Construction of subtype classifiers and validation of a prognostic risk model based on hypoxia-associated lncRNAs for lung adenocarcinoma
Highlight box
Key findings
• We developed a 4 hypolncRNAs prognostic model in lung adenocarcinoma.
What is known and what is new?
• Hypoxia has been found in advanced solid tumors and is associated with poor prognosis in various tumors, including lung adenocarcinoma.
• We first constructed a hypoxia-associated lncRNAs prognostic signature in lung adenocarcinoma.
What is the implication, and what should change now?
• Our study contributed to the development of personalized therapy for LUAD and guide targeted drug selection was established using four of the hypolncRNAs.
Introduction
Lung adenocarcinoma (LUAD) is a global lethal malignancy with extensive molecular heterogeneity (1,2). Since 2015, the World Health Organization (WHO) has classified LUAD into inert invasive, microinvasive, and predominantly adnexal. Furthermore, LUADs can be classified as intermediate- or high-grade tumors based on the predominant invasive pattern(3). In recent years, the discovery of several therapeutic diagnostic molecular biomarkers for LUAD has significantly changed the classification system (4). Several subtypes have been delineated at the genomic, transcriptomic, and epigenomic levels, thereby offering new therapeutic opportunities for certain patients (5). Although marked progress has been made in the molecular subtyping of LUAD patients, there remains a lack of consensus on the specific biomarkers and genes that can be used for precise identification of the different molecular subtypes of LUAD.
A pathobiological hallmark of solid tumors, hypoxia is caused by an imbalance between the cellular consumption and availability of oxygen (6,7). This imbalance generates an intratumor oxygen gradient that contributes to tumor plasticity and heterogeneity and promotes tumor aggression and metastasis (8). Thus, hypoxia-associated classifiers may serve as reliable markers to characterize tumor heterogeneity. Based on hypoxia-associated genes, two hypoxia-associated molecular subtypes have been characterized for clear cell renal cell carcinoma, both having different clinical responses to and outcomes of targeted therapies, including immunotherapy (9). Additionally, by consistent clustering of 397 hypoxia-related genes and the development of models for diagnosis, prognosis, and recurrence, a robust hypoxia gene signature for prognostic prediction in these patients has been constructed (10). Hepatocellular carcinoma (HCC) hypoxia-associated noncoding RNAs can also classify cancer specimens. Chen et al. identified two heterogeneous molecular subtypes and constructed a risk model for bladder cancer based on the expression of hypoxia-associated long noncoding RNAs (hypolncRNAs), which substantially improved the prognostic prediction for bladder cancer patients with varied clinical conditions (11). A hypoxia- and immune-associated prognosis signature for esophageal squamous cell carcinoma was constructed (12). He et al. constructed a model for risk signatures based on 7 immune-associated lncRNAs and showed its prognostic value in LUAD (13). According to literature research, lncRNA regulates hypoxia mainly through transcriptional activation and partly through epigenetic regulation (14). It is certain that further understanding of lncrna regulating hypoxia will provide useful insights into its tumorigenicity and may lead to new clinical applications. Therefore, we reasonably speculated that hypolncRNAs may also help characterize the heterogeneity and prognosis prediction of LUAD.
In this study, we analyzed the hypolncRNAs of LUAD by bioinformatics and used consistent clustering analysis to identify specific molecular subgroups; the relationship between tumor microenvironment (TME) characteristics and immunotherapy among the different subgroups was also evaluated. Finally, a hypolncRNA signature was constructed to predict prognoses in LUAD. We present this article in accordance with the TRIPOD reporting checklist (available at https://jtd.amegroups.com/article/view/10.21037/jtd-23-952/rc).
Methods
LUAD datasets and samples
The workflow was showed in Figure 1. The transcriptomic data of six LUAD cohorts and the corresponding clinical annotations of patients were extracted from The Cancer Genome Atlas (TCGA; http://cancergenome.nih.gov/) data portal and the Gene Expression Omnibus (GEO, https://www.ncbi.nlm.nih.gov/geo/) database, including the GSE19188, GSE30219, GSE31210, GSE37745, and GSE50081 datasets. Patients with incomplete clinical annotations were removed. The ‘remove-batch-effect’ function in the limma package was used to eliminate batch effects. The genes in the GEO and TCGA samples were identified as mRNAs or lncRNAs according to the annotations on the GENCODE website. The study was conducted in accordance with the Declaration of Helsinki (as revised in 2013).
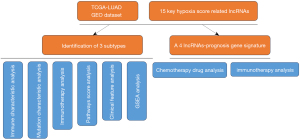
Identification of prognosis-related pathways in LUAD
Using the ‘GSVA’ package in R, single-sample gene set enrichment analysis (ssGSEA) was performed, and the scores of the samples in the GEO and TCGA cohorts were determined for each of the hallmark pathways. Univariate Cox regression analysis with LUAD overall survival (OS) was performed to identify pathways that were significantly (P<0.05) related to the prognoses of patients in both datasets.
Identification of hypoxia pathway-related lncRNAs
The hypolncRNA-related pathways were identified according to the procedure described in a previously published study (15). The mRNAs were sorted according to their decreasing order of relevance for specific lncRNAs and input into the ‘fgsea’ R package. Next, the hypoxia pathway enrichment scores (ESs) for all lncRNAs were computed, and those with significant ESs were selected. These lncRNAs were considered hypoxia pathway-derived lncRNAs. The first-order partial correlation coefficient (PCC) of an lncRNA, i, and a gene, j, after controlling tumor purity as a covariate was calculated as follows:
Herein, the Pearson correlation coefficients between lncRNA i and mRNA j, miRNA i and tumor purity, and mRNA j and tumor purity are represented by Rlncm, Rlncp, and Rmp, respectively.
To estimate the P value of PCC(ij), we performed the following calculation:
where pnorm represents the normal distribution and n represents the sample size.
Using the formula , the rank index (RI) of mRNA j was obtained. All mRNAs were sorted in descending order of their RIs, and GSEA was performed. The genes in each hypoxia pathway were mapped to the list of sorted genes. The ESs and P values were obtained using the ‘fgsea’ package in R and input into the following TES calculation formula: . The lncRNAs with a false discovery rate (FDR) <0.05 and |TES| >0.995 were defined as hypoxia pathway-derived lncRNAs.
Unsupervised clustering of hypoxia pathway-derived lncRNAs
The hypolncRNAs common to both the TCGA and GEO datasets were selected for clustering subtyping of LUAD, performed using the ‘ConsensuClusterPlus’ package in R. The clustering algorithm parameter was set at ‘km’, and the similarity between the samples was assessed by computing the ‘Euclidean’ distance. The number of iterations was set to 1,000 to ensure the stability of the classification. The number of clusters was assigned according to the k-value, and for the LUAD samples, it was defined as the figure of consensus cumulative distribution function (CDF).
Analysis of the tumor-free microenvironment among subgroups
The TME includes the blood vessels surrounding the tumor and various immune and nonimmune cell types. These cells secrete several signaling molecules (cytokines and chemokines) (16). In this study, the TME between different subtypes of LUAD was profiled by analyzing the expression of immune cell infiltration, immune components, stromal components, immune checkpoints, and chemokines between subgroups, wherein immune cell infiltration was predicted by analyzing immune cell marker genes. The stromal and immune components were assessed by predicting the immune and stromal scores, respectively, between the subgroups using the ESTIMATE method.
Differentially expressed genes and functional annotations between subgroups
Using Limma in R, differentially expressed gene (DEG) analysis among the subgroups was performed based on the cutoffs of FDR <0.05 and |FC| >1.5. The biological functions of DEGs were determined using WebGestaltR (V0.4.4) for Kyoto Encyclopedia of Genes and Genomes (KEGG) pathway enrichment analysis and Gene Ontology (GO) annotations; the significance cutoff value was set at FDR <0.05.
Construction of the hypolncRNA signature
By univariate and multivariate Cox regression analyses, the prognosis-related lncRNAs and their correlation coefficients were identified, and the risk scores of LUAD samples were determined according to the following calculation: risk score = ∑(regression coefficient of lncRNA × expression of signature lncRNA). OS in the low-risk and high-risk groups was determined according to the median risk score.
Prediction of drug sensitivity
Based on the PRISM and Cancer Therapeutics Response Portal (CTRP) version 2 databases, the drug sensitivities among low-risk and high-risk patients were predicted by ridge regression according to gene expression. The cell line expression profiles from the Cancer Cell Line Encyclopedia (CCLE) database were collated as the training set, while TCGA-LUAD was the test set for the prediction of drug sensitivities. Among high-risk patients, components having a significantly lower dose-response area under the curve (dr-AUC) were identified. Spearman correlation coefficients between dr-AUC and risk scores were determined. Components with a significantly negative value of rho (less than −0.2) were retained.
Statistical analysis
All statistical analyses were performed using R software [version 3.6.3 (http://www.r-project.org)]. Comparisons between two groups were made using the Wilcoxon test, while those among three or more groups were made using the Kruskal-Wallis test. All statistical tests were two-sided, and the significance level was set at P<0.05.
Results
Hypoxia pathway-derived lncRNA expression divides LUAD into three subgroups
Through univariate Cox regression, 13 prognosis-related pathways were identified from TCGA specimens and 26 from GEO specimens (Figure 2A,2B). The prognosis-related hypoxia pathways common to both databases were used as the targets. In the TCGA-LUAD cohort, 74 lncRNAs were associated with hypoxia pathways, while in the GEO cohort, 108 lncRNAs were involved in hypoxia pathways. Of these, 24 lncRNAs were common to both datasets (Figure S1). Among them, univariate Cox analysis showed that 14 lncRNAs were significantly related to the prognoses of LUAD patients. Unsupervised cluster analysis based on their expression showed that the consensus index of the CDF curve was the flattest at K=3; consequently, the LUAD samples obtained from both datasets were divided into three subgroups (Figure 2C,2D). Among the three subgroups, the OS of Cluster 1 (C1) was significantly greater than that of Cluster 3 (C3), and the OS of C3 was markedly greater than that of Cluster 2 (C2) (Figure 2E,2F).
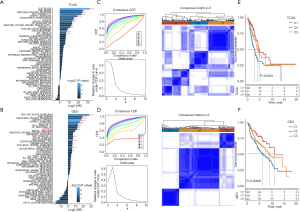
TME characteristics of hypoxia pathway-derived lncRNA-regulated subtypes
We observed an abundant infiltration of immune cell types, including B cells, T cells, neutrophils, and eosinophils, with different infiltration scores among the three hypolncRNA-regulated subtypes (Figure 3A,3B). Although the immune scores of the three hypolncRNA-based subgroups did not show any significant differences, the stromal scores of C2 and C3 were significantly higher than those of C1, which indicated a higher matrix content in the TME of C2 and C3 (Figure 3C). Similar trends were observed in the GEO cohorts (Figure 3D-3F). Analysis of immune checkpoint expression among the three hypolncRNA-based subgroups revealed the presence of several aberrantly expressed immune checkpoints in 31/47 TCGA-LUAD specimens and 25/41 GEO specimens (Figure 4A,4B). In the analysis of secreted chemokines and their receptors in the TME, more than 60% were significantly differentially expressed among the three-hypoxia pathway-derived lncRNA-based subtypes of TCGA-LUAD (Figure 4C,4D). The expression levels of 34 chemokines and 15 chemokine receptors were examined in the GEO cohorts. Significant differences in the expression of a considerable number of chemokine receptors (7/15) and chemokines (22/34) were observed among the three hypolncRNA-based subgroups (Figure 4E,4F), consistent with our expectation. In addition, several scores associated with the TME were evaluated, and it was observed that the IFNγ score (17), immune cytolytic (CYT) score (18), and cancer-associated fibroblast (CAF) score (19) were significantly different among the three hypolncRNA-based subgroups in TCGA samples. However, the angiogenic score (20) showed no statistically significant difference among the three subgroups (Figure 4G). In contrast, IFNγ and CAF scores were significantly different among the hypolncRNA-based subgroups in the GEO cohorts (Figure 4H). These results suggested that substantial heterogeneity existed in the TME among the three hypolncRNA-based subtypes.
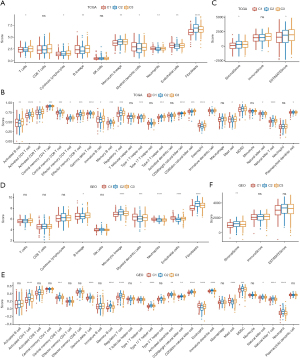
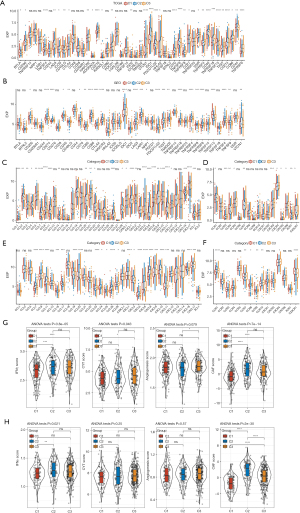
Combination of hypolncRNA-based subtypes and immune classification for prognostic stratification
We evaluated the relevance of the subtypes basis the classification derived from hypolncRNA expressions for the immune categories. Thorsson et al. proposed six immune classifications spanning multiple cancer types based on the immunogenomic analysis of more than 10,000 tumors as follows: C6 (TGF-β dominant), C5 (immunologically quiet), C4 (lymphocyte-depleted), C3 (inflammatory), C2 (IFN-γ dominant), and C1 (wound healing) (21). Comparing these six immune subtypes with those obtained based on hypolncRNA expression, the Sankey diagram illustrated the allocation of the subtypes according to hypolncRNA expression in the immune classifications (Figure 5A). Comparing the percentages of different immune subtypes in the three hypolncRNA-based subtypes, we observed that all of them were distributed among five immune subtypes, including C6, C4, C3, C2, and C1; the percentages of each immune subtype in the three hypolncRNA-based subtypes were significantly different. The most predominant immune subtypes in C1 were C2 (26%) and C3 (55%); those in C2 were C1 (31%), C2 (38%), C3 (14%), and C6 (14%); and those in C3 were C1 (17%), C2 (34%), and C3 (40%) (Figure 5B). Survival analysis of LUAD patients according to immune-molecular subtypes showed a significant difference in survival rates among LUAD patients belonging to the five immune subtype categories (Figure 5C). In addition, GSEA was performed to compare the functional attributes of C1, the subtype with the highest survival rate, and C2, the subtype with the lowest survival rate. Classical cancer-promoting signaling pathways, including the P53 signaling pathway, DNA replication, and the cell cycle, were found to be significantly enriched in C2 (Figure 5D,5E).
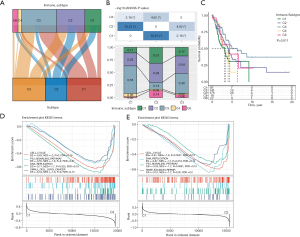
Evaluating potential immunotherapeutic responses
According to the different TMEs among the three hypolncRNA-based subtypes, we hypothesized that these patients may respond differently to immunotherapy. The Tumor Immune Dysfunction and Exclusion (TIDE) scores predict potential clinical responses to immunotherapy based on pretreatment tumor characteristics (19). We observed that the TIDE scores among the three hypolncRNA-regulated subtypes in the TCGA and GEO cohorts showed significant differences, with C1 having the lowest TIDE score (Figure 6A,6B). Myeloid-derived suppressor cells (MDSCs) are a heterogeneous population of immature myeloid cells with an immunosuppressive phenotype that renders tumors resistant to immunotherapy (22). MDSC scores differed significantly among the three subtypes classified by hypolncRNA expression, with C1 having the lowest score and C2 having the highest score, which implied that immunotherapy was most likely to be effective in C1 relative to C2 (Figure 6C,6D). Patients with true response had prolonged survival compared with those with false response in TCGA-LUAD (Figure 6E). Among the patients in the GEO cohorts, the difference in OS rate between the two states was not significant (Figure 6F).
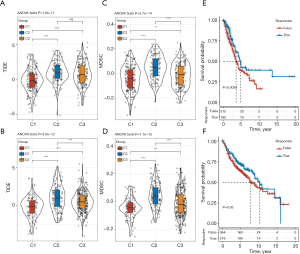
Construction of a prognostic signature consisting of four hypolncRNAs and prediction of responses to targeted drugs
The 14 hypolncRNAs screened by univariate Cox regression analysis were subjected to multivariate Cox regression analysis and stepAIC after removing the confounding variables. Four hypolncRNAs (MIR31HG, LINC00857, LINC01116, and AP000679.1) were used as components for the construction of the prognostic signature. MIR31HG, LINC00857, LINC01116 were obviously correlated with immune cells (Figure S2). The four selected hypolncRNA signatures were used to predict the risk scores for LUAD samples in the TCGA and GEO cohorts. The survival analysis showed a considerable survival advantage for patients with low-risk scores (Figure 7A,7B). We observed high drug sensitivity for three CTRP-derived compounds, paclitaxel, GSK461364, and SB-743921, in the high-risk patients (Figure 7C). Spearman correlation analysis for five PRISM-derived drugs and differential drug response analyses showed higher efficacies of ispinesib, NVP-AUY922, LY2606368, dolastatin-10, and cabazitaxel in patients with high scores (Figure 7D). The low-risk score was featured by lower TIDE score in TCGA and GEO dataset in comparison to high-risk score group, suggested more suitable to immunotherapy (Figure S3).
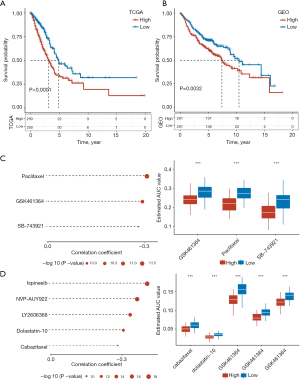
Discussion
Hypoxia is a representative microenvironmental feature in cancer that substantially hinders the efficacies of conventional treatments, including immunotherapy, chemotherapy, and radiotherapy (23). In the last few decades, accumulating evidence suggests the relevance of noncoding RNAs (ncRNAs) as molecular mediators for inducing a hypoxic response, as they play crucial roles in regulating hypoxia-related gene expression at the translational and posttranslational levels, as well as transcriptionally and post-transcriptionally (24). Many lncRNAs are also induced by hypoxia, and their mechanisms and functions in cancer have been described previously. For example, hypoxia-inducible lncRNA BX111 enhances the metastasis of pancreatic cancer by promoting the transcription of ZEB1 (25). The hypoxia-inducible lncRNA LUCAT1 interacts with polypyrimidine tract binding protein 1 (PTBP1) in colorectal cancer cells to promote cancer cell viability and drug resistance (26). Cancer progression is promoted by inhibiting HIF-1α degradation in ovarian cancer through the hypoxia-induced lncRNA MIR210HG(27). McCarty et al.’s study showed hypoxia-sensitive changes in histone methylation in the WT1 locus and pointed that these changes are dependent upon expression of the lncRNA (28). Yang et al. previously also showed that the HIF-1α-lincRNA-p21 axis played a vital role in promoting tumorigenesis (29). Therefore, we speculated that lncRNAs based on hypoxic profiles may have potential involvement in LUAD patients.
In this study, we classified LUAD patients into three subtypes using the transcriptomic profiles of hypolncRNAs. The LUAD samples in C1 showed a survival advantage, while those in C2 had the least favorable survival outcome. Several studies have suggested that hypoxia affects the TME in its entirety (8,30,31). Differences in the degrees of immune cell infiltration, stromal scores, and expression of secreted chemokines in the TME were detected among the three hypolncRNA-based subtypes. Overall, the TME of C2 was the least effective. A hypoxic TME is widely considered to be an independent prognostic indicator that consistently correlates with poor survival across different cancer types (32). This provided evidence for the poor prognosis in C2. Strong evidence suggests that an altered metabolic hypoxic TME inhibits immunotherapeutic efficacy. Several treatments targeting hypoxia exert synergistic effects with immunotherapy (33). Herein, our analysis suggested that immunotherapy was indeed the least effective in C2 among the three subtypes classified based on hypolncRNA expression.
Some previous studies have reported that multiple hypoxia-related signatures are associated with cancer prognoses. Yang et al. developed a hypoxia-related miRNA signature with strong predictive abilities for identifying patients at high risk of colorectal cancer (34). Lin et al. constructed a risk model dependent on hypoxia to assess the immune microenvironment and predict prognosis in glioma (35). Pei et al. constructed a hypoxia-related signature that was closely associated with the TME to predict prognosis in gastric cancer (36). Herein, we also constructed a signature consisting of four hypolncRNAs to assess the prognoses of patients with LUAD. With the exception of AP000679.1, the association of the remaining three hypolncRNAs with cancer development has been reported previously. MIR31HG exhibits oncogenic properties and is associated with head and neck cancer (37), melanoma (38), and cancers of the digestive system (39). The overexpression of LINC00857 accelerates the deterioration of HCC (40) and pancreatic cancer (41) conditions. LINC00857 has been shown to recruit the serine/arginine-rich splicing factor 1 (SRSF1) to promote alternative splicing (AS) of CLDN12, thereby affecting the phenotype of pancreatic adenocarcinoma cells (42). LINC01116 promotes tumor proliferation and metastasis in LUAD (43). LINC01116 was highly expressed in small cell lung cancer and promoting cell invasion and migration in small cell lung cancer through ceRNA method (44). We observed that the signature comprising four hypolncRNAs could not only predict the OS of LUAD but also help in determining targeted drugs with higher effectiveness for high-risk patients. Paclitaxel, GSK461364, SB-743921, ispinesib, NVP-AUY922, LY2606368, dolastatin-10, and cabazitaxel have been used for the treatment of high-risk patients.
Conclusions
In this study, 14 hypolncRNA classifiers found to be significantly associated with LUAD prognosis were identified, which consequently led to the classification of LUAD into three subtypes based on the expression of these hypolncRNAs. In addition, a risk score model capable of predicting LUAD prognoses and guiding targeted drug selection was established using four of the hypolncRNAs. However, some limitations to this study need to be emphasized and addressed in future studies. First, the data were retrospective and lacked details of clinical treatment, which could have introduced a potential bias in the results of our analysis. Second, the applicability of the lncRNA signature should be further evaluated in a large clinical sample size. Finally, further functional experiments to study its role in vivo and potential molecular mechanisms are necessary.
Acknowledgments
Funding: None.
Footnote
Reporting Checklist: The authors have completed the TRIPOD reporting checklist. Available at https://jtd.amegroups.com/article/view/10.21037/jtd-23-952/rc
Peer Review File: Available at https://jtd.amegroups.com/article/view/10.21037/jtd-23-952/prf
Conflicts of Interest: All authors have completed the ICMJE uniform disclosure form (available at https://jtd.amegroups.com/article/view/10.21037/jtd-23-952/coif). The authors have no conflicts of interest to declare.
Ethical Statement: The authors are accountable for all aspects of the work in ensuring that questions related to the accuracy or integrity of any part of the work are appropriately investigated and resolved. The study was conducted in accordance with the Declaration of Helsinki (as revised in 2013).
Open Access Statement: This is an Open Access article distributed in accordance with the Creative Commons Attribution-NonCommercial-NoDerivs 4.0 International License (CC BY-NC-ND 4.0), which permits the non-commercial replication and distribution of the article with the strict proviso that no changes or edits are made and the original work is properly cited (including links to both the formal publication through the relevant DOI and the license). See: https://creativecommons.org/licenses/by-nc-nd/4.0/.
References
- Devarakonda S, Morgensztern D, Govindan R. Genomic alterations in lung adenocarcinoma. Lancet Oncol 2015;16:e342-51. [Crossref] [PubMed]
- Sun S, Li S, Li J, et al. Prognosis of the second predominant subtype in lung adenocarcinoma: a retrospective single-center cohort study. J Thorac Dis 2022;14:4846-64. [Crossref] [PubMed]
- Borczuk AC. Prognostic considerations of the new World Health Organization classification of lung adenocarcinoma. Eur Respir Rev 2016;25:364-71. [Crossref] [PubMed]
- Calvayrac O, Pradines A, Pons E, et al. Molecular biomarkers for lung adenocarcinoma. Eur Respir J 2017;49:1601734. [Crossref] [PubMed]
- Cros J, Raffenne J, Couvelard A, et al. Tumor Heterogeneity in Pancreatic Adenocarcinoma. Pathobiology 2018;85:64-71. [Crossref] [PubMed]
- Rey S, Schito L, Wouters BG, et al. Targeting Hypoxia-Inducible Factors for Antiangiogenic Cancer Therapy. Trends Cancer 2017;3:529-41. [Crossref] [PubMed]
- Shi Y, Dai S, Lei Y. Development and validation of a combined metabolism and immune prognostic model in lung adenocarcinoma. J Thorac Dis 2022;14:4983-97. [Crossref] [PubMed]
- Jing X, Yang F, Shao C, et al. Role of hypoxia in cancer therapy by regulating the tumor microenvironment. Mol Cancer 2019;18:157. [Crossref] [PubMed]
- Zhong W, Zhong H, Zhang F, et al. Characterization of Hypoxia-Related Molecular Subtypes in Clear Cell Renal Cell Carcinoma to Aid Immunotherapy and Targeted Therapy via Multi-Omics Analysis. Front Mol Biosci 2021;8:684050. [Crossref] [PubMed]
- Sun X, Luo H, Han C, et al. Identification of a Hypoxia-Related Molecular Classification and Hypoxic Tumor Microenvironment Signature for Predicting the Prognosis of Patients with Triple-Negative Breast Cancer. Front Oncol 2021;11:700062. [Crossref] [PubMed]
- Chen X, Zhang Y, Wang F, et al. A Novel Assessment Model Based on Molecular Subtypes of Hypoxia-Related LncRNAs for Prognosis of Bladder Cancer. Front Cell Dev Biol 2021;9:718991. [Crossref] [PubMed]
- Lian L, Teng SB, Xia YY, et al. Development and verification of a hypoxia- and immune-associated prognosis signature for esophageal squamous cell carcinoma. J Gastrointest Oncol 2022;13:462-77. [Crossref] [PubMed]
- He C, Yin H, Zheng J, et al. Identification of immune-associated lncRNAs as a prognostic marker for lung adenocarcinoma. Transl Cancer Res 2021;10:998-1012. [Crossref] [PubMed]
- Chang YN, Zhang K, Hu ZM, et al. Hypoxia-regulated lncRNAs in cancer. Gene 2016;575:1-8. [Crossref] [PubMed]
- Li Y, Jiang T, Zhou W, et al. Pan-cancer characterization of immune-related lncRNAs identifies potential oncogenic biomarkers. Nat Commun 2020;11:1000. [Crossref] [PubMed]
- Pitt JM, Marabelle A, Eggermont A, et al. Targeting the tumor microenvironment: removing obstruction to anticancer immune responses and immunotherapy. Ann Oncol 2016;27:1482-92. [Crossref] [PubMed]
- Danilova L, Ho WJ, Zhu Q, et al. Programmed Cell Death Ligand-1 (PD-L1) and CD8 Expression Profiling Identify an Immunologic Subtype of Pancreatic Ductal Adenocarcinomas with Favorable Survival. Cancer Immunol Res 2019;7:886-95. [Crossref] [PubMed]
- Rooney MS, Shukla SA, Wu CJ, et al. Molecular and genetic properties of tumors associated with local immune cytolytic activity. Cell 2015;160:48-61. [Crossref] [PubMed]
- Jiang P, Gu S, Pan D, et al. Signatures of T cell dysfunction and exclusion predict cancer immunotherapy response. Nat Med 2018;24:1550-8. [Crossref] [PubMed]
- Masiero M, Simões FC, Han HD, et al. A core human primary tumor angiogenesis signature identifies the endothelial orphan receptor ELTD1 as a key regulator of angiogenesis. Cancer Cell 2013;24:229-41. [Crossref] [PubMed]
- Thorsson V, Gibbs DL, Brown SD, et al. The Immune Landscape of Cancer. Immunity 2018;48:812-830.e14. [Crossref] [PubMed]
- Tesi RJ. MDSC; the Most Important Cell You Have Never Heard Of. Trends Pharmacol Sci 2019;40:4-7. [Crossref] [PubMed]
- Shi R, Liao C, Zhang Q. Hypoxia-Driven Effects in Cancer: Characterization, Mechanisms, and Therapeutic Implications. Cells 2021;10:678. [Crossref] [PubMed]
- Barreca MM, Zichittella C, Alessandro R, et al. Hypoxia-Induced Non-Coding RNAs Controlling Cell Viability in Cancer. Int J Mol Sci 2021;22:1857. [Crossref] [PubMed]
- Deng SJ, Chen HY, Ye Z, et al. Hypoxia-induced LncRNA-BX111 promotes metastasis and progression of pancreatic cancer through regulating ZEB1 transcription. Oncogene 2018;37:5811-28. [Crossref] [PubMed]
- Huan L, Guo T, Wu Y, et al. Hypoxia induced LUCAT1/PTBP1 axis modulates cancer cell viability and chemotherapy response. Mol Cancer 2020;19:11. [Crossref] [PubMed]
- Liu P, Huang H, Qi X, et al. Hypoxia-Induced LncRNA-MIR210HG Promotes Cancer Progression By Inhibiting HIF-1α Degradation in Ovarian Cancer. Front Oncol 2021;11:701488. [Crossref] [PubMed]
- McCarty G, Loeb DM. Hypoxia-sensitive epigenetic regulation of an antisense-oriented lncRNA controls WT1 expression in myeloid leukemia cells. PLoS One 2015;10:e0119837. [Crossref] [PubMed]
- Yang F, Zhang H, Mei Y, et al. Reciprocal regulation of HIF-1α and lincRNA-p21 modulates the Warburg effect. Mol Cell 2014;53:88-100. [Crossref] [PubMed]
- Ancel J, Perotin JM, Dewolf M, et al. Hypoxia in Lung Cancer Management: A Translational Approach. Cancers (Basel) 2021;13:3421. [Crossref] [PubMed]
- Bai R, Li Y, Jian L, et al. The hypoxia-driven crosstalk between tumor and tumor-associated macrophages: mechanisms and clinical treatment strategies. Mol Cancer 2022;21:177. [Crossref] [PubMed]
- McDonald PC, Chafe SC, Dedhar S. Overcoming Hypoxia-Mediated Tumor Progression: Combinatorial Approaches Targeting pH Regulation, Angiogenesis and Immune Dysfunction. Front Cell Dev Biol 2016;4:27. [Crossref] [PubMed]
- Wang B, Zhao Q, Zhang Y, et al. Targeting hypoxia in the tumor microenvironment: a potential strategy to improve cancer immunotherapy. J Exp Clin Cancer Res 2021;40:24. [Crossref] [PubMed]
- Yang Y, Qu A, Wu Q, et al. Prognostic value of a hypoxia-related microRNA signature in patients with colorectal cancer. Aging (Albany NY) 2020;12:35-52. [Crossref] [PubMed]
- Lin W, Wu S, Chen X, et al. Characterization of Hypoxia Signature to Evaluate the Tumor Immune Microenvironment and Predict Prognosis in Glioma Groups. Front Oncol 2020;10:796. [Crossref] [PubMed]
- Pei JP, Zhang CD, Yusupu M, et al. Screening and Validation of the Hypoxia-Related Signature of Evaluating Tumor Immune Microenvironment and Predicting Prognosis in Gastric Cancer. Front Immunol 2021;12:705511. [Crossref] [PubMed]
- Wang R, Ma Z, Feng L, et al. LncRNA MIR31HG targets HIF1A and P21 to facilitate head and neck cancer cell proliferation and tumorigenesis by promoting cell-cycle progression. Mol Cancer 2018;17:162. [Crossref] [PubMed]
- Xu HL, Tian FZ. Clinical significance of lncRNA MIR31HG in melanoma. Eur Rev Med Pharmacol Sci 2020;24:4389-95. [PubMed]
- Zhou Y, Fan Y, Zhou X, et al. Significance of lncRNA MIR31HG in predicting the prognosis for Chinese patients with cancer: a meta-analysis. Biomark Med 2020;14:303-16. [Crossref] [PubMed]
- Xia C, Zhang XY, Liu W, et al. LINC00857 contributes to hepatocellular carcinoma malignancy via enhancing epithelial-mesenchymal transition. J Cell Biochem 2019;120:7970-7. [Crossref] [PubMed]
- Li T, Zhao H, Zhou H, et al. LncRNA LINC00857 strengthens the malignancy behaviors of pancreatic adenocarcinoma cells by serving as a competing endogenous RNA for miR-340-5p to upregulate TGFA expression. PLoS One 2021;16:e0247817. [Crossref] [PubMed]
- Zhang Y, Fang Y, Ma L, et al. LINC00857 regulated by ZNF460 enhances the expression of CLDN12 by sponging miR-150-5p and recruiting SRSF1 for alternative splicing to promote epithelial-mesenchymal transformation of pancreatic adenocarcinoma cells. RNA Biol 2022;19:548-59. [Crossref] [PubMed]
- Wang J, Gao J, Chen Q, et al. LncRNA LINC01116 Contributes to Cisplatin Resistance in Lung Adenocarcinoma. Onco Targets Ther 2020;13:9333-48. [Crossref] [PubMed]
- Liu W, Liang F, Yang G, et al. LncRNA LINC01116 sponges miR-93-5p to promote cell invasion and migration in small cell lung cancer. BMC Pulm Med 2021;21:50. [Crossref] [PubMed]