Predicting key gene related to immune infiltration and myofibroblast-like valve interstitial cells in patients with calcified aortic valve disease based on bioinformatics analysis
Highlight box
Key findings
• CTHRC1 was identified as the key gene of CAVD. CTHRC1 highly expressed in the VIC subpopulation, which abundantly distributed in the stage of calcification.
What is known and what is new?
• The myofibroblast phenotype of VICs results in pathological fibrosis and eventually contributes to the transition to osteoblast phenotype that promotes calcium phosphate deposition. Inflammatory response mainly mediates the initiation phase of CAVD.
• CTHRC1 highly expressed in the VIC subpopulation, and correlation analysis demonstrated that CTHRC1 was correlated with mast cells mostly.
What is the implication, and what should change now?
• CTHRC1 was a key gene of CAVD and CTHRC1 might participate in the potential molecular pathways involved in the connection between infiltrating immune cells and myofibroblast phenotype VICs. Experiments are needed to show the connection between VICs and immune cells.
Introduction
Calcified aortic valve disease (CAVD) is a common cardiovascular disorder affecting between 13% of the population aged 65 and older (1), and the disease burden is expected to double over the next 50 years due to an ever-increasing aging population (2). When the remodeling of valve tissues is sufficiently severe to result in hemodynamic changes at the aortic valve, CAVD manifests as aortic stenosis (AS), which is associated with high morbidity and mortality, with a 5-year mortality of 56% and 67% of moderate and severe aortic stenosis respectively in a large Australian registry (3). Currently, there is no pharmacological treatment for CAVD. The only treatment available to patients with symptomatic severe AS is to implant mechanical or bioprosthetic valves either surgically or percutaneously (through a catheter). However, the intervention has several serious problems including anticoagulation therapy for life, deterioration and the substantial societal costs (estimated at £13,000 per patient over 5 years) (4). Therefore, identifying more suitable treatment targets or biomarkers to prevent progressive leaflet calcification or to delay the time to valve replacement is urgent.
CAVD is characterized by fibro-calcific remodeling of aortic valves, which is a progressive process involving lipoprotein deposition, chronic inflammation, myoblastic transition, and osteoblastic transition of valve interstitial cells (VICs) (5-7). Lipid-lowering therapy has failed to suppress CAVD progression (8). Inflammatory response mainly mediates the initiation phase of CAVD (9). The endothelial damage results in lipid infiltration and subsequent oxidation, initiating the inflammatory response within the valvular endothelium (10). Macrophages, neutrophils and mast cells have been proved associated with valvular thickening and the increase in osseous metaplasia within the valve (11-15), and the pro-inflammatory cytokines secreted by immune cells were identified to mediate the inflammatory response during AS (16,17). The propagation phase is characterized by the myofibroblast and osteoblast-like activation of VICs (10). VICs exist primarily as quiescent fibroblasts to maintain valve function and homeostasis (18). During the propagation phase, VICs transition to pro-fibrotic myofibroblast phenotype mediated by secreted biochemical cues (e.g., pro-inflammatory cytokines and growth factors) (19-21). The myofibroblast phenotype of VICs results in pathological fibrosis and eventually contributes to the transition to osteoblast phenotype that promotes calcium phosphate deposition (22). It is known that preosteoblasts undergo a proliferative phase before fully committing to osteoblast-like cells (23). Although previous studies suggested several pro-inflammatory cytokines participate in the eventual differentiation of myofibroblasts to osteoblast-like cells in the valve (18,24), only a few studies have explored the mechanism of the interaction between immune cells and myofibroblast-like phenotype of VICs in aortic valve calcification.
In this study, we downloaded the microarray dataset of CAVD from the Gene Expression Omnibus (GEO) database and then used machine learning algorithms to screen the markers of CAVD. Additionally, we used a combination of single-cell sequencing technology and CIBERSORT, an analysis tool using RNA-seq data to evaluate the expression of immune cells (25), to conduct target verification and explore underlying molecular mechanism of the interaction between immune cells and myofibroblast-like VICs. We present this article in accordance with the TRIPOD reporting checklist (available at https://jtd.amegroups.com/article/view/10.21037/jtd-23-72/rc).
Methods
Microarray data download
We used the “GEOquery” package (26) of R software (version 4.1.0, http://r-project.org/) to download human calcified aortic valve tissue sample datasets GSE12644 (27), GSE51472 (28) and GSE83453 (29) from the GEO (https://www.ncbi.nlm.nih.gov/geo/) database (30). GSE12644 and GSE51472 are both based on GPL570 platform. The inclusion criteria of the samples were as follows: calcified and normal aortic valve, excluding sclerotic aortic valve. Calcified aortic valves were collected from patients undergoing aortic valve replacement. Normal aortic valves were collected from hearts harvested at the time of cardiac transplantation or aortic replacement. GSE12644 contains 10 calcification samples and 10 normal samples, and GSE51472 dataset contained 5 calcification and 5 normal samples. In addition, we used GSE83453, based on GPL10558 platform, to serve as the validation cohort, which contains 9 calcification samples and 8 normal samples.
Microarray data processing
Raw data of GSE12644 and GSE51472 datasets were read through the “affy” package (31), and the Robust Multi-Array Average (RMA) algorithm was used for background correction and data normalization. The gene expression matrix of GSE12644 and GSE51472 were then combined, and the inter-batch difference was removed using the “sva” package (32). The effect of inter-sample correction was demonstrated using a two-dimensional principal component analysis (PCA) cluster plot. Differentially expressed genes (DEGs) was screened by the “limma” package (33), DEGs with P<0.05 and |log2FC|>1 were considered statistically significant. Subsequently, we used “clusterProfiler” package (34) to perform Gene Ontology (GO) and Kyoto Encyclopedia of Genes and Genomes (KEGG) enrichment analyses of DEGs. Gene set enrichment analysis (GSEA) was performed on the gene expression matrix through the “clusterProfiler” package and “c2.cp.kegg.v7.0.symbols.gmt” from the Molecular Signatures Database (MSigDB) was selected as the reference gene set (35). A false discovery rate (FDR) <0.25 and P<0.05 was considered significant enrichment.
Human specimen and ethics
Aortic valve tissue specimens were obtained from patients underwent surgical aortic valve replacement or heart transplantation at Fuwai Hospital in Beijing, China. Once collected, the tissues were submerged in Dulbecco’s modified Eagle’s medium (DMEM, Gibco, USA) for cell isolation. The study was conducted in accordance with the Declaration of Helsinki (as revised in 2013). The study protocol was approved by the Institutional Review Board of Fuwai Hospital, Peking Union Medical College, Beijing, China. Written informed consent was obtained from each patient.
Single cell sequencing data processing
Aortic valve tissues were obtained from patients and prepared single-cell suspension to perform droplet sequencing. A paired-end sequencing library was prepared using the Nextera XT DNA library preparation kit (Illumina, San Diego, CA, USA) and libraries were sequenced using paired-end sequencing in samples sent to GenomeScan. Single-cell expression data were processed using the FastQC to perform quality control and low-quality reads were filtered by cutadapt. Single-cell expression data were then used to map read to the reference genome (hg38, UCSC) based on STAR (2.5.2b). Gene annotation was implemented using featureCounts according to GENECODE (GRCh38.81). Cells with more than 500 genes and less than 20% mitochondrial genes were retained.
Data integration was performed by Seurat (2.0.1). Unique molecular identifiers (UMIs) were calculated by Seurat to identify genes with significant expression variation and then used for PCA. The number of principal components for downstream analysis was obtained by evaluating principal components and corresponding genes. The above principal components were used to cluster the cells, and T-distributed Stochastic Neighbor Embedding (t-SNE) was used to visualize the clustering results. Pseudotime trajectory was constructed using semi-unsupervised Monocle. GO and KEGG enrichment analysis of differentially expressed genes was performed by DAVID (6.8).
Screening diagnostic markers
We combined least absolute shrinkage and selection operator (LASSO) logistic regression (36), support vector machine-recursive feature elimination (SVM-RFE) (37) and random forest (RF) (38) to perform feature selection to screen diagnostic markers for CAVD. The expression matrix of the GSE12644 and GSE51472 datasets were merged into an independent dataset after quality control, and then the diagnostic efficiency of the obtained diagnostic marker was determined based on this independent dataset. The LASSO algorithm was applied with the “glmnet” package (39) using 10-fold across-validation. SVM-RFE is a machine learning method based on support vector machine, which is used to find the best variables by deleting SVM-generated eigenvectors. SVM module was established to further identify the diagnostic utility of the biomarkers in CAVD by “e1071” package with the 10-fold cross validation (40). The RF algorithm was a machine learning algorithm based on decision tree theory classified according to its ability to deal with high-dimensional data and select the most informative gene clusters. We integrated LASSO, SVM-RFE and RF algorithms for further analysis. A two-sided P<0.05 was considered to be statistically significant.
Evaluation of immune cell infiltration
The gene expression matrix data were uploaded to CIBERSORT. CIBERSORT presents cell-type identification by estimating relative subsets of RNA transcripts. Newman et al. designed and validated a leukocyte gene signature matrix, termed LM22. LM22 is a gene matrix that contains 547 white blood cells characteristic genes to differentiate 22 types of immune cells, including myeloid subgroup, natural killer (NK) cells, naive and memory B-cells, and seven types of T-cells (25). We used CIBERSORT in combination with the LM22 characteristic matrix to estimate the proportion of 22 human immune cell phenotypes. Moreover, CIBERSORT apply linear support vector regression to deconvolve a given mixture based the genes from the signature matrix. Data with a CIBERSORT P value <0.05 were filtered and reserved for the following analysis. Proportions of infiltrating immune cells were visualized in R software using “ggplot2” package and “pheatmap” package. The difference of immune cells infiltration between aortic valve tissue samples from CAVD patients and normal individuals were shown in the boxplot using the “ggplot” package.
Correlation analysis between diagnostic markers and infiltrating immune cells
Spearman correlation analysis was used to identify genes significantly associated with immune cell abundance. The Spearman correlation coefficient was used to access the threshold effect, with r>0.6 and P<0.05, indicating a significant threshold effect between studies. The correlation between biomarkers and immune infiltration was visualized by the “ggplot2” package.
Statistical analysis
Statistical analyses and figures were implemented and obtained using R software (version 4.1.0). The pheatmap package was applied to constructed the expression heat maps of important genes and immune infiltration in calcified aortic valves and normal aortic valves. The area under the curve (AUC) value in the receiver operating characteristic (ROC) curve was used to evaluate the diagnostic efficacy. P<0.05 was considered statistically significant.
Results
Identified DEGs in calcified aortic valve sample and functional correlation analysis showed DEGs mainly associated with extracellular matrix remodeling and immune response
The batch effect was removed after merging the GSE12644 and GSE51472 datasets. The merged gene expression matrix was then normalized and processed, and it is presented in a two-dimensional PCA cluster diagram before and after normalization (Figure 1A,1B). The results showed that the clustering of the two groups of samples was more obvious after normalization, indicating that the sample source was reliable. 205 DEGs were identified using the “limma” package form the gene expression matrix, as shown in the heat map (Figure 1C).
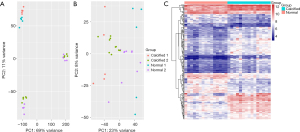
GO analysis results showed that DEGs mainly related to extracellular matrix structural constituent, serine-type endopeptidase activity, serine-type peptidase activity, serine hydrolase activity and extracellular matrix structural constituent conferring tensile strength (Figure 2A). The relationship between biological processes terms and each DEG was shown in Figure 2B. KEGG pathway analysis shows that DEGs were mainly enriched in the PI3K-Akt signaling pathway, ECM-receptor interaction, focal adhesion, protein digestion and absorption and complement and coagulation cascades (Figure 2C). Additionally, GSEA results showed that the enriched pathways mainly involved chemokine signaling pathway, cytokine receptor interaction and hematopoietic cell lineage (Figure 2D). These results indicate that collagen matrix deposition and the immune response significantly affect the process of CAVD.
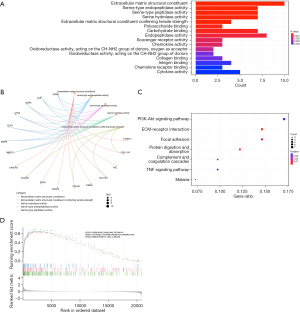
Screening CTHRC1 as characteristic gene via the comprehensive strategy
We used three different machine learning algorithms to screen key DEGs as biomarkers of CAVD. The LASSO logistic regression algorithm with 10-fold cross validation was used to identify 12 genes from robust DEGs as diagnostic markers for CAVD (Figure 3A,3B). Ten genes were identified as potential biomarkers by SVM-RFE algorithms (Figure 3C). Fourteen genes were identified using RF algorithm. The gene markers obtained by the three algorithms were overlapped, and finally one diagnostic related gene [collagen triple helix repeat containing 1 (CTHRC1)] was obtained. The diagnostic effectiveness of CTHRC1 was further validated in another independent dataset (GSE83453) with an AUC of 0.917 (Figure 3D). CTHRC1 was initially found in the balloon-injured rat arteries (41), and subsequent studies found that CTHRC1 was involved in many physiological and pathological processes, including vascular remodeling, tissue fibrosis, bone formation, developmental morphogenesis, inflammatory arthritis and cancer progression (42-46).
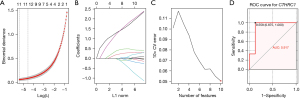
Single-cell RNA sequencing showed CTHRC1 highly expressed in a VIC subpopulation
We performed PCA on gene expression variability across all 4,314 cells and then classified the cells into cell-type groups as shown in Figure 4A,4B and Table 1. CTHRC1 highly expressed in the VIC subpopulation, cluster 4 [valve interstitial cells-fibroblast 2 (VIC-FB2)]. VIC-FB2 had an abundance in myxoid, fibrotic, and calcified valves, with the highest proportion in calcified valves. VIC-FB2 was barely emerged in normal aortic valve sample (Figure 4C). We performed pseudotime differentiation trajectory analysis using Monocle to determine how the newly identified cell types were related to developmental states. The trajectory timing of VIC-FB2 was abundantly distributed in the stage of calcification (Figure 4D). GO analysis of cell cluster VIC-FB2 showed highly expressed genes related to collagen fibril organization, negative regulation of angiogenesis, endodermal cell differentiation, skeletal system development, positive regulation of smooth muscle cell proliferation and osteoblast differentiation (Figure 4E), which turned out to be related to pathways driving the calcification process, indicating VIC-FB2 played an important role in CAVD.
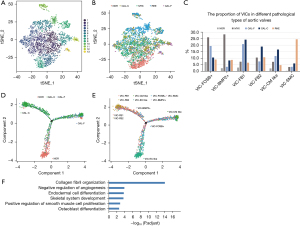
Table 1
Cell cluster | 1 | 2 | 3 | 4 | 5 | 6 | 7 | 8 | 9 | 10 | 11 | 12 | 13 |
---|---|---|---|---|---|---|---|---|---|---|---|---|---|
Cell, n | 601 | 489 | 482 | 426 | 408 | 358 | 347 | 244 | 233 | 200 | 163 | 96 | 69 |
Cell type | VIC-FOSB+ | VIC-FB1 | VIC-BMP2+ | VIC-FB2 | VIC-EC like | VIC-SMC | VIC-CM like | EC-CD34+ | MC-MRC1+/CD86+ | MC like | T cell | CM | B cell |
VIC-FOSB+, valve interstitial cell-FosB Proto-Oncogene positive; VIC-FB1, valve interstitial cell-fibroblast 1; VIC-BMP2+, valve interstitial cell-bone morphogenetic protein 2 positive; VIC-FB2, valve interstitial cell-fibroblast 2; VIC-EC like, valve interstitial cell-endothelial cell like; VIC-SMC, valve interstitial cell-smooth muscle cell like; VIC-CM like, valve interstitial cell-cardiomyocyte like; EC-CD34+, endothelial cell-CD34+; MC-MRC1+/CD86+, macrophage cell-mannose receptor C-type 1+/CD86+; MC like, macrophage cell like; CM, cardiomyocyte.
Differences in immune cells suggested the important role of the immune system in CAVD
We evaluated immune cells infiltration with GSE12644 and GSE51472 merged data matrix based on CIBERSORT algorithm. The proportion of immune cells from 15 calcified valve tissue samples and 15 normal valve tissue samples was illustrated in Figure 5A,5B. As shown in the boxplot of the immune cell infiltration difference, compared with the control sample, naïve B cells, resting dendritic cells and activated mast cells infiltrated more, whereas memory B cells, monocytes, activated dendritic cells resting mast cells and eosinophils infiltrated less (Figure 5C).
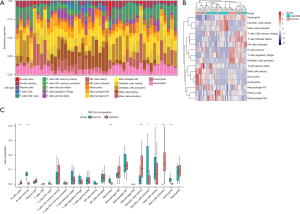
CTHRC1 associated with mast cells mostly
Correlation analysis showed that CTHRC1 was correlated 15 of the 22 types of immune cells (Table 2). In correlation analysis, activated mast cells (r=0.708, P<0.001; Figure 6A), resting dendritic cells (r=0.680, P<0.001; Figure 6B) and neutrophils (r=0.588, P<0.001; Figure 6C) were demonstrated to be positively correlated with CTHRC1 mostly, and eosinophils (r=−0.666, P<0.001; Figure 6D), resting mast cells (r=−0.617, P<0.001; Figure 6E) and monocytes (r=−0.594, P<0.001; Figure 6F) were negatively correlated with CTHRC1 mostly.
Table 2
Immune cells | Correlation | P value |
---|---|---|
B.cells.naive | 0.493 | 4.248e−04 |
T.cells.CD4.memory.resting | 0.322 | 2.728e−02 |
Dendritic.cells.resting | 0.68 | 1.497e−07 |
Mast.cells.activated | 0.708 | 2.61e−08 |
Neutrophils | 0.588 | 1.407e−05 |
B.cells.memory | −0.046 | 1.676e−03 |
T.cells.CD8 | −0.306 | 3.654e−02 |
T.cells.CD4.naive | −0.345 | 1.746e−02 |
T.cells.CD4.memory.activated | −0.301 | 3.957e−02 |
T.cells.regulatory.Tregs. | −0.342 | 1.865e−02 |
T.cells.gamma.delta | −0.308 | 3.521e−02 |
NK.cells.resting | −0.426 | 2.865e−03 |
Monocytes | −0.594 | 1.072e−05 |
Eosinophils | −0.666 | 3.313e−07 |
Mast.cells.resting | −0.617 | 3.87e−06 |
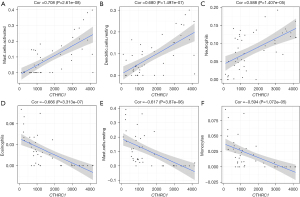
Discussion
CAVD is a common cardiovascular disease in developed countries and characterized by progressive fibrous calcification remodeling of valve leaflets (2,47). For advanced CAVD and CAVD accompanied by significant clinical symptoms, surgical and transcatheter aortic valve replacement remain the most effective treatment options. However, surgical aortic valve replacement was associated with a higher incidence of reoperation and bleeding (48). The complications of transcatheter aortic valve replacement included major vascular complications, stroke, coronary obstruction, intraventricular conduction abnormalities and paravalvular regurgitation (2,49). Therefore, it is important to understand the potential molecular mechanisms of CAVD to yield potential therapeutic targets to prevent or to reverse CAVD (2,50). Inflammatory response ignites the initiation phase, however, the molecular mechanisms mediated by immune cells are still not clear. We sought to identify potential target for CAVD based on GEO datasets and single-cell data and further explore the role of immune cell infiltration in CAVD.
In this study, we integrated three CAVD datasets from the GEO database and identified 205 DEGs. Enrichment analysis showed that these DEGs were mainly correlated with immune and inflammatory responses and extracellular matrix structural constituent formation process. In addition, the pathway enriched by GSEA mainly involves chemokine signaling pathway, cytokine receptor interaction and hematopoietic cell lineage. Inflammatory response, extracellular matrix transformation and cellular ossification have been proposed to be associated with the dynamic process of CAVD. Research have shown that combining multiple machine learning methods could improve predictive performance. We further identified CTHRC1 as the characteristic gene for CAVD by combining machine learning algorithms and DEGs in the public database. In another independent dataset, CTHRC1 got a high diagnostic effectiveness with an AUC of 0.917.
CTHRC1 is a secreted 28 kDa glycoprotein that is highly conserved from chordates to vertebrates (41). CTHRC1 expression has been correlated with conditions associated with deregulated wound and tissue repair, including arterial injury (41), liver and lung fibrosis and myocardial infarction (43,51-55), liver injury caused by Hepatitis B infection (56,57), suggesting the pro-fibrotic effect of CTHRC1. Additionally, several researches showed CTHRC1 was closely related to the osteogenesis. Takeshita et al. concluded that CTHRC1 might function as a guidance molecule for targeting stromal cells to promote the initiation of subsequent bone-forming activity (45). Mouse studies have clearly demonstrated that CTHRC1 played the essential regulatory role in bone homeostasis (44,45,58). Chen et al. demonstrated that extracellular vesicles obtained from human urine-derived stem cells alleviated bone loss in osteoporotic mice by transferring CTHRC1 and osteoprotegerin to enhance osteoblastic bone formation (59). Evidence from above studies concluded that CTHRC1 might be a key gene of CAVD.
We used the valve samples from our hospital for single-cell RNA sequencing to obtain insight into key gene expression at the single-cell level and verify the transcriptomic results. After quality control, the VICs was divided into seven clusters. We found that expression level of CTHRC1 was upregulated, especially in VIC-FB2 clusters. Decano et al. also identified a disease-driver population (DDP) within VICs, and temporal proteomic profiling of DDP-VICs identified potential targets for therapy, including Monoamine Oxidase A (MAOA) and CTHRC1. This strengthened the evidence for CTHRC1 as a potential therapeutic target. Moreover, our study included different types of diseased valves, including fibrotic valves, and performed the pseudotime analysis, which showed the changing tendency of the cells (60). Pseudotime analysis concluded that the cluster VIC-FB2 was abundantly distributed in the stage of calcification in CAVD, suggesting that CTHRC1 might have a potential role in the phenotype transformation of VICs. The function analysis showed that CTHRC1 was primarily involved in binding to a Wnt-protein, frizzled binding and growth hormone receptor binding. Increased Wnt pathway gene expression has been proved to be associated with CAVD in adults (61). VICs treated with the noncanonical Wnt ligands exhibited significant apoptosis and enhanced calcification (62). This is likely mediated through frizzled and lipoprotein receptor-related protein5/6 (LRP5/6) co-receptor activation in calcified aortic valve leaflets, which play overlapping roles in Wnt/β-catenin signaling (63). Transforming growth factor β1 (TGF-β1) is a member of the bone morphogenetic protein (BMP) superfamily and is recognized as a potent pro-osteogenic factor and demonstrated associated with increased VIC activation of diseased aortic valves (64-66). Plentiful evidence links CTHRC1 to the TGF-β pathway (43,45,51,53,67,68). TGF-β1 has been demonstrated to induce VIC myofibroblast differentiation in a matrix stiffness-dependent manner (69). In an ex vivo CAVD model, TGF-β1 together with mechanical strain enhanced the activation, remodeling, and calcification potential of cultured VICs (70,71). Evidence from above studies concluded that CTHRC1 had the potential to be the therapeutic target.
The initial stage of is CAVD pathological process characterized by lesion development which is similar to that of atherosclerosis, such as endothelial injury, lipid infiltration, and inflammatory response (72). Nordquist et al. showed that in the mouse model of heart valve endothelial cell injury, positive injury response was associated with an acute increase in TGF-β1 enriched within the endothelial compartment and upregulation of CTHRC1 within the interstitium. And further experiments proved that the paracrine TGF-β1-CTHRC1 signaling axis potentially between valve endothelial cells (VECs) and VICs, influenced this biological function (73). Therefore, whether VECs-derived CTHRC1 was involved in the progression of the propagation phase is worth investigating.
In addition, based on CIBERSORT, we found that CTHRC1 was significantly correlated with activated mast cells mostly. A review had summarized that mast cells were associated with severity of aortic stenosis, restriction in leaflet motion, and calcification development (14). The chymase released by mast cells could convert angiotensin I to angiotensin II, contributing to the promotion of CAVD (11,12,74). Undetermined are the specifics of mast cells interactions with VICs. Fibroblasts and myofibroblasts are responsible for the excessive deposition of extracellular matrix (ECM). A study has demonstrated that mechanical stretch activated mast cells reseeded in fibrotic matrix. The activated mast cells degranulation then induced TGF-β1 activation, which contributed to pulmonary fibrosis progression (75). Previous experiments showed that CTHRC1 involved in the TGF-β1 signaling pathway. The existence of CTHRC1-immunocyte-VIC regulatory pathway is the future research direction. In addition, Zhang et al. found the cardiac fibroblasts exposed to sustained inflammatory signaling exhibited an increased pro-fibrotic phenotypic response in response to TGF-β signaling between cardiac mast cells and resident cardiac fibroblasts. The treatment with type I TGF-β receptor (TβR I) antagonist significantly attenuated the mast cell-induced the increase of alpha-smooth muscle actin (α-SMA) staining in fibroblasts (76). Studies have also reported that CTHRC1 was derived from fibroblasts and could be induced by TGF-β to modulate fibrotic process (53,77,78). Moreover, CTHRC1 was reported to be enhanced in fibroblasts and chondrocytic cells in response to TGF-β family members (68) and Ni et al. demonstrated that treatment with recombinant TGF-β increased the CTHRC1 level in colorectal cancer (CRC) cells, resulting in epithelial-mesenchymal transition promotion, by activating the TGF-β signaling pathway (79). Therefore, we speculate that the VICs with over-expressed CTHRC1 exhibit an increased repertoire of pro-fibrotic phenotypic responses in response to TGF-β released by mast cells. The immune cell activation-CTHRC1-VICs activation-VICs myofibroblast transformation signaling pathway is also worth further investigation.
Our study has certain limitations. First, the study lacks experimental verification, but the datasets used for analysis can be mutually verified to increase the reliability of the results. Second, direct analysis of the CTHRC1-immunocyte-VIC pathway was lacking, but the emphasis of the study was on the new regulatory gene, CTHRC1. Future tests in cell biology are needed to verify the causal relationship between CTHRC1 and CAVD, as well as to verify the regulatory pathway involved in mast cells and VICs in CAVD.
Conclusions
In summary, we found that CTHRC1 is a biomarker associated with CAVD. Mast cells, B cells, dendritic cells and eosinophils are related to CAVD occurrence. CTHRC1 and immune cells may play an important role in CAVD. Further exploration for the specific molecular mechanism of CTHRC1 and immune cells is required.
Acknowledgments
We thank the GEO datasets for providing data support to this study.
Funding: This work was supported by the National Key R&D Program of China (No. 2020YFC2008100).
Footnote
Reporting Checklist: The authors have completed the TRIPOD reporting checklist. Available at https://jtd.amegroups.com/article/view/10.21037/jtd-23-72/rc
Data Sharing Statement: Available at https://jtd.amegroups.com/article/view/10.21037/jtd-23-72/dss
Peer Review File: Available at https://jtd.amegroups.com/article/view/10.21037/jtd-23-72/prf
Conflicts of Interest: All authors have completed the ICMJE uniform disclosure form (available at https://jtd.amegroups.com/article/view/10.21037/jtd-23-72/coif). The authors have no conflicts of interest to declare.
Ethical Statement:
Open Access Statement: This is an Open Access article distributed in accordance with the Creative Commons Attribution-NonCommercial-NoDerivs 4.0 International License (CC BY-NC-ND 4.0), which permits the non-commercial replication and distribution of the article with the strict proviso that no changes or edits are made and the original work is properly cited (including links to both the formal publication through the relevant DOI and the license). See: https://creativecommons.org/licenses/by-nc-nd/4.0/.
References
- Nasir K, Katz R, Takasu J, et al. Ethnic differences between extra-coronary measures on cardiac computed tomography: multi-ethnic study of atherosclerosis (MESA). Atherosclerosis 2008;198:104-14. [Crossref] [PubMed]
- Lindman BR, Clavel MA, Mathieu P, et al. Calcific aortic stenosis. Nat Rev Dis Primers 2016;2:16006. [Crossref] [PubMed]
- Strange G, Stewart S, Celermajer D, et al. Poor Long-Term Survival in Patients With Moderate Aortic Stenosis. J Am Coll Cardiol 2019;74:1851-63. [Crossref] [PubMed]
- Brecker S, Mealing S, Padhiar A, et al. Cost-utility of transcatheter aortic valve implantation for inoperable patients with severe aortic stenosis treated by medical management: a UK cost-utility analysis based on patient-level data from the ADVANCE study. Open Heart 2014;1:e000155. [Crossref] [PubMed]
- Freeman RV, Otto CM. Spectrum of calcific aortic valve disease: pathogenesis, disease progression, and treatment strategies. Circulation 2005;111:3316-26. [Crossref] [PubMed]
- Rajamannan NM, Bonow RO, Rahimtoola SH. Calcific aortic stenosis: an update. Nat Clin Pract Cardiovasc Med 2007;4:254-62. [Crossref] [PubMed]
- Jian B, Narula N, Li QY, et al. Progression of aortic valve stenosis: TGF-beta1 is present in calcified aortic valve cusps and promotes aortic valve interstitial cell calcification via apoptosis. Ann Thorac Surg 2003;75:457-65; discussion 465-6. [Crossref] [PubMed]
- Teo KK, Corsi DJ, Tam JW, et al. Lipid lowering on progression of mild to moderate aortic stenosis: meta-analysis of the randomized placebo-controlled clinical trials on 2344 patients. Can J Cardiol 2011;27:800-8. [Crossref] [PubMed]
- New SE, Aikawa E. Molecular imaging insights into early inflammatory stages of arterial and aortic valve calcification. Circ Res 2011;108:1381-91. [Crossref] [PubMed]
- Peeters FECM, Meex SJR, Dweck MR, et al. Calcific aortic valve stenosis: hard disease in the heart: A biomolecular approach towards diagnosis and treatment. Eur Heart J 2018;39:2618-24. [Crossref] [PubMed]
- Helske S, Lindstedt KA, Laine M, et al. Induction of local angiotensin II-producing systems in stenotic aortic valves. J Am Coll Cardiol 2004;44:1859-66. [Crossref] [PubMed]
- Fujisaka T, Hoshiga M, Hotchi J, et al. Angiotensin II promotes aortic valve thickening independent of elevated blood pressure in apolipoprotein-E deficient mice. Atherosclerosis 2013;226:82-7. [Crossref] [PubMed]
- Wypasek E, Natorska J, Grudzień G, et al. Mast cells in human stenotic aortic valves are associated with the severity of stenosis. Inflammation 2013;36:449-56. [Crossref] [PubMed]
- Bartoli-Leonard F, Zimmer J, Aikawa E. Innate and adaptive immunity: the understudied driving force of heart valve disease. Cardiovasc Res 2021;117:2506-24. [Crossref] [PubMed]
- Kopytek M, Kolasa-Trela R, Ząbczyk M, et al. NETosis is associated with the severity of aortic stenosis: Links with inflammation. Int J Cardiol 2019;286:121-6. [Crossref] [PubMed]
- Liu Y, Jiang P, An L, et al. The role of neutrophil elastase in aortic valve calcification. J Transl Med 2022;20:167. [Crossref] [PubMed]
- Syväranta S, Alanne-Kinnunen M, Oörni K, et al. Potential pathological roles for oxidized low-density lipoprotein and scavenger receptors SR-AI, CD36, and LOX-1 in aortic valve stenosis. Atherosclerosis 2014;235:398-407. [Crossref] [PubMed]
- Gonzalez Rodriguez A, Schroeder ME, Grim JC, et al. Tumor necrosis factor-α promotes and exacerbates calcification in heart valve myofibroblast populations. FASEB J 2021;35:e21382. [Crossref] [PubMed]
- Benton JA, Kern HB, Leinwand LA, et al. Statins block calcific nodule formation of valvular interstitial cells by inhibiting alpha-smooth muscle actin expression. Arterioscler Thromb Vasc Biol 2009;29:1950-7. [Crossref] [PubMed]
- Walker GA, Masters KS, Shah DN, et al. Valvular myofibroblast activation by transforming growth factor-beta: implications for pathological extracellular matrix remodeling in heart valve disease. Circ Res 2004;95:253-60. [Crossref] [PubMed]
- Dolivo DM, Larson SA, Dominko T. Fibroblast Growth Factor 2 as an Antifibrotic: Antagonism of Myofibroblast Differentiation and Suppression of Pro-Fibrotic Gene Expression. Cytokine Growth Factor Rev 2017;38:49-58. [Crossref] [PubMed]
- Merryman WD, Schoen FJ. Mechanisms of calcification in aortic valve disease: role of mechanokinetics and mechanodynamics. Curr Cardiol Rep 2013;15:355. [Crossref] [PubMed]
- Rutkovskiy A, Stensløkken KO, Vaage IJ. Osteoblast Differentiation at a Glance. Med Sci Monit Basic Res 2016;22:95-106. [Crossref] [PubMed]
- Grim JC, Aguado BA, Vogt BJ, et al. Secreted Factors From Proinflammatory Macrophages Promote an Osteoblast-Like Phenotype in Valvular Interstitial Cells. Arterioscler Thromb Vasc Biol 2020;40:e296-308. [Crossref] [PubMed]
- Newman AM, Liu CL, Green MR, et al. Robust enumeration of cell subsets from tissue expression profiles. Nat Methods 2015;12:453-7. [Crossref] [PubMed]
- Davis S, Meltzer PS. GEOquery: a bridge between the Gene Expression Omnibus (GEO) and BioConductor. Bioinformatics 2007;23:1846-7. [Crossref] [PubMed]
- Bossé Y, Miqdad A, Fournier D, et al. Refining molecular pathways leading to calcific aortic valve stenosis by studying gene expression profile of normal and calcified stenotic human aortic valves. Circ Cardiovasc Genet 2009;2:489-98. [Crossref] [PubMed]
- Ohukainen P, Syväranta S, Näpänkangas J, et al. MicroRNA-125b and chemokine CCL4 expression are associated with calcific aortic valve disease. Ann Med 2015;47:423-9. [Crossref] [PubMed]
- Guauque-Olarte S, Droit A, Tremblay-Marchand J, et al. RNA expression profile of calcified bicuspid, tricuspid, and normal human aortic valves by RNA sequencing. Physiol Genomics 2016;48:749-61. [Crossref] [PubMed]
- Barrett T, Wilhite SE, Ledoux P, et al. NCBI GEO: archive for functional genomics data sets--update. Nucleic Acids Res 2013;41:D991-5. [Crossref] [PubMed]
- Gautier L, Cope L, Bolstad BM, et al. affy--analysis of Affymetrix GeneChip data at the probe level. Bioinformatics 2004;20:307-15. [Crossref] [PubMed]
- Parker HS, Leek JT, Favorov AV, et al. Preserving biological heterogeneity with a permuted surrogate variable analysis for genomics batch correction. Bioinformatics 2014;30:2757-63. [Crossref] [PubMed]
- Ritchie ME, Phipson B, Wu D, et al. limma powers differential expression analyses for RNA-sequencing and microarray studies. Nucleic Acids Res 2015;43:e47. [Crossref] [PubMed]
- Yu G, Wang LG, Han Y, et al. clusterProfiler: an R package for comparing biological themes among gene clusters. OMICS 2012;16:284-7. [Crossref] [PubMed]
- Powers RK, Goodspeed A, Pielke-Lombardo H, et al. GSEA-InContext: identifying novel and common patterns in expression experiments. Bioinformatics 2018;34:i555-64. [Crossref] [PubMed]
- Tibshirani R. Regression Shrinkage and Selection Via the Lasso. J R Stat Soc 1996;58:267-88.
- Lin X, Yang F, Zhou L, et al. A support vector machine-recursive feature elimination feature selection method based on artificial contrast variables and mutual information. J Chromatogr B Analyt Technol Biomed Life Sci 2012;910:149-55. [Crossref] [PubMed]
- Alakwaa FM, Chaudhary K, Garmire LX. Deep Learning Accurately Predicts Estrogen Receptor Status in Breast Cancer Metabolomics Data. J Proteome Res 2018;17:337-47. [Crossref] [PubMed]
- Friedman J, Hastie T, Tibshirani R. Regularization Paths for Generalized Linear Models via Coordinate Descent. J Stat Softw 2010;33:1-22. [Crossref] [PubMed]
- Huang ML, Hung YH, Lee WM, et al. SVM-RFE based feature selection and Taguchi parameters optimization for multiclass SVM classifier. ScientificWorldJournal 2014;2014:795624. [Crossref] [PubMed]
- Pyagay P, Heroult M, Wang Q, et al. Collagen triple helix repeat containing 1, a novel secreted protein in injured and diseased arteries, inhibits collagen expression and promotes cell migration. Circ Res 2005;96:261-8. [Crossref] [PubMed]
- Chen YL, Wang TH, Hsu HC, et al. Overexpression of CTHRC1 in hepatocellular carcinoma promotes tumor invasion and predicts poor prognosis. PLoS One 2013;8:e70324. [Crossref] [PubMed]
- Li J, Wang Y, Ma M, et al. Autocrine CTHRC1 activates hepatic stellate cells and promotes liver fibrosis by activating TGF-beta signaling. EBioMedicine 2019;40:43-55. [Crossref] [PubMed]
- Kimura H, Kwan KM, Zhang Z, et al. Cthrc1 is a positive regulator of osteoblastic bone formation. PLoS One 2008;3:e3174. [Crossref] [PubMed]
- Takeshita S, Fumoto T, Matsuoka K, et al. Osteoclast-secreted CTHRC1 in the coupling of bone resorption to formation. J Clin Invest 2013;123:3914-24. [Crossref] [PubMed]
- Wang P, Wang YC, Chen XY, et al. CTHRC1 is upregulated by promoter demethylation and transforming growth factor-β1 and may be associated with metastasis in human gastric cancer. Cancer Sci 2012;103:1327-33. [Crossref] [PubMed]
- Mathieu P, Arsenault BJ. CAVD: civilization aortic valve disease. Eur Heart J 2017;38:2198-200. [Crossref] [PubMed]
- Chiang YP, Chikwe J, Moskowitz AJ, et al. Survival and long-term outcomes following bioprosthetic vs mechanical aortic valve replacement in patients aged 50 to 69 years. JAMA 2014;312:1323-9. [Crossref] [PubMed]
- Rodés-Cabau J. Transcatheter aortic valve implantation: current and future approaches. Nat Rev Cardiol 2011;9:15-29. [Crossref] [PubMed]
- Gould ST, Srigunapalan S, Simmons CA, et al. Hemodynamic and cellular response feedback in calcific aortic valve disease. Circ Res 2013;113:186-97. [Crossref] [PubMed]
- Jin J, Togo S, Kadoya K, et al. Pirfenidone attenuates lung fibrotic fibroblast responses to transforming growth factor-β1. Respir Res 2019;20:119. [Crossref] [PubMed]
- Bauer Y, Tedrow J, de Bernard S, et al. A novel genomic signature with translational significance for human idiopathic pulmonary fibrosis. Am J Respir Cell Mol Biol 2015;52:217-31. [Crossref] [PubMed]
- Bian Z, Miao Q, Zhong W, et al. Treatment of cholestatic fibrosis by altering gene expression of Cthrc1: Implications for autoimmune and non-autoimmune liver disease. J Autoimmun 2015;63:76-87. [Crossref] [PubMed]
- Tsukui T, Sun KH, Wetter JB, et al. Collagen-producing lung cell atlas identifies multiple subsets with distinct localization and relevance to fibrosis. Nat Commun 2020;11:1920. [Crossref] [PubMed]
- Ruiz-Villalba A, Romero JP, Hernández SC, et al. Single-Cell RNA Sequencing Analysis Reveals a Crucial Role for CTHRC1 (Collagen Triple Helix Repeat Containing 1) Cardiac Fibroblasts After Myocardial Infarction. Circulation 2020;142:1831-47. [Crossref] [PubMed]
- Bai L, Zhang W, Tan L, et al. Hepatitis B virus hijacks CTHRC1 to evade host immunity and maintain replication. J Mol Cell Biol 2015;7:543-56. [Crossref] [PubMed]
- Zhang R, Cao Y, Bai L, et al. The collagen triple helix repeat containing 1 facilitates hepatitis B virus-associated hepatocellular carcinoma progression by regulating multiple cellular factors and signal cascades. Mol Carcinog 2015;54:1554-66. [Crossref] [PubMed]
- Jin YR, Stohn JP, Wang Q, et al. Inhibition of osteoclast differentiation and collagen antibody-induced arthritis by CTHRC1. Bone 2017;97:153-67. [Crossref] [PubMed]
- Chen CY, Rao SS, Tan YJ, et al. Extracellular vesicles from human urine-derived stem cells prevent osteoporosis by transferring CTHRC1 and OPG. Bone Res 2019;7:18. [Crossref] [PubMed]
- Decano JL, Iwamoto Y, Goto S, et al. A disease-driver population within interstitial cells of human calcific aortic valves identified via single-cell and proteomic profiling. Cell Rep 2022;39:110685. [Crossref] [PubMed]
- Caira FC, Stock SR, Gleason TG, et al. Human degenerative valve disease is associated with up-regulation of low-density lipoprotein receptor-related protein 5 receptor-mediated bone formation. J Am Coll Cardiol 2006;47:1707-12. [Crossref] [PubMed]
- Albanese I, Yu B, Al-Kindi H, et al. Role of Noncanonical Wnt Signaling Pathway in Human Aortic Valve Calcification. Arterioscler Thromb Vasc Biol 2017;37:543-52. [Crossref] [PubMed]
- Siddique A, Yu B, Khan K, et al. Expression of the Frizzled receptors and their co-receptors in calcified human aortic valves. Can J Physiol Pharmacol 2018;96:208-14. [Crossref] [PubMed]
- Yetkin E, Waltenberger J. Molecular and cellular mechanisms of aortic stenosis. Int J Cardiol 2009;135:4-13. [Crossref] [PubMed]
- Watson KE, Boström K, Ravindranath R, et al. TGF-beta 1 and 25-hydroxycholesterol stimulate osteoblast-like vascular cells to calcify. J Clin Invest 1994;93:2106-13. [Crossref] [PubMed]
- Clark-Greuel JN, Connolly JM, Sorichillo E, et al. Transforming growth factor-beta1 mechanisms in aortic valve calcification: increased alkaline phosphatase and related events. Ann Thorac Surg 2007;83:946-53. [Crossref] [PubMed]
- Binks AP, Beyer M, Miller R, et al. Cthrc1 lowers pulmonary collagen associated with bleomycin-induced fibrosis and protects lung function. Physiol Rep 2017;5:e13115. [Crossref] [PubMed]
- Durmus T, LeClair RJ, Park KS, et al. Expression analysis of the novel gene collagen triple helix repeat containing-1 (Cthrc1). Gene Expr Patterns 2006;6:935-40. [Crossref] [PubMed]
- Pho M, Lee W, Watt DR, et al. Cofilin is a marker of myofibroblast differentiation in cells from porcine aortic cardiac valves. Am J Physiol Heart Circ Physiol 2008;294:H1767-78. [Crossref] [PubMed]
- Fisher CI, Chen J, Merryman WD. Calcific nodule morphogenesis by heart valve interstitial cells is strain dependent. Biomech Model Mechanobiol 2013;12:5-17. [Crossref] [PubMed]
- Merryman WD, Lukoff HD, Long RA, et al. Synergistic effects of cyclic tension and transforming growth factor-beta1 on the aortic valve myofibroblast. Cardiovasc Pathol 2007;16:268-76. [Crossref] [PubMed]
- Liu X, Zheng Q, Wang K, et al. Sam68 promotes osteogenic differentiation of aortic valvular interstitial cells by TNF-α/STAT3/autophagy axis. J Cell Commun Signal 2023; Epub ahead of print. [Crossref] [PubMed]
- Nordquist EM, Dutta P, Kodigepalli KM, et al. Tgfβ1-Cthrc1 Signaling Plays an Important Role in the Short-Term Reparative Response to Heart Valve Endothelial Injury. Arterioscler Thromb Vasc Biol 2021;41:2923-42. [Crossref] [PubMed]
- Helske S, Syväranta S, Kupari M, et al. Possible role for mast cell-derived cathepsin G in the adverse remodelling of stenotic aortic valves. Eur Heart J 2006;27:1495-504. [Crossref] [PubMed]
- Shimbori C, Upagupta C, Bellaye PS, et al. Mechanical stress-induced mast cell degranulation activates TGF-β1 signalling pathway in pulmonary fibrosis. Thorax 2019;74:455-65. [Crossref] [PubMed]
- Zhang W, Chancey AL, Tzeng HP, et al. The development of myocardial fibrosis in transgenic mice with targeted overexpression of tumor necrosis factor requires mast cell-fibroblast interactions. Circulation 2011;124:2106-16. [Crossref] [PubMed]
- Li J, Cao J, Li M, et al. Collagen triple helix repeat containing-1 inhibits transforming growth factor-b1-induced collagen type I expression in keloid. Br J Dermatol 2011;164:1030-6. [Crossref] [PubMed]
- LeClair RJ, Durmus T, Wang Q, et al. Cthrc1 is a novel inhibitor of transforming growth factor-beta signaling and neointimal lesion formation. Circ Res 2007;100:826-33. [Crossref] [PubMed]
- Ni S, Ren F, Xu M, et al. CTHRC1 overexpression predicts poor survival and enhances epithelial-mesenchymal transition in colorectal cancer. Cancer Med 2018;7:5643-54. [Crossref] [PubMed]