Dynamic changes in macrophage subtypes during lung cancer progression and metastasis at single-cell resolution
Highlight box
Key findings
• We found a chemotaxis of macrophages and a monocyte-macrophage differentiation process during lung cancer progression, and identified 9 macrophage-specific interactions that were enriched during this progression process.
What is known and what is new?
• Macrophages play an important role during cancer progression.
• Detailed changes of macrophage subtypes and polarization status were revealed among different stages of lung cancer progression.
What is the implication, and what should change now?
• Our results shed light on exploring the progression mechanism of lung cancer from the perspective of the immune microenvironment, as well as providing potential therapeutic targets for future lung adenocarcinoma treatments.
Introduction
Lung cancer is a leading cause of cancer deaths. In 2020, it caused approximately 1.8 million deaths (18% of the total cancer deaths) worldwide (1,2). Although current treatments for lung cancer include surgery, radiotherapy, chemotherapy, and targeted therapy, the overall prognosis is relatively poor due to tumor progression and metastasis (3,4). Extensive genomic screening has identified many oncogenes, such as epidermal growth factor receptor (EGFR), B-Raf (BRAF), and EMAP like 4-ALK (EML4-ALK) fusions, that could be used in targeted therapies (5,6); however, only 10–15% of patients benefit from these therapies, while the majority of patients do not achieve satisfactory effects due to the emergence of resistance mechanisms (6-8). Hence, further exploration of the tumor landscape is critical in identifying new therapeutic targets.
Over the past decade, the impact of changes in the tumor microenvironment (TME) on tumor progression and metastasis has been increasingly recognized, as summarized by Altorki et al. (9). Drugs that target certain TME components, such as vascular endothelial growth factor (VEGF) and immune checkpoint proteins, have been clinically approved (1). Macrophages (Macs) represent one important and abundant type of innate immune component in the lung TME, and these cells are highly plastic and exhibit a variety of phenotypes (10), including the M1 state (proinflammatory, classically activated, antitumor activity) and M2 state (anti-inflammatory, alternatively activated, protumor activity). Currently, the prognostic relevance of Macs in lung cancer remains unclear due to the heterogeneity of these phenotypes (11).
A cellular Mac transcriptome atlas is critical for studying the role of Macs in lung cancer progression and metastasis. Advances in single-cell RNA sequencing (scRNAseq) technology have provided us with a precise method for examining Macs. In this study, we systematically compared the changes in Mac subtypes across different stages of lung cancer progression and metastasis [paracancer normal lung samples (nLung), early-stage lung cancer samples (tLung), advanced-stage lung cancer samples (tL/B), normal lymph node samples (nLN), metastatic lymph node samples (mLN), and metastatic brain samples (mBrain)] and found a series of relevant features. Moreover, through ligand-receptor (LR) analysis, we identified 9 interactions related to lung cancer progression and metastasis that could be used as potential therapeutic targets. We present this article in accordance with the MDAR reporting checklist (available at https://jtd.amegroups.com/article/view/10.21037/jtd-23-1012/rc).
Methods
scRNAseq data preparation
scRNAseq matrix files were downloaded from the Gene Expression Omnibus (GEO) online database (www.ncbi.nlm.nih.gov/geo) under accession number GSE131907 (12). RDS files of 10 nLung (“LUNG_N01”, “LUNG_N06”, “LUNG_N08”, “LUNG_N09”, “LUNG_N19”, “LUNG_N20”, “LUNG_N28”, “LUNG_N30”, “LUNG_N31”, “LUNG_N34”) were reconstructed separately using CreateSeuratObject() function of R Seurat package V4 (13), which was followed by an integration step using FindIntegrationAnchors() and IntegrateData() functions. The RDS file of tLung was integrated from 11 lung tumor samples (“LUNG_T06”, “LUNG_T08”, “LUNG_T09”, “LUNG_T18”, “LUNG_T19”, “LUNG_T20”, “LUNG_T25”, “LUNG_T28”, “LUNG_T30”, “LUNG_T31”, “LUNG_T34”). The RDS file of tL/B was integrated from 4 lung tumor samples (“BRONCHO_58”, “EBUS_06”, “EBUS_28”, “EBUS_49”). The RDS file of nLN was integrated from 10 lymph node samples (“LN_01”, “LN_02”, “LN_03”, “LN_04”, “LN_05”, “LN_06”, “LN_07”, “LN_08”, “LN_11”, “LN_12”). The RDS file of mLN was integrated from 7 lymph node samples (“BRONCHO_11”, “EBUS_10”, “EBUS_12”, “EBUS_13”, “EBUS_15”, “EBUS_19”, “EBUS_51”). The RDS file of mBrain was integrated from 10 brain samples (“NS_02”, “NS_03”, “NS_04”, “NS_06”, “NS_07”, “NS_12”, “NS_13”, “NS_16”, “NS_17”, “NS_19”). The study was conducted in accordance with the Declaration of Helsinki (as revised in 2013).
scRNAseq data analysis
Integrated RDS files (nLung, tLung, tL/B, nLN, mLN, and mBrain) were further scaled using the ScaleData() function, followed by a RunPCA() step (npcs =30). Different subgroups were clustered using the FindNeighbors() and FindClusters() functions with dims set to 30 and resolution set to 1. All subgroups were visualized using the Uniform Manifold Approximation and Projection (UMAP) method through the RunUMAP() function. All the marker genes were identified using R Seurat FindAllMarkers() function with “test.use” set as “wilcox”, logfc.threshold set as “0.25” and min.pct set as “0.3”.
PseudoTime trajectory analysis
PseudoTime analysis was performed using the R monocle package (14). All myeloid components, including alveolar macrophages (aMacs), Macs, monocytes (Monos), and cycling macrophages (cMacs), from the 6 sample types were used as inputs. A Monocle file was generated using the newCellDataSet() function with the lowerDetectionLimit set to 0.5. The top 1,000 differentially expressed genes calculated by differetialGeneTest() were used in pseudoTime analysis. A heatmap representing gene expression patterns among the 3 states was illustrated using the plot_genes_branched_heatmap() function.
Gene Ontology (GO) enrichment analysis
GO enrichment analysis was performed using the R clusterProfiler package (15). Input genes from each group were first transformed to “ENTREZID” using the bitr() function, followed by the enrichGO() function with “ont” set to “BP”, “pAdjustMethod” set to “BH”, “pvalueCutoff” set to “0.05”, and “qvalueCutoff” set to “0.2”.
LR interaction analysis
Cytokines were determined using annotations from The Human Protein Atlas (THPA, www.proteinatlas.org). Their corresponding membrane receptors were retrieved from the BioGrid (16) online database (www.thebiogrid.org). Interaction intensity was calculated by multiplying the average expression values of ligands by the average expression values of receptors, and the intensity heatmap was visualized using the R ggplot2 package.
Statistical analysis
A Student t-test (unpaired) was performed among the comparisons in this study, and a P=0.05 was used as significance cutoff. For multiple comparisons, a one-way analysis of variance (ANOVA) analysis was performed, with “Bonferroni” corrections applied. All the statistical analysis was performed using R (version 3.6.3).
Results
Reconstruction of a single-cell atlas of lung cancer
To investigate the changes in Mac subtypes in the lung cancer TME, we retrieved scRNAseq data from the GEO online database under accession number GSE131907 (12). To avoid batch effects due to different sample origins, we performed separate scRNAseq integration on samples from different origins, and the results are summarized in Figure S1.
The following markers were used in the identification process of different subgroups: EPCAM/KRT19/KRT18/CDH for epithelial cells; DCN/THY1/COL1A1/COL1A2 for fibroblasts; PECAM1/CLDN5/FLT1/RAMP2 for endothelial cells; CD3D/CD3E/CD3G/TRAC for T lymphocytes; NKG7/GLNY/NCAM1/KLRD1 for NK cells; CD79A/IGHM/IGHG3/IGHA2 for B lymphocytes; LYZ/MARCO/CD68/FCGR3A for myeloid cells; KIT/MS4A2/GATA2 for mast cells; OLIG1/OLIG2/MOG/CLDN11 for oligodendrocytes.
An atlas of 38,367 cells was constructed for 10 paratumorous nLung (Figure S1A) and further clustered into 7 groups using markers from Kim et al. (12): 14,631 myeloid cells (38.13%), 10,330 T and natural killer cells (T/NK, 26.14%), 3,304 epithelial cells (8.61%), 1,429 fibroblasts (3.72%), 1,122 endothelial cells (2.92%), 997 mast cells (2.60%), 545 B cells (1.42%) and 6,009 undefined cells [not applied (NA), 15.66%].
An atlas of 45,149 cells was constructed for 11 tLung (Figure S1B) and further clustered into 7 groups: 13,941 T/NK cells (28.88%), 8,794 myeloid cells (19.48), 7,270 epithelial cells (16.10%), 5,312 B cells (11.77%), 1,809 mast cells (4.01%), 1,654 fibroblasts (3.66%), 627 endothelial cells (13.89%) and 5,742 undefined cells (12.72%).
An atlas of 12,073 cells was constructed for 4 tL/B (Figure S1C) and further clustered into 6 groups: 6,582 epithelial cells (54.52%), 2,627 T/NK cells (21.76%), 1,329 myeloid cells (11.00%), 469 B cells (3.88%), 85 fibroblasts (0.70%), 18 endothelial cells (0.15%) and 963 undefined cells (7.98%).
An atlas of 37,466 cells was constructed for 10 nLN (Figure S1D) and further clustered into 3 groups: 19,478 T/NK cells (52.02%), 10,584 B cells (28.26%), 1,288 myeloid cells (3.44%) and 6,096 undefined cells (16.28%).
An atlas of 21,479 cells was constructed for 7 mLNs (Figure S1E) and further clustered into 6 groups: 6,062 B cells (28.22%), 5,442 myeloid cells (25.34%), 5,069 T/NK cells (23.60%), 3,053 epithelial cells (14.21%), 26 fibroblasts (0.12%), 8 endothelial cells (0.03%) and 1,819 undefined cells (8.47%).
An atlas of 29,060 cells was constructed for 10 mBrain (Figure S1F) and further clustered into 7 groups: 15,463 epithelial cells (53.21%), 5,657 myeloid cells (19.47%), 2,683 T/NK cells (9.23%), 1,311 B cells (4.51%), 508 mast cells (1.75%), 444 fibroblasts (1.53%), 159 endothelial cells (0.55%) and 2,835 undefined cells (9.76%).
To sum up, different cell-type patterns were examined in samples from different origins, such as increased numbers of epithelial cells during the progression of lung cancer (nLung < tLung < tL/B samples), suggesting the existence of more cancer cells as the tumor progresses. More epithelial cells were examined in mLN samples than nLN samples, which is expected for metastatic lymph node samples. Less myeloid cells were examined during the progression of lung cancer (nLung < tLung < tL/B samples), and more myeloid cells were examined in mLN samples than nLN samples, suggesting the recruitment of myeloid cells might contribute to the metastasis progress.
Identification of myeloid components
We further clustered myeloid cells from each atlas into 5 different subgroups: Macs, aMacs, cMacs, Monos, and dendritic cells (DCs) (Figure 1A) based on the cell types described in Sorin et al. (17) and Travaglini et al. (18). The relative expression levels of representative markers used to identify each subgroup are illustrated in Figure 1B. Specifically, Macs were identified as CD14+/HLA-DRA+/CD86+/CD163+/MRC1+; aMacs were identified as MARCO+/FABP4+/MCEMP1+; cMacs were identified as MKI67+/STMN1+/TOP2A+; Monos were identified as CD14+/MRC1−/CD163−; and DCs were identified as CD1C+/PTCRA+/CD1A+/CCR7+/LAMP3+.
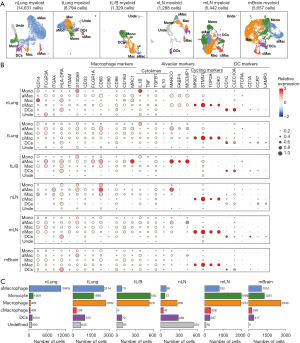
Among myeloid cells in nLung samples, there were 10,405 aMacs (71.12%), 1,369 Monos (9.36%), 499 Macs (3.41%), 408 cMacs (2.79%), 1,050 DCs (7.18%), and 900 undefined cells (6.15%). Among myeloid cells from tLung samples, there were 2,514 aMacs (28.59%), 1,690 Monos (19.22%), 2,682 Macs (30.50%), 288 cMacs (3.24%), 975 DCs (11.09%), and 645 unidentified cells (7.33%). Among myeloid cells from tL/B samples, there were 79 aMacs (0.90%), 550 Monos (6.25%), 528 Macs (6.00%), 79 DCs (0.90%), and 93 unidentified cells (1.06%). Among myeloid cells from nLN samples, there were 89 aMacs (6.91%), 67 Monos (5.20%), 268 Macs (20.81%), 41 cMacs (3.18%), 288 DCs (22.36%), and 535 unidentified cells (41.54%). Among myeloid cells from mLN samples, there were 792 aMacs (14.55%), 1,996 Monos (36.68%), 1,972 Macs (36.24%), 326 cMacs (5.99%), 287 DCs (5.27%), and 69 unidentified cells (1.27%). Among myeloid cells from mBrain samples, there were 1,051 aMacs (18.58%), 1,287 Monos (22.75%), 2,644 Macs (46.74%), 258 cMacs (4.56%), and 417 DCs (7.37%). Detailed numbers of each myeloid component in the different samples are shown in Figure 1C.
Changes in myeloid components among different lung adenocarcinoma (LUAD) samples
To explore the changes in myeloid components in different LUAD samples, we first compared their ratios, and the results are shown in Figure 2A. Among these components, nLung samples had the highest ratio of aMacs; mBrain samples had the highest ratio of Macs; mLN and tL/B samples had higher ratios of Monos compared to these in other samples; nLN samples had the highest ratio of DCs.
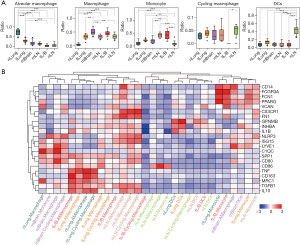
To explore the functional difference among these myeloid subgroups, we applied a set of Mac-specific markers from Cheng et al. (19) and compared their expressional status across different subgroups. The results are shown in Figure 2B. Subgroups from different origins were mainly grouped into 7 clusters: cluster1 contained mainly subgroups from mBrain samples (nLung.Macrophage, mBrain.Monocyte, mBrain.Macrophage, mBrain.Cycling.Macrophage) featured with higher expression of NLRP3/ISG15/LYVE1/C1QC/SPP1/CD80; cluster2 contained mainly aMac (tL/B.AMacrophage, tLung.AMacrophage, nLung.AMacrophage, nLung.Cycling.Macrophage) featured with higher expression of TNF/CD163/MRC1/TGFB1/IL10; cluster3 contained mainly Macs from tumor samples (tLung.Macrophage, tLung.Cycling.Macrophage, tL/B.Macrophage) featured by higher expression of SPP1/CD80/CD86/MRC1/TGFB1; cluster4 contained mainly Macs from mLN samples (mLN.AMacrophage, mLN.Macrophage, mLN.Cycling.Macrophage) featured by higher expression of CX3CR1/FN1/NLRP3/ISG15/TGFB1/IL10; cluster5 contained mainly Macs from nLN samples (tL/B.Cycling.Macrophage, nLN.Macrophage, nLN.AMacrophage, nLN.Monocyte) featured by higher expression of CD86; cluster6 contained mainly DCs (nLung.DCs, tLung.DCs, nLN.Cycling.Macrophage, mLN.DCs, tL/B.DCs, nLN.DCs) featured by higher expression of GPNMB/INHBA/IL1B; cluster7 contained mainly Monos (nLung.Monocyte, tL/B.Monocyte, mBrain.AMacrophage, mBrain.DCs, tLung.Monocyte, mLN.Monocyte) featured by higher expression of CD14/FCGR3A/FCN1/PPARG/VCAN.
Polarization represents the activation status of Macs, and there are typically two polarization states: the M1 proinflammatory state, which is characterized by the expression of cell surface markers including CD80 (20)/CD86 (21) and cytokines including interleukin 1 beta (IL1B) (22)/tumor necrosis factor (TNF) (23), and the M2 anti-inflammatory state, which is characterized by the expression of cell surface markers including CD163 (24)/MRC1 (25) and cytokines including TGFB1 (26)/IL10 (27). In this study, we compared the expression levels of these marker genes among different myeloid subgroups in different samples, and the results are shown in Figure S2.
Macs in lung tissues were examined, and higher expression levels of the M1 markers CD80/IL1B and lower expression levels of TNF were identified in tL/B samples. Lower expression levels of the M2 marker TGFB1 were found in tLung samples, and higher expression levels of IL10 were found in tLung and tL/B samples. Among aMacs in metastasis tissues, higher expression levels of the M1 markers IL1B and TNF were found in mLN and mBrain samples. Higher expression of the M2 markers CD163 and MRC1 were found in mLN and mBrain samples, and higher expression levels of IL10 were found in mBrain samples.
Among Macs in lung tissues, higher expression levels of the M1 marker CD80 were found in tLung and tL/B samples, and higher expression levels of IL1B and TNF were found in tL/B samples. Lower expression levels of the M2 marker MRC1 were found in tLung and tL/B samples. Among Macs in metastasis tissues, higher expression levels of the M1 markers CD86 and IL1B were found in mLN samples, and higher expression levels of TNF were found in tLung and tL/B samples. Higher expression levels of the M2 markers CD163 and IL10 were found in tLung and tL/B samples, and higher expression levels of MRC1 were found in mBrain samples.
Among Monos in lung tissues, higher expression levels of the M1 markers CD80, CD86, and TNF were found in tLung samples, and higher expression levels of the M2 markers CD163, MRC1, and IL10 were found in tLung samples. Among Monos in metastasis tissues, higher expression levels of the M1 marker CD80 were found in tLung samples, and higher expression levels of IL1B and TNF were found in mLN and mBrain samples. Higher expression levels of the M2 markers CD163, MRC1, and IL10 were found in mLN and mBrain samples, and higher expression levels of TGFB1 were found in mLN samples.
Among cMacs in lung tissues, higher expression levels of the M1 marker CD80 were found in tLung samples. Higher expression levels of the M2 marker IL10 were found in tLung samples. Among cMacs from metastasis tissues, higher expression levels of the M1 markers CD86 and IL1B were found in mLN and mBrain samples, and higher expression levels of TNF were found in mLN samples. Higher expression levels of the M2 markers CD163, MRC1 and IL10 were found in mLN and mBrain samples, and higher expression levels of TGFB1 were found in mLN samples.
IL10 is one type of immunosuppressive cytokines, and higher expression of IL10 was examined in aMacs as tumor progresses (nLung < tLung < tL/B), and in Monos/Macs during the metastasis (mLN > nLN), suggesting a contribution to the generation of a tumor immunosuppressive microenvironment.
Evolutionary relationship between different Mac subtypes
To examine the evolutionary relationship between different Mac subtypes (aMacs, cMacs, Monos, Macs), a pseudotime analysis was performed on cells from different samples, and a clear 3-branch trajectory pattern was found, as shown in Figure 3. Within this trajectory tree, state 1 was considered the root (Figure 3A,3B). Trees representing different subgroup sources and sample sources are shown in Figure 3C,3D.
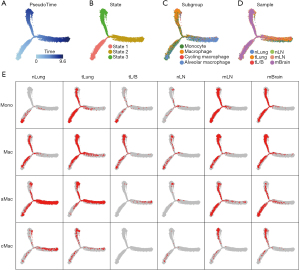
As shown in Figure 3E, most of the Monos resided in the state 1 branch, most of the aMacs resided in the state 2 branch, and most of the Macs resided in the state 3 branch. To screen for featured genes during pseudotime changes, we compared the gene expression profiles of highly variable genes among these 3 states, and the results are shown in Figure 4A. These genes were further divided into 6 groups using “ward.D2” method, and the GO enrichment results are listed in Figure 4B. Group 1 genes were highly expressed in state 2 cells, and these genes were enriched in functions such as “mitotic nuclear division” and “antigen processing and presentation of exogenous peptide antigen”. Group 2 genes were also highly expressed in state 2 cells and these genes were enriched in functions such as “leukocyte migration” and “response to hypoxia”. Group 3 genes were highly expressed in state 3 cells (Macs), and these genes were enriched in “antigen processing and presentation of peptide antigen” and “myeloid cell differentiation”. Group 4 and 5 genes were highly expressed in state 1 cells, and these genes were enriched in functions such as “cytoplasmic translation” and “response to lipopolysaccharide”. Group 6 genes were highly expressed in state 1 cells and state 2 cells, and these genes were enriched in functions such as “regulation of hemopoiesis” and “regulation of myeloid cell differentiation”.
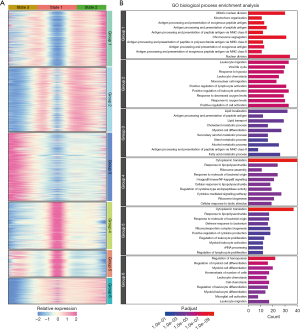
We also performed gene set variation analysis (GSVA) on all these GO biological process (BP) enriched terms to subgroups from all sample origins, and the results are illustrated in Figure 5. “Mitotic nuclear division” term had higher scores in cMac subgroups, suggesting a duplication process in these cells; “antigen processing and presentation” related terms had higher scores in DC and Mac subgroups, suggesting an activation process of T lymphocytes of these cells; certain metabolic processes such as “cholesterol metabolic process”, “secondary alcohol metabolic process”, “sterol metabolic process”, “alcohol metabolic process”, and “fatty acid metabolic process” were activated in aMac subgroups. Expression profiles of genes involved in these metabolic processes are examined and illustrated in Figure S3. We further examined other metabolism related Kyoto Encyclopedia of Genes and Genomes (KEGG) terms across all subgroups, and the results are illustrated in Figure S4. Consistent with GO BP terms, most of the metabolism related KEGG terms had higher scores in aMac subgroups.
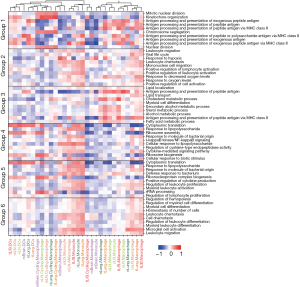
Regulation of myeloid components through LR interactions
There were five cytokines in Group 3 genes: CCL18 (encoding C-C motif chemokine ligand 18), CXCL16 (encoding CXC motif chemokine ligand 16), CXCL5 (encoding C-X-C motif chemokine ligand 5), FAM3B (encoding FAM3 metabolism regulating signaling molecule B), and GRN (encoding granulin precursor). The relative expression levels of these cytokines among different myeloid components from different samples are shown in Figure 6 (left panel). Higher CCL18 expression was found in Macs from tLung samples than in nLung samples and in cMacs from mLN and mBrain samples compared to nLN samples. Higher CXCL16 expression was found in Monos from tLung samples than nLung samples and in cMacs from mLN and mBrain samples compared to nLN samples. Higher CXCL5 expression was found in aMacs from mLN samples than nLN samples. Higher FAM3B expression was found in aMacs from mBrain samples than nLung samples and in cMacs from mBrain samples compared to nLN samples. Higher GRN expression was found in Macs from tLung samples than nLung samples, in Monos from tLung samples compared to nLung samples, and in cMacs from mLN and mBrain samples compared to nLN samples.

The expression levels of receptors corresponding to these ligands were also examined (Figure 6 right panel). Regarding CXCR6 (encoding C-X-C motif chemokine receptor 6, receptor of CXCL16), increased expression was found in Macs from tLung samples and mLN samples, in Monos from tLung samples, mLN samples and mBrain samples. Regarding CXCR2 (encoding C-X-C motif chemokine receptor 2, receptor of CXCL5), increased expression was found in aMacs from mBrain samples, in Macs from tLung samples, mLN samples and mBrain samples, Monos from mLN samples, cMacs from mLN and mBrain samples. Regarding ATP1B3 (encoding ATPase Na+/K+ transport subunit beta 3, receptor of GRN), increased expression was found in Macs from mBrain samples, in Monos from mLN and mBrain samples, and in cMacs from mLN and mBrain samples. Regarding EGFR (encoding EGFR, receptor of GRN), increased expression was found in Macs from tLung and tL/B samples, and in Monos from mLN and mBrain samples. Regarding GPC1 (encoding glypican 1, receptor of GRN), increased expression was found in cMacs from mLN samples, in Macs from tLung samples, tL/B samples, mLN samples and mBrain samples, in Monos from tLung samples, mLN samples and mBrain samples. Regarding NF2 (encoding neurofibromin 2, receptor of GRN), increased expression was found in aMacs from mLN samples. Regarding PKP2 (encoding plakophilin 2, receptor of GRN), increased expression was found in Macs from mLN and mBrain samples, and in Monos from mBrain samples.
The interaction intensity was also calculated and compared among subgroup combinations, as shown in Figure 7. Most of the interactions had higher intensities in tumor (tLung, tL/B) samples and metastasis (mLN and mBrain) samples. Specifically, higher intensities of CCL18-CCR8/CXCL16-CXCR6 were found in Mac subtypes in tLung samples, suggesting that these two interactions might play roles in early-stage lung cancer. Higher intensities of GRN-EGFR were found in Mac subtypes of tL/B and mBrain samples, suggesting that this interaction might a play role in advanced-stage lung cancer. Higher intensities of GRN-PKP2 were found in tLung and tL/B samples, suggesting that this interaction might play a role in lung cancer progression. GRN-GNB2/GRN-ATP1B3 interactions had increased intensities in all tumor and metastasis samples, suggesting that these interactions might be related to lung cancer progression and metastasis.
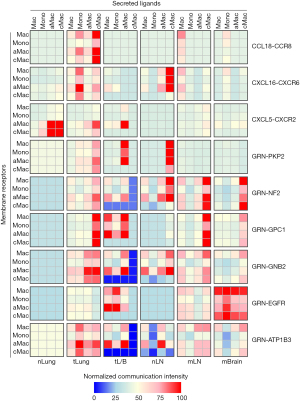
Potential correlation between secreted phosphoprotein 1 (SPP1+) Macs and LUAD progression
The existence of SPP1+ Macs have been found pan-cancer wide, and in this study, we also explored the expression of SPP1 in myeloid subgroups, as shown in Figure 8A. SPP1 had the highest expression values in Macs from tL/B samples, and the expression of SPP1 increased in Macs with the progression of LUAD (tL/B > tLung > nLung). We further screened for co-expressing genes with SPP1 in Macs, and 9 genes (including GAPDH/LDHA/ISG15/LSP1/IFI6/TPI1/MIF/LY6E/ALOX5AP) showed a Pearson correlation score of over 0.4 (Figure 8B). Among these 9 genes, 8 of them (except for ALOX5AP) had increased expressions corresponding to LUAD progression, as shown in Figure 8C.
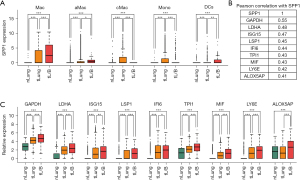
Regarding these overexpressed genes, Chen et al. reported encoding lactate dehydrogenase A (LDHA) could alleviate reactive oxygen species (ROS) and induce M2 Mac polarization (28); Chen et al. (29) found that encoding interferon-stimulated gene 15 (ISG15) could promote immune suppression by inducing a Mac M2-phenotype; Kwon et al. showed that encoding leukocyte-specific protein 1 (LSP1) could affect migration and infiltration of T cells into tumor in mouse (30); Liu et al. (31) reported that interferon alpha inducible protein 6 (IFI6) could promote the progression of esophageal squamous cell carcinoma through regulating ROS; Liu et al. (32) found that overexpression of encoding triosephosphate isomerase 1 (TPI1) could promote LUAD and enhance chemoresistance; encoding macrophage inhibitory factor (MIF) has been found involved in many types of cancers including LUAD, and overexpression of MIF is associated with angiogenesis and tumor metastasis (33). All the above findings confirm the relevance of these genes to tumor progression and deserves more attention in the future research.
Discussion
In this study, we systematically compared changes in myeloid components, especially Mac-related subgroups, during the progression and metastasis of LUAD through scRNAseq analysis. We found that the proportion of aMacs gradually decreased with the progression of lung cancer (Figure 2A), while the expression of M1 marker genes significantly increased in aMacs from tL/B samples (Figure 2B), suggesting the antitumor function of these aMacs, which is consistent with previous findings, as reviewed in Almatroodi et al. (34).
Macs or tumor-associated macrophages (TAMs) also originate from blood Monos and are recruited to tumor sites through a series of chemoattractant signals, such as CCL2 (35). We found increased proportions of Macs and Monos as the tumor progressed (Figure 2A). Interestingly, lower expression of M1 markers such as IL1B/TNF and higher expression of M2 markers such as CD163/MRC1/IL10 were found in Macs and Monos from mBrain samples than those from metastatic lymph node samples, suggesting that an M2 polarization process might contribute to lung cancer brain metastases. Further pseudotime analysis revealed a Mono-Mac differentiation trajectory track, as shown in Figure 3 (state 1 to state 3). Monos were enriched in functions such as “cytokine-mediated signaling pathway” and “response to lipopolysaccharide”, suggesting a chemotaxis process and M1-polarization process during differentiation. Macs were enriched in functions such as “lipid localization” and “lipid transport”. Lipid metabolic reprogramming has been considered a relevant feature during lung cancer progression (36,37), and our results further confirmed this process.
Macs can reshape their TMEs through cytokine secretion (38,39). In this study, we further explored the regulatory relationships among different Mac subtypes and identified 9 cytokine-receptor pairs that Macs could use to regulate the surrounding myeloid cells. Among them, CCL18 could cause M2 polarization of Monos (40), and CCL18 secreted by M2 Macs could further promote the migration and invasion of cancer cells (41). CXCL16 could promote M2-Mac infiltration (42), which might contribute to the increase in Macs during lung cancer progression. Regarding GRN, Nielsen et al. reported that Mac-secreted granulin could promote metastasis in pancreatic cancer (43), and Quaranta et al. further clarified that granulin promoted metastasis in pancreatic cancer by contributing to cytotoxic T-cell exhaustion (44). Our results identified several regulatory interactions among myeloid components through Mac-derived granulin, suggesting that this regulation might also contribute to metastasis progression in lung cancer.
Conclusions
In summary, scRNAseq analysis revealed the characteristics of Mac subtypes during lung cancer progression and metastasis. Moreover, LR analysis identified several interactions that tumor-enriched Macs used to regulate their surrounding Mac subtypes. Our results shed light on the changes in the tumor immune microenvironment of lung cancer and provide potential therapeutic targets for future treatments.
Acknowledgments
Funding: This research was supported by
Footnote
Reporting Checklist: The authors have completed the MDAR reporting checklist. Available at https://jtd.amegroups.com/article/view/10.21037/jtd-23-1012/rc
Peer Review File: Available at https://jtd.amegroups.com/article/view/10.21037/jtd-23-1012/prf
Conflicts of Interest: All authors have completed the ICMJE uniform disclosure form (available at https://jtd.amegroups.com/article/view/10.21037/jtd-23-1012/coif). The authors have no conflicts of interest to declare.
Ethical Statement: The authors are accountable for all aspects of the work in ensuring that questions related to the accuracy or integrity of any part of the work are appropriately investigated and resolved. The study was conducted in accordance with the Declaration of Helsinki (as revised in 2013).
Open Access Statement: This is an Open Access article distributed in accordance with the Creative Commons Attribution-NonCommercial-NoDerivs 4.0 International License (CC BY-NC-ND 4.0), which permits the non-commercial replication and distribution of the article with the strict proviso that no changes or edits are made and the original work is properly cited (including links to both the formal publication through the relevant DOI and the license). See: https://creativecommons.org/licenses/by-nc-nd/4.0/.
References
- Sung H, Ferlay J, Siegel RL, et al. Global Cancer Statistics 2020: GLOBOCAN Estimates of Incidence and Mortality Worldwide for 36 Cancers in 185 Countries. CA Cancer J Clin 2021;71:209-49. [Crossref] [PubMed]
- Hernandez D, Cheng CY, Hernandez-Villafuerte K, et al. Survival and comorbidities in lung cancer patients: Evidence from administrative claims data in Germany. Oncol Res 2022;30:173-85. [Crossref] [PubMed]
- Herbst RS, Morgensztern D, Boshoff C. The biology and management of non-small cell lung cancer. Nature 2018;553:446-54. [Crossref] [PubMed]
- Shi J, Chen Y, Peng C, et al. Advances in Targeted Therapy Against Driver Mutations and Epigenetic Alterations in Non-Small Cell Lung Cancer. Oncologie 2022;24:613-48.
- Chen Z, Fillmore CM, Hammerman PS, et al. Non-small-cell lung cancers: a heterogeneous set of diseases. Nat Rev Cancer 2014;14:535-46. [Crossref] [PubMed]
- Mayekar MK, Bivona TG. Current Landscape of Targeted Therapy in Lung Cancer. Clin Pharmacol Ther 2017;102:757-64. [Crossref] [PubMed]
- Xu L, Li K, Li J, et al. MiR-21/Sonic Hedgehog (SHH)/PI3K/AKT Pathway is Associated with NSCLC of Primary EGFR-TKI Resistance. Oncologie 2022;24:579-90.
- Zhang D, Liu H, Yi Z, et al. Thymidylate synthase confers pemetrexed resistance of non-small cell lung cancer cells by EGFR/PI3K/AKT pathway. Biocell 2021;45:617-25.
- Altorki NK, Markowitz GJ, Gao D, et al. The lung microenvironment: an important regulator of tumour growth and metastasis. Nat Rev Cancer 2019;19:9-31. [Crossref] [PubMed]
- Ginhoux F, Schultze JL, Murray PJ, et al. New insights into the multidimensional concept of macrophage ontogeny, activation and function. Nat Immunol 2016;17:34-40. [Crossref] [PubMed]
- Conway EM, Pikor LA, Kung SH, et al. Macrophages, Inflammation, and Lung Cancer. Am J Respir Crit Care Med 2016;193:116-30. [Crossref] [PubMed]
- Kim N, Kim HK, Lee K, et al. Single-cell RNA sequencing demonstrates the molecular and cellular reprogramming of metastatic lung adenocarcinoma. Nat Commun 2020;11:2285. [Crossref] [PubMed]
- Hao Y, Hao S, Andersen-Nissen E, et al. Integrated analysis of multimodal single-cell data. Cell 2021;184:3573-3587.e29. [Crossref] [PubMed]
- Trapnell C, Cacchiarelli D, Grimsby J, et al. The dynamics and regulators of cell fate decisions are revealed by pseudotemporal ordering of single cells. Nat Biotechnol 2014;32:381-6. [Crossref] [PubMed]
- Wu T, Hu E, Xu S, et al. clusterProfiler 4.0: A universal enrichment tool for interpreting omics data. Innovation (Camb) 2021;2:100141. [Crossref] [PubMed]
- Oughtred R, Stark C, Breitkreutz BJ, et al. The BioGRID interaction database: 2019 update. Nucleic Acids Res 2019;47:D529-41. [Crossref] [PubMed]
- Sorin M, Rezanejad M, Karimi E, et al. Single-cell spatial landscapes of the lung tumour immune microenvironment. Nature 2023;614:548-54. [Crossref] [PubMed]
- Travaglini KJ, Nabhan AN, Penland L, et al. A molecular cell atlas of the human lung from single-cell RNA sequencing. Nature 2020;587:619-25. [Crossref] [PubMed]
- Cheng S, Li Z, Gao R, et al. A pan-cancer single-cell transcriptional atlas of tumor infiltrating myeloid cells. Cell 2021;184:792-809.e23. [Crossref] [PubMed]
- Bertani FR, Mozetic P, Fioramonti M, et al. Classification of M1/M2-polarized human macrophages by label-free hyperspectral reflectance confocal microscopy and multivariate analysis. Sci Rep 2017;7:8965. [Crossref] [PubMed]
- Smith TD, Tse MJ, Read EL, et al. Regulation of macrophage polarization and plasticity by complex activation signals. Integr Biol (Camb) 2016;8:946-55. [Crossref] [PubMed]
- Orecchioni M, Ghosheh Y, Pramod AB, et al. Macrophage Polarization: Different Gene Signatures in M1(LPS+) vs. Classically and M2(LPS-) vs. Alternatively Activated Macrophages. Front Immunol 2019;10:1084. [Crossref] [PubMed]
- Wu X, Xu W, Feng X, et al. TNF-a mediated inflammatory macrophage polarization contributes to the pathogenesis of steroid-induced osteonecrosis in mice. Int J Immunopathol Pharmacol 2015;28:351-61. [Crossref] [PubMed]
- Kwiecień I, Polubiec-Kownacka M, Dziedzic D, et al. CD163 and CCR7 as markers for macrophage polarization in lung cancer microenvironment. Cent Eur J Immunol 2019;44:395-402. [Crossref] [PubMed]
- Martinez FO, Gordon S. The M1 and M2 paradigm of macrophage activation: time for reassessment. F1000Prime Rep 2014;6:13. [Crossref] [PubMed]
- Zhang F, Wang H, Wang X, et al. TGF-β induces M2-like macrophage polarization via SNAIL-mediated suppression of a pro-inflammatory phenotype. Oncotarget 2016;7:52294-306. [Crossref] [PubMed]
- Lopes RL, Borges TJ, Zanin RF, et al. IL-10 is required for polarization of macrophages to M2-like phenotype by mycobacterial DnaK (heat shock protein 70). Cytokine 2016;85:123-9. [Crossref] [PubMed]
- Chen Y, Wu G, Li M, et al. LDHA-mediated metabolic reprogramming promoted cardiomyocyte proliferation by alleviating ROS and inducing M2 macrophage polarization. Redox Biol 2022;56:102446. [Crossref] [PubMed]
- Chen RH, Xiao ZW, Yan XQ, et al. Tumor Cell-Secreted ISG15 Promotes Tumor Cell Migration and Immune Suppression by Inducing the Macrophage M2-Like Phenotype. Front Immunol 2020;11:594775. [Crossref] [PubMed]
- Kwon R, Hong BK, Lee KG, et al. Regulation of tumor growth by leukocyte-specific protein 1 in T cells. J Immunother Cancer 2020;8:e001180. [Crossref] [PubMed]
- Liu Z, Gu S, Lu T, et al. IFI6 depletion inhibits esophageal squamous cell carcinoma progression through reactive oxygen species accumulation via mitochondrial dysfunction and endoplasmic reticulum stress. J Exp Clin Cancer Res 2020;39:144. [Crossref] [PubMed]
- Liu P, Sun SJ, Ai YJ, et al. Elevated nuclear localization of glycolytic enzyme TPI1 promotes lung adenocarcinoma and enhances chemoresistance. Cell Death Dis 2022;13:205. [Crossref] [PubMed]
- Guda MR, Rashid MA, Asuthkar S, et al. Pleiotropic role of macrophage migration inhibitory factor in cancer. Am J Cancer Res 2019;9:2760-73.
- Almatroodi SA, McDonald CF, Pouniotis DS. Alveolar Macrophage Polarisation in Lung Cancer. Lung Cancer Int 2014;2014:721087. [Crossref] [PubMed]
- Xu F, Wei Y, Tang Z, et al. Tumor associated macrophages in lung cancer: Friend or foe? Mol Med Rep 2020;22:4107-15. (Review). [Crossref] [PubMed]
- Merino Salvador M, Gómez de Cedrón M, Moreno Rubio J, et al. Lipid metabolism and lung cancer. Crit Rev Oncol Hematol 2017;112:31-40. [Crossref] [PubMed]
- Eltayeb K, La Monica S, Tiseo M, et al. Reprogramming of Lipid Metabolism in Lung Cancer: An Overview with Focus on EGFR-Mutated Non-Small Cell Lung Cancer. Cells 2022;11:413. [Crossref] [PubMed]
- Chen Y, Tan W, Wang C. Tumor-associated macrophage-derived cytokines enhance cancer stem-like characteristics through epithelial-mesenchymal transition. Onco Targets Ther 2018;11:3817-26. [Crossref] [PubMed]
- Duan Z, Luo Y. Targeting macrophages in cancer immunotherapy. Signal Transduct Target Ther 2021;6:127. [Crossref] [PubMed]
- Schraufstatter IU, Zhao M, Khaldoyanidi SK, et al. The chemokine CCL18 causes maturation of cultured monocytes to macrophages in the M2 spectrum. Immunology 2012;135:287-98. [Crossref] [PubMed]
- Zhou Z, Peng Y, Wu X, et al. CCL18 secreted from M2 macrophages promotes migration and invasion via the PI3K/Akt pathway in gallbladder cancer. Cell Oncol (Dordr) 2019;42:81-92. [Crossref] [PubMed]
- Kim MJ, Sun HJ, Song YS, et al. CXCL16 positively correlated with M2-macrophage infiltration, enhanced angiogenesis, and poor prognosis in thyroid cancer. Sci Rep 2019;9:13288. [Crossref] [PubMed]
- Nielsen SR, Quaranta V, Linford A, et al. Macrophage-secreted granulin supports pancreatic cancer metastasis by inducing liver fibrosis. Nat Cell Biol 2016;18:549-60. [Crossref] [PubMed]
- Quaranta V, Rainer C, Nielsen SR, et al. Macrophage-Derived Granulin Drives Resistance to Immune Checkpoint Inhibition in Metastatic Pancreatic Cancer. Cancer Res 2018;78:4253-69. [Crossref] [PubMed]