Selection of key genes for dilated cardiomyopathy based on machine learning algorithms and assessment of diagnostic accuracy
Highlight box
Key findings
• The purpose of this study is to screen key pathogenic genes and clarify the pathogenesis of the disease through the transcriptome sequencing data set of myocardial samples from patients with dilated cardiomyopathy.
What is known and what is new?
• At present, the mechanism of the occurrence and progression of Dilated cardiomyopathy is still unclear, and further exploration is still needed.
• This study found CCL5 may be related to immune cell enrichment and CTGF may be related to myocardial fibrosis.
What is the implication, and what should change now?
• CCL5 and CTGF are critical pathogenic genes in dilated cardiomyopathy, and they possess good diagnostic efficacy.
Introduction
Dilated cardiomyopathy is a common heart disease that is mainly caused by cardiac injury and apoptosis and increased ventricular wall thickness (1). Its etiology is unclear, but it may be related to genetic and environmental factors (2,3). It is estimated that there are approximately 25 million new cases each year (4). The incidence of dilated cardiomyopathy increases with age (5). The pathological and physiological mechanisms of dilated cardiomyopathy mainly include 2 parts: one is cardiac injury and apoptosis, and the other is increased ventricular wall thickness. Dilated cardiomyopathy is irreversible and usually leads to heart failure (6). Research has pointed out that hypertensive cardiomyopathy and dilated cardiomyopathy are the most common causes of heart failure (7). One of the main therapeutic goals of dilated cardiomyopathy is to prevent the occurrence of heart failure (8). For dilated cardiomyopathy with end-stage heart failure, heart transplantation is currently the only feasible treatment method, and new treatment methods and pathophysiological mechanisms need to be further explored.
Dilated cardiomyopathy has complex genetic mutations. A variety of gene mutations and abnormal expressions may lead to the occurrence and progression of the disease. With the upgrading of programming languages and the improvement of biological databases, bioinformatics analysis has become an increasingly rich means of clinical disease research. Conducting analysis at the nucleic acid and protein levels to explore the structural and functional information of biological macromolecules provides us with more relevant pathway annotation information on the role of differential genes in disease progression. The MYH7 and TNNT2 genes are the most common gene mutations in dilated cardiomyopathy, accounting for 20–35% and 7–30% of dilated cardiomyopathy patients, respectively (9-12). In addition, many other genes (such as TNNI3, LMNA, ACTC1, etc.) may also cause dilated cardiomyopathy (10,13). Currently, the mechanisms of the occurrence and progression of dilated cardiomyopathy are still unclear and further exploration is needed.
This study aimed to further explore the differentially expressed genes (DEGs) and disease-related signaling pathways in dilated cardiomyopathy using bioinformatics analysis methods, using transcriptome sequencing data sets of myocardial samples from patients with dilated cardiomyopathy. Screen key pathogenic genes through machine learning algorithms and explore the correlation between key genes and immune microenvironment, in order to predict relevant genes that may play an important role in the progression of dilated cardiomyopathy at the molecular level. We present this article in accordance with the STREGA reporting checklist (available at https://jtd.amegroups.com/article/view/10.21037/jtd-23-1086/rc).
Methods
Data download
The transcriptome sequencing data sets of heart tissues from patients with dilated cardiomyopathy (GSE29819 and GSE21610) were downloaded from the Gene Expression Omnibus (GEO) database. The corresponding platform file is GPL570. GSE29819 contains 26 samples of diseased heart tissues and 12 samples of normal heart tissues. The GSE21610 chip dataset includes 21 myocardial tissue samples of dilated cardiomyopathy with heart failure before and after ventricular assist device support treatment, and 8 samples of normal heart tissues. Our inclusion criteria include selecting myocardial tissue samples from all dilated cardiomyopathy patients who have only received standard anti heart failure medication treatment without special treatment or treatment, as well as samples from all normal cardiac function patients. The 2 data sets were merged into 1 data matrix, and batch correction was performed. The normal tissues were set as the Control group, and the diseased tissues were set as the treat group. The study was conducted in accordance with the Declaration of Helsinki (as revised in 2013).
Differential gene expression
R software (R Foundation for statistical Computing, Vienna, Austria) and the limma package were used to screen for DEGs between the control and treat groups. The screening criteria were fold change (FC) >2 and false discovery rate (FDR) <0.05.
DEG enrichment analysis
The Gene Ontology (GO) functional enrichment analysis and Kyoto Encyclopedia of Genes and Genomes (KEGG) pathway enrichment analysis were performed using the online database (https://david.ncifcrf.gov/) with background of Homo sapiens. The enriched functions and pathways of the DEGs were screened with a q-value <0.05 threshold.
Key gene screening
Key genes were screened using both least absolute shrinkage and selection operator (LASSO) regression analysis and random forest tree algorithms. LASSO regression analysis removes redundant gene information by constructing a penalty function. The lambda value and gene set that represented the overall features were determined when the binomial deviance value was minimum. In the random forest tree algorithm, the number of trees was determined when the cross-validation error was minimum and genes with a mean decrease Gini value greater than 2 were retained. The intersection of the gene sets obtained from the 2 algorithms was considered to contain the key genes.
Immune cell subtype infiltration content calculation
The immune cell subtype infiltration content in heart tissues from patients with dilated cardiomyopathy was calculated using single-sample gene set enrichment analysis (ssGSEA). Firstly, rank normalization is performed on the gene expression values of a given sample, and then the enrichment score is calculated using an empirical cumulative distribution function. The enrichment score generated by ssGSEA in a gene set is related to the phenotypic differences in the sample set in a dataset. In this way, ssGSEA converts the gene expression profile into a gene set enrichment profile. Through the gene set related to immune cell markers, we can calculate the immune cell infiltration score.
Diagnostic performance evaluation
The diagnostic performance was assessed by the receiver operating characteristic (ROC) curve. The area under the curve (AUC) represented the diagnostic performance.
Statistical analysis
This study used R software (V3.5.1) for statistical analysis. Group differences were compared, and correlation analysis was performed with a 2-tailed P<0.05 indicating statistical significance.
Results
DEG screening
Compared to normal heart tissue samples (control group), the heart tissue samples of dilated cardiomyopathy with congestive heart failure (treat group) showed differential expression of 213 genes, including 101 upregulated genes and 102 downregulated genes (Figure 1).
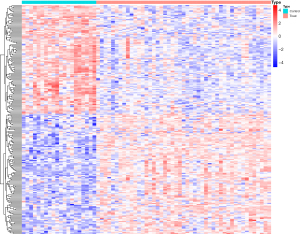
DEG enrichment analysis
The DAVID online database was used to perform enrichment analysis of the commonly DEGs obtained, using a background of Homo sapiens. Enrichment information was obtained for GO. The biological processes enriched by the DEGs included granulocyte chemotaxis, neutrophil chemotaxis, leukocyte chemotaxis, granulocyte migration, and myeloid leukocyte migration. In terms of cell composition, the DEGs were mainly enriched in collagen-containing extracellular matrix, tertiary granule, secretory granule lumen, cytoplasmic vesicle lumen, and vesicle lumen. In terms of molecular function, the DEGs were primarily associated with cytokine activity, receptor ligand activity, signaling receptor activator activity, glycosaminoglycan binding, and chemokine activity (Figure 2). In the KEGG signaling pathway enrichment analysis, the DEGs were enriched in cytokine-cytokine receptor interaction, the interleukin 17 (IL-17) signaling pathway, viral protein interaction with cytokine and cytokine receptor, the tumor necrosis factor (TNF) signaling pathway, FoxO signaling pathway, Hippo signaling pathway, chemokine signaling pathway, lipid and atherosclerosis, and Toll-like receptor signaling pathway (Figure 3).
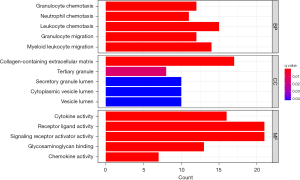
Identification of key genes
In this study, based on the messenger RNA (mRNA) levels of DEGs, the key genes were screened using LASSO regression analysis and random forest tree algorithm. The LASSO regression analysis showed that when lg(lambda) =−4.46 and the binomial deviance value was minimum, a total of 21 key genes were screened (Figure 4). The random forest tree algorithm showed that when the number of trees was 200, the cross-validation error was minimum (Figure 5). Under this condition, there were 9 genes with mean decrease Gini values greater than 2 (Figure 6). The gene sets obtained from the 2 algorithms had 2 overlapping genes, namely, CCL5 and CTGF.
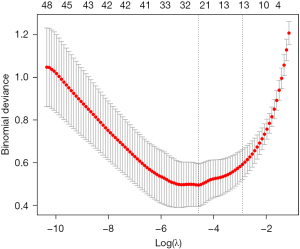
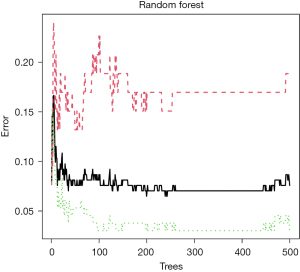
Assessment of diagnostic performance of key genes
The expression of CCL5 and CTGF was shown to be upregulated in the treat group compared to the control group (Figure 7A,7B). The ROC curve showed that the AUCs for CCL5 and CTGF were 0.821 and 0.902, respectively (Figure 7C,7D, P<0.05).
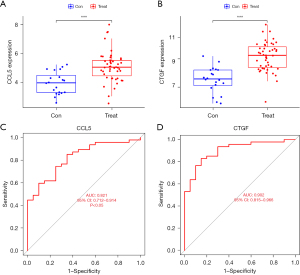
Differentiation in immune cell subtypes
Compared to the control group samples, activated CD8 T cells, effector memory CD4 T cells, central memory CD4 T cells, central memory CD8 T cells, and effector memory CD8 T cells were highly expressed in the Treat group samples. Meanwhile, activated dendritic cells (DCs), CD56dim natural killer (NK) cells, eosinophils, gamma delta T cells, immature DCs, myeloid-derived suppressor cells (MDSCs), macrophages, mast cells, NK T cells, plasmacytoid DCs, and Regulatory T helper type 1 Cells were lowly expressed in the treat group samples compared to the control group samples (Figure 8).
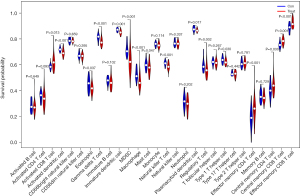
Correlation between key genes and immune cell subtypes
In the treat group samples, CCL5 was positively correlated with the infiltration levels of many immune cell subtypes (Figure 9).
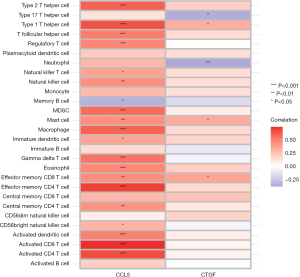
Discussion
Over the past several decades, using genomic analysis data from patients, researchers have discovered several genes that play a crucial role in heart disease, many of which regulate or participate in the regulation of the synchronous contractions of the heart muscle. These genes, by regulating the mechanism of heart contractions, affect the synchronous contractions of the heart muscle, ultimately leading to heart failure. Some gene expression abnormalities may also affect the development of heart muscle, mediate cardiac remodeling, and cause abnormal cardiac electrophysiology, leading to a decline in cardiac function. Key pathogenic genes can explain the specific pathological changes of the disease to some extent. Abnormal gene expression may lead to dilated cardiomyopathy and ultimately heart failure. Xu et al. (14) reported that genes such as CYP2J2, ETNPPL, and FGF1 lead to the occurrence of dilated cardiomyopathy by affecting fatty acid metabolism. Zheng et al. (15) proposed that the IL-17 signaling pathway may be an important pathogenic mechanism for dilated cardiomyopathy. Li et al. (16) suggested that the downregulation of FGF1 expression led to pathological cardiac remodeling. Commonly found key pathogenic genes for heart failure include mutated cardiac actin, anticoagulant plasminogen activator inhibitor-1, alkaline phosphatase A2, threonine kinase 2, and transcription factor 4 (17-19). These genes play crucial roles in the development and repair of heart muscle tissue, and their hereditary mutations may cause functional disorders of heart muscle cells and eventually lead to heart failure. Additionally, pathways associated with cardiac disturbance such as extracellular regulated kinase (ERK), janus kinase (JAK)- signal transducer and activator of transcription (STAT), phosphatidylinositol-3-kinase/ protein kinase B (PI3K/Akt), and P38-mitogen-activated protein kinase (MAPK) may also play an important role in the pathogenesis of heart failure (20-22). The abnormal activities of these pathways may increase cardiac cell death, thus leading to heart failure. But we need to note that heart failure is an inevitable outcome of the progression of all cardiomyopathy, a description of the state of cardiac function, and not a strict pathological diagnosis. Dilated cardiomyopathy with concomitant heart failure belongs to the late stage of the disease. Comparing with normal myocardial samples can help identify key genes involved in disease occurrence and progression. Su et al. identified carbonic anhydrase 2 and 3 as risk biomarkers for dilated cardiomyopathy related heart failure in heart tissue samples of patients with concomitant heart failure and asymptomatic dilated cardiomyopathy (23).
In this study, the results of transcriptome sequencing of normal heart tissue and heart tissue with dilated cardiomyopathy were compared, and DEGs were obtained. These DEGs were significantly enriched in pathways related to inflammation response, activation of chemokines, or migration of inflammatory cells. This indicates that inflammation is an important pathogenic mechanism in dilated cardiomyopathy. This is consistent with some previous results. Liang et al. (24) reported that dilated cardiomyopathy is related to the occurrence of immune response. In dilated cardiomyopathy, the transcription factor ETS1 regulates the expression of inflammation-related genes. Sun et al. (25) suggested that inhibiting the activation of inflammatory phagocytes could inhibit heart remodeling and improve dilated cardiomyopathy. Harding et al. (26) pointed out that although clinical trials of immune suppression therapy have not achieved the expected results in dilated cardiomyopathy, the evidence of abnormal immune response in dilated cardiomyopathy is sufficient. They also highlighted that in subsequent studies, further subtyping of immune cell subtypes is needed (26). Yang et al. suggested that macrophage infiltration may participate in myocardial remodeling and ultimately lead to dilated cardiomyopathy (27). In our study, the infiltration of immune cell subtypes in normal and diseased samples was displayed. We found that CD8+ T cells and CD4+ T cells were enriched in diseased tissues. This may provide reference for the clustering and subtyping of immune cell subtypes.
Through the use of LASSO regression analysis and the random forest tree algorithm, 2 key pathogenic genes, CCL5 and CTGF, were identified. Both CCL5 and CTGF possess good diagnostic efficacy for dilated cardiomyopathy. Our findings also revealed that CCL5 is positively correlated with the infiltration of various immune cell subtypes, which may be one of the driving factors for immune cell enrichment. Lu et al. (28) found that CCL5 is associated with the development of dilated cardiomyopathy. Zhu et al. (29) reported that CCL2, CCL5, and TLR2 can serve as characteristic biological markers for dilated cardiomyopathy. CTGF has also been confirmed to be related to dilated cardiomyopathy in previous studies. Tan et al. (30) argued that downregulation of CTGF can inhibit myocardial fibrosis and improve dilated cardiomyopathy. Additionally, some studies have indicated that CTGF can act as a marker of myocardial fibrosis in dilated cardiomyopathy, with its serum level declining as the ejection fraction decreases (31).
In this study, through the RNA sequencing data sets of myocardial samples from patients with dilated cardiomyopathy, bioinformatics analysis was used to show the DEGs of dilated cardiomyopathy and disease-related signaling pathways. The key pathogenic genes were screened by machine learning algorithm, the correlation between which and immune microenvironment was explored. However, there are still some shortcomings in this study. Firstly, this study lacks in vivo and in vitro experimental validation, and further experiments are needed to confirm the conclusions we have obtained; Secondly, this study only compared normal myocardial samples with dilated cardiomyopathy samples with concomitant heart failure, and did not set up a set of asymptomatic dilated cardiomyopathy samples as groups at different stages of disease development. Finally, there is insufficient depth in exploring gene function.
Conclusions
CCL5 and CTGF are critical pathogenic genes in dilated cardiomyopathy, and they possess good diagnostic efficacy. CCL5 may be related to immune cell enrichment and CTGF may be related to myocardial fibrosis.
Acknowledgments
Funding: This study was supported by Zhejiang Medical and Health Science and Technology Plan Project (No. 2022518604) and Hangzhou Medical Key Disciplines (Nos. OO20200265, OO20200121).
Footnote
Reporting Checklist: The authors have completed the STREGA reporting checklist. Available at https://jtd.amegroups.com/article/view/10.21037/jtd-23-1086/rc
Peer Review File: Available at https://jtd.amegroups.com/article/view/10.21037/jtd-23-1086/prf
Conflicts of Interest: All authors have completed the ICMJE uniform disclosure form (available at https://jtd.amegroups.com/article/view/10.21037/jtd-23-1086/coif). The authors have no conflicts of interest to declare.
Ethical Statement: The authors are accountable for all aspects of the work in ensuring that questions related to the accuracy or integrity of any part of the work are appropriately investigated and resolved. The study was conducted in accordance with the Declaration of Helsinki (as revised in 2013).
Open Access Statement: This is an Open Access article distributed in accordance with the Creative Commons Attribution-NonCommercial-NoDerivs 4.0 International License (CC BY-NC-ND 4.0), which permits the non-commercial replication and distribution of the article with the strict proviso that no changes or edits are made and the original work is properly cited (including links to both the formal publication through the relevant DOI and the license). See: https://creativecommons.org/licenses/by-nc-nd/4.0/.
References
- Orphanou N, Papatheodorou E, Anastasakis A. Dilated cardiomyopathy in the era of precision medicine: latest concepts and developments. Heart Fail Rev 2022;27:1173-91. [Crossref] [PubMed]
- McGurk KA, Halliday BP. Dilated cardiomyopathy - details make the difference. Eur J Heart Fail 2022;24:1197-9. [Crossref] [PubMed]
- Huggins GS, Kinnamon DD, Haas GJ, et al. Prevalence and Cumulative Risk of Familial Idiopathic Dilated Cardiomyopathy. JAMA 2022;327:454-63. [Crossref] [PubMed]
- Tayal U, Verdonschot JAJ, Hazebroek MR, et al. Precision Phenotyping of Dilated Cardiomyopathy Using Multidimensional Data. J Am Coll Cardiol 2022;79:2219-32. [Crossref] [PubMed]
- Aragam KG. Identifying Dilated Cardiomyopathy Through Family-Based Screening. JAMA 2022;327:430-1. [Crossref] [PubMed]
- Giri P, Mukhopadhyay A, Gupta M, et al. Dilated cardiomyopathy: a new insight into the rare but common cause of heart failure. Heart Fail Rev 2022;27:431-54. [Crossref] [PubMed]
- Dzudie A, Barche B, Nkoke C, et al. Survival rate and predictors of 36-month mortality in patients with heart failure in Sub Saharan Africa: insights from the Douala Heart Failure Registry (Do-HF). Cardiovasc Diagn Ther 2022;12:577-88. [Crossref] [PubMed]
- Schultheiss HP, Fairweather D, Caforio ALP, et al. Dilated cardiomyopathy. Nat Rev Dis Primers 2019;5:32. [Crossref] [PubMed]
- Njoroge JN, Mangena JC, Aribeana C, et al. Emerging Genotype-Phenotype Associations in Dilated Cardiomyopathy. Curr Cardiol Rep 2022;24:1077-84. [Crossref] [PubMed]
- Hinson JT. Molecular genetic mechanisms of dilated cardiomyopathy. Curr Opin Genet Dev 2022;76:101959. [Crossref] [PubMed]
- de Frutos F, Ochoa JP, Navarro-Peñalver M, et al. Natural History of MYH7-Related Dilated Cardiomyopathy. J Am Coll Cardiol 2022;80:1447-61. [Crossref] [PubMed]
- Jordan E, Peterson L, Ai T, et al. Evidence-Based Assessment of Genes in Dilated Cardiomyopathy. Circulation 2021;144:7-19. [Crossref] [PubMed]
- Mazzarotto F, Tayal U, Buchan RJ, et al. Reevaluating the Genetic Contribution of Monogenic Dilated Cardiomyopathy. Circulation 2020;141:387-98. [Crossref] [PubMed]
- Xu M, Guo YY, Li D, et al. Screening of Lipid Metabolism-Related Gene Diagnostic Signature for Patients With Dilated Cardiomyopathy. Front Cardiovasc Med 2022;9:853468. [Crossref] [PubMed]
- Zheng Y, Liu Z, Yang X, et al. Exploring Key Genes to Construct a Diagnosis Model of Dilated Cardiomyopathy. Front Cardiovasc Med 2022;9:865096. [Crossref] [PubMed]
- Li G, Shao Y, Guo HC, et al. MicroRNA-27b-3p down-regulates FGF1 and aggravates pathological cardiac remodelling. Cardiovasc Res 2022;118:2139-51. [Crossref] [PubMed]
- Gomes CPC, Schroen B, Kuster GM, et al. Regulatory RNAs in Heart Failure. Circulation 2020;141:313-28. [Crossref] [PubMed]
- Rosik J, Szostak B, Machaj F, et al. Potential targets of gene therapy in the treatment of heart failure. Expert Opin Ther Targets 2018;22:811-6. [Crossref] [PubMed]
- Howie-Esquivel J, White M. Biomarkers in acute cardiovascular disease. J Cardiovasc Nurs 2008;23:124-31. [Crossref] [PubMed]
- Meng H, Du Z, Lu W, et al. Baoyuan decoction (BYD) attenuates cardiac hypertrophy through ANKRD1-ERK/GATA4 pathway in heart failure after acute myocardial infarction. Phytomedicine 2021;89:153617. [Crossref] [PubMed]
- Shen Y, Zhang W, Lee L, et al. Down-regulated microRNA-195-5p and up-regulated CXCR4 attenuates the heart function injury of heart failure mice via inactivating JAK/STAT pathway. Int Immunopharmacol 2020;82:106225. Retraction in: Int Immunopharmacol 2023;117:109868. [Crossref] [PubMed]
- Qin W, Cao L, Massey IY. Role of PI3K/Akt signaling pathway in cardiac fibrosis. Mol Cell Biochem 2021;476:4045-59. [Crossref] [PubMed]
- Su H, Hu K, Liu Z, et al. Carbonic anhydrase 2 and 3 as risk biomarkers for dilated cardiomyopathy associated heart failure. Ann Palliat Med. 2021;10:12554-65. [Crossref] [PubMed]
- Liang L, Sun J, Teng T, et al. Expression Profile of Inflammation Response Genes and Potential Regulatory Mechanisms in Dilated Cardiomyopathy. Oxid Med Cell Longev 2022;2022:1051652. [Crossref] [PubMed]
- Sun X, Duan J, Gong C, et al. Colchicine Ameliorates Dilated Cardiomyopathy Via SIRT2-Mediated Suppression of NLRP3 Inflammasome Activation. J Am Heart Assoc 2022;11:e025266. [Crossref] [PubMed]
- Harding D, Chong MHA, Lahoti N, et al. Dilated cardiomyopathy and chronic cardiac inflammation: Pathogenesis, diagnosis and therapy. J Intern Med 2023;293:23-47. [Crossref] [PubMed]
- Yang Y, Liu P, Teng R, et al. Integrative bioinformatics analysis of potential therapeutic targets and immune infiltration characteristics in dilated cardiomyopathy. Ann Transl Med 2022;10:348. [Crossref] [PubMed]
- Lu Y, Wu Q, Liao J, et al. Identification of the distinctive role of DPT in dilated cardiomyopathy: a study based on bulk and single-cell transcriptomic analysis. Ann Transl Med 2021;9:1401. [Crossref] [PubMed]
- Zhu T, Wang M, Quan J, et al. Identification and Verification of Feature Biomarkers Associated With Immune Cells in Dilated Cardiomyopathy by Bioinformatics Analysis. Front Genet 2022;13:874544. [Crossref] [PubMed]
- Tan CY, Wong JX, Chan PS, et al. Yin Yang 1 Suppresses Dilated Cardiomyopathy and Cardiac Fibrosis Through Regulation of Bmp7 and Ctgf. Circ Res 2019;125:834-46. [Crossref] [PubMed]
- Rubiś P, Holcman K, Dziewięcka E, et al. Relationships between circulating galectin-3, extracellular matrix fibrosis and outcomes in dilated cardiomyopathy. Adv Clin Exp Med 2021;30:245-53. [Crossref] [PubMed]