Development and validation of a diagnostic model based on left atrial diameter to predict postoperative atrial fibrillation after off-pump coronary artery bypass grafting
Highlight box
Key findings
• AF can be predicted more accurately using the LAD system compared with the original risk prediction system. Here, LAD was added to the previous scoring system to optimize its predictive ability.
What is known and what is new?
• POAF score, CHA2DS2-VASc risk index are currently used to predict POAF in patients undergoing elective CABG surgery or valve surgery.
• The benefit of this scoring system is the inclusion of simple preoperative variables, which can appropriately predict the development of POAF when a patient is admitted to receive clinical measures, such as medication or atrial pacing, depending on the patient’s level of risk.
What is the implication, and what should change now?
• The scoring system developed is not without flaws, and validation with a large sample size is needed to further refine the postoperative evaluation system.
Introduction
Background
The most common postoperative arrhythmic complication following cardiovascular surgery is postoperative atrial fibrillation (POAF), which occurs in approximately 20% to 40% of cases, usually within 2 to 4 days after surgery. Approximately one-third of patients receiving coronary artery bypass grafting (CABG) experience POAF (1). Studies have shown that the occurrence and development of POAF following CABG is associated with longer hospital stays, a higher risk of thromboembolism, and increased risk of death during hospitalization and hospital costs, while also being significantly related to an increased risk of stroke at long-term follow-up (2,3).
Rationale and knowledge gap
The exact mechanisms underlying POAF are complex and remain unclear. However, there is growing evidence that inflammation plays a crucial role in both the initiation and maintenance of POAF, and systemic inflammatory status has been shown to predict the development and progression of POAF in patients who have undergone cardiac surgery, including CABG (4-6). Since Bruins et al. first demonstrated in 1997 that complement system stimulation and proinflammatory cytokine secretion are related to POAF development, Navani et al. also verified the relationship between additional clinically available inflammatory markers and POAF had been investigated in 2020 (7,8). The systemic immune-inflammation index (SII) is a newly developed inflammatory marker that integrates neutrophil, lymphocyte, and platelet counts to accurately represent the body’s overall inflammatory condition (9). It was found that preoperative SII plays an important role in predicting POAF in patients undergoing CABG and is better and more effective in identifying POAF in these patients compared with other inflammatory biomarkers (10,11).
Objective
Previous studies have recognized multiple risk factors that may impact POAF development, including age, heart failure, cardiac rheumatic disease, chronic renal failure, and chronic obstructive pulmonary disease (COPD) (12,13). Many models have been developed and validated to anticipate the occurrence of POAF following cardiac surgery to enhance the efficacy of prophylactic measures and minimize the overall patient burden. However, there are no widely accepted risk models, and the POAF, CHA2DS2-VASc [congestive heart failure, hypertension, age ≥75 years (doubled), diabetes, stroke (doubled), vascular disease, age 65 to 74 years, and sex category (female)], and HATCH [hypertension, age (above 75 years), transient ischemic attack or stroke, COPD, and heart failure] scores are widely used to anticipate POAF following cardiac surgery and have shown good discrimination and calibration in patients with CABG (14-16). These scoring systems only consider influencing factors such as age and comorbidities and ignore the important role of left atrial size on POAF. Therefore, this study’s aims were the following: to determine if left atrial size is an independent risk factor for atrial fibrillation (AF) following off-pump CABG (OPCABG) (17-19), to construct and validate a prediction model of POAF in patients with OPCABG based on left atrial size and compare it with a commonly used POAF prediction scoring system, and to evaluate the effect of adding left atrial size to the commonly used POAF prediction scoring system. It is hoped this model improvements can better predict the development of POAF, thus assisting clinicians in detecting patients at a high risk of POAF and optimizing medical decision-making in clinical practice. We present this article in accordance with the TRIPOD reporting checklist (available at https://jtd.amegroups.com/article/view/10.21037/jtd-22-1706/rc).
Methods
Patients and design
This study included patients (n=749) who underwent OPCABG at Beijing Anzhen Hospital, Capital Medical University, between May 1, 2021, and February 1, 2022, with no prior history of arrhythmia. The patients were divided into the derivation and validation sets (7:3).
Participant selection
The enrollment criteria were the following: patients undergoing isolated OPCABG in sinus rhythm preoperatively and with complete clinical data preservation. The exclusion criteria were as follows: (I) previous diagnosis of AF; (II) comorbidities of other malignancies; (III) coexisting infectious disease (positive secretions or tissue cultures); (IV) coexisting autoimmune diseases (e.g., rheumatoid arthritis, ankylosing spondylitis, or systemic lupus erythematosus); and (V) concomitant neurological disorders with communication disorders (Figure 1). According to the optimal cutoff point derived from receiver operating characteristic (ROC) curve analysis, 749 patients were separated into two groups: a group with POAF (n=188) and a group without POAF (n=561). Perioperative data for each patient were obtained retrospectively. Preoperative laboratory data were available for all patients. Emergency surgery was defined as surgery conducted within 48 hours of hospitalization; palpitations as unpleasant sensations of an abnormal heartbeat; and POAF as that occurring within 1 week after surgery as confirmed by bedside electrocardiogram (ECG) or remote ECG monitoring without distinguishable P waves, with an absolute RR interval arrhythmia, and episodes lasting at least 30 s, regardless of symptoms (20).
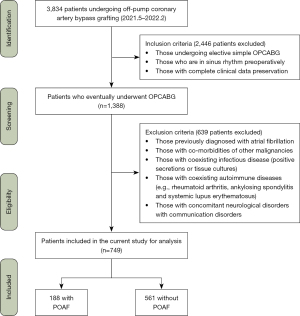
Systems for assessing risk
Retrospectively, scores were calculated using three systems.
The CHA2DS2-VASc score consisted of the following: age ≥75 years, 2 points; age 65–74 years, 1 point; female sex, 1 point; history of heart failure, 1 point; combined hypertension, 1 point; combined stroke/transient ischemic attack, 2 points; combined diabetes mellitus, 1 point; and combined peripheral vascular disease, 1 point (15).
The POAF score consisted of the following: age 60–69 years, 1 point; age 70–79 years, 2 points; age ≥80 years, 3 points; comorbid COPD, 1 point; glomerular filtration rate on dialysis <15 mL/min/1.73 m2, 1 point; emergency surgery, 1 point; preoperative intra-aortic balloon pump, 1 point; left ventricular ejection fraction <30%, 1 point; and any heart valve surgery, 1 point (14).
The HATCH consisted of the following: combined hypertension, 1 point; age ≥75 years, 1 point; combined stroke or transient ischemic attack, 2 points; combined COPD, 1 point; and history of heart failure, 2 points (16).
Statistical analysis
All analyses were run in the R language (version 4.1.0, The R Foundation for Statistical Computing, Vienna, Austria). The means and standard deviations of the quantitative data were compared using at-test, which is an analysis of variance. Dichotomous variables are presented as absolute values and proportions, and differences between proportions were analyzed with χ2 tests or Fisher exact tests. In this study, nonnormally distributed ordinal and continuous variables are expressed as median and interquartile range (IQR) and were compared using the Mann-Whitney U test. Variables apparently associated with POAF after univariate analysis (P<0.05) were entered in a multivariable logistic regression model. Stepwise logistic regression was used to identify predictors of POAF, and each variable score was included in the final model comparison. The model variables are presented as odds ratios (ORs) along with 95% confidence intervals (CIs). The area under the ROC curve (AUC-ROC) was calculated to assess the predicted values of different scoring systems. We used the nomogram to visualize the new POAF models. To compare the concordance indexes (C-indexes) of two models, a two-sided DeLong test was used. ROC curves were compared with the method of DeLong et al. (21). To assess the performance of nomograms in the derivation and validation cohorts, calibration curves were plotted. Decision curve analysis was carried out to evaluate the clinical benefit of the model. To determine a suitable cutoff point, the Youden index was used (22).
Ethical statement
The study was conducted in accordance with the Declaration of Helsinki (as revised in 2013). The study was approved by the Ethics Committee of Beijing Anzhen Hospital (No. 2023075X) and individual consent for this retrospective analysis was waived.
Results
Patient characteristics
During the period analyzed, 749 patients were included in the analysis according to the inclusion and exclusion criteria. The baseline features of the patients with and without POAF are shown in Table 1. There were 449 patients in the derivation cohort and 300 in the validation cohort. The mean age of the group without POAF was 61.20±8.75 years, of whom 122 (21.7%) were female, 338 (60.2%) were hypertensive, and 211 (37.6%) were diabetic. The mean age of the group with POAF was 65.32±8.20 years, of whom 43 (22.9%) were female, 117 had hypertension (62.2%), and 83 had diabetes (44.1%).
Table 1
Variables | Overall (n=749) | Non-POAF (n=561) | POAF (n=188) | P |
---|---|---|---|---|
Age (years) | 62.23±8.80 | 61.20±8.75 | 65.32±8.20 | <0.001 |
BSA (m2) | 1.80±0.28 | 1.80±0.31 | 1.81±0.19 | 0.711 |
Height (cm) | 168.30±37.05 | 168.66±42.54 | 167.22±8.24 | 0.644 |
Weight (kg) | 72.64±11.37 | 72.29±11.18 | 73.67±11.88 | 0.150 |
BMI (kg/m2) | 25.95±3.27 | 25.85±3.34 | 26.25±3.03 | 0.147 |
Coronary artery lesions | 2.69±0.66 | 2.69±0.67 | 2.68±0.61 | 0.772 |
Volume of drainage (mL) | 858.26±522.83 | 846.18±519.40 | 894.31±532.72 | 0.275 |
Hospital length of stay (d) | 13.09±4.00 | 12.82±3.76 | 13.89±4.53 | 0.001 |
ICU time (d) | 1.57±1.50 | 1.47±1.32 | 1.85±1.91 | 0.003 |
LVM (g) | 167.88±41.55 | 164.85±39.35 | 176.92±46.45 | 0.001 |
LVMI | 93.36±20.84 | 91.89±19.81 | 97.72±23.17 | 0.001 |
SII (×109/L) | 1,283.11±1,630.46 | 1,317.46±1,759.97 | 1,180.63±1,159.12 | 0.320 |
CHA2DS2-VASc score | 2.41±1.57 | 2.25±1.50 | 2.90±1.69 | <0.001 |
HATCH score | 1.20±1.17 | 1.12±1.08 | 1.44±1.36 | 0.001 |
POAF score | 0.91±0.84 | 0.78±0.79 | 1.29±0.87 | <0.001 |
Preoperative LVEF (%) | 59.95±7.96 | 60.20±7.65 | 59.21±8.80 | 0.140 |
LAD (mm) | 43.96±9.62 | 43.10±9.51 | 46.53±9.52 | <0.001 |
IVST (mm) | 10.26±1.65 | 10.19±1.57 | 10.46±1.86 | 0.047 |
LVPWT (mm) | 9.18±1.36 | 9.14±1.27 | 9.30±1.61 | 0.177 |
LVEDD (mm) | 48.34±5.23 | 48.05±5.09 | 49.20±5.56 | 0.009 |
ALT (U/L) | 27.11±44.21 | 26.84±30.63 | 27.90±70.76 | 0.777 |
AST (U/L) | 23.52±64.79 | 21.44±16.06 | 29.72±126.37 | 0.129 |
GGT (U/L) | 33.16±36.15 | 33.58±38.89 | 31.91±26.39 | 0.583 |
ALP (U/L) | 80.89±29.44 | 81.07±31.06 | 80.37±24.05 | 0.777 |
T-Bil (μmol/L) | 11.02±4.71 | 10.83±4.72 | 11.58±4.63 | 0.059 |
D-Bil (μmol/L) | 4.66±1.94 | 4.59±1.99 | 4.87±1.80 | 0.084 |
IBIL (μmol/L) | 6.36±3.18 | 6.24±3.20 | 6.71±3.10 | 0.082 |
TP (g/L) | 68.28±5.25 | 68.17±5.09 | 68.60±5.70 | 0.327 |
ALB (g/L) | 42.62±3.31 | 42.63±3.30 | 42.56±3.36 | 0.783 |
GLB (g/L) | 25.66±3.65 | 25.54±3.56 | 26.04±3.87 | 0.100 |
A/G | 1.69±0.26 | 1.70±0.26 | 1.66±0.24 | 0.103 |
Urea (mmol/L) | 5.96±2.41 | 5.81±2.01 | 6.43±3.28 | 0.002 |
Creatinine (mg/dL) | 79.75±47.31 | 77.98±50.71 | 85.04±34.85 | 0.077 |
Unstable angina (μmol/L) | 323.47±90.17 | 320.43±90.86 | 332.54±87.70 | 0.111 |
TC (mmol/L) | 3.93±1.02 | 3.93±1.01 | 3.92±1.03 | 0.949 |
TG (mmol/L) | 1.67±0.96 | 1.70±1.00 | 1.59±0.84 | 0.179 |
LDL-C (mmol/L) | 2.18±0.83 | 2.18±0.84 | 2.18±0.81 | 0.954 |
HDL-C (mmol/L) | 0.96±0.23 | 0.96±0.23 | 0.98±0.22 | 0.297 |
WBC (×109/L) | 8.74±3.70 | 8.81±3.77 | 8.55±3.47 | 0.400 |
RBC (×1012/L) | 4.11±0.75 | 4.10±0.73 | 4.12±0.81 | 0.780 |
PLT (×109/L) | 210.68±70.17 | 212.35±69.63 | 205.69±71.72 | 0.260 |
Hb (g/L) | 126.15±23.16 | 125.92±22.68 | 126.83±24.60 | 0.641 |
LYM (×109/L) | 1.53±0.75 | 1.54±0.75 | 1.50±0.73 | 0.580 |
MONO (×109/L) | 0.44±0.24 | 0.43±0.23 | 0.45±0.26 | 0.373 |
NE (×109/L) | 6.61±3.83 | 6.68±3.92 | 6.40±3.57 | 0.385 |
MPV (fL) | 10.02±1.15 | 9.98±1.14 | 10.15±1.18 | 0.086 |
EOS (×109/L) | 0.13±0.14 | 0.13±0.15 | 0.13±0.12 | 0.898 |
BAS (×109/L) | 0.02±0.02 | 0.02±0.02 | 0.02±0.02 | 0.251 |
CRP (mg/L) | 73.63±74.39 | 73.21±73.93 | 74.90±75.94 | 0.788 |
RDW-SD (fL) | 41.60±3.45 | 41.50±3.49 | 41.89±3.31 | 0.183 |
RDW-CV (%) | 12.87±0.94 | 12.84±0.94 | 12.93±0.96 | 0.297 |
Female | 165 (22.0) | 122 (21.7) | 43 (22.9) | 0.825 |
Smoking history | 381 (50.9) | 293 (52.2) | 88 (46.8) | 0.229 |
Drinking history | 224 (29.9) | 161 (28.7) | 63 (33.5) | 0.248 |
CHF | 82 (10.9) | 58 (10.3) | 24 (12.8) | 0.431 |
Previous valve surgery | 2 (0.3) | 1 (0.2) | 1 (0.5) | 1.000 |
Hypertension | 455 (60.7) | 338 (60.2) | 117 (62.2) | 0.692 |
Diabetes | 294 (39.3) | 211 (37.6) | 83 (44.1) | 0.133 |
Hypercholesterolemia | 222 (29.6) | 160 (28.5) | 62 (33.0) | 0.286 |
COPD | 7 (0.9) | 5 (0.9) | 2 (1.1) | 1.000 |
CVD | 93 (12.4) | 68 (12.1) | 25 (13.3) | 0.768 |
PVD | 217 (29.0) | 145 (25.8) | 72 (38.3) | 0.002 |
NYHA functional class | 0.935 | |||
1 | 58 (7.7) | 44 (7.8) | 14 (7.4) | |
2 | 438 (58.5) | 331 (59.0) | 107 (56.9) | |
3 | 239 (31.9) | 176 (31.4) | 63 (33.5) | |
4 | 14 (1.9) | 10 (1.8) | 4 (2.1) | |
Previous PCI | 107 (14.3) | 78 (13.9) | 29 (15.4) | 0.692 |
Unstable angina | 559 (74.6) | 414 (73.8) | 145 (77.1) | 0.417 |
LMD | 26 (3.5) | 20 (3.6) | 6 (3.2) | 0.990 |
TVD | 110 (14.7) | 88 (15.7) | 22 (11.7) | 0.224 |
AMI | 49 (6.5) | 34 (6.1) | 15 (8.0) | 0.453 |
Other CAD | 5 (0.7) | 5 (0.9) | 0 (0.0) | 0.435 |
Beta-blocker | 579 (77.3) | 433 (77.2) | 146 (77.7) | 0.973 |
CCB | 268 (35.8) | 206 (36.7) | 62 (33.0) | 0.402 |
ACE inhibitor | 43 (5.7) | 36 (6.4) | 7 (3.7) | 0.233 |
ARB | 147 (19.6) | 113 (20.1) | 34 (18.1) | 0.611 |
Diuretic | 95 (12.7) | 70 (12.5) | 25 (13.3) | 0.868 |
Emergency | 27 (3.6) | 0 (0.0) | 27 (14.4) | <0.001 |
OPCABG + CE | 9 (1.2) | 8 (1.4) | 1 (0.5) | 0.557 |
Dialysis | 1 (0.1) | 1 (0.2) | 0 (0.0) | 1.000 |
Positive inotropic drug | 730 (97.5) | 544 (97.0) | 186 (98.9) | 0.224 |
β-agonist | 725 (96.8) | 540 (96.3) | 185 (98.4) | 0.227 |
PDE-III inhibitor | 23 (3.1) | 18 (3.2) | 5 (2.7) | 0.894 |
CS | 40 (5.3) | 22 (3.9) | 18 (9.6) | 0.005 |
IABP | 28 (3.7) | 18 (3.2) | 10 (5.3) | 0.272 |
Preoperative IABP | 10 (2.0) | 6 (1.2) | 4 (40.0) | <0.001 |
Blood transfusion | 147 (19.6) | 103 (18.4) | 44 (23.4) | 0.161 |
Postoperative complications | 0.349 | |||
Cardiac insufficiency | 1 (2.8) | 1 (4.8) | 0 (0.0) | |
SIRS | 1 (2.8) | 0 (0.0) | 1 (6.7) | |
CPR | 1 (2.8) | 1 (4.8) | 0 (0.0) | |
Metabolic acidosis | 17 (47.2) | 10 (47.6) | 7 (46.7) | |
New renal failure | 5 (13.9) | 4 (19.1) | 1 (6.7) | |
Respiratory insufficiency | 4 (11.1) | 1 (4.8) | 3 (20.0) | |
Stroke | 7 (19.4) | 4 (19.0) | 3 (20.0) | |
Reoperation | 11 (1.5) | 5 (0.9) | 6 (3.2) | 0.055 |
POAF | 188 (25.1) | 0 (0.0) | 188 (100.0) | <0.001 |
Data are presented as mean ± standard deviation or n (%). POAF, postoperative atrial fibrillation; BSA, body surface area; BMI, body mass index; ICU, intensive care unit; LVM, left ventricular mass; LVMI, LVM index; SII, systemic immune-inflammation index; CHA2DS2-VASc, congestive heart failure, hypertension, age ≥75 years (doubled), diabetes, stroke (doubled), vascular disease, age 65 to 74 years, and sex category (female); HATCH, hypertension, age (above 75 years), transient ischemic attack or stroke, COPD, and heart failure; COPD, chronic obstructive pulmonary disease; LVEF, left ventricular ejection fraction; LAD, left atrial diameter; IVST, interventricular septal thickness; LVPWT, left ventricular posterior wall thickness; LVEDD, left ventricular end-diastolic dimension; ALT, alanine aminotransferase; AST, aspartate aminotransferase; GGT, gamma-glutamyl transferase; ALP, alkaline phosphatase; T-Bil, total bilirubin; D-Bil, direct bilirubin; IBIL, indirect bilirubin; TP, total protein; ALB, albumin; GLB, globulin; A/G, ALB/GLB ratio; TC, total cholesterol; TG, triglyceride; LDL-C, low-density lipoprotein cholesterol; HDL-C, high-density lipoprotein cholesterol; WBC, white blood cell; RBC, red blood cell; PLT, platelet; Hb, hemoglobin; LYM, lymphocyte; MONO, monocyte; NE, neutrophils; MPV, mean platelet volume; EOS, eosinophils; BAS, basophils; CRP, C-reactive protein; RDW-SD, red cell volume distribution width-standard deviation; RDW-CV, red cell volume distribution width-coefficient of variation; CHF, congestive heart failure; CVD, cerebrovascular disease; PVD, peripheral vascular disease; NYHA, New York Heart Association; PCI, percutaneous coronary intervention; LMD, left main disease; TVD, triple vessel disease; AMI, acute myocardial infarction; CAD, coronary artery disease; CCB, calcium channel blocker; ACE, angiotensin-converting enzyme; ARB, angiotensin-receptor blocker; OPACBG, off-pump coronary artery bypass grafting; CE, coronary endarterectomy; PDE-III, phosphodiesterase III; CS, calcium sensitizer; IABP, intra-aortic balloon pump; SIRS, systemic inflammatory response syndrome; CPR, cardiopulmonary resuscitation.
This study found that 25.1% (n=188) of patients had POAF. Previous studies reported a 20–40% incidence of POAF in patients undergoing CABG (1,23,24). Patients with AF had more comorbidities and were significantly older (65.32±8.20 vs. 61.20±8.75 years). Additionally, participants with POAF had a greater prevalence of comorbid conditions, including peripheral vascular disease, left ventricular dysfunction, and left atrial enlargement. We found no significant variation in the administration of beta blockers prior to surgery (P=0.227).
Feature selection and nomogram models
Univariable logistic regression analysis revealed variables in the derivation set that were significantly correlated with POAF (Table 2). These variables included age, left ventricular mass (LVM), LAD, interventricular septal thickness (IVST), urea, mean platelet volume (MVP), peripheral vascular disease, post-surgery complications and calcium sensitizer (CS) use. The variables that were significantly associated with POAF were then included in a stepwise regression, and the resulting variables were combined in a new predictive model for POAF. Since calcium ion sensitizers are commonly used in patients with postoperative hypocalcemia and poor contraction of the heart muscle, they are postoperative factors. To consider the predictive ability of preoperative factors and postoperative factors for POAF, it was decided to establish a prediction model respectively in this study. The final model variables with (model 1) and without (model 2) CSs are displayed as OR and 95% CI (Tables 3,4). User-friendly nomograms were generated for clinical use (Figure 2A,2B).
Table 2
Variables | OR (95% CI) | P |
---|---|---|
Age | 1.05 (1.02–1.07) | <0.001 |
LVM | 1.01 (1.00–1.01) | 0.005 |
LAD | 1.04 (1.02–1.06) | 0.001 |
IVST | 1.15 (1.02–1.31) | 0.028 |
Urea | 1.16 (1.06–1.28) | 0.003 |
MPV | 1.27 (1.06–1.55) | 0.012 |
Drinking history | 1.64 (1.05–2.54) | 0.027 |
Peripheral vascular disease | 1.61 (1.02–2.54) | 0.040 |
Post-surgery complications | 2.56 (1.05–6.09) | 0.034 |
CS | 3.88 (1.49–10.41) | 0.005 |
CHA2DS2-VASc score | 1.23 (1.08–1.40) | 0.002 |
HATCH score | 1.20 (1.01–1.44) | 0.039 |
POAF score | 1.79 (1.39–2.32) | <0.001 |
OR, odds ratio; CI, confidence interval; LVM, left ventricular mass; LAD, left atrial diameter; IVST, interventricular septal thickness; MPV, mean platelet volume; CS, calcium sensitizer; CHA2DS2-VASc, congestive heart failure, hypertension, age ≥75 years (doubled), diabetes, stroke (doubled), vascular disease, age 65 to 74 years, and sex category (female); HATCH, hypertension, age (above 75 years), transient ischemic attack or stroke, COPD, and heart failure; COPD, chronic obstructive pulmonary disease; POAF, postoperative atrial fibrillation.
Table 3
Variables | OR (95% CI) | P |
---|---|---|
Age | 1.05 (1.02–1.08) | <0.001 |
LVM | 1.01 (1.00–1.01) | 0.064 |
LAD | 1.03 (1.00–1.05) | 0.039 |
Urea | 1.07 (0.98–1.18) | 0.162 |
MPV | 1.21 (0.99–1.48) | 0.068 |
Drinking history | 1.59 (0.99–2.56) | 0.056 |
CS | 3.14 (1.10–9.21) | 0.033 |
POAF, postoperative atrial fibrillation; CS, calcium sensitizer; OR, odds ratio; CI, confidence interval; LVM, left ventricular mass; LAD, left atrial diameter; MPV, mean platelet volume.
Table 4
Variables | OR (95% CI) | P value |
---|---|---|
Age | 1.05 (1.02–1.08) | <0.001 |
LVM | 1.00 (1.00–1.01) | 0.075 |
LAD | 1.03 (1.00–1.05) | 0.024 |
Urea | 1.09 (0.99–1.20) | 0.093 |
MPV | 1.22 (1.00–1.49) | 0.057 |
Drinking history | 1.59 (0.99–2.54) | 0.056 |
POAF, postoperative atrial fibrillation; CS, calcium sensitizer; OR, odds ratio; CI, confidence interval; LVM, left ventricular mass; LAD, left atrial diameter; MPV, mean platelet volume.
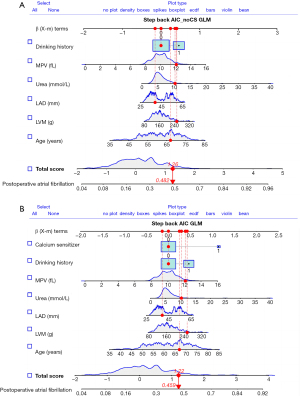
In the derivation set, the AUC-ROC of the new POAF risk prediction model 1 was 0.687 (95% CI: 0.6296–0.7449) in the derivation set, with the remaining scores showing lower discrimination compared with model 1: CHA2DS2-VASc (AUC-ROC =0.603, 95% CI: 0.5449–0.6610, P<0.05), POAF (AUC-ROC =0.628, 95% CI: 0.5729–0.6840, P>0.05), and HATCH (AUC-ROC =0.549, 95% CI: 0.5729–0.6840, P<0.05) (Table 5, Figure 3A).
Table 5
Risk models | Derivation, AUC-ROC (95% CI) | Validation, AUC-ROC (95% CI) |
---|---|---|
Model 1 | 0.687 (0.6296–0.7449) | 0.661 (0.5921–0.7307) |
Model 2 | 0.677 (0.6182–0.7354) | 0.665 (0.5959–0.7346) |
CHA2DS2-VASc | 0.603 (0.5449–0.6610)†,‡ | 0.644 (0.5706–0.7183) |
POAF | 0.628 (0.5729–0.6840) | 0.700 (0.6370–0.7640)‡ |
HATCH | 0.549 (0.5729–0.6840)†,‡ | 0.569 (0.4952–0.6424) |
Model 1: without CSs; model 2: without CSs. †, indicate comparing with model 1, there was differences; ‡, indicates comparing with model 2, there was differences. AUC-ROC, area under the ROC curve; ROC, receiver operating characteristic; CI, confidence interval; CHA2DS2-VASc, congestive heart failure, hypertension, age ≥75 years (doubled), diabetes, stroke (doubled), vascular disease, age 65 to 74 years, and sex category (female); POAF, postoperative atrial fibrillation; HATCH, hypertension, age (above 75 years), transient ischemic attack or stroke, COPD, and heart failure; COPD, chronic obstructive pulmonary disease; CS, calcium sensitizer.
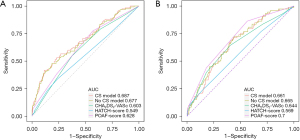
In the validation set, the AUC-ROC of the new POAF risk prediction model 1 was 0.661 (95% CI: 0.5921–0.7307), with the remaining scores showing similar discrimination compared with model 1: CHA2DS2-VASc (AUC-ROC =0.644, 95% CI: 0.5706–0.7183, P>0.05), POAF (AUC-ROC =0.700, 95% CI: 0.6370–0.7640, P>0.05), and HATCH (AUC-ROC =0.569, 95% CI: 0.4952–0.6424, P>0.05) (Table 5, Figure 3B).
Calibration curves of the two nomogram models and their clinical utility
The calibration curves of nomogram model 1 (with CSs) and model 2 (without CSs) in predicting the probability of POAF demonstrated good consistency between predictive POAF probability and observed recovery probability in both the derivation and validation cohorts (Figure 4). The decision curves for the two nomogram models in both cohorts (Figure 5A-5D) suggested that they were useful between threshold probabilities of 0.2 to 0.8 in the derivation cohort and between of 0.2 to 0.7 in the validation cohort.
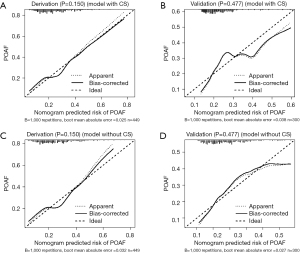
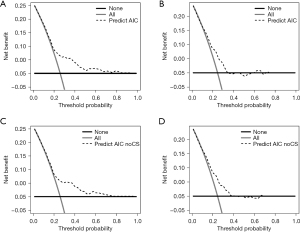
Optimal cutoffs of important factors
The best cutoff point for predicting left atrial diameter (LAD) for the new score was ≥39 mm (Figure S1) and that for age was ≥66 years (Figure S2), with a sensitivity of 82% (95% CI: 78–85%), a specificity of 65.9% (95% CI: 64–68%), and a negative predictive value of 92.9% (95% CI: 91–94%). Survival curves were plotted for the two groups according to cutoff values (log-rank P value, Figure 6). Figure 7 shows a comparison between the two groups based on the Mann-Whitney U test.
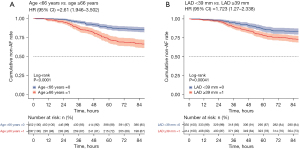
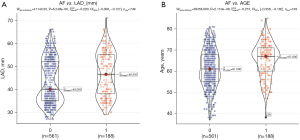
We further evaluated the predictive effect of adding LAD to the commonly used POAF prediction scoring system. We first incorporated the indicators of previous scores into the regression model to obtain the ROC curve and then compared the new ROC curve after LAD was added (Table 6). The comparison of AUC-ROCs of the improved risk prediction model with those of the traditional models was as follows: CHA2DS2-VASc + LAD (AUC-ROC =0.65, 95% CI: 0.602–0.693) vs. CHA2DS2-VASc (AUC-ROC =0.62, 95% CI: 0.572–0.663, P=0.057); POAF + LAD (AUC-ROC =0.68, 95% CI: 0.635–0.721) vs. POAF (AUC-ROC =0.66, 95% CI: 0.614–0.698, P<0.001); and HATCH + LAD (AUC-ROC =0.61, 95% CI: 0.563–0.657) vs. HATCH (AUC-ROC =0.56, 95% CI: 0.510–0.603, P=0.009) (Table 6). After the addition of LAD to HATCH, the POAF scoring system significantly improved, while the improvement of adding LAD to CHA2DS2-VASc was not obvious (Figure 8).
Table 6
Risk models | AUC-ROC | 95% CI | P | |
---|---|---|---|---|
Lower bound | Upper bound | |||
CHA2DS2-VASc + LAD | 0.65 | 0.602 | 0.693 | 0.057 |
CHA2DS2-VASc | 0.62 | 0.572 | 0.663 | |
POAF + LAD | 0.68 | 0.635 | 0.721 | 0.009 |
POAF | 0.66 | 0.614 | 0.698 | |
HATCH + LAD | 0.61 | 0.563 | 0.657 | <0.001 |
HATCH | 0.56 | 0.510 | 0.603 |
AUC-ROC, area under the ROC curve; ROC, receiver operating characteristic; CI, confidence interval; CHA2DS2-VASc, congestive heart failure, hypertension, age ≥75 years (doubled), diabetes, stroke (doubled), vascular disease, age 65 to 74 years, and sex category (female); POAF, postoperative atrial fibrillation; HATCH, hypertension, age (above 75 years), transient ischemic attack or stroke, COPD, and heart failure; COPD, chronic obstructive pulmonary disease; LAD, left atrial diameter.
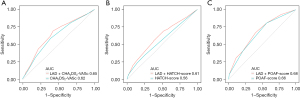
Discussion
Key findings
This retrospective study developed a new clinical model produced by a newly established scoring system incorporating the variables derived from preoperative stepwise regression that had the highest predictive value for AF initiation in patients following cardiac surgery. The new scoring system performed well showing good discrimination and calibration ability as well as a high level of predictive accuracy.
Strengths and limitations
The benefit of this scoring system is the inclusion of simple preoperative variables that can appropriately predict the development of POAF from the moment of patient admission so that the patient can receive preventive measures, such as medication or atrial pacing, depending on the level of assessed risk. The present study not only combined medical history and blood sampling tests but also incorporated indicators from echocardiography, combining several aspects to comprehensively assess the occurrence of POAF in patients, yielding a performance superior to that of previous models. Since this study only investigated the effect of left atrial size on POAF after OPCABG, the scoring system is not perfect, and validation with a large sample size is needed to further refine the postoperative evaluation system.
Comparison with similar research
A previous study reported the SII as a new inflammatory marker that integrates neutrophil, lymphocyte, and platelet counts to more accurately represent the body’s overall inflammatory condition (9). It was found that preoperative SII performs well in predicting POAF in patients with CABG and has a better and more effective ability to identify POAF in patients with CABG compared with other inflammatory biomarkers (10,11). However, preoperative SII was not found to be an independent risk factor for POAF in this study and was not included in the risk assessment model, which may be related to the fact that this study examined non-extracorporeal circulation CABG, which to some extent decreases the postoperative systemic inflammatory response and myocardial injury. However, it has also been noted that non-extracorporeal circulation CABG is not superior to extracorporeal circulation CABG in reducing inflammation and myocardial damage nor does it completely avoid the difficulties of extracorporeal circulation CABG (25). Therefore, the difference in postoperative systemic inflammatory response between on-pump CABG and OPCABG needs to be discussed and studied further.
Earlier investigations into this subject indicated that undesirable outcomes are more common in patients with postoperative recurrent AF and that these patients have longer hospitalizations, more infections, and more renal and neurological problems than do those with single-onset AF (2,3). The same conclusion was reached in the present study. Among the previous scoring systems, the POAF score is the only one established and validated for the prediction of POAF in patients undergoing CABG or valve surgery, and the discriminatory power of this score is moderate. The HATCH score was created by de Vos (26) for the prediction of AF progression from paroxysmal to persistent, and it involves simple clinical parameters, which are easy to calculate. Every variable of the HATCH score is related to long-term left atrial expansion, which may be essential for POAF development.
In this study, LAD was added to the CHA2DS2-VASc, HATCH, and POAF scores and compared with their previous scoring systems. The results indicated that the HATCH and POAF scores had significantly improved predictive power after this adjustment, while the CHA2DS2-VASc was not improved. Moreover, the improvements were not significant. However, a previous study (20) aimed at investigating the relationship between HATCH score and AF after cardiac surgery (AFCS) after isolated CABG showed that the HATCH score was an independent predictor of AF after CABG (OR =1.334, 95% CI: 1.022–1.741, P=0.034) but was less discriminatory in predicting AFCS, with an AUC-ROC of 0.57. In a prospective study, Chua et al. (27) examined 277 patients who underwent CABG or valve surgery and reported the CHA2DS2-VASc (AUC-ROC =0.87), which was higher than that found in this study.
Interpretation of findings
The results of the present study suggest LAD (OR =1.03, 95% CI: 1.00–1.05, P=0.039) to be a predictive factor for POAF in patients with coronary artery disease undergoing OPCABG. Previous studies have shown that structural remodeling of the atrium is important in the pathogenesis of dynamic AF (28,29), which may also be important for POAF. A large body of supporting evidence indicates that left atrial abnormalities are a risk factor for the development of AFCS and that left atrial enlargement or increased LAD (30,31) is strongly associated with the development of POAF. With the increase of the inner diameter of the left atrium, the structure of the heart changes, and the electrical remodeling of the atrium leads to poor coordination of atrial contraction and the inability to maintain normal myocardial electrical activity.
Furthermore, aging itself is involved in a variety of proarrhythmic processes, including atrial electrical and structural remodeling, disturbances in calcium homeostasis, and enhanced atrial ectopic activity/increased susceptibility to reentry induction (32). The balance between sympathetic and parasympathetic modulation of cardiac electrophysiology changes with age, and with increasing age there is an overall decline in autonomic control of the heart, dominated by sympathetic modulation and relatively diminished by vagal modulation (33). Aging is an inherently time-dependent risk factor for AF, although its dynamics are slow and unidirectional. Thus, aging will be one of the main reasons for the increase in the prevalence of AF in the future (32).
Moreover, this study also revealed calcium receptor sensitizers (OR =3.14, 95% CI: 1.10–9.21, P=0.033) to be an independent risk factor. The calcium ion sensitizer used in this study was levosimendan, a positive inotropic compound with vasodilatory properties (34). Its main mechanism of action involves opening the adenosine triphosphate-sensitive potassium (KATP) channels in vascular smooth muscle cells (35), inducing vasodilation of coronary arteries (36), and thereby improving postoperative arrhythmia (37). However, in this study, calcium ion sensitizers (OR =3.14, 95% CI: 1.10–9.21, P=0.033) were independent risk factors, and, therefore, they should be applied cautiously after surgery. A previous study also confirmed that levosimendan may increase the risk of postoperative arrhythmia (38). There is evidence that calcium overload due to reperfusion of ischemic areas is one of the potential arrhythmogenic mechanisms of arrhythmia after cardiac surgery (39). Therefore, the application of calcium ion sensitizers or calcium-containing electrolyte solutions for postoperative low-serum calcium should be discussed to avoid the occurrence of arrhythmia caused by calcium overload.
A retrospective study compared the predictive ability of POAF score, CHA2DS2-VASc, and AF risk index in patients undergoing elective CABG surgery or valve surgery (40). The incidence of POAF was 32.6% significantly higher than that in this study (25.1%), and the three scoring systems exhibited limited discriminatory ability, with an AUC-ROC of 0.66 (95% CI: 0.62–0.70) for the POAF score, AUC-ROC of 0.77 (95% CI: 0.74–0.81) for the McSPI AFRisk score and AUC-ROC of 0.58 (95% CI: 0.54–0.62) for the CHA2DS2-VASc score. The predictive power of perioperative AF risk scores in patients undergoing cardiac surgery was also compared and found to be limited in predicting POAF, with AUC-ROCs of 0.58 and 0.66 for CHA2DS2-VASc and PoAF scores, respectively (40). Compared with the present study, all of these studies were retrospective studies and may carry their own bias, and the resulting scoring criteria may not be representative of other centers. More prospective studies are needed to determine whether the scoring system can be generalized to a broader group of patients. In a recent large-cohort study, a new clinical model COM-AF (OR =1.91) was created from the variables with the highest predictive value in the CHA2DS2-VASc, HATCH, and POAF scoring systems (95% CI: 1.63–2.23); it was also compared with the previous scoring system and was found to have better predictive power compared with the original model (20).
Implications and actions needed
An increasing number of studies have shown that POAF can reduce the likelihood of postoperative complications if it is prevented early (3,41,42). Therefore, there is a need for continuous improvement of relevant scoring criteria and new prediction models for earlier preventive measures related to POAF.
Compared with the original system, the new LAD-based risk system was better at predicting AFCS. Moreover, the addition of LAD to the previous scoring system provided better predictive power. Therefore, the addition of LAD to the scoring system should be considered in the future, but further studies involving larger samples and multicenter prospective validation are needed to confirm this.
Conclusions
A nomogram was developed and validated for patients with POAF after OPCABG that provided better predictive accuracy than did the original risk system. It thus may assist doctors to facilitate more accurate management of patients with POAF.
Acknowledgments
We would like to thank Dr. Wenxing Peng (Department of Cardiac Surgery, Beijing Anzhen Hospital, Capital Medical University, Beijing, China) for her help in data analysis. We also appreciate Dr. Xiaonan Li (Department of Cardiac Surgery, Beijing Chaoyang Hospital, Capital Medical University, Beijing, China) and Dr. Wen Liu (Department of Cardiology, Affiliated Hospital of Inner Mongolia Medical University, Inner Mongolia, China) for drawing the figures in our paper.
Funding: This work was funded by the Beijing Municipal Science & Technology Commission (No. Z191100006619094).
Footnote
Reporting Checklist: The authors have completed the TRIPOD reporting checklist. Available at https://jtd.amegroups.com/article/view/10.21037/jtd-22-1706/rc
Data Sharing Statement: Available at https://jtd.amegroups.com/article/view/10.21037/jtd-22-1706/dss
Conflicts of Interest: All authors have completed the ICMJE uniform disclosure form (available at https://jtd.amegroups.com/article/view/10.21037/jtd-22-1706/coif). The authors have no conflicts of interest to declare.
Ethical Statement: The authors are accountable for all aspects of the work in ensuring that questions related to the accuracy or integrity of any part of the work are appropriately investigated and resolved. The study was conducted in accordance with the Declaration of Helsinki (as revised in 2013). The study was approved by the Ethics Committee of Beijing Anzhen Hospital (No. 2023075X) and individual consent for this retrospective analysis was waived.
Open Access Statement: This is an Open Access article distributed in accordance with the Creative Commons Attribution-NonCommercial-NoDerivs 4.0 International License (CC BY-NC-ND 4.0), which permits the non-commercial replication and distribution of the article with the strict proviso that no changes or edits are made and the original work is properly cited (including links to both the formal publication through the relevant DOI and the license). See: https://creativecommons.org/licenses/by-nc-nd/4.0/.
References
- Filardo G, Damiano RJ Jr, Ailawadi G, et al. Epidemiology of new-onset atrial fibrillation following coronary artery bypass graft surgery. Heart 2018;104:985-92. [Crossref] [PubMed]
- Lowres N, Mulcahy G, Jin K, et al. Incidence of postoperative atrial fibrillation recurrence in patients discharged in sinus rhythm after cardiac surgery: a systematic review and meta-analysis. Interact Cardiovasc Thorac Surg 2018;26:504-11. [Crossref] [PubMed]
- Eikelboom R, Sanjanwala R, Le ML, et al. Postoperative Atrial Fibrillation After Cardiac Surgery: A Systematic Review and Meta-Analysis. Ann Thorac Surg 2021;111:544-54. [Crossref] [PubMed]
- Hu YF, Chen YJ, Lin YJ, et al. Inflammation and the pathogenesis of atrial fibrillation. Nat Rev Cardiol 2015;12:230-43. [Crossref] [PubMed]
- Jacob KA, Nathoe HM, Dieleman JM, et al. Inflammation in new-onset atrial fibrillation after cardiac surgery: a systematic review. Eur J Clin Invest 2014;44:402-28. [Crossref] [PubMed]
- Gibson PH, Cuthbertson BH, Croal BL, et al. Usefulness of neutrophil/lymphocyte ratio as predictor of new-onset atrial fibrillation after coronary artery bypass grafting. Am J Cardiol 2010;105:186-91. [Crossref] [PubMed]
- Bruins P, te Velthuis H, Yazdanbakhsh AP, et al. Activation of the complement system during and after cardiopulmonary bypass surgery: postsurgery activation involves C-reactive protein and is associated with postoperative arrhythmia. Circulation 1997;96:3542-8. [Crossref] [PubMed]
- Navani RV, Baradi A, Colin Huang KL, et al. Preoperative Platelet-to-Lymphocyte Ratio Is Not Associated With Postoperative Atrial Fibrillation. Ann Thorac Surg 2020;110:1265-70. [Crossref] [PubMed]
- Yang YL, Wu CH, Hsu PF, et al. Systemic immune-inflammation index (SII) predicted clinical outcome in patients with coronary artery disease. Eur J Clin Invest 2020;50:e13230. [Crossref] [PubMed]
- Selcuk M, Cinar T, Saylik F, et al. Predictive Value of Systemic Immune Inflammation Index for Postoperative Atrial Fibrillation in Patients Undergoing Isolated Coronary Artery Bypass Grafting. Medeni Med J 2021;36:318-24. [Crossref] [PubMed]
- Hinoue T, Yatabe T, Nishida O. Prediction of postoperative atrial fibrillation with the systemic immune-inflammation index in patients undergoing cardiac surgery using cardiopulmonary bypass: a retrospective, single-center study. J Artif Organs 2023;26:112-8. [Crossref] [PubMed]
- Seo EJ, Hong J, Lee HJ, et al. Perioperative risk factors for new-onset postoperative atrial fibrillation after coronary artery bypass grafting: a systematic review. BMC Cardiovasc Disord 2021;21:418. [Crossref] [PubMed]
- Yamashita K, Hu N, Ranjan R, et al. Clinical Risk Factors for Postoperative Atrial Fibrillation among Patients after Cardiac Surgery. Thorac Cardiovasc Surg 2019;67:107-16. [Crossref] [PubMed]
- Mariscalco G, Biancari F, Zanobini M, et al. Bedside tool for predicting the risk of postoperative atrial fibrillation after cardiac surgery: the POAF score. J Am Heart Assoc 2014;3:e000752. [Crossref] [PubMed]
- Chen YL, Zeng M, Liu Y, et al. CHA(2)DS(2)-VASc Score for Identifying Patients at High Risk of Postoperative Atrial Fibrillation After Cardiac Surgery: A Meta-analysis. Ann Thorac Surg 2020;109:1210-6. [Crossref] [PubMed]
- Engin M, Aydın C. Investigation of the Effect of HATCH Score and Coronary Artery Disease Complexity on Atrial Fibrillation after On-Pump Coronary Artery Bypass Graft Surgery. Med Princ Pract 2021;30:45-51. [Crossref] [PubMed]
- Xu H, Zhang GD, Fan GP, et al. Preoperative plasma predictive factors of new-onset atrial fibrillation after coronary artery bypass graft surgery: A propensity score matching study. Beijing Da Xue Xue Bao Yi Xue Ban 2021;53:1139-43. [PubMed]
- Nardi F, Diena M, Caimmi PP, et al. Relationship between left atrial volume and atrial fibrillation following coronary artery bypass grafting. J Card Surg 2012;27:128-35. [Crossref] [PubMed]
- Folla CO, Melo CC, Silva RC. Predictive factors of atrial fibrillation after coronary artery bypass grafting. Einstein (Sao Paulo) 2016;14:480-5. [Crossref] [PubMed]
- Burgos LM, Ramírez AG, Seoane L, et al. New combined risk score to predict atrial fibrillation after cardiac surgery: COM-AF. Ann Card Anaesth 2021;24:458-63. [Crossref] [PubMed]
- DeLong ER, DeLong DM, Clarke-Pearson DL. Comparing the areas under two or more correlated receiver operating characteristic curves: a nonparametric approach. Biometrics 1988;44:837-45. [Crossref] [PubMed]
- Youden WJ. Index for rating diagnostic tests. Cancer 1950;3:32-5. [Crossref] [PubMed]
- Phan K, Ha HS, Phan S, et al. New-onset atrial fibrillation following coronary bypass surgery predicts long-term mortality: a systematic review and meta-analysis. Eur J Cardiothorac Surg 2015;48:817-24. [Crossref] [PubMed]
- Dimagli A, Di Tommaso E, Bruno VD. Commentary: The importance of being predictable: Postoperative atrial fibrillation. J Thorac Cardiovasc Surg 2021; Epub ahead of print. [Crossref] [PubMed]
- Shaefi S, Mittel A, Loberman D, et al. Off-Pump Versus On-Pump Coronary Artery Bypass Grafting-A Systematic Review and Analysis of Clinical Outcomes. J Cardiothorac Vasc Anesth 2019;33:232-44. [Crossref] [PubMed]
- de Vos CB, Pisters R, Nieuwlaat R, et al. Progression from paroxysmal to persistent atrial fibrillation clinical correlates and prognosis. J Am Coll Cardiol 2010;55:725-31. [Crossref] [PubMed]
- Chua SK, Shyu KG, Lu MJ, et al. Clinical utility of CHADS2 and CHA2DS2-VASc scoring systems for predicting postoperative atrial fibrillation after cardiac surgery. J Thorac Cardiovasc Surg 2013;146:919-926.e1. [Crossref] [PubMed]
- Iwasaki YK, Nishida K, Kato T, et al. Atrial fibrillation pathophysiology: implications for management. Circulation 2011;124:2264-74. [Crossref] [PubMed]
- Burstein B, Nattel S. Atrial fibrosis: mechanisms and clinical relevance in atrial fibrillation. J Am Coll Cardiol 2008;51:802-9. [Crossref] [PubMed]
- Greenberg JW, Lancaster TS, Schuessler RB, et al. Postoperative atrial fibrillation following cardiac surgery: a persistent complication. Eur J Cardiothorac Surg 2017;52:665-72. [Crossref] [PubMed]
- Quan D, Huang H, Kong B, et al. Predictors of late atrial fibrillation recurrence after cryoballoon-based pulmonary vein isolation: a meta-analysis. Kardiol Pol 2017;75:376-85. [Crossref] [PubMed]
- Laredo M, Waldmann V, Khairy P, et al. Age as a Critical Determinant of Atrial Fibrillation: A Two-sided Relationship. Can J Cardiol 2018;34:1396-406. [Crossref] [PubMed]
- Abhishekh HA, Nisarga P, Kisan R, et al. Influence of age and gender on autonomic regulation of heart. J Clin Monit Comput 2013;27:259-64. [Crossref] [PubMed]
- Papp Z, Édes I, Fruhwald S, et al. Levosimendan: molecular mechanisms and clinical implications: consensus of experts on the mechanisms of action of levosimendan. Int J Cardiol 2012;159:82-7. [Crossref] [PubMed]
- Pataricza J, Krassói I, Höhn J, et al. Functional role of potassium channels in the vasodilating mechanism of levosimendan in porcine isolated coronary artery. Cardiovasc Drugs Ther 2003;17:115-21. [Crossref] [PubMed]
- Kaheinen P, Pollesello P, Levijoki J, et al. Levosimendan increases diastolic coronary flow in isolated guinea-pig heart by opening ATP-sensitive potassium channels. J Cardiovasc Pharmacol 2001;37:367-74. [Crossref] [PubMed]
- Kowalczyk M, Banach M, Lip GY, et al. Levosimendan - a calcium sensitising agent with potential anti-arrhythmic properties. Int J Clin Pract 2010;64:1148-54. [Crossref] [PubMed]
- Frommeyer G, Kohnke A, Ellermann C, et al. Experimental evidence for a severe proarrhythmic potential of levosimendan. Int J Cardiol 2017;228:583-7. [Crossref] [PubMed]
- Pivatto Júnior F, Teixeira Filho GF, Sant'anna JR, et al. Advanced age and incidence of atrial fibrillation in the postoperative period of aortic valve replacement. Rev Bras Cir Cardiovasc 2014;29:45-50. [Crossref] [PubMed]
- Waldron NH, Cooter M, Piccini JP, et al. Predictive ability of perioperative atrial fibrillation risk indices in cardiac surgery patients: a retrospective cohort study. Can J Anaesth 2018;65:786-96. [Crossref] [PubMed]
- Gaudino M, Sanna T, Ballman KV, et al. Posterior left pericardiotomy for the prevention of atrial fibrillation after cardiac surgery: an adaptive, single-centre, single-blind, randomised, controlled trial. Lancet 2021;398:2075-83. [Crossref] [PubMed]
- van Boven WJ, de Groot JR, Kluin J. A short cut to prevent postoperative atrial fibrillation. Lancet 2021;398:2052-3. [Crossref] [PubMed]