both MIA | 4 [5.34] | 0 | 0 | 0 | |||
Both invasive adenocarcinoma | 21 (28.4) | 48 (100.0) | 2 (10.5) | 12 (100.0) | |||
Mixed | 50 (66.7) | 0 | 17 (89.5) | 0 | |||
TNM stage | <0.001 | <0.001* | |||||
I-II | 66 (89.2) | 0 | 16 (84.2) | 0 | |||
III-IV | 11 (14.9) | 48 (100.0) | 3 (15.8) | 12 (100.0) | |||
Lymphatic metastasis | 8 (10.8) | 25 (52.1) | <0.001 | 6 (31.6) | 8 (66.7) | 0.093 | |
Distant metastasis | 3 (4.1) | 10 (20.8) | 0.005 | 1 (5.3) | 4 (33.3) | 0.051 | |
Tumor location | 0.015 | 0.705 | |||||
Same lobe | 47 (63.5) | 19 (39.6) | 13 (68.4) | 7 (58.3) | |||
Different lobe | 27 (36.5) | 29 (60.4) | 6 (31.6) | 5 (41.7) | |||
Size difference (mm) | 8.9±7.0 | 15.5±11.9 | <0.001 | 11.6±12.4 | 17.4±17.5 | 0.286 | |
Tumor type | <0.001 | 0.065 | |||||
(−) group | 43 (58.1) | 43 (89.6) | 9 (47.4) | 10 (83.3) | |||
(+) group | 31 (41.9) | 5 (10.4) | 10 (52.6) | 2 (16.7) | |||
CT signs# | |||||||
Pleural_attachment | 0.359 | 0.461 | |||||
(−) group | 32 (43.2) | 25 (52.1) | 9 (47.4) | 8 (66.7) | |||
(+) group | 42 (56.8) | 23 (47.9) | 10 (52.6) | 4 (33.3) | |||
Spiculate | 1.0 | 0.065 | |||||
(−) group | 24 (32.4) | 15 (31.3) | 10 (52.6) | 2 (16.7) | |||
(+) group | 50 (67.6) | 33 (68.8) | 9 (47.4) | 10 (83.3) | |||
Lobulated | 0.579 | 0.724 | |||||
(−) group | 37 (50.0) | 21 (43.8) | 11 (57.9) | 6 (50.0) | |||
(+) group | 37 (50.0) | 27 (56.3) | 8 (42.1) | 6 (50.0) | |||
Calcifications | 0.153 | 1.0 | |||||
(−) group | 0 (0.0) | 2 (4.2) | 0 | 0 | |||
(+) group | 74 (100.0) | 46 (95.8) | 19 (100.0) | 12 (100.0) | |||
Bubblelike_lucency | 0.144 | 0.201 | |||||
(−) group | 24 (32.4) | 9 (18.8) | 6 (31.6) | 1 (8.3) | |||
(+) group | 50 (67.6) | 39 (81.3) | 13 (68.4) | 11 (91.7) | |||
Air_bronchogram | 1.0 | 1.0 | |||||
(−) group | 22 (29.7) | 14 (29.2) | 6 (31.6) | 4 (33.3) | |||
(+) group | 52 (70.3) | 34 (70.8) | 13 (68.4) | 8 (66.7) | |||
Vascular_convergence | 0.137 | 1.0 | |||||
(−) group | 9 (12.2) | 11 (22.9) | 7 (36.8) | 4 (33.3) | |||
(+) group | 65 (87.8) | 37 (77.1) | 12 (63.2) | 8 (66.7) | |||
Cavitation | 1.0 | 1.0 | |||||
(−) group | 4 (5.4) | 3 (6.3) | 1 (5.3) | 0 (0.0) | |||
(+) group | 70 (94.6) | 45 (93.8) | 18 (94.7) | 12 (100.0) |
Data are mean ± SD, n (%), or median [IQR]. *, P values were based on comparisons between the two groups. #, We grouped pairs of nodules that either had or did not have a certain CT sign into the (−) group, while pairs of nodules that had only one nodule with that CT sign are grouped into the (+) group. SDPLA, synchronous double primary lung adenocarcinoma; IPM, intrapulmonary metastasis; MIA, minimally invasive adenocarcinoma; CT, computed tomography; TNM, tumor node metastasis.
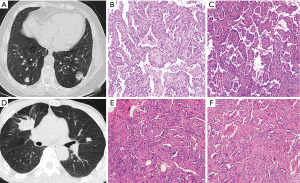

Radiomics model building and validation
According to the results of Pearson’s correlation analysis, 332 differences of radiomics features were selected to establish the radiomics model. The five-fold cross-validation LASSO analysis was performed on the training data set to mitigate the risk of overfitting (Figure 5). Based on LASSO penalized logistic regression analysis, four differences of radiomics features showed a significant difference between SDPLA and IPM. The four radiomics features (first-order 90Percentile, and three second order parameters, including two GLCM and one GLSZM) were identified by the LR model. As presented in Table 2, patients with SDPLA demonstrated significantly higher values for firstorder_90Percentile, glcm_ClusterShade, and _glcm_Imc2 compared to those with IPM. Conversely, the value of glszm_SizeZoneNonUniformity was significantly lower in patients with SDPLA than in individuals with IPM, across both the training and testing sets Features and their coefficients contained in the radiomics model are listed in Table 3. The radiomics model achieved excellent performance with an AUC of 0.944 in the training cohort; the AUC was 0.886 in the testing cohort (Figure 4).
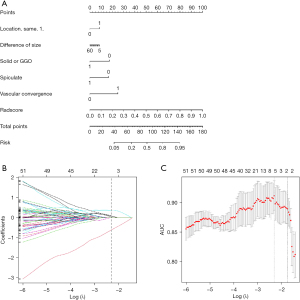
Table 2
Radiomics features | Train cohort | Test cohort | |||||
---|---|---|---|---|---|---|---|
SDPLA (n=74) | IPM (n=48) | P value | SDPLA (n=19) | IPM (n=13) | P value | ||
differences_wavelet.LLL_firstorder_90Percentile | −0.67±0.14 | 0.41±1.1 | <0.001 | −0.68±0.27 | 0.46±1.1 | 0.001 | |
differences_wavelet.LLL_glcm_ClusterShade | −0.54±0.6 | 0.33±1.1 | <0.001 | 0.08±0.35 | −0.11±1.5 | 0.09 | |
Differences_wavelet.LLL_glcm_Imc2 | −0.33±0.88 | 0.55±0.94 | <0.001 | −0.33±0.8 | 0.49±1.1 | 0.13 | |
differences_glszm_SizeZoneNonUniformity | 0.49±1.1 | −0.30±0.83 | <0.001 | 0.49±1.1 | −0.34±0.74 | 0.046 |
Data are shown as mean ± standard deviation. SDPLA, synchronous double primary lung adenocarcinoma; IPM, intrapulmonary metastasis.
Table 3
Differences of radiomics features | Estimate | Standard error | z value | Pr(>|z|) |
---|---|---|---|---|
(Intercept) | −2.057 | 0.901 | −2.283 | 0.022 |
differences _wavelet.HHH_firstorder_Maximum | 1.049 | 0.378 | 2.778 | 0.005 |
differences _wavelet.LLL_firstorder_90Percentile | −4.396 | 1.455 | −3.021 | 0.003 |
differences _wavelet.LLL_glcm_Imc2 | 0.398 | 0.383 | 1.041 | 0.298 |
differences _log.sigma.6.0.mm.3D_glszm_SizeZoneNonUniformity | 0.639 | 0.450 | 1.419 | 0.156 |
differences _gradient_glcm_ClusterProminence | 3.464 | 1.264 | 2.741 | 0.006 |
The following formula was used to calculate the rad-score of each lesion:
Rad-Score = −2.1+1.049 * differences _wavelet.HHH_firstorder_Maximum − 4.396 * differences _wavelet.LLL_firstorder_90Percentile + 0.398 * differences _wavelet.LLL_glcm_Imc2 + 0.639 * differences _log.sigma.6.0.mm.3D_glszm_SizeZoneNonUniformity + 3.464 * differences _gradient_glcm_ClusterProminence
Tumors of SDPLA had significantly lower rad-scores than tumors of IPM in both the training and testing sets (P<0.001).
Performance comparison
A comprehensive nomogram model, MixModel, was developed with the retained clinical-CT characteristics and Rad-score (Figure 5) and showed AUCs of 0.950 and 0.873 in the training and testing cohorts, respectively (Figure 4, Table 4). In both the training and testing cohorts, the AUCs of the radiomics model were larger than those of the clinical-CT model (0.944 vs. 0.793 and 0.886 vs. 0.735 on training and testing cohorts, respectively), and statistically significant differences between the two models in the testing set (P<0.001). Furthermore, the MixModel showed sightly improvement in diagnostic ability compared with the radiomics model in the training set. Still, there were no statistically significant differences between the two models (P>0.05 for both). Meanwhile, three radiologists had sensitivities of 84.2%, 63.9%, and 68.4%, and specificities of 76.9%, 69.2%, and 76.9% in differentiating 19 SDPLA cases from 13 cases of IPM in the testing set (Figure 6). The radiomics model outperformed all the three radiologists.
Table 4
Characteristics | Estimate | Standard error | z value | Pr(>|z|) |
---|---|---|---|---|
(Intercept) | −3.39 | 0.769 | −4.41 | <0.001 |
Location | 0.405 | 0.645 | 0.629 | 0.529 |
Difference of size | 0.00049 | 0.037 | 0.013 | 0.989 |
Solid or GGO | −0.633 | 0.839 | −0.755 | 0.451 |
Spiculate | −0.712 | 0.671 | −1.06 | 0.289 |
Vascular_convergence | 1.212 | 0.881 | 1.38 | 0.169 |
Radscore | 6.46 | 1.151 | 5.61 | <0.001 |
GGO, ground-glass opacity.
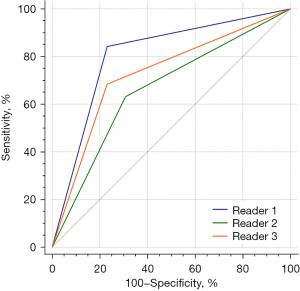
Calibration analysis and clinical use
For the radiomics model and the MixModel, calibration curve analysis showed P values of 0.879, 0.985 and 0.225, 0.592 in the training and testing sets, respectively, indicating a reasonable degree of fit for the model in both sets (Figure S1). In contrast, the clinical-CT model showed poor calibration abilities in both training and testing sets (P<0.001). Through decision curve analysis (Figure S2), the clinical usefulness of the three predictive models was examined. The results showed all three models were able to bring net benefits to the patients, among which the radiomics model showed the best net benefits.
Discussion
According to the CHA standard (17,18), multiple primary tumors are generally manifested as different histological types, while intrapulmonary metastases usually have similar main histological types. Numerous studies have confirmed that CT features and CT-based radiomics features were closely related to the histological features and pathological grade of non-small cell carcinoma (7,27,28). Hence, the difference between two lesions in radiomics features might indirectly represent the difference in histological type.
Consistent with the previous publications (2,29), the present study found the patients with SDPLA showing significant differences from those with IPM in histopathological findings and tumor staging. Correspondingly, patients with multiple primary lung cancer had early stages (I–II), and 73% of SDPLA had at least one MIA tumor, whereas all tumors of IPM were invasive adenocarcinoma. These suggested that SDPLA was more of an early stage in nature and should have a different treatment and prognosis from IPM. Regarding images, some scholars (3) suggested that CT morphological features are of low value in the definite diagnosis of SDPLA. In the present study, no significant differences were found in shape features such as spiculate, lobulated, and air bronchogram, between groups. This study showed SDPLA exhibited significant differences in tumor location, Δd, and tumor type compared with IPM. Furthermore, the model based on selected clinical and CT features (tumor location, Δd, and tumor type) performed well in distinguishing SDPLA and IPM in both the training and testing cohorts. Such differences in CT morphological features were reasonable, as each lesion of SDPLA was independent and had no relationship with its location. At the same time, intrapulmonary metastases are mostly located in the same lobe or within the ipsilateral lung. Moreover, SDPLAs were mostly early lesions with ground-glass opacity (GGO) appearances, while IPM is advanced lesions with a solid appearance. These findings indicate that medical imaging may provide valuable information for diagnosing and differentiating multiple lung cancer patients.
As the current study showed, two categories of radiomics features were selected for discrimination of SDPLA and IPM. (I) The differences first-order 90Percentile. This feature is a robust replacement of the maximum gray-level intensity within the tumor. This may be related to the difference in density between the two lesions in the two groups. (II) The differences of second order included GLCM and GLSZM, reflecting the heterogeneity of tumors. Taken together, we could reasonably assume that the radiomics model could distinguish between SDPLA and IPM by these two indicators: the differences in gray-level intensity and heterogeneity. In addition, the tumor type (GGO or solid) was the most critical feature in determining SDPLA, consistent with the radiomics results. Combining these biomarkers into a signature may provide more helpful information for making reliable clinical decisions. Since the computer-automated extraction radiomics features are more objective and accurate than subjective and manual measurement (30), our study verified the reliability and interpretability of the radiomics features.
The limitations of the present study cannot be ignored. Firstly, as our study required an external validation cohort, it was not possible to accurately evaluate the generalizability and stability of the radiomics model, as well as the reproducibility of radiomic features. Secondly, long-term follow-up is required in order to more reliably compare the difference in the outcome of the two groups. Thirdly, we only included multiple lung cancers with two lesions due to methodological limitations and ignored the cases with ≥3 lesions. Finally, the present study analyzed only adenocarcinoma and did not include other histologic subtypes. But this is understandable because most synchronous lung cancers are found in adenocarcinomas (31).
Conclusions
In summary, the radiomics model obtained an outstanding diagnostic performance for preoperatively discriminating SDPLA from IPM disease, outperforming the clinical model and radiologists. However, due to the single-center retrospective study design, our conclusions must be verified in prospective multicentric studies with different scanners, acquisition parameters, and resolution.
Acknowledgments
Funding: This study was supported by the National Natural Science Foundation of China (Nos. 82071921, 82001788) and Health Commission Foundation of Hubei Province (No. WJ2021M244).
Footnote
Reporting Checklist: The authors have completed the TRIPOD reporting checklist. Available at https://jtd.amegroups.com/article/view/10.21037/jtd-23-133/rc
Data Sharing Statement: Available at https://jtd.amegroups.com/article/view/10.21037/jtd-23-133/dss
Peer Review File: Available at https://jtd.amegroups.com/article/view/10.21037/jtd-23-133/prf
Conflicts of Interest: All authors have completed the ICMJE uniform disclosure form (available at https://jtd.amegroups.com/article/view/10.21037/jtd-23-133/coif). CD is an employee of ShuKun (Beijing) Technology Co., Ltd. XZ is an employee of Philips Healthcare. The other authors have no conflicts of interest to declare.
Ethical Statement: The authors are accountable for all aspects of the work in ensuring that questions related to the accuracy or integrity of any part of the work are appropriately investigated and resolved. The study was conducted in accordance with the Declaration of Helsinki (as revised in 2013).This retrospective study was approved by the Ethics Committee of Wuhan Union Hospital (code: 0648-01, date of approval: 08/20/2021), and all patients have given verbal consent via telephone.
Open Access Statement: This is an Open Access article distributed in accordance with the Creative Commons Attribution-NonCommercial-NoDerivs 4.0 International License (CC BY-NC-ND 4.0), which permits the non-commercial replication and distribution of the article with the strict proviso that no changes or edits are made and the original work is properly cited (including links to both the formal publication through the relevant DOI and the license). See: https://creativecommons.org/licenses/by-nc-nd/4.0/.
References
- Gazdar AF, Minna JD. Multifocal lung cancers--clonality vs field cancerization and does it matter? J Natl Cancer Inst 2009;101:541-3. [Crossref] [PubMed]
- Asamura H. Multiple primary cancers or multiple metastases, that is the question. J Thorac Oncol 2010;5:930-1. [Crossref] [PubMed]
- Dijkman BG, Schuurbiers OC, Vriens D, et al. The role of (18)F-FDG PET in the differentiation between lung metastases and synchronous second primary lung tumours. Eur J Nucl Med Mol Imaging 2010;37:2037-47. [Crossref] [PubMed]
- Voulaz E, Novellis P, Rossetti F, et al. Distinguishing multiple lung primaries from intra-pulmonary metastases and treatment implications. Expert Rev Anticancer Ther 2020;20:985-95. [Crossref] [PubMed]
- Goldstraw P, Chansky K, Crowley J, et al. The IASLC Lung Cancer Staging Project: Proposals for Revision of the TNM Stage Groupings in the Forthcoming (Eighth) Edition of the TNM Classification for Lung Cancer. J Thorac Oncol 2016;11:39-51. [Crossref] [PubMed]
- Martini N, Melamed MR. Multiple primary lung cancers. J Thorac Cardiovasc Surg 1975;70:606-12. [Crossref] [PubMed]
- Cohen JG, Reymond E, Jankowski A, et al. Lung adenocarcinomas: correlation of computed tomography and pathology findings. Diagn Interv Imaging 2016;97:955-63. [Crossref] [PubMed]
- Gao F, Li M, Zhang Z, et al. Morphological classification of pre-invasive lesions and early-stage lung adenocarcinoma based on CT images. Eur Radiol 2019;29:5423-30. [Crossref] [PubMed]
- Cho HH, Lee HY, Kim E, et al. Radiomics-guided deep neural networks stratify lung adenocarcinoma prognosis from CT scans. Commun Biol 2021;4:1286. [Crossref] [PubMed]
- Han X, Fan J, Liu T, et al. Differentiating synchronous double primary lung adenocarcinomas from intrapulmonary metastasis by CT features, EGFR mutations and ALK rearrangement status. J Thorac Dis 2020;12:5505-16. [Crossref] [PubMed]
- Zhong F, Liu Z, Wang B, et al. A Predictive Model to Differentiate Between Second Primary Lung Cancers and Pulmonary Metastasis. Acad Radiol 2022;29:S137-44. [Crossref] [PubMed]
- Ge J, Gou HF, Chen Y, et al. Clinical characteristics of patients with solitary pulmonary mass after radical treatment for primary cancers: pulmonary metastasis or second primary lung cancer? Cancer Invest 2013;31:397-403. [Crossref] [PubMed]
- Gillies RJ, Kinahan PE, Hricak H. Radiomics: Images Are More than Pictures, They Are Data. Radiology 2016;278:563-77. [Crossref] [PubMed]
- Wang M, Perucho JAU, Hu Y, et al. Computed Tomographic Radiomics in Differentiating Histologic Subtypes of Epithelial Ovarian Carcinoma. JAMA Netw Open 2022;5:e2245141. [Crossref] [PubMed]
- Park S, Lee SM, Noh HN, et al. Differentiation of predominant subtypes of lung adenocarcinoma using a quantitative radiomics approach on CT. Eur Radiol 2020;30:4883-92. [Crossref] [PubMed]
- He B, Dong D, She Y, et al. Predicting response to immunotherapy in advanced non-small-cell lung cancer using tumor mutational burden radiomic biomarker. J Immunother Cancer 2020;8:e000550. [Crossref] [PubMed]
- Lee G, Park H, Sohn I, et al. Comprehensive Computed Tomography Radiomics Analysis of Lung Adenocarcinoma for Prognostication. Oncologist 2018;23:806-13. [Crossref] [PubMed]
- Alahmari SS, Cherezov D, Goldgof D, et al. Delta Radiomics Improves Pulmonary Nodule Malignancy Prediction in Lung Cancer Screening. IEEE Access 2018;6:77796-806.
- Barabino E, Rossi G, Pamparino S, et al. Exploring Response to Immunotherapy in Non-Small Cell Lung Cancer Using Delta-Radiomics. Cancers (Basel) 2022;14:350. [Crossref] [PubMed]
- Kozower BD, Larner JM, Detterbeck FC, et al. Special treatment issues in non-small cell lung cancer: Diagnosis and management of lung cancer, 3rd ed: American College of Chest Physicians evidence-based clinical practice guidelines. Chest 2013;143:e369S-99S.
- Girard N, Deshpande C, Lau C, et al. Comprehensive histologic assessment helps to differentiate multiple lung primary nonsmall cell carcinomas from metastases. Am J Surg Pathol 2009;33:1752-64. [Crossref] [PubMed]
- Travis WD, Brambilla E, Noguchi M, et al. International association for the study of lung cancer/american thoracic society/european respiratory society international multidisciplinary classification of lung adenocarcinoma. J Thorac Oncol 2011;6:244-85. [Crossref] [PubMed]
- Braakhuis BJ, Tabor MP, Leemans CR, et al. Second primary tumors and field cancerization in oral and oropharyngeal cancer: molecular techniques provide new insights and definitions. Head Neck 2002;24:198-206. [Crossref] [PubMed]
- van Griethuysen JJM, Fedorov A, Parmar C, et al. Computational Radiomics System to Decode the Radiographic Phenotype. Cancer Res 2017;77:e104-7. [Crossref] [PubMed]
- Leijenaar RT, Nalbantov G, Carvalho S, et al. The effect of SUV discretization in quantitative FDG-PET Radiomics: the need for standardized methodology in tumor texture analysis. Sci Rep 2015;5:11075. [Crossref] [PubMed]
- Fave X, Zhang L, Yang J, et al. Delta-radiomics features for the prediction of patient outcomes in non-small cell lung cancer. Sci Rep 2017;7:588. [Crossref] [PubMed]
- Zhu X, Dong D, Chen Z, et al. Radiomic signature as a diagnostic factor for histologic subtype classification of non-small cell lung cancer. Eur Radiol 2018;28:2772-8. [Crossref] [PubMed]
- Ganeshan B, Goh V, Mandeville HC, et al. Non-small cell lung cancer: histopathologic correlates for texture parameters at CT. Radiology 2013;266:326-36. [Crossref] [PubMed]
- Tanvetyanon T, Robinson L, Sommers KE, et al. Relationship between tumor size and survival among patients with resection of multiple synchronous lung cancers. J Thorac Oncol 2010;5:1018-24. [Crossref] [PubMed]
- Lambin P, Rios-Velazquez E, Leijenaar R, et al. Radiomics: extracting more information from medical images using advanced feature analysis. Eur J Cancer 2012;48:441-6. [Crossref] [PubMed]
- Pagan CA, Shu CA, Crapanzano JP, et al. Synchronous Pulmonary Adenocarcinomas. Am J Clin Pathol 2020;154:57-69. [Crossref] [PubMed]