Bioinformatics analysis and single-cell RNA sequencing: elucidating the ubiquitination pathways and key enzymes in lung adenocarcinoma
Highlight box
Key findings
• Through the integration of single-cell sequencing and conventional transcriptomic analyses, we pinpointed PSMD14, a critical deubiquitination enzyme, as a promising therapeutic target for lung adenocarcinoma.
What is known and what is new?
• An understanding of the mechanisms of ubiquitination modifications can reveal critical therapeutic targets for LUAD.
• Using single-cell sequencing, we discovered that PSMD14 stabilized the AGR2 protein, promoting LUAD progression and highlighting a novel therapeutic target.
What is the implication, and what should change now?
• Single-cell sequencing allowed for more accurate detection of ubiquitination variations, and combined with experimental validation and bioinformatics analysis, provided valuable insight for LUAD treatment.
Introduction
Lung cancer constitutes a predominant malignancy, exerting a considerable influence on worldwide health. As per recent statistics, the leading cancer types diagnosed in China in 2022 encompassed lung cancer, colorectal cancer, stomach cancer, liver cancer, and breast cancer. Notably, lung cancer was the leading cause of cancer-related deaths (1). In the United States, despite the rising incidence of breast cancer, lung cancer remains the primary cause of cancer death (2). The two primary histological subtypes are non-small cell lung cancer (NSCLC) and small cell lung cancer (SCLC). NSCLC accounts for about 80–85% of all cases and includes adenocarcinoma, squamous cell carcinoma, and large cell carcinoma (3). The incidence of lung adenocarcinoma (LUAD) has been on the rise in recent years, particularly in nonsmoking patients (4,5). Traditional treatments encompass various modalities, including surgery, radiotherapy, and chemotherapy, and they have demonstrated survival benefits for patients (6-8). However, these treatments can also elicit severe side effects, negatively impacting the quality of life of affected individuals (9). The introduction of innovative technologies and approaches such as liquid biopsy, image-guided radiotherapy, immune checkpoint inhibitors, and chimeric antigen receptor (CAR) T-cell therapy has expanded the therapeutic arsenal for LUAD, providing clinicians with more comprehensive decision-making tools (10-13). Nevertheless, drug resistance, immunosuppression, and lack of specificity have led to poor 5-year survival rates, particularly among patients with advanced LUAD.
Single-cell sequencing is a powerful analytical method that enables the exploration of individual cell-level omics information. Unlike conventional sequencing methods, single-cell sequencing facilitates the assessment of cellular characteristics in a sample and can examine the molecular alterations taking place in a single cell, including changes in gene expression, epigenetic modifications, and protein levels (14,15). The application of single-cell sequencing has transformed the investigation of intricate tissues, particularly in the fields of neuroscience and oncology (16-18). Regarding LUAD, single-cell sequencing can reveal subclonal populations of cancer cells by detecting unique cellular phenotypes and their respective proportions within the tumor. Such findings can provide valuable insights into treatment selection (19). Furthermore, single-cell sequencing can track dynamic changes in tumor cells over time and monitor the emergence of therapeutic resistance.
Ubiquitination modification is a vital posttranslational modification process that regulates intracellular protein levels by adding ubiquitin molecules, which target substrate proteins for degradation by the 26S proteasome. This modification is involved in various cellular processes, including cell proliferation, apoptosis, invasion, migration, and DNA damage repair, which play crucial roles in tumorigenesis and cancer progression (20-23). Thus, defects in this process can promote cancer development and progression, rendering ubiquitination an attractive target for therapeutic intervention (24,25). In the realm of ubiquitination modification, single-cell sequencing serves as a valuable investigative tool for examining the relationship between alterations in ubiquitination patterns and cell proliferation, apoptosis, and differentiation. Additionally, this technique enables the identification of cell subpopulations that exhibit distinct ubiquitination patterns, thereby facilitating a more comprehensive understanding of the regulatory effects of ubiquitination modifications on cellular processes and their contributions to the development and progression of tumors.
In this study, we integrated genomic and transcriptomic data from single-cell and common transcriptome datasets in the Gene Expression Omnibus (GEO) database as well as The Cancer Genome Atlas (TCGA)-LUAD dataset to comprehensively explore the ubiquitin modification landscape of LUAD from a single-cell multiomics perspective. The identification and validation of key molecules associated with ubiquitination modification in LUAD was conducted through a series of experiments, with the ultimate goal of discovering novel therapeutic targets for LUAD. We present this article in accordance with the TRIPOD reporting checklist (available at https://jtd.amegroups.com/article/view/10.21037/jtd-23-795/rc).
Methods
Acquisition and preprocessing of data from public databases
This study utilized datasets obtained from the GEO database, consisting of 2 types of transcriptome data: bulk and single-cell RNA sequencing (scRNA-seq). The scRNA-seq data included GSE117570 (26), GSE131907 (27), GSE149655 (28), and GSE123902 (29), with details summarized in Table 1. Samples in the single-cell dataset underwent screening to retain only primary LUAD tissues and exclude metastatic tumors. The bulk transcriptome data comprised GSE3141 (30), GSE11969 (31), GSE30219 (32), GSE31210 (33), GSE50081 (34), GSE32863 (35), and GSE118370 (36), all sourced from Homo sapiens (Table 2). Samples in the bulk transcriptome dataset underwent screening to maintain consistency of subsequent data analyses by retaining only normal lung tissues and LUAD tissues, while excluding other pathological subtypes. Clinical characteristics of patients, including sample phenotype and prognostic information, were also collected.
Table 2
Dataset | Data type | Platform | Samples | Ref. |
---|---|---|---|---|
GSE3141 | Expression profiling by array | GPL570 | 111 | (30) |
GSE11969 | Expression profiling by array | GPL7015 | 163 | (31) |
GSE30219 | Expression profiling by array | GPL570 | 307 | (32) |
GSE31210 | Expression profiling by array | GPL570 | 246 | (33) |
GSE50081 | Expression profiling by array | GPL570 | 181 | (34) |
GSE32863 | Expression profiling by array | GPL6884 | 116 | (35) |
GSE118370 | Expression profiling by array | GPL570 | 12 | (36) |
GEO, Gene Expression Omnibus.
In addition, we analyzed the TCGA-LUAD dataset available on the UCSC Xena platform (https://xenabrowser.net/datapages/) to investigate various aspects of LUAD, including transcriptomics, somatic mutations, copy number variations (CNVs), and clinical characteristics. The dataset consists of 585 samples with count and fragments per kilobase of transcript per million mapped reads (FPKM) values, which were converted to transcript per million (TPM) format for analysis. For somatic mutation analysis, we selected 561 samples with VarScan2 variant aggregation and masking data and used the maftools R package (37) for visualization. CNV analysis was performed on 532 samples with masked copy number segment data. Phenotypic information was obtained for 877 samples, and survival data were available for 738 samples, which were matched with the transcriptome data. Samples lacking phenotypic information or survival data were excluded from the analysis.
The integrated annotations for Ubiquitin and Ubiquitin-like Conjugation Database (iUUCD) (38) is a comprehensive repository that provides detailed information on various enzymes and domains involved in ubiquitin modification. It comprises 27 ubiquitin activating enzymes (E1s), 109 ubiquitin binding enzymes (E2s), 1,153 ubiquitin ligases (E3s), 164 deubiquitination enzymes (DUBs), 396 ubiquitin binding proteins (UBDs), and 183 ubiquitin-like domains (ULDs). To identify molecular genes associated with ubiquitination modification, a list of relevant genes was extracted from iUUCD.
Processing of single-cell datasets
The 4 scRNA-seq datasets (GSE117570, GSE131907, GSE149655, and GSE123902) were processed using the Seurat package in R (version 4.0.5) (39). Cells were filtered based on several quality control criteria, including the retention of features between 200 and 5,000, unique molecular identifier (UMI) counts between 400 and 100,000, log10GenesPerUMI value above 0.7, and a mitochondrial UMI proportion less than 20%. These measures ensured the exclusion of low-quality cells and resulted in a reliable and robust dataset for further downstream analysis.
After normalizing the sequencing depth using the “SCTransform” function and applying the “glmGamPoi” method for normalization, we identified the 3000 most variable features using the “vst” method and detected significant principal components using principal component analysis (PCA) (40). The “FindVariableFeatures” function was employed to call the “vst” method, and the data were scaled using the “ScaleData” function to exclude sequencing depth effects. For each of the 4 datasets (GSE117570, GSE131907, GSE149655, and GSE123902), we selected 10, 10, 15, and 10 significant principal components, respectively, for t-distributed stochastic neighbor embedding (tSNE) analysis. We used the Louvain algorithm to optimize the clusters and divided the cells into different clusters using the “FindClusters” function with a resolution of 0.5 for all 4 datasets. Finally, dimensionality reduction was performed using the “RunTSNE” function to enable visualization and exploration of the dataset. We annotated different cell groups according to literature published by Wu et al. (41), and the list of annotations is provided in Table S1.
We then used scRNA-seq data processing with InferCNV (https://github.com/broadinstitute/inferCNV) to determine the composition of malignant cells in epithelial cells. InferCNV analysis was performed on the GSE117570, GSE131907, GSE149655, and GSE123902 datasets, with immune cells serving as the reference. Copy number status information of each genomic region was used to identify malignant cells in the samples. To investigate the modification characteristics of E1, E2, E3, and DUBs in epithelial cells, we utilized ubiquitination modification-related genes from iUUCD and analyzed them using AUCell (42). This allowed us to identify the ubiquitination modification states of different cells and key molecules involved in this process.
Survival analysis
A set of 1,296 molecules related to the process of ubiquitination modification were obtained from iUUCD, and a bulk prognostic analysis was performed on the GSE3141, GSE11969, GSE30219, GSE31210, GSE50081, and TCGA-LUAD datasets. The median expression level was utilized to perform prognostic analysis, and patients were classified into high- and low-expression groups based on dichotomous variables. Kaplan-Meier method was applied to carry out survival analysis, and statistical significance was evaluated by log-rank test.
Multivariate Cox regression analysis and construction of clinical prediction model
Clinical data of patients with LUAD were obtained from the TCGA-LUAD dataset, including age, sex, race, and tumor stage. Univariate Cox analysis was performed by combining these clinical variables with the expression level of PSMD14 (log2 normalized). Variables with a P value less than 0.05 in the univariate Cox analysis were selected for multivariate Cox analysis. The variables with a P value less than 0.05 in the multivariate Cox analysis were considered to be independent prognostic factors.
A clinical prediction model was constructed using the rms R package (43), incorporating clinical characteristics and PSMD14 expression values. A nomogram was then created to visualize the model, and calibration curves were used to assess its accuracy. Resampling was performed using the bootstrap method, with 1,000 iterations and a sample size of 50. The model was designed to predict survival at 1, 3, and 5 years.
Acquisition of LUAD tissues
Clinical tissue specimens were obtained from the Department of Thoracic Surgery, The Second Affiliated Hospital of Harbin Medical University. Surgically resected lung lobes from patients were collected from March 2021 to July 2021. A total of 44 fresh tissue specimens were included, comprising 22 LUAD tissues and 22 paracancer tissues (located within 2 cm of the tumor). After isolation, the specimens were rinsed with normal saline and promptly stored in freezer storage tubes in a liquid nitrogen tank. Experienced pathologists confirmed all specimens. The study was conducted in accordance with the Declaration of Helsinki (as revised in 2013). Patient specimens were obtained with the approval of the Ethics Committee of The Second Affiliated Hospital of Harbin Medical University (Ethics No. 2021-166) and with the consent of the patients.
Cultivation, transfection, and lentiviral infection of cells
A549, H1299, and H358 cell lines were procured from the Cell Bank of the Chinese Academy of Sciences (Shanghai, China). HEK-293T cells were obtained from the American Type Culture Collection (Manassas, VA, USA). The cells were cultured in Dulbecco’s Modified Eagle Medium (DMEM) with high glucose, which was supplemented with 10% fetal bovine serum (FBS) obtained from Biological Industries (Beit Haemek, Israel). The cells were maintained at 37 °C in a 5% CO2 environment.
Lentivirus and negative control vectors were procured from GeneChem (Shanghai, China). A549 and H1299 cells were infected for 48 hours with a multiplicity of infection (MOI) of 10 and 30, respectively. Plasmids expressing HA-PSMD14, Myc-AGR2, or His-Ub were procured from GeneChem. Small interfering RNAs (siRNAs) targeting AGR2 were purchased from GenePharma (Suzhou, China). The sequences of shPSMD14 and siAGR2 can be found in Table S2. Transfection was carried out with Lipofectamine 2000 (Invitrogen, Carlsbad, CA, USA) according to the manufacturer’s instructions.
Cell Counting Kit-8 (CCK-8) assay
Cell viability was evaluated using the CCK-8 kit (C0038, Beyotime, Shanghai, China). Cell suspensions were seeded at a density of 3×103 cells per well in 96-well plates. Subsequently, a 10 µL solution of CCK-8 was added to each well every 24 hours. Following a 2-hour incubation period, the absorbance was measured at 450 nm using an enzymatic assay.
Colony formation assays
To perform colony formation assays, cells were seeded in 6-well plates containing 2 mL of DMEM. The medium was replenished every 3 days until visible cell colonies formed. The cells were then fixed using 4% paraformaldehyde and stained with 0.5% crystal violet. The number of colonies was manually counted.
Wound healing assay
A cell suspension was seeded in a 6-well plate and allowed to adhere for 24 hours. The adherent cells were then subjected to a scratch assay by creating a straight scratch line using a 200-µL pipette tip. The scratch area was washed with phosphate-buffered saline (PBS), and the cells were further cultured in serum-free medium for 24 hours. The width of the scratch was measured at 0 and 24 hours under a microscope.
Transwell assay
A cell suspension of 5×104 cells was mixed with 100 µL of serum-free medium and seeded onto the upper chamber of an 8-µm pore size polycarbonate membrane, either precoated with a substrate or left uncoated (Corning Incorporated, New York, NY, USA), as per the manufacturer’s instructions. The lower chamber was filled with 600 µL of conventional medium. After 24 hours of incubation, the migrated or invaded cells were stained with crystal violet. Three random fields of view were photographed, and the cells were manually counted.
Reverse-transcription quantitative polymerase chain reaction (RT-qPCR) assay
Total RNA was extracted from the cells using the RNAiso Plus reagent (Takara Bio, Kusatsu, Japan), and the RNA concentration and purity were evaluated using the NanoDrop 2000 system (Thermo Fisher, Carlsbad, CA, USA). The extracted RNA was reverse transcribed into complementary DNA (cDNA) using the PrimeScript™ RT reagent Kit with gDNA Eraser (Perfect Real Time) (Takara Bio). qPCR was performed using SYBR® Premix Ex Taq™ II (Tli RNaseH Plus) (Takara Bio) on an ABI 7500 Fast system (Life Technologies, Carlsbad, CA, USA). The primer sequences used for the qPCR analysis are listed in Table S2. The relative gene expression was determined using the 2−ΔΔCt method, with glyceraldehyde 3-phosphate dehydrogenase (GAPDH) serving as the internal control.
Western blot assay
Total cellular proteins were extracted using radioimmunoprecipitation assay (RIPA) lysis buffer (Beyotime). The proteins were separated by sodium dodecyl sulphate-polyacrylamide gel electrophoresis (SDS-PAGE) on either 10% or 15% polyacrylamide gels and subsequently transferred onto polyvinylidene difluoride (PVDF) membranes. The membranes were then blocked for 2 hours at room temperature and incubated with primary antibodies. After washing, the membranes were incubated with the appropriate secondary antibodies. The protein bands were visualized using an enhanced chemiluminescence system. Antibodies used in this study included: normal rabbit immunoglobulin G (IgG) [2729], anti-His [2365], and anti-Ubiquitin [3936] from Cell Signaling Technology (Danvers, MA, USA); anti-PSMD14 (ab109130), anti-AGR2 (ab76473), and anti-β-Actin antibodies (ab8227) from Abcam (Cambridge, MA, USA); and anti-HA (H6908) and anti-Myc (06-549) from Sigma-Aldrich (St Louis, MO, USA).
Animal experiment
We established a xenograft mouse model by subcutaneously injecting 5×106 cells in 200 µL of PBS into the left axillary region of 5-week-old female BALB/c nude mice (Beijing Vital River Laboratory Animal Technology Co., Ltd., China). The mice were housed in a specific pathogen-free (SPF) animal facility with ad libitum access to food and water. We monitored the tumor growth every 3 days using a Vernier caliper and calculated the tumor volumes using the formula V = 1/2(length × width2). The mice were euthanized 15 days after injection. Animal experiments were performed under a project license (No. 2021-167) granted by The Animal Care and Use Committee of Harbin Medical University, in compliance with institutional guidelines for the care and use of animals.
Co-immunoprecipitation (Co-IP)
We transfected 6×105 HEK-293T cells per well in 6-well plates with indicated plasmids. After 48 hours, the cells were treated with 10 µM MG132 for 6 hours. We extracted total proteins in RIPA lysis buffer and incubated the cell lysates with anti-HA M2 affinity gel at 4 °C overnight. We washed the beads 3 times with RIPA buffer and collected the immunoprecipitates for immunoblotting. For endogenous immunoprecipitation, we incubated the lysates overnight with 2 µg anti-AGR2 antibodies or IgG and then added 30 µL 50% protein A agarose bead for another 6 hours of incubation. We washed the beads 3 times with cold 1× PBS and analyzed the immunoprecipitated proteins by immunoblotting.
Statistical analyses
GraphPad Prism 9.3.0 was used for statistical analyses. Data are expressed as mean ± standard deviation from at least 3 independent experiments. We performed chi-squared tests and t-test to analyze the count data and the measurement data, respectively. We used the Kaplan-Meier method and the log-rank test for survival analysis. We set the significance level at P<0.05 (*P<0.05, **P<0.01, ***P<0.001).
Results
Aberrant ubiquitination modification landscape of LUAD microenvironment revealed by single-cell transcriptome sequencing
We processed and normalized 4 single-cell datasets (GSE117570, GSE131907, GSE123902, and GSE149655) containing LUAD tissues (Figure S1). After dimensionality reduction and clustering, we obtained 10, 16, 16, and 18 cell subpopulations in the 4 datasets respectively (Figure 1A-1D). Based on the cell marker genes, we annotated epithelial cells, endothelial cells, myeloid cells, T cells, and B cells. To highlight the composition features of the tumor microenvironment in LUAD more intuitively, we classified all cell populations into three categories: epithelial cells, immune cells, and stromal cells (Figure 1E-1H). The four datasets included different numbers and proportions of these cell types across different samples (Figure 1I), which further highlighted the importance of multiple datasets corroborating each other.
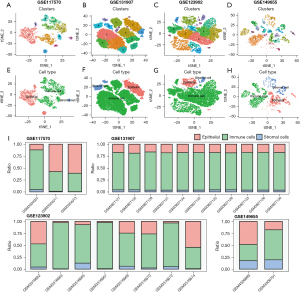
Next, InferCNV was used to detect CNVs in epithelial cells from the 4 single-cell datasets (GSE117570, GSE123902, GSE131907, and GSE149655). Some immune cells were selected as references (“Spike-imm”). The heatmap in Figure S2 shows the CNVs of immune, stromal, and epithelial cells. We found that malignant epithelial cells accounted for 66.67–85.49% of total epithelial cells in each dataset (Figure 2A). CNV scores were then used to compare the differences between normal epithelial cells and malignant cells, revealing that in all 4 datasets, malignant cell CNVs were higher than normal epithelial cell CNVs, and that the differences were statistically significant (Figure 2B).
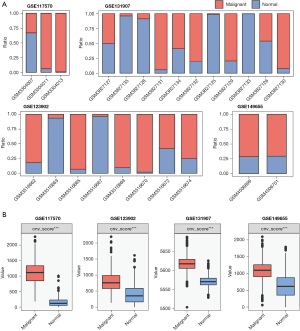
We then applied AUCell analysis to the four single-cell datasets (GSE117570, GSE123902, GSE131907, and GSE149655) to assess the ubiquitination level in LUAD cells. We found that E1 had lower AUC scores than E2, E3, and DUBs, suggesting a minor role of E1 in epithelial cells (Figure 3A-3D). We then compared the AUC scores of E1, E2, E3, and DUBs between normal and malignant cells using InferCNV analysis. We observed that E2, E3, and DUBs had higher AUC scores in malignant cells than in normal cells in most datasets, indicating their involvement in tumorigenesis and progression (Figure 3E-3H). However, E1 showed no consistent difference between normal and malignant cells.
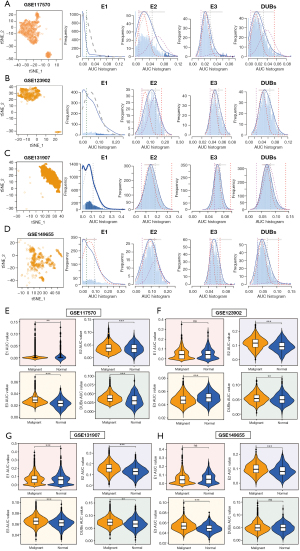
Identification of ubiquitination modification molecules associated with prognosis
To further identify key ubiquitination-related molecules, we performed prognostic analysis using bulk transcriptome data from six datasets: TCGA-LUAD, GSE3141, GSE31210, GSE11969, GSE30219, and GSE50081. We plotted Kaplan-Meier curves for each dataset and selected molecules with P<0.05 as prognostic candidates. We then screened for molecules that had prognostic value in at least 3 datasets and found 53 ubiquitination-related molecules (Figure 4A,4B).
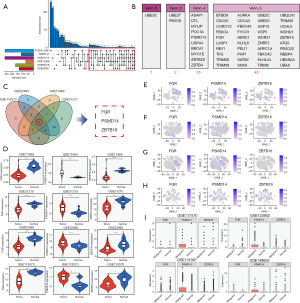
The expression level of the 53 molecules related to LUAD prognosis were then analyzed in four datasets (GSE118370, GSE32863, GSE11969 and GSE31210) containing both normal and tumor tissues. Among the molecules, FGR, PSMD14, and ZBTB16 genes were consistently differentially expressed across the datasets (Figure 4C,4D). We further examined their expression in single-cell data and found that PSMD14 was highly expressed in malignant epithelial cells compared to normal cells, while FGR and ZBTB16 showed no significant difference (Figure 4E-4I). Given these results, we focused on the PSMD14 gene and conducted further analysis and validation.
Mutation analysis of PSMD14 in TCGA-LUAD
We analyzed the genomic changes of PSMD14 in the TCGA-LUAD dataset and found that it had few single nucleotide mutations and a high copy number amplification ratio (Figure S3A,S3B). Moreover, there was a significant positive correlation between PSMD14 copy number and mRNA expression (Figure S3C). This suggested that PSMD14 expression was increased by copy number amplification in LUAD, which may have enabled its deubiquitination function and contributed to tumor progression.
PSMD14 as a novel biomarker for survival prediction
To further clarify the potential clinical value of PSMD14, we performed transcriptome and prognostic analysis using the TCGA-LUAD dataset. We found that PSMD14 expression was significantly higher in tumor tissues than in normal tissues in TCGA-LUAD, consistent with our previous findings (Figure 5A). Moreover, high PSMD14 expression was associated with poor overall survival (OS), progression-free survival (PFS), and disease-specific survival (DSS) (Figure 5B-5D). In addition, we conducted univariate and multivariate Cox regression analyses using clinical characteristics such as age, gender, pathological stage, race, and PSMD14 expression. The results showed that PSMD14 was an independent prognostic factor for LUAD patients (Table 3).
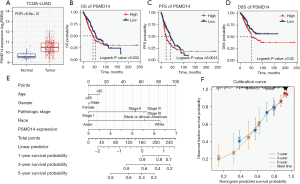
Table 3
Characteristics | Total (N) | Univariate analysis | Multivariate analysis | |||
---|---|---|---|---|---|---|
Hazard ratio (95% CI) | P value | Hazard ratio (95% CI) | P value | |||
Age (years) | 516 | – | – | |||
≤65 | 255 | Reference | ||||
>65 | 261 | 1.223 (0.916–1.635) | 0.172 | – | – | |
Gender | 526 | – | – | |||
Female | 280 | Reference | ||||
Male | 246 | 1.070 (0.803–1.426) | 0.642 | – | – | |
Race | 468 | |||||
Black or African American | 55 | Reference | ||||
Asian | 7 | 0.710 (0.094–5.345) | 0.740 | – | – | |
White | 406 | 1.442 (0.870–2.388) | 0.155 | – | – | |
Pathologic stage | 518 | |||||
Stage I | 290 | Reference | ||||
Stage II | 121 | 2.418 (1.691–3.457) | <0.001* | 2.318 (1.617–3.321) | <0.001* | |
Stage III | 81 | 3.544 (2.437–5.154) | <0.001* | 3.433 (2.358–5.000) | <0.001* | |
Stage IV | 26 | 3.790 (2.193–6.548) | <0.001* | 3.471 (1.996–6.034) | <0.001* | |
PSMD14 expression | 526 | 1.427 (1.123–1.812) | 0.004* | 1.296 (1.012–1.660) | 0.040* |
*, P<0.05. TCGA-LUAD, The Cancer Genome Atlas-lung adenocarcinoma; CI, confidence interval.
Next, we developed a clinical prediction model for LUAD patients based on multivariate Cox regression analysis of patient characteristics and PSMD14 expression. The model could illustrate the combined effect of clinical characteristics and PSMD14 expression on prognosis more clearly (Figure 5E). To validate the accuracy of the model, we used bootstrap resampling with 1,000 repetitions. The calibration curves of the model showed high agreement between predicted and observed survival rates at 1, 3, and 5 years, close to the ideal value (Figure 5F).
LUAD progression enhanced by PSMD14 upregulation
To validate the bioinformatic analysis, we measured PSMD14 expression levels in LUAD cell lines (A549, H1299, and H358) and tissues by RT-qPCR assay. We found that PSMD14 was significantly upregulated in cell lines and tissues compared with normal lung epithelial cells (BEAS-2B) and paracancer tissues, respectively (Figure 6A). We then evaluated the protein expression of PSMD14 by Western blot, which showed that PSMD14 was significantly overexpressed in LUAD cells relative to BEAS-2B cells (Figure 6B). We also analyzed 6 LUAD tissues with a diameter greater than 0.5 cm from a total of 22 patients and their corresponding adjacent normal tissues (Figure 6C). Western blot analysis revealed that PSMD14 protein expression was also markedly elevated in LUAD tissues relative to normal tissues.
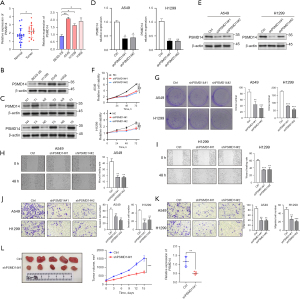
To investigate how PSMD14 influenced the malignant behaviors of LUAD cells, such as proliferation, invasion, and migration, we knocked down PSMD14 expression in A549 and H1299 cells using shPSMD14#1 and shPSMD14#2. We verified the knockdown efficiency by RT-qPCR and Western blot (Figure 6D,6E). We then assessed cell proliferation by CCK-8 assay and found that PSMD14 knockdown significantly reduced cell activity compared to the control group (Figure 6F). In addition, we measured cell clonogenicity by colony formation assay and observed that PSMD14 knockdown decreased cell clonogenicity (Figure 6G). These results indicated that lowering PSMD14 expression could inhibit LUAD cell proliferation in vitro. We also investigated how PSMD14 affected the invasion and migration ability by scratch and transwell assays. As shown in Figure 6H and Figure 6I, PSMD14 knockdown reduced cell migration of A549 and H1299 cells in scratch assays. Similarly, transwell assays showed that PSMD14 knockdown decreased cell invasion and migration of A549 and H1299 cells (Figure 6J,6K). These results demonstrated that PSMD14 knockdown could inhibit LUAD cell invasion and migration in vitro.
We then used a subcutaneous tumor model with nude mice to test how PSMD14 influenced LUAD cell proliferation in vivo. Knockdown of PSMD14 in A549 cells significantly reduced subcutaneous tumor size, growth rate, and weight compared to the control group (Figure 6L). This indicated that PSMD14 promoted LUAD cell proliferation in vivo. In summary, our results demonstrated that PSMD14 expression was essential for LUAD cell proliferation, invasion, and migration as inhibiting it significantly reduced these processes.
AGR2 protein stability is regulated by PSMD14 via deubiquitination
The role of PSMD14 in LUAD cell malignancy was explored by examining its possible binding proteins using the BioGRID database. The database suggested 513 proteins that could interact with PSMD14, with most of them supported by Co-IP and mass spectrometry. Among the 100 most reliable proteins (Table S3), we verified AGR2 as a relevant target for further validation. We then used Co-IP to confirm the interaction between PSMD14 and AGR2 proteins in different cell lines. We first transfected HEK-293T cells with HA-PSMD14 and Myc-AGR2 plasmids and performed exogenous Co-IP using anti-HA tag antibody. Figure 7A shows that Myc tag protein was detected in Co-IP samples, indicating that PSMD14 directly binds to AGR2 protein. We then conducted endogenous bidirectional Co-IP in LUAD cell lines A549 and H1299 using anti-PSMD14 antibody or anti-AGR2 antibody as baits. We observed that both antibodies co-immunoprecipitated their respective target proteins as well as their interacting partners (Figure 7B). These results demonstrated that PSMD14 interacted with AGR2 protein in LUAD cells.
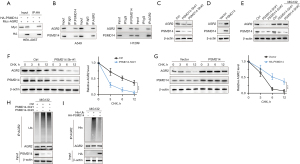
Subsequently, the role of PSMD14 in deubiquitinating AGR2 protein levels was investigated. We used Western blot to measure AGR2 protein levels after PSMD14 knockdown and overexpression. We found that PSMD14 knockdown reduced AGR2 protein levels, while PSMD14 overexpression increased them (Figure 7C,7D). This was reversed by MG132, a proteasome inhibitor, indicating that PSMD14 acted on AGR2 through the ubiquitin-proteasome pathway (Figure 7E). We also tested the stability of AGR2 protein by cycloheximide (CHX) chase experiments and observed that PSMD14 knockdown increased AGR2 degradation rate, while PSMD14 overexpression decreased it (Figure 7F,7G). These data suggested that PSMD14 stabilized AGR2 protein. We then performed immunoprecipitation (IP) assays using anti-AGR2 antibodies to detect the ubiquitin (Ub) levels of AGR2 protein in A549 cells with PSMD14 knockdown or overexpression. We found that PSMD14 knockdown (shPSMD14#1 and shPSMD14#2) significantly increased the ubiquitination of AGR2 protein, whereas PSMD14 overexpression significantly decreased it (Figure 7H,7I). These results suggested that PSMD14 modulated the stability of AGR2 protein by reducing its ubiquitination.
PSMD14 influences the malignant behavior of LUAD cells through AGR2
We hypothesized that PSMD14 promoted LUAD cell proliferation, invasion, and metastasis by regulating AGR2 protein expression. To test this hypothesis, we performed a rescue experiment using A549 and H1299 cells with different combinations of PSMD14 and AGR2 expression levels (Figure 8A). We assessed the effects of these manipulations on cell viability, proliferation, invasion, and migration using CCK-8, colony formation, wound healing, and transwell assays, respectively. The results showed that AGR2 knockdown significantly reversed the increased cell viability (Figure 8B), proliferation (Figure 8C), invasion, and migration (Figure 8D-8F) induced by PSMD14 overexpression. These results suggested that PSMD14 regulated LUAD cell behavior by modulating AGR2 protein expression.

Discussion
Recently, a significant amount of research has focused on identifying and characterizing genes that regulate cancer cell growth and that may serve as therapeutic targets. These studies have provided a promising outlook for cancer treatment. A number of protein-coding genes, whether mutated or unmutated, have been identified as potential targets for cancer therapy, with a particular emphasis on enzymes, which represent a major focus for the development of small-molecule anticancer drugs (44-46). The Sanger Institute has identified 627 priority targets, of which 232 are enzymes (37%). However, 395 targets do not belong to the enzyme category but to other proteins such as transcription factors, which typically lack activation sites for essential functions or active sites targeted by small-molecule inhibitors (45). Targeted protein degradation (TPD) offers a promising approach for eliminating target proteins and may hold great potential for cancer treatment (47). The involvement of the ubiquitin-proteasome system in this process underscores the need to understand the molecular mechanisms underlying TPD as this knowledge may lead to the development of new strategies for tumor therapy.
Traditional next-generation sequencing involves analyzing bulk tissue blocks to qualitatively characterize complex tissues, providing an average assessment of the levels of cancer and non-cancer cells (48-50). While this approach has contributed significantly to cancer research, it overlooks the unique phenotypic and functional characteristics of individual cells within tumor samples (51-53). Single-cell sequencing provides a means of assessing the risk of tumor susceptibility based on changes in single-cell expression, monitoring tumor progression, and formulating targeted intervention strategies at an early stage to prevent tumor development (54-56). It also enables researchers to analyze ubiquitination genes, transcription changes, and protein ubiquitination status at the single-cell level, providing a high-resolution view of the heterogeneity of ubiquitination modifications within cell populations. The combined analysis of single-cell sequencing and ubiquitination modification has been reported in glioma, laying the foundation for understanding the role of ubiquitination genes in glioma and identifying USP4 as a potential biomarker for this type of tumor (57). By exploring the heterogeneity of ubiquitination modifications in individual cancer cells, researchers can gain insight into the diversity and complexity of cellular states present in tumors, identify new therapeutic targets, and predict response to therapy.
In this study, the GSE117570, GSE131907, GSE149655, and GSE123902 datasets were utilized for scRNA-seq analysis. Cell subsets were obtained and annotated based on a study by Wu et al. on single-cell sequencing in NSCLC (41). We used InferCNV to detect cell copy number changes, which allowed us to evaluate the benign and malignant cells. Additionally, we used AUCell to calculate the AUC values of E1, E2, E3, and DUBs in epithelial cells to assess their relative degree. Our results indicated that, with the exception of E1, the AUC values of E2, E3, and DUBs displayed a normal distribution in epithelial cells, implying that they may be generally functional. In addition, based on the InferCNV results, we evaluated the variation in enzyme modification between malignant cells and normal epithelial cells. The results showed that the AUC values of E2, E3, and DUBs in malignant cells were significantly higher than those in normal cells, suggesting a more extensive ubiquitination modification in tumor cells. We then performed a comprehensive bioinformatics analysis by integrating scRNA-seq data with bulk data. Through batch prognostic analysis of ubiquitination modified-related molecules, we identified 53 genes that displayed prognostic differences in at least 3 datasets. Subsequently, we conducted batch differential analysis, which led to the identification of FGR, PSMD14, and ZBTB16 as differentially expressed genes exhibiting prognostic significance. Several studies have been observed that these genes are involved in the oncogenesis of LUAD (58-61). In addition, our analysis revealed a marked downregulation in the expression of FGR and ZBTB16, whereas the expression of PSMD14 was significantly upregulated in LUAD. Further analysis of the single-cell data set revealed that PSMD14 might have played a critical role in driving the pathogenesis of LAUD, as evidenced by its significant upregulation in the malignant cells. Therefore, we directed our focus towards exploring the functional significance of PSMD14.
Through analysis of TCGA-LUAD whole-genome sequencing data, we identified two missense mutation sites within the PSMD14 gene. Additionally, our investigation revealed a substantial association between CNV of PSMD14 and its mRNA expression, indicating that certain factors in LUAD might drive the copy number amplification of PSMD14, leading to its overexpression. These findings suggested a potential role for PSMD14 in the occurrence and progression of LUAD. To determine the clinical prognostic significance of the PSMD14 gene in LUAD, we conducted an analysis of patient survival data. The results revealed a strong association between high expression of PSMD14 and unfavorable outcomes in OS, PFS, and DSS. Furthermore, multivariate Cox analysis revealed that tumor stage and PSMD14 expression were independent prognostic factors when combined with age, sex, race, and stage. These results indicated that the PSMD14 gene may serve as a potential prognostic marker in patients with LUAD. To further evaluate the impact of various factors on patient prognosis, we constructed a nomogram based on the results of multivariate Cox regression and generated calibration curves, which demonstrated high accuracy at 1, 3, and 5 years. In summary, we investigated differences in ubiquitination modification between LUAD and normal epithelial cells at the single-cell level and found that PSMD14 gene expression was significantly increased in LUAD patients. Our findings suggested that PSMD14 may have played a critical role in the progression of LUAD and that targeting this gene may offer potential therapeutic benefits.
To validate our bioinformatic findings, we carried out experimental verification utilizing cell lines and tissue specimens. Using RT-qPCR and Western blotting, we assessed the expression of PSMD14 mRNA and protein in LUAD cells and tissues and in normal cells and tissues, respectively. Our findings demonstrated that the expression of PSMD14 was markedly upregulated in LUAD cells and tissues compared to their normal counterparts. Additionally, the cell phenotypic results showed that PSMD14 knockdown decreased the proliferation, invasion, and metastasis ability of the cells. Furthermore, subcutaneous tumor formation experiments in nude mice revealed that PSMD14 knockdown significantly inhibited the growth rate and size of subcutaneous graft tumors, suggesting that PSMD14 promoted the malignant biological behavior of LUAD.
We then explored the potential substrate proteins that interacted with PSMD14, a deubiquitination enzyme that has been implicated in the pathogenesis of several cancers, including esophageal squamous cell carcinoma (62), head and neck squamous cell carcinoma (63), and glioma (64). Through analysis of the BioGRID database, we identified several substrate proteins that interacted with PSMD14, among which the AGR2 protein was selected for further investigation based on the strength of evidence and the characteristics of the substrate protein itself (65). AGR2 is a member of the disulfide isomerase family and plays a role in cell invasion and metastasis as well as inhibiting the tumor suppressor p53 (66). To verify that PSMD14 regulated AGR2 through deubiquitination, we performed endogenous and exogenous Co-IP experiments and confirmed that PSMD14 directly binds to AGR2 protein. We also treated LUAD cells with MG132 to assess the impact of PSMD14 on AGR2 protein stability and ubiquitination levels, as measured by CHX assay and IP. Our results showed that PSMD14 reduced the ubiquitination level of AGR2 protein and enhanced its stability. In addition, we conducted CCK-8, colony formation, cell scratch, and transwell assays to investigate the effect of PSMD14-mediated AGR2 regulation on cell activity, invasion, and migration in LUAD cells. The results showed that knockdown of AGR2 significantly inhibited the enhancement of cell activity, invasion, and migration caused by overexpression of PSMD14. These findings suggested that PSMD14 regulated AGR2 to impact the proliferation, invasion, and migration of LUAD cells.
LUAD is a complex disease characterized by aberrant activation of multiple signaling pathways that promote tumor progression and metastasis. E3 ubiquitin ligases and deubiquitination enzymes have been shown to play important roles in LUAD by affecting the malignant biological behavior of tumor cells. For instance, Tantai et al. reported that TRIM46 could promote glycolysis and chemotherapy resistance of lung cancer cells by modifying PHLPP2 ubiquitination to activate the AKT/HK2 signaling pathway (67). Similarly, Sun et al. demonstrated that RNF6 enhanced chemotherapy resistance in LUAD by transcriptionally activating proliferating cell nuclear antigen expression and attenuating DNA damage (68). USP4 has also been implicated in LUAD by regulating the metastasis of cancer cells through the stabilization of β-catenin (69). The expression of USP4 and PSMD14 is associated with poorer clinical outcomes in LUAD patients (59). In addition to these enzymes, PSMD14 has also been reported in LUAD. Zhang et al. reported that the expression of deubiquitinase PSMD14 was upregulated in LUAD and had prognostic value (61). However, their study lacked multiomics analysis of PSMD14 and did not investigate the mechanism of PSMD14 in LUAD. This study utilized a combination of bioinformatics analysis and experiments to investigate the role of PSMD14 in LUAD. We found that PSMD14 was involved in the malignant biological behavior of LUAD cells and could affect cell proliferation, invasion, and migration by regulating AGR2. Although this study provided important insights into the function of PSMD14 in LUAD, some limitations remain. For example, further rigorous verification of the interaction between PSMD14 and AGR2 protein is needed, as well as site-binding verification of PSMD14 with AGR2 protein. Additionally, the clinical sample size needs to be expanded to confirm the potential use of PSMD14 as a marker for clinical monitoring. In the current context of LUAD research, it is crucial to determine if newly identified biomarkers are associated with actionable mutations. However, no studies have reported a potential link between PSMD14 and actionable mutations.
Conclusions
In summary, the integration of single-cell sequencing and bulk sequencing data in this study offered a robust approach for characterizing the ubiquitination modification differences in diverse cell types within the tumor microenvironment of LUAD. Our study further established the critical role of PSMD14 and provided insights into the molecular mechanisms by which PSMD14 regulates the malignant behavior of cancer cells. These findings provided a novel understanding of the pathogenesis and treatment of LUAD and demonstrated that PSMD14 holds potential as a new biomarker for monitoring and treating this disease.
Acknowledgments
Funding: This work was supported by the National Key R&D Plan, Ministry of Science and Technology of the People’s Republic of China (No. 2017YFC1308700).
Footnote
Reporting Checklist: The authors have completed the TRIPOD reporting checklist. Available at https://jtd.amegroups.com/article/view/10.21037/jtd-23-795/rc
Data Sharing Statement: Available at https://jtd.amegroups.com/article/view/10.21037/jtd-23-795/dss
Peer Review File: Available at https://jtd.amegroups.com/article/view/10.21037/jtd-23-795/prf
Conflicts of Interest: All authors have completed the ICMJE uniform disclosure form (available at https://jtd.amegroups.com/article/view/10.21037/jtd-23-795/coif). RPM has received lecture fees for AstraZeneca, Amgen, Bayer, Merck Sharp and Dohme, Pfizer, and support for attending meeting “ESMO 2022” from Amgen. The other authors have no conflicts of interest to declare.
Ethical Statement: The authors are accountable for all aspects of the work in ensuring that questions related to the accuracy or integrity of any part of the work are appropriately investigated and resolved. The study was conducted in accordance with the Declaration of Helsinki (as revised in 2013). Patient specimens were obtained with the approval of the Ethics Committee of The Second Affiliated Hospital of Harbin Medical University (Ethics No. 2021-166) and with the consent of the patients. Animal experiments were performed under a project license (No. 2021-167) granted by The Animal Care and Use Committee of Harbin Medical University, in compliance with institutional guidelines for the care and use of animals.
Open Access Statement: This is an Open Access article distributed in accordance with the Creative Commons Attribution-NonCommercial-NoDerivs 4.0 International License (CC BY-NC-ND 4.0), which permits the non-commercial replication and distribution of the article with the strict proviso that no changes or edits are made and the original work is properly cited (including links to both the formal publication through the relevant DOI and the license). See: https://creativecommons.org/licenses/by-nc-nd/4.0/.
References
- Xia C, Dong X, Li H, et al. Cancer statistics in China and United States, 2022: profiles, trends, and determinants. Chin Med J (Engl) 2022;135:584-90. [Crossref] [PubMed]
- Siegel RL, Miller KD, Fuchs HE, et al. Cancer statistics, 2022. CA Cancer J Clin 2022;72:7-33. [Crossref] [PubMed]
- Chen P, Liu Y, Wen Y, et al. Non-small cell lung cancer in China. Cancer Commun (Lond) 2022;42:937-70. [Crossref] [PubMed]
- Devarakonda S, Li Y, Martins Rodrigues F, et al. Genomic Profiling of Lung Adenocarcinoma in Never-Smokers. J Clin Oncol 2021;39:3747-58. [Crossref] [PubMed]
- Rivera GA, Wakelee H. Lung Cancer in Never Smokers. Adv Exp Med Biol 2016;893:43-57. [Crossref] [PubMed]
- He T, Cao J, Xu J, et al. Minimally invasive therapies for early stage non-small cell lung cancer. Zhongguo Fei Ai Za Zhi 2020;23:479-86. [PubMed]
- Collins LG, Haines C, Perkel R, et al. Lung cancer: diagnosis and management. Am Fam Physician 2007;75:56-63. [PubMed]
- Seguin L, Durandy M, Feral CC. Lung Adenocarcinoma Tumor Origin: A Guide for Personalized Medicine. Cancers (Basel) 2022;14:1759. [Crossref] [PubMed]
- Sun R, Hou Z, Zhang Y, et al. Drug resistance mechanisms and progress in the treatment of EGFR-mutated lung adenocarcinoma. Oncol Lett 2022;24:408. [Crossref] [PubMed]
- Chaft JE, Shyr Y, Sepesi B, et al. Preoperative and Postoperative Systemic Therapy for Operable Non-Small-Cell Lung Cancer. J Clin Oncol 2022;40:546-55. [Crossref] [PubMed]
- Ruiz-Cordero R, Devine WP. Targeted Therapy and Checkpoint Immunotherapy in Lung Cancer. Surg Pathol Clin 2020;13:17-33. [Crossref] [PubMed]
- Miller M, Hanna N. Advances in systemic therapy for non-small cell lung cancer. BMJ 2021;375: [Crossref] [PubMed]
- Hirsch FR, Scagliotti GV, Mulshine JL, et al. Lung cancer: current therapies and new targeted treatments. Lancet 2017;389:299-311. [Crossref] [PubMed]
- Suvà ML, Tirosh I. Single-Cell RNA Sequencing in Cancer: Lessons Learned and Emerging Challenges. Mol Cell 2019;75:7-12. [Crossref] [PubMed]
- Zhang L, Zhang Y, Wang C, et al. Integrated single-cell RNA sequencing analysis reveals distinct cellular and transcriptional modules associated with survival in lung cancer. Signal Transduct Target Ther 2022;7:9. [Crossref] [PubMed]
- Ochocka N, Segit P, Walentynowicz KA, et al. Single-cell RNA sequencing reveals functional heterogeneity of glioma-associated brain macrophages. Nat Commun 2021;12:1151. [Crossref] [PubMed]
- Li Q, Cheng Z, Zhou L, et al. Developmental Heterogeneity of Microglia and Brain Myeloid Cells Revealed by Deep Single-Cell RNA Sequencing. Neuron 2019;101:207-223.e10. [Crossref] [PubMed]
- Lei Y, Tang R, Xu J, et al. Applications of single-cell sequencing in cancer research: progress and perspectives. J Hematol Oncol 2021;14:91. [Crossref] [PubMed]
- Guo X, Zhang Y, Zheng L, et al. Global characterization of T cells in non-small-cell lung cancer by single-cell sequencing. Nat Med 2018;24:978-85. [Crossref] [PubMed]
- Faktor J, Pjechová M, Hernychová L, et al. Protein Ubiquitination Research in Oncology. Klin Onkol 2019;32:56-64. [Crossref] [PubMed]
- Popovic D, Vucic D, Dikic I. Ubiquitination in disease pathogenesis and treatment. Nat Med 2014;20:1242-53. [Crossref] [PubMed]
- Mansour MA. Ubiquitination: Friend and foe in cancer. Int J Biochem Cell Biol 2018;101:80-93. [Crossref] [PubMed]
- Nandi D, Tahiliani P, Kumar A, et al. The ubiquitin-proteasome system. J Biosci 2006;31:137-55. [Crossref] [PubMed]
- Hyer ML, Milhollen MA, Ciavarri J, et al. A small-molecule inhibitor of the ubiquitin activating enzyme for cancer treatment. Nat Med 2018;24:186-93. [Crossref] [PubMed]
- Park J, Cho J, Song EJ. Ubiquitin-proteasome system (UPS) as a target for anticancer treatment. Arch Pharm Res 2020;43:1144-61. [Crossref] [PubMed]
- Song Q, Hawkins GA, Wudel L, et al. Dissecting intratumoral myeloid cell plasticity by single cell RNA-seq. Cancer Med 2019;8:3072-85. [Crossref] [PubMed]
- Kim N, Kim HK, Lee K, et al. Single-cell RNA sequencing demonstrates the molecular and cellular reprogramming of metastatic lung adenocarcinoma. Nat Commun 2020;11:2285. [Crossref] [PubMed]
- Dost AFM, Moye AL, Vedaie M, et al. Organoids Model Transcriptional Hallmarks of Oncogenic KRAS Activation in Lung Epithelial Progenitor Cells. Cell Stem Cell 2020;27:663-678.e8. [Crossref] [PubMed]
- Laughney AM, Hu J, Campbell NR, et al. Regenerative lineages and immune-mediated pruning in lung cancer metastasis. Nat Med 2020;26:259-69. [Crossref] [PubMed]
- Bild AH, Yao G, Chang JT, et al. Oncogenic pathway signatures in human cancers as a guide to targeted therapies. Nature 2006;439:353-7. [Crossref] [PubMed]
- Takeuchi T, Tomida S, Yatabe Y, et al. Expression profile-defined classification of lung adenocarcinoma shows close relationship with underlying major genetic changes and clinicopathologic behaviors. J Clin Oncol 2006;24:1679-88. [Crossref] [PubMed]
- Rousseaux S, Debernardi A, Jacquiau B, et al. Ectopic activation of germline and placental genes identifies aggressive metastasis-prone lung cancers. Sci Transl Med 2013;5:186ra66. [Crossref] [PubMed]
- Okayama H, Kohno T, Ishii Y, et al. Identification of genes upregulated in ALK-positive and EGFR/KRAS/ALK-negative lung adenocarcinomas. Cancer Res 2012;72:100-11. [Crossref] [PubMed]
- Der SD, Sykes J, Pintilie M, et al. Validation of a histology-independent prognostic gene signature for early-stage, non-small-cell lung cancer including stage IA patients. J Thorac Oncol 2014;9:59-64. [Crossref] [PubMed]
- Selamat SA, Chung BS, Girard L, et al. Genome-scale analysis of DNA methylation in lung adenocarcinoma and integration with mRNA expression. Genome Res 2012;22:1197-211. [Crossref] [PubMed]
- Xu L, Lu C, Huang Y, et al. SPINK1 promotes cell growth and metastasis of lung adenocarcinoma and acts as a novel prognostic biomarker. BMB Rep 2018;51:648-53. [Crossref] [PubMed]
- Mayakonda A, Lin DC, Assenov Y, et al. Maftools: efficient and comprehensive analysis of somatic variants in cancer. Genome Res 2018;28:1747-56. [Crossref] [PubMed]
- Zhou J, Xu Y, Lin S, et al. iUUCD 2.0: an update with rich annotations for ubiquitin and ubiquitin-like conjugations. Nucleic Acids Res 2018;46:D447-53. [Crossref] [PubMed]
- Satija R, Farrell JA, Gennert D, et al. Spatial reconstruction of single-cell gene expression data. Nat Biotechnol 2015;33:495-502. [Crossref] [PubMed]
- Lever J, Krzywinski M, Altman N. Principal component analysis. Nature Methods 2017;14:641-2. [Crossref]
- Wu F, Fan J, He Y, et al. Single-cell profiling of tumor heterogeneity and the microenvironment in advanced non-small cell lung cancer. Nat Commun 2021;12:2540. [Crossref] [PubMed]
- Aibar S, González-Blas CB, Moerman T, et al. SCENIC: single-cell regulatory network inference and clustering. Nat Methods 2017;14:1083-6. [Crossref] [PubMed]
- Harrell FE Jr, Harrell MFE Jr, Hmisc DJVU. Package ‘rms’. 2017, 229:Q8. Available online: https://cran.r-project.org/web/packages/rms/index.html
- McDonald ER 3rd, de Weck A, Schlabach MR, et al. Project DRIVE: A Compendium of Cancer Dependencies and Synthetic Lethal Relationships Uncovered by Large-Scale, Deep RNAi Screening. Cell 2017;170:577-592.e10. [Crossref] [PubMed]
- Behan FM, Iorio F, Picco G, et al. Prioritization of cancer therapeutic targets using CRISPR-Cas9 screens. Nature 2019;568:511-6. [Crossref] [PubMed]
- Peng Y, Liu J, Inuzuka H, et al. Targeted protein posttranslational modifications by chemically induced proximity for cancer therapy. J Biol Chem 2023;299:104572. [Crossref] [PubMed]
- Zhao L, Zhao J, Zhong K, et al. Targeted protein degradation: mechanisms, strategies and application. Signal Transduct Target Ther 2022;7:113. [Crossref] [PubMed]
- van Dijk EL, Auger H, Jaszczyszyn Y, et al. Ten years of next-generation sequencing technology. Trends Genet 2014;30:418-26. [Crossref] [PubMed]
- Behjati S, Tarpey PS. What is next generation sequencing? Arch Dis Child Educ Pract Ed 2013;98:236-8. [Crossref] [PubMed]
- Levy SE, Boone BE. Next-Generation Sequencing Strategies. Cold Spring Harb Perspect Med 2019;9:a025791. [Crossref] [PubMed]
- Stark R, Grzelak M, Hadfield J. RNA sequencing: the teenage years. Nat Rev Genet 2019;20:631-56. [Crossref] [PubMed]
- Gu Y, Lawrence T, Mohamed R, et al. The emerging roles of interstitial macrophages in pulmonary fibrosis: A perspective from scRNA-seq analyses. Front Immunol 2022;13:923235. [Crossref] [PubMed]
- Zhao J, Shi Y, Cao G. The Application of Single-Cell RNA Sequencing in the Inflammatory Tumor Microenvironment. Biomolecules 2023;13:344. [Crossref] [PubMed]
- Sklavenitis-Pistofidis R, Getz G, Ghobrial I. Single-cell RNA sequencing: one step closer to the clinic. Nat Med 2021;27:375-6. [Crossref] [PubMed]
- Haque A, Engel J, Teichmann SA, et al. A practical guide to single-cell RNA-sequencing for biomedical research and clinical applications. Genome Med 2017;9:75. [Crossref] [PubMed]
- Lee HW, Chung W, Lee HO, et al. Single-cell RNA sequencing reveals the tumor microenvironment and facilitates strategic choices to circumvent treatment failure in a chemorefractory bladder cancer patient. Genome Med 2020;12:47. [Crossref] [PubMed]
- Tang Q, Chen Z, Xie J, et al. Transcriptome analysis and single-cell sequencing analysis constructed the ubiquitination-related signature in glioma and identified USP4 as a novel biomarker. Front Immunol 2022;13:915709. [Crossref] [PubMed]
- Chen Q, Ma J, Wang X, et al. Identification of prognostic candidate signatures by systematically revealing transcriptome characteristics in lung adenocarcinoma with differing tumor microenvironment immune phenotypes. Aging (Albany NY) 2022;14:4786-818. [Crossref] [PubMed]
- Lei J, Liu X, Liu W, et al. The prognostic value of USP14 and PSMD14 expression in non-small cell lung cancer. Ann Transl Med 2021;9:1019. [Crossref] [PubMed]
- Nie W, Hu MJ, Zhang Q, et al. DUBR suppresses migration and invasion of human lung adenocarcinoma cells via ZBTB11-mediated inhibition of oxidative phosphorylation. Acta Pharmacol Sin 2022;43:157-66. [Crossref] [PubMed]
- Zhang L, Xu H, Ma C, et al. Upregulation of deubiquitinase PSMD14 in lung adenocarcinoma (LUAD) and its prognostic significance. J Cancer 2020;11:2962-71. [Crossref] [PubMed]
- Zhu R, Liu Y, Zhou H, et al. Deubiquitinating enzyme PSMD14 promotes tumor metastasis through stabilizing SNAIL in human esophageal squamous cell carcinoma. Cancer Lett 2018;418:125-34. [Crossref] [PubMed]
- Jing C, Duan Y, Zhou M, et al. Blockade of deubiquitinating enzyme PSMD14 overcomes chemoresistance in head and neck squamous cell carcinoma by antagonizing E2F1/Akt/SOX2-mediated stemness. Theranostics 2021;11:2655-69. [Crossref] [PubMed]
- Zhi T, Jiang K, Xu X, et al. ECT2/PSMD14/PTTG1 axis promotes the proliferation of glioma through stabilizing E2F1. Neuro Oncol 2019;21:462-73. [Crossref] [PubMed]
- Tiemann K, Garri C, Lee SB, et al. Loss of ER retention motif of AGR2 can impact mTORC signaling and promote cancer metastasis. Oncogene 2019;38:3003-18. [Crossref] [PubMed]
- Zhang Z, Li H, Deng Y, et al. AGR2-dependent nuclear import of RNA polymerase II constitutes a specific target of pancreatic ductal adenocarcinoma in the context of wild-type p53. Gastroenterology 2021;161:1601-1614.e23. [Crossref] [PubMed]
- Tantai J, Pan X, Chen Y, et al. TRIM46 activates AKT/HK2 signaling by modifying PHLPP2 ubiquitylation to promote glycolysis and chemoresistance of lung cancer cells. Cell Death Dis 2022;13:285. [Crossref] [PubMed]
- Sun Y, Sun H, Qi Y, et al. Ring finger protein 6 enhances chemo-resistance by transcriptionally activating proliferating cell nuclear antigen expression and attenuating DNA damage in lung adenocarcinoma. Cancer Lett 2022;534:215609. [Crossref] [PubMed]
- Hwang SJ, Lee HW, Kim HR, et al. Ubiquitin-specific protease 4 controls metastatic potential through β-catenin stabilization in brain metastatic lung adenocarcinoma. Sci Rep 2016;6:21596. [Crossref] [PubMed]
(English Language Editor: A. Mulywyk)