Diagnostic performance of a deep learning-based method in differentiating malignant from benign subcentimeter (≤10 mm) solid pulmonary nodules
Highlight box
Key findings
• A deep-learning (DL) method could more accurately differentiate between benign and malignant subcentimeter (≤10 mm) solid pulmonary nodules (SSPNs) using computed tomography than radiologists with 10 and 15 years of experience in thoracic imaging and reduce uncertainty in diagnosis.
What is known and what is new?
• The diagnosis of SSPNs remains challenging, and misdiagnoses can lead to severe negative consequences for patients. The diagnosis of SSPNs using the DL method provides a more accurate diagnosis of benign or malignant SSPNs with reduced uncertainty. Unlike the human performance, the performance of the DL model is not affected by nodule size.
What is the implication, and what should change now?
• This DL method may reduce uncertainty in diagnosis and improve diagnostic accuracy, especially for SSPNs smaller than 8 mm.
Introduction
With the wide application of chest computed tomography (CT) and popularization of low-dose CT (LDCT), the detection rate of solid pulmonary nodules has increased remarkably. A study from the Early Lung Cancer Action Project showed that solid nodules accounted for 81% of all nodules detected at baseline (1). Solid nodules are extremely common, with a malignancy rate of approximately 30% (range, 23–75%) (2). It is often difficult to differentiate between benign and malignant solid pulmonary nodules in clinical practice. Malignant solid nodules usually show high-grade malignancy, early metastasis, rapid growth, and poor prognosis (3-5). According to the 8th edition of the tumor-node-metastasis (TNM) classification for lung cancer, the 5-year survival rate of stage IA1 lung cancer is 90%, and 12% in stage IIIC, demonstrating the increased urgency for early diagnosis (6). For T1aN0M0 lung cancer, the recurrence risk of solid nodules is higher than that of part-solid and pure ground-glass nodules, although the diameter of solid nodules is ≤10 mm (7). Lymph nodal metastasis can occur even in subcentimeter solid non-small cell lung cancer (8). Hence, the prognosis of patients can be effectively improved if malignant solid nodules are diagnosed and treated at the subcentimeter stage.
However, the diagnosis of subcentimeter (≤10 mm) solid pulmonary nodules (SSPNs) remains challenging. The differential diagnosis of nodules is primarily dependent upon their CT features, including nodule size, density, lobulation, and spiculation (9). Unfortunately, these features are not typical in most SSPNs, making diagnosis difficult for radiologists. For the majority of SSPNs that cannot be determined qualitatively, follow-up observation is often recommended (10-13). However, no consensus has been reached, and follow-up causes additional radiation exposure and psychological and financial burdens for patients (14). Invasive biopsies and surgeries can lead to overtreatment and subsequent unnecessary complications for patients with benign nodules that are misdiagnosed as malignant, or who require surgical diagnosis (15). Hence, a more accurate and safe diagnostic approach is needed for SSPN patients.
Recently, with the advancement of artificial intelligence (AI), deep learning (DL) has achieved multilayer nonlinear transformation without requiring time-intensive manual feature extraction, and has shown high efficiency and robustness (16,17). DL models can extract and learn image features imperceptible to the human eye, avoid subjective influences, and assist radiologists in diagnosis (18), proving effective in differentiating malignant from benign nodules (19-22). For instance, Tang et al. (19) proposed a DL model to improve the classification performance for benign and malignant pulmonary nodules, which achieved an accuracy, sensitivity, specificity, and receiver operating characteristic (ROC) area under the curve (AUC) of 94.4%, 90.9%, 92.6%, and 0.931, respectively. However, few studies have evaluated the performance of DL models in diagnosing SSPNs. Our prior study has shown that the accuracy of the DL model is comparable to that of radiologists, but only 11 SSPNs were included in the test set (23). Thus, the model’s diagnostic efficiency for SSPNs remains unknown.
This study aimed to assess the diagnostic performance of the previously proposed DL model for differentiating malignant SSPNs from benign ones by comparing it against the performance of radiologists in clinical practice. We present this article in accordance with the STARD reporting checklist (available at https://jtd.amegroups.com/article/view/10.21037/jtd-23-985/rc).
Methods
Nodule selection
The study was conducted in accordance with the Declaration of Helsinki (as revised in 2013). The study was approved by the Ethics Committee of National Cancer Center/Cancer Hospital, Chinese Academy of Medical Sciences and Peking Union Medical College (No. 21/473-3144), and individual consent for this retrospective analysis was waived. We retrospectively selected 200 cases of SSPNs (100 benign and 100 malignant), confirmed by surgical pathology or follow-up, between April 2011 and November 2021 from our database. The inclusion criteria for the nodules were as follows: (I) mean solid nodule diameter ≤10 mm; (II) malignant and benign nodules confirmed by surgical pathology, with nodules that remained stable for more than 2 years after follow-up or that disappeared for less than 2 years of follow-up considered benign; and (III) chest CT slice thickness ≤1.25 mm with a 50% overlap reconstruction. The exclusion criteria were as follows: (I) metastases confirmed histologically by surgical resection or that could not be excluded by follow-up; (II) poor image quality or evident artifacts on CT images; and (III) calcified nodules. All images were interpreted by two radiologists. The diagnostic reports were given by a junior radiologist and confirmed by a medium-senior radiologist. The CT reports were retrospectively reviewed.
Image acquisition and equipment
Chest CT images were obtained using 8-detector (LightSpeed Ultra, General Electric Medical Systems, GE HealthCare, Anaheim, CA, USA), 16-detector (ProSpeed or Discovery ST, General Electric Medical Systems), and 64-detector (LightSpeed VCT or Optima CT660, General Electric Medical Systems; Toshiba Aquilion, Toshiba Medical Systems, Toshiba International Corporation, Houston, TX, USA) multi-sliced scanners and were reconstructed using standard algorithms at 120 kVp and 250–350 mA. Reconstruction thicknesses were 0.625, 0.75, 1.00, or 1.25 mm at a 0.8-mm interval. For CT-enhanced scanning, 80–100 mL contrast agent was injected intravenously at 2.5 mL/s, and imaging was performed 25–30 s after injection.
Chest CT image analysis
The results of the clinical CT reports were divided into three groups: benign (radiologists diagnosed the lesion as benign or indicated a high probability of benignity), malignant (the radiologists diagnosed the lesion as malignant or indicated a high probability of malignancy), and indeterminate (the report did not offer a definitive diagnosis, except suggesting follow-up, or showed that benign and malignant remained to be distinguished).
All pulmonary nodules included in this study were processed by DL-based AI software, “Dr. Wise System” (National Device Registration Approval No. 20203210920), which was developed by the Deepwise AI Lab and has been applied to clinical work. The DL-based model tested in this study for diagnosing SSPNs adopted a filter-guided pyramid network with DenseNet (24) as the backbone, which can relate local features to global context (Figure 1). The DL model included 4,978 pulmonary nodules for training, and the validation set contained another 500 nodules. Its diagnostic accuracy for all types of pulmonary nodules was confirmed as comparable with the radiologists (70% vs. 64%, P=0.243), and more details of the model were presented elsewhere (23). The model provided the ability to determine the malignant probability (0–100%) of each nodule. The diagnostic criteria of the model were as follows: benign, (malignant probability of 0–39.9%), indeterminate (malignant probability of 40–59.9%), and malignant (malignant probability of 60–100%).
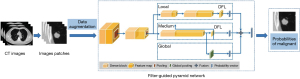
The diagnostic results of the model for all nodules were compared to those of the radiologists. Considering the mean diameter of each nodule, the 200 nodules were divided into three size groups: 3–6-, 6–8-, and 8–10-mm. For each group, the results of the model were compared to those of the radiologists.
Statistical analyses
Statistical analyses were performed using SPSS software version 25.0 (IBM Corp., Armonk, NY, USA) and MedCalc version 19.2.6 (MedCalc Software Ltd., Ostend, Belgium). Diagnostic differences between the AI model and the radiologists were examined using the McNemar-Bowker test, and the differences in the composition of diagnostic results between the two methods were evaluated using the McNemar test. Taking the results of histopathology and follow-up as the gold standard, the diagnostic accuracy of the model and radiologists for benign nodules (benign vs. malignant and indeterminate nodules) was evaluated using the ROC curve, and the AUCs of the two methods were compared using the Delong test. The chi-squared or Fisher’s exact test was used to evaluate the differences in diagnostic results among the 3–6-, 6–8-, and 8–10-mm groups. All statistical tests were two-sided, and values of P<0.05 were considered significant.
Results
Clinical and histopathological characteristics
In total, 200 SSPNs were included. The distribution of benign and malignant nodules in the three groups is shown in Figure 2. The clinical characteristics of the patients and nodules are shown in Table 1. The histopathological findings of the 43 benign nodules and 100 malignant nodules are summarized in Figure 3, and the remaining 57 nodules were proven to be benign by follow-up.
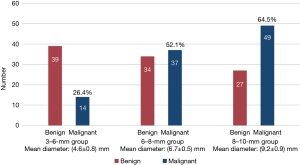
Table 1
Clinical characteristics | Value |
---|---|
Age (years) | 56.5±10.1 |
Sex | |
Male | 79 (43.6) |
Female | 102 (56.4) |
Diameter (mm) | 7.1±2.0 |
Nodule location | |
RUL | 35 (17.5) |
RML | 18 (9.0) |
RLL | 59 (29.5) |
LUL | 40 (20.0) |
LLL | 48 (24.0) |
Data are expressed as mean ± SD or n (%). RUL, right upper lobe; RML, right middle lobe; RLL, right lower lobe; LUL, left upper lobe; LLL, left lower lobe; SD, standard deviation.
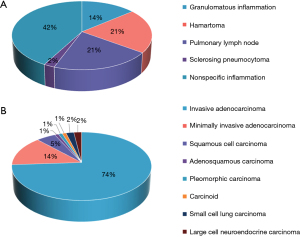
Diagnostic results of the AI model
The AI model could automatically detect nodules in CT images without manual segmentation, with all the 200 nodules detected successfully. The model diagnosed 124 (62.0%) as benign, 66 (33.0%) as malignant, and 10 as indeterminate (5.0%), of which 7 were malignant and 3 were benign. Regarding indeterminate results as errors, the diagnostic accuracy of the model was 71.5% (143/200). The diagnostic accuracies of the model for the 3–6-, 6–8-, and 8–10-mm groups were 75.5% (40/53), 62.0% (44/71), and 77.6% (59/76), respectively. There were no diagnostic differences for nodules among the three size groups (P=0.083). Rates of indeterminate diagnoses for the three nodule groups were 3.8% (2/53), 8.5% (6/71), and 2.6% (2/76), respectively, and the difference in the results among these groups was not significant (P=0.29).
Diagnostic results of the radiologists
The radiologists diagnosed 44 (22.0%) benign, 47 (23.5%) malignant, and 109 indeterminate (54.5%) lesions, of which 55 were malignant and 54 were benign. Regarding indeterminate results as errors, the diagnostic accuracy of the radiologists was 38.5% (77/200). The diagnostic accuracies of the radiologists for the 3–6-, 6–8-, and 8–10-mm groups were 28.3% (15/53), 28.2% (20/71), and 55.3% (42/76), respectively. The results for the three nodule size groups were not completely equivalent (χ2=14.548, P=0.001). Multiple comparison analysis showed that the radiologists’ diagnostic accuracy for the 8–10-mm group was higher than that for the 6–8- and 3–6-mm groups, with these differences being statistically significant (χ2=11.049, P=0.001; χ2=9.203, P=0.002). However, there was no significant difference between the radiologists’ accuracy for nodules in the 6–8- and 3–6-mm groups (P=0.98). The indeterminate diagnoses of the radiologists for the three size groups were 66.0% (35/53), 66.2% (47/71), and 35.5% (27/76), respectively, and the differences among the three groups were statistically significant (χ2=17.796, P<0.001). The results of the multiple comparison analysis showed that the radiologists’ indeterminate diagnoses for the 8–10-mm group were less than those for the 6–8- and 3–6-mm groups (χ2=13.813, P<0.001; χ2=11.645, P=0.001). However, there was no significant difference between the 6–8- and 3–6-mm groups (P=0.98).
Comparison of diagnostic performance between the AI and radiologists
Both the AI model and the radiologists’ diagnoses were benign, malignant, and indeterminate. The results of the McNemar-Bowker test indicated that the diagnostic difference between the two methods was statistically significant (χ2=98.345, P<0.001). Regarding the composition of the diagnostic results, the benign and malignant results of the model were higher than those of the radiologists, whereas its indeterminate results were lower than those of the radiologists, with statistically significant differences (χ2=66.426, P<0.001; χ2=6.353, P=0.01; χ2=91.467, P<0.001). Regarding indeterminate results as errors, the diagnostic accuracy of the model was higher than that of the radiologists, and the difference between the two methods was statistically significant (71.5% vs. 38.5%, χ2=49.128, P<0.001). The above results are summarized in Table 2.
Table 2
Diagnostic results | AI model | Radiologists | P value |
---|---|---|---|
Benign | 124 (62.0) | 44 (22.0) | <0.001 |
Malignant | 66 (33.0) | 47 (23.0) | 0.011 |
Indeterminate | 10 (5.0) | 109 (54.5) | <0.001 |
Accuracy | 143 (71.5) | 77 (38.5) | <0.001 |
Data are expressed as n (%). P<0.05 indicates significant difference. AI, artificial intelligence.
ROC curves were drawn to compare the diagnostic accuracy of the model and that of the radiologists (benign vs. malignant and indeterminate), as shown in Figure 4. The AUCs of the DL-based model and the radiologists were 0.750 [95% confidence interval (CI), 0.648–0.808] and 0.660 (95% CI, 0.590–0.725), respectively. The difference in the AUCs between the two methods was statistically significant (P=0.02). The sensitivities, specificities of the model and radiologists were 63% (63/100) and 87% (87/100) vs. 94% (94/100) and 38% (38/100), respectively, with significant differences in all parameters between the two methods (P<0.001).
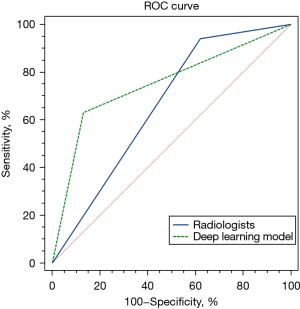
Regarding indeterminate results as errors, for the 3–6-mm group, the diagnostic accuracies and indeterminate results of the model and the radiologists were 75.5% and 28.3% vs. 3.8% and 66.0%, respectively, with significant differences in results between the two methods (χ2=21.333, P<0.001; χ2=29.257, P<0.001). For the 6–8-mm group, the diagnostic accuracies and indeterminate results of the model and the radiologists were 62.0% and 28.2% vs. 8.5% and 66.2%, respectively. Significant differences were found between the results of the two methods (χ2=15.559, P<0.001; χ2=35.556, P<0.001). For the 8–10-mm group, the accuracies and indeterminate results of the model and radiologists were 77.6% and 55.3% vs. 2.6% and 35.5%, respectively. The results of the two methods were significantly different (χ2=10.240, P=0.001; χ2=23.040, P<0.001). These results are summarized in Table 3. Some examples of nodules diagnosed by the AI model and the radiologists are shown in Figure 5.
Table 3
Groups | AI model | Radiologists | P value |
---|---|---|---|
3–6 mm | |||
Accuracy | 40 (75.5) | 15 (28.3) | <0.001 |
Indeterminate | 2 (3.8) | 35 (66.0) | <0.001 |
6–8 mm | |||
Accuracy | 44 (62.0) | 20 (28.2) | <0.001 |
Indeterminate | 6 (8.5) | 47 (66.2) | <0.001 |
8–10 mm | |||
Accuracy | 59 (77.6) | 42 (55.3) | 0.001 |
Indeterminate | 2 (2.6) | 27 (35.5) | <0.001 |
Data are expressed as n (%). AI, artificial intelligence.
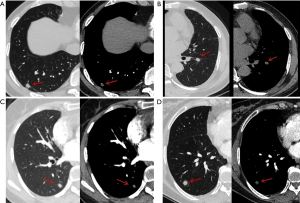
Discussion
This study aimed to test the diagnostic performance of the DL model by comparing it with that of radiologists in differentiating benign from malignant SSPNs. The results revealed that the model provided a more accurate diagnosis of benign or malignant SSPNs with reduced uncertainty. Unlike the radiologists, the performance of the model was not affected by nodule size.
Recently, DL has made significant advancements in the classification of benign and malignant pulmonary nodules (25). Nevertheless, most studies on DL have focused on all types of nodules, rather than specifically exploring SSPNs. In most recent studies, nodules were diagnosed as benign or malignant (26,27). Large nodules can be qualitatively determined by their shape, margins, contour, and other characteristics, whereas most SSPNs are not morphologically typical. Radiologists tend to diagnose indeterminate nodules and recommend further follow-up or examination because malignancy cannot be ruled out in clinical work. Therefore, it fits better with the actual clinical condition to classify the results into benign, malignant, and indeterminate. Based on a prior study, we considered the interval of 40–59.9% malignancy as the indeterminate diagnosis for the model (23).
Considering the indeterminate results as errors, the diagnostic accuracy of the model was significantly higher than that of the radiologists. Compared with previous outcomes, there was little difference in the diagnostic accuracy of the model for different types of nodules, whereas the radiologists’ accuracy decreased considerably for SSPNs (23), due to the increased uncertain diagnoses. The above results demonstrate that the DL model can assist radiologists in enhancing the diagnostic accuracy of SSPNs and reducing uncertain diagnoses. The ROC curve, which was used to compare the diagnostic performance of benign nodules (benign vs. malignant and indeterminate) of the model and radiologists, revealed that the model had a higher accuracy and AUC than the radiologists, indicating that it achieves better diagnostic performance. In addition, the model showed lower sensitivity and higher specificity than the radiologists because of their conservative diagnosis; they were more cautious and tended to recommend follow-up for nodules that could not be judged as benign.
In this study, 200 subcentimeter solid nodules were divided into three groups based on size. The results showed that the radiologists’ diagnostic accuracy for the 8–10-mm group was higher than that for the 6–8- and 3–6-mm groups, and the indeterminate results of the radiologists for the 8–10-mm group were lower than that of the 6–8- and 3–6-mm groups. No statistically significant difference was found in the diagnostic accuracy and indeterminate results of the radiologists between the 3–6- and 6–8-mm groups, suggesting that the radiologists’ diagnosis is susceptible to the size of the nodules, especially nodules <8 mm, and diagnostic efficiency might be further impaired. In contrast, the model showed similar diagnostic accuracy and indeterminate results among the three nodule size groups, indicating stable diagnostic efficacy for nodules of different sizes. The analysis for each group revealed that the model had higher accuracy and reported fewer indeterminate results than the radiologists, especially for nodules <8 mm, which may give the radiologists confidence.
Although the AI model outperformed the radiologists in diagnosis, the number of misdiagnoses (malignant nodules diagnosed as benign or vice versa) was higher for the model than for the radiologists. Compared to the radiologists, it was more common for the model to misdiagnose the malignant nodules as benign (37/100), and the benign nodules were misdiagnosed as malignant less frequently (10/100). Moreover, the corresponding results for the radiologists’ diagnoses were 6/100 and 8/100. This is probably because the radiologists were more cautious and would comprehensively evaluate patients’ medical history, serological indicators, and related clinical information, whereas the model’s judgments were simply based on CT images, which is a deficiency. If the indeterminate results were classified as errors, the misdiagnosis rate of the model would be lower than that of the radiologists. The numbers of the model misdiagnosing malignancy as benign or indeterminate and misdiagnosing benign as malignant or indeterminate were 44/100 and 13/100, respectively, and the corresponding results for the radiologists’ diagnoses were 61/100 and 62/100, respectively. These results suggest that radiologists are conservative in diagnosing nodules without typical imaging and clinical characteristics, and the model can effectively reduce this uncertainty. In the future, a multimodal DL model can be developed to overcome the above shortcomings and further improve efficiency by incorporating patients’ clinical information.
This study has some limitations. First, this was a single-center retrospective study of nodules selected from an oncology center. There might be bias in the database, which can be resolved by using a larger sample size from multiple centers. Prospective studies are necessary to better observe the diagnostic value of DL methods in patients with SSPNs. Besides, it is difficult to characterize SSPNs for malignancy or benign due to the small size in clinical practice, because some morphological features of SSPNs such as margin, border, etc. are difficult to determine. What’s more, because few DL models have been developed for SSPNs, this study lacks effective comparisons to other studies. Although the DL-based model was superior to the radiologists in achieving differential diagnosis, the model was trained and validated using solid and subsolid nodules of different sizes instead of only SSPNs, which might lead to insufficient training of the model for SSPNs and hinder the model from achieving optimal diagnostic performance. The development of a DL model dedicated exclusively to SSPNs is expected to further improve diagnostic accuracy for small nodules.
Conclusions
In summary, this study indicates that compared to radiologists, the DL diagnostic method is more effective in differentiating benign and malignant subcentimeter solid nodules in clinical practice, which can effectively reduce uncertainty for radiologists while improving their accuracy, make up for deficiencies in established diagnoses, and mitigate follow-up pressures on patients to some extent.
Acknowledgments
Funding: This work was supported by
Footnote
Reporting Checklist: The authors have completed the STARD reporting checklist. Available at https://jtd.amegroups.com/article/view/10.21037/jtd-23-985/rc
Data Sharing Statement: Available at https://jtd.amegroups.com/article/view/10.21037/jtd-23-985/dss
Peer Review File: Available at https://jtd.amegroups.com/article/view/10.21037/jtd-23-985/prf
Conflicts of Interest: All authors have completed the ICMJE uniform disclosure form (available at https://jtd.amegroups.com/article/view/10.21037/jtd-23-985/coif). ZZ is an employee of Beijing Deepwise & League of PhD Technology Co., Ltd., Beijing, China. The other authors have no conflicts of interest to declare.
Ethical Statement: The authors are accountable for all aspects of the work in ensuring that questions related to the accuracy or integrity of any part of the work are appropriately investigated and resolved. The study was conducted in accordance with the Declaration of Helsinki (as revised in 2013). The study was approved by the Ethics Committee of National Cancer Center/Cancer Hospital, Chinese Academy of Medical Sciences and Peking Union Medical College (No. 21/473-3144) and individual consent for this retrospective analysis was waived.
Open Access Statement: This is an Open Access article distributed in accordance with the Creative Commons Attribution-NonCommercial-NoDerivs 4.0 International License (CC BY-NC-ND 4.0), which permits the non-commercial replication and distribution of the article with the strict proviso that no changes or edits are made and the original work is properly cited (including links to both the formal publication through the relevant DOI and the license). See: https://creativecommons.org/licenses/by-nc-nd/4.0/.
References
- Henschke CI, Yankelevitz DF, Mirtcheva R, et al. CT screening for lung cancer: frequency and significance of part-solid and nonsolid nodules. AJR Am J Roentgenol 2002;178:1053-7. [Crossref] [PubMed]
- She Y, Zhao L, Dai C, et al. Development and validation of a nomogram to estimate the pretest probability of cancer in Chinese patients with solid solitary pulmonary nodules: A multi-institutional study. J Surg Oncol 2017;116:756-62. [Crossref] [PubMed]
- Chu ZG, Zhang Y, Li WJ, et al. Primary solid lung cancerous nodules with different sizes: computed tomography features and their variations. BMC Cancer 2019;19:1060. [Crossref] [PubMed]
- McWilliams A, Tammemagi MC, Mayo JR, et al. Probability of cancer in pulmonary nodules detected on first screening CT. N Engl J Med 2013;369:910-9. [Crossref] [PubMed]
- Ye T, Deng L, Wang S, et al. Lung Adenocarcinomas Manifesting as Radiological Part-Solid Nodules Define a Special Clinical Subtype. J Thorac Oncol 2019;14:617-27. [Crossref] [PubMed]
- Goldstraw P, Chansky K, Crowley J, et al. The IASLC Lung Cancer Staging Project: Proposals for Revision of the TNM Stage Groupings in the Forthcoming (Eighth) Edition of the TNM Classification for Lung Cancer. J Thorac Oncol 2016;11:39-51. [Crossref] [PubMed]
- Sun K, You A, Wang B, et al. Clinical T1aN0M0 lung cancer: differences in clinicopathological patterns and oncological outcomes based on the findings on high-resolution computed tomography. Eur Radiol 2021;31:7353-62. [Crossref] [PubMed]
- Hattori A, Matsunaga T, Hayashi T, et al. Prognostic Impact of the Findings on Thin-Section Computed Tomography in Patients with Subcentimeter Non-Small Cell Lung Cancer. J Thorac Oncol 2017;12:954-62. [Crossref] [PubMed]
- Snoeckx A, Reyntiens P, Desbuquoit D, et al. Evaluation of the solitary pulmonary nodule: size matters, but do not ignore the power of morphology. Insights Imaging 2018;9:73-86. [Crossref] [PubMed]
- Wood DE, Kazerooni EA, Baum SL, et al. Lung Cancer Screening, Version 3.2018, NCCN Clinical Practice Guidelines in Oncology. J Natl Compr Canc Netw 2018;16:412-41. [Crossref] [PubMed]
- MacMahon H, Naidich DP, Goo JM, et al. Guidelines for Management of Incidental Pulmonary Nodules Detected on CT Images: From the Fleischner Society 2017. Radiology 2017;284:228-43. [Crossref] [PubMed]
- Gould MK, Donington J, Lynch WR, et al. Evaluation of individuals with pulmonary nodules: when is it lung cancer? Diagnosis and management of lung cancer, 3rd ed: American College of Chest Physicians evidence-based clinical practice guidelines. Chest 2013;143:e93S-e120S.
- Bai C, Choi CM, Chu CM, et al. Evaluation of Pulmonary Nodules: Clinical Practice Consensus Guidelines for Asia. Chest 2016;150:877-93. [Crossref] [PubMed]
- Zhuo Y, Zhan Y, Zhang Z, et al. Clinical and CT Radiomics Nomogram for Preoperative Differentiation of Pulmonary Adenocarcinoma From Tuberculoma in Solitary Solid Nodule. Front Oncol 2021;11:701598. [Crossref] [PubMed]
- Iaccarino JM, Wiener RS. Pulmonary Nodule Guidelines: What Physicians Do When Evidence-Based Guidelines Lack High-Quality Evidence. Chest 2017;152:232-4. [Crossref] [PubMed]
- Sun W, Zheng B, Qian W. Automatic feature learning using multichannel ROI based on deep structured algorithms for computerized lung cancer diagnosis. Comput Biol Med 2017;89:530-9. [Crossref] [PubMed]
- Meyer P, Noblet V, Mazzara C, et al. Survey on deep learning for radiotherapy. Comput Biol Med 2018;98:126-46. [Crossref] [PubMed]
- Shi F, Chen B, Cao Q, et al. Semi-Supervised Deep Transfer Learning for Benign-Malignant Diagnosis of Pulmonary Nodules in Chest CT Images. IEEE Trans Med Imaging 2022;41:771-81. [Crossref] [PubMed]
- Tang S, Ma R, Li Q, et al. Classification of benign and malignant pulmonary nodules based on the multiresolution 3D DPSECN model and semisupervised clustering. IEEE Access 2021;9:43397-410.
- Huang H, Wu R, Li Y, et al. Self-Supervised Transfer Learning Based on Domain Adaptation for Benign-Malignant Lung Nodule Classification on Thoracic CT. IEEE J Biomed Health Inform 2022;26:3860-71. [Crossref] [PubMed]
- Zhang H, Chen L, Gu X, et al. Trustworthy learning with (un)sure annotation for lung nodule diagnosis with CT. Med Image Anal 2023;83:102627. [Crossref] [PubMed]
- Lv W, Wang Y, Zhou C, et al. Development and validation of a clinically applicable deep learning strategy (HONORS) for pulmonary nodule classification at CT: A retrospective multicentre study. Lung Cancer 2021;155:78-86. [Crossref] [PubMed]
- Wang YW, Wang JW, Yang SX, et al. Proposing a deep learning-based method for improving the diagnostic certainty of pulmonary nodules in CT scan of chest. Eur Radiol 2021;31:8160-7. [Crossref] [PubMed]
- Tu X, Xie M, Gao J, et al. Automatic Categorization and Scoring of Solid, Part-Solid and Non-Solid Pulmonary Nodules in CT Images with Convolutional Neural Network. Sci Rep 2017;7:8533. [Crossref] [PubMed]
- Li L, Yang F, Li J, et al. Research Progress on Benign and Malignant Lung Nodule Classification Based on Deep Learning. In: Fuzhou: 2020 Cross Strait Radio Science & Wireless Technology Conference (CSRSWTC); 2020:1-3.
- Sun K, Chen S, Zhao J, et al. Convolutional Neural Network-Based Diagnostic Model for a Solid, Indeterminate Solitary Pulmonary Nodule or Mass on Computed Tomography. Front Oncol 2021;11:792062. [Crossref] [PubMed]
- Yang K, Liu J, Tang W, et al. Identification of benign and malignant pulmonary nodules on chest CT using improved 3D U-Net deep learning framework. Eur J Radiol 2020;129:109013. [Crossref] [PubMed]