Proteomic sequencing analysis in a rat model of atrial fibrosis caused by chronic intermittent hypoxia
Highlight box
Key findings
• We identified 145 differentially expressed proteins between the atrial fibrillation and control groups. These included Myh7, Myl2, Myl3, and Atpla3, which are involved in signaling pathways related to hypertrophic cardiomyopathy, glycerolipid metabolism, and cardiac muscle contraction. Western blotting revealed the upregulation of Myh7, Myl2, and Myl3 and the downregulation of Atpla3 in the atrial fibrillation group compared with the control group.
What is known and what is new?
• Proteins play key roles in many cellular processes, and dysregulation of proteins may lead to disease. As a characteristic change in atrial structural remodeling in atrial fibrillation, atrial fibrosis provides a basis for the induction and maintenance of atrial fibrillation.
• Myh7, Myl2, Myl3, and Atpla3 may play key roles in the progression of atrial fibrillation through their involvement in cardiovascular-disease-related signaling pathways.
What is the implication, and what should change now?
• These findings improve our understanding of the pathogenesis of atrial fibrillation and provide potential treatment targets for atrial fibrillation.
Introduction
Atrial fibrillation (AF) is a common type of tachyarrhythmia with an occurrence rate of 2% (1). As the global population ages, the prevalence of AF is increasing (2). Disordered contraction of the atria in AF may lead to thrombus shedding, resulting in cerebral and pulmonary embolisms. Moreover, a long-term rapid ventricular rate can induce heart failure, seriously affecting patients’ quality of life (3). The current clinical treatment for AF includes medical and surgical interventions. The goal of medical interventions is to convert the sinus rhythm or control the ventricular rate and prevent thrombosis. Treatment methods mainly involve radiofrequency ablation or left atrial appendage occlusion (4). However, the cure rate of AF remains low, and atrial fibrosis caused by long-term AF influences the outcomes of clinical treatment (5). An improved understanding of the mechanisms underlying AF may reveal new therapeutic targets.
Proteins play key roles in many cellular processes, and dysregulation of proteins may lead to disease (6). Characterizing specific proteins is important for understanding disease development (7). Proteomics based on mass spectrometry (MS) has been widely performed over the past decade (1) and has revealed detailed information on the pathological mechanisms underlying the development of AF. For instance, Mayr et al. identified 17 differentially expressed proteins (DEPs) between patients with persistent AF and those with sinus rhythm using proteome analysis (8). Additionally, Zhang et al. analyzed proteins in the left and right atria of patients with AF resulting from mitral valve disease and identified 223 DEPs in these patients compared with patients with sinus rhythm (9). Although these dysregulated proteins are potential targets for AF treatment, few proteins have been validated as targets for AF treatment by using alternative technologies (10).
As a characteristic change in atrial structural remodeling in AF, atrial fibrosis provides a basis for the induction and maintenance of AF. We previously constructed a rat model of atrial fibrosis using the chronic intermittent hypoxia (CIH) method (11). In the current study, we performed proteome sequencing and detected DEPs in atrial samples between CIH and control groups. Additionally, the functions and pathways associated with these DEPs and their interactions were identified. The expression levels of several key proteins were further validated. This study was conducted to understand the changes occurring in protein levels in the atrial tissue in AF and determine the mechanism whereby atrial fibrosis contributes to AF progression. We present this article in accordance with the ARRIVE reporting checklist (available at https://jtd.amegroups.com/article/view/10.21037/jtd-23-704/rc).
Methods
Animal experiments
Thirty-two male Sprague-Dawley rats aged 6 weeks (weighing 150–200 g) were provided by the Second Hospital of Tianjin Medical University and Tianjin Institute of Cardiology, China. The rats were allowed to acclimate for 1 week at 25±2 ℃ with a photoperiod of 12 h/12 h. They were then weighed, sorted by weight, and randomly assigned to CIH (n=16) or control groups (n=16) with random number table. The rats were maintained under sterile conditions and given free access to water and food. Rats in the CIH group were subjected to 7 h of intermittent hypoxia every day for 8 weeks to establish an AF model, as previously described (12). After 8 weeks, the rats were weighed, and echocardiography was performed. Atrial tissues were collected from the rats after intraperitoneal anesthesia with 3% amobarbital sodium and the tissues were stored at −80 ℃ until analysis. The animals died during model establishment were excluded from analysis. Experiments were performed under a project license (No. TMUaMEC2016012) granted by the Animal Care and Ethics Committee of Tianjin Medical University, in compliance with institutional guidelines for the care and use of animals. A protocol was prepared before the study without registration.
Hematoxylin-eosin staining, Masson’s trichrome staining, and immunohistochemistry
Histopathological changes were determined using hematoxylin and eosin staining, and myocardial fibrosis was visualized using Masson’s trichrome staining.
For immunohistochemistry, the atrial tissues were cleaned with a phosphoric acid buffer solution, followed by paraformaldehyde fixation, ethanol dehydration, and paraffin embedding. The paraffin block was sectioned at a thickness of 0.05 mm, and the sections were immersed in citrate buffer for antigen retrieval. They were then incubated with primary and secondary antibodies (Table 1) to detect the expression of fibrosis indicators, including connective tissue growth factor (CTGF), collagen I (Col I), Col III, matrix metalloproteinase 2 (MMP2), MMP9, alpha-smooth muscle actin (α-SMA), and transforming growth factor-β1 (TGF-β1). Histological slices were observed under a microscope (Olympus, Tokyo, Japan), and areas of positive staining were quantified using Image-Pro software (Media Cybernetics, Rockville, MD, USA).
Table 1
Antibodies | Dilution (IHC/WB) | Solvent (IHC/WB) |
---|---|---|
Primary antibodies | ||
Rabbit anti-collagen I antibody (ab270993) | 1:500/1:1,000 | PBS/TBST |
Rabbit anti-collagen III antibody (ab7778) | 1:200/1:5,000 | PBS/TBST |
Rabbit anti-CTGF (ab227180) | 1:100/1:1,000 | PBS/TBST |
Rabbit anti-TGF-β1 (ab170874) | 1:150/1:1,000 | PBS/TBST |
Mouse anti-MMP2 (ab86607) | 1:200/1:1,000 | PBS/TBST |
Rabbit anti-MMP9 (ab76003) | 1:1,000/1:5,000 | PBS/TBST |
Mouse anti-α-SMA (ab7817) | 1:200/1:1,000 | PBS/TBST |
Rabbit anti-Myh7 (ab172967) | –/1:5,000 | –/TBST |
Mouse anti-Atp1a3 (ab2826) | –/1:1,000 | –/TBST |
Rabbit anti-Myl2 (ab92721) | –/1:10,000 | –/TBST |
Rabbit anti-Myl3 (ab108516) | –/1:10,000 | –/TBST |
Mouse anti-β-actin (ab8226) | –/1:5,000 | PBS/TBST |
Secondary antibodies | ||
Goat anti-rabbit IgG-HRP (ab7090) | 1:5,000 | PBS/TBST |
Goat anti-mouse IgG-HRP (ab97040) | 1:5,000 | PBS/TBST |
IHC, immunohistochemistry; WB, western blotting; PBS, phosphate buffer saline; TBST, Tris buffered saline tween; CTGF, connective tissue growth factor; TGF, transforming growth factor; MMP, matrix metalloproteinase; SMA, smooth muscle actin; Myh, myosin heavy chain; Atp1a3, ATPase Na+/K+ transporting subunit alpha 3; Myl, myosin light chain; IgG, immunoglobin G; HRP, horseradish peroxidase.
Western blotting
Proteins were extracted from atrial tissues (50 mg), and their concentrations were determined using the bicinchoninic acid method. The proteins were then transferred onto a methanol-activated polyvinylidene fluoride membrane, which was blocked with 5% bovine serum albumin. The membrane was incubated with primary antibodies (rabbit polyclonal anti-Col I, -Col III, -CTGF -MMP2, -MMP9, -α-SMA, -TGF-β1, -Myh7, -Atp1a3, -Myl2, and -Myl3 antibodies) overnight in a shaker at 4 ℃. After rinsing, the membrane was incubated with a horseradish-peroxidase-labeled secondary antibody (1:10,000) for 45 min. Finally, the bands were visualized using an EZ-ECL kit. ImageJ software (NIH, Bethesda, MD, USA) was used for grayscale measurements. Detailed information on the primary and secondary antibodies is presented in Table 1.
Tandem mass tag-based quantitative proteomics
Protein extraction and quantification
Three atrial samples from rats in each of the two groups were randomly selected and used for quantitative proteomics. The samples were transferred into centrifuge tubes, to which radioimmunoprecipitation assay lysis buffer (300 µL) and phenylmethylsulfonyl fluoride (Sigma, St. Louis, MO, USA) were added, followed by centrifugation at room temperature. The protein concentration in the supernatant was determined using the bicinchoninic acid method (13).
Protein (10 µg) was extracted and separated using 12% sodium dodecyl sulfate-polyacrylamide gel electrophoresis. The gel was stained with Coomassie brilliant blue (14) and scanned using an ImageScanner scanner (GE Healthcare, Chicago, IL, USA) in full-color mode.
Tandem mass tag labeling
After quantification, 100 µg of protein was added to a 10 K ultrafiltration tube, and 120 µL of reducing agent buffer (10 mM dithiothreitol, 8 M urea, 100 mM tetraethyl-ammonium bromide, pH 8.0) was reacted with the protein sample at 60 ℃ for 1 h. Iodoacetamide was added to a final concentration of 50 mM and reacted for 40 min in the dark. After centrifugation, the supernatant was collected, 100 µL of tetraethyl-ammonium bromide buffer (300 mM) was added, and the mixture was centrifuged for 20 min. Next, 100 µL of tetraethylammonium bromide (TEAB) buffer (300 mM) and 2 µL of sequence-grade trypsin solution (1 µg/µL) were added. After performing the reaction for 12 h, centrifugation was performed, and the peptides were collected following enzymatic hydrolysis. TEAB buffer was added again, and the bottom layer was collected, centrifuged, and lyophilized. Next, 100 µL of TEAB buffer (200 mM) was added to the lyophilized sample, and 40 µL of the resulting sample was transferred to a 1.5 mL tube for the labeling reaction. Anhydrous acetonitrile (41 µL) was added to the tubes containing the tandem mass tags and the resulting mixture was added to the sample and allowed to react for 1 h. Finally, 8 µL of 5% hydroxylamine was added to quench the reaction. The samples were then lyophilized and stored at −80 ℃.
Reversed-phase chromatographic separation
Samples were fractionated using an Agilent 1100 HPLC system (Agilent Technologies, Santa Clara, CA, USA). The peptide mixture was loaded onto an Agilent Zorbax Extend-C18 reversed-phase column (2.1×150 mm, 5 µm). The detection wavelengths were 210 and 280 nm. Solvent A was acetonitrile-H2O (2:98, v/v), and solvent B was acetonitrile-H2O (90:10, v/v). The flow rate was set at 300 µL/min. The gradient elution conditions were: 98% A (0–8 min); 98–95% A (8–8.01 min); 95–75% A (8.01–38 min); 75–60% A (38–50 min); 60–10% A (50–50.01 min); 10% A (50.01–60 min); 10–98% A (60–60.01 min); and 98% A (60.01–65 min). Ten fractions were collected each minute for 8–50 min.
Liquid chromatography-tandem MS analysis
The samples were injected into a C18 column (75 µm × 150 mm, C18, 2 µm, 100 Å; Acclaim PepMap RSLC; Thermo Fisher Scientific, Waltham, MA, USA) for gradient elution (flow rate: 300 nL/min). Solvent A was H2O-formic acid (99.9:0.1, v/v), and solvent B was acetonitrile-H2O-formic acid (80:19.9:0.1, v/v/v). The gradient elution conditions were 8% B (0–55 min), 30% B (55–79 min), 50% B (79–80 min), and 100% B (80–90 min).
The first stage of MS quality resolution was 70,000, and the automatic gain control value was 1e6. MS scanning was set to m/z 300–1,600, and the 10 highest peaks were subjected to tandem MS (MS/MS) scanning. All MS/MS maps were collected via high-energy collision cracking in the data-dependent positive ion mode (collision energy, 32; MS/MS resolution, 17,500; automatic gain control, 2e5; maximum ion accumulation time, 80 ms; dynamic exclusion time, 15 s).
Data analysis
Data were analyzed using Proteome DiscovererTM 2.2 software (Thermo Fisher Scientific). The UniProt rat database was used for this study.
Bioinformatics analysis
The results of liquid chromatography-MS/MS analysis were screened using a Sequest HT score >0 and ≥1 unique peptides after removing blank values. The DEPs between the CIH and control groups were identified based on thresholds of fold-change >1.2 or <5/6 and P<0.05. Correlations among the DEPs were analyzed based on Pearson’s algorithm.
The OmicsBean omics database platform was used to perform Gene Ontology (GO) (15) and Kyoto Encyclopedia of Genes and Genomes (KEGG) pathway (16) enrichment analyses of the DEPs. The GO annotations included biological process (BP), molecular function (MF), and cellular component (CC). The top ten BP, CC, and MF items and the top 11 KEGG pathways were displayed.
The interactions between the DEPs and pathways were analyzed using OmicsBean. The interaction network of the ten most significant pathways in the KEGG results and their interacting proteins were visualized using Cytoscape (17).
Enzyme-linked immunosorbent assay (ELISA)
The serum concentrations of key DEPs, including Myh7, Myl2, Myl3 and Atpla3, of the rats in the Cont and CIH groups were determined using ELISA kits according to the manufacturer’s instructions (Cloud-Clone Corp., Wuhan, China).
Statistical analysis
Statistical analyses were performed using SPSS 19.0. Student’s t-tests were used to assess differences between the two groups. Statistical analysis was performed by authors who were not aware of the group allocation of the experiment. Data points with a high degree of dispersion were excluded from analysis. P<0.05 was considered to indicate statistical significance.
Results
Model validation
The echocardiography results showed that the left atrial diameter (5.08±0.29 vs. 4.58±0.39 mm), left ventricular end-diastolic diameter (7.92±0.50 vs. 7.50±0.41 mm), left ventricular end-systolic diameter (4.94±0.44 vs. 4.42±0.31 mm), diastolic left ventricular volume (338.76±46.72 vs. 299.39±35.70 µL), and systolic left ventricular volume (116.00±23.35 vs. 89.36±14.74 µL) in the CIH group increased significantly compared with those in the control group (n=15, P<0.05). Additionally, the interventricular septal thickness (1.78±0.13 vs. 2.07±0.22 mm), systolic ventricular septal thickness (2.79±0.27 vs. 3.16±0.25 mm), left ventricular posterior wall depth (1.95±0.21 vs. 2.19±0.26 mm), left ventricular posterior wall thickness during systole (2.95±0.30 vs. 3.21±0.28 mm), left ventricular fractional shortening (37.70%±3.15% vs. 41.03±2.62%), and left ventricular ejection fraction (65.83%±3.88% vs. 70.17%±3.11%) in the CIH group decreased significantly compared with those in the control group (n=15, P<0.05). Ultrasound data showed that CIH led to left atrial enlargement and a decline in left ventricular function in the rats (Table 2, Figure 1A). AF was successfully induced in the CIH group (Figure 1B,1C). There was no significant difference in systolic or diastolic blood pressure in the rat caudal artery between the CIH and control groups (n=15, P>0.05).
Table 2
Variables | CONT (n=15) | CIH (n=15) | P |
---|---|---|---|
mPAP (mmHg) | 69.63±2.22* | 66.62±2.86 | 0.003 |
LAD (mm) | 4.58±0.39 | 5.08±0.29* | <0.001 |
IVS-D (mm) | 2.07±0.22* | 1.78±0.13 | <0.001 |
IVS-S (mm) | 3.16±0.25* | 2.79±0.27 | <0.001 |
LVEDD (mm) | 7.50±0.41 | 7.92±0.50* | 0.018 |
LVESD (mm) | 4.42±0.31 | 4.94±0.44* | <0.001 |
LVPW-D (mm) | 2.19±0.26* | 1.95±0.21 | 0.009 |
LVPW-S (mm) | 3.21±0.28* | 2.95±0.30 | 0.022 |
LVvol-D (µL) | 299.39±35.70 | 338.76±46.72* | 0.015 |
LVvol-S (µL) | 89.36±14.74 | 116.00±23.35* | 0.001 |
LVEF (%) | 70.17±3.11* | 65.83±3.88 | 0.002 |
FS (%) | 41.03±2.62* | 37.70±3.15 | 0.004 |
BP-S (mmHg) | 124.07±10.12 | 128.00±6.88 | 0.224 |
BP-D (mmHg) | 88.27±12.07 | 94.60±14.04 | 0.196 |
Data are presented as mean ± standard deviation. *, P<0.05 compared with CONT (n=15). CONT, control; CIH, chronic intermittent hypoxia; mPAP, mean pulmonary artery pressure; LAD, left atrial diameter; IVS-D, interventricular septal thickness during diastole; IVS-S, interventricular septal thickness during systole; LVEDD, left ventricular end-diastolic diameter; LVESD, left ventricular end-systolic diameter; LVPW-D, left ventricular posterior wall thickness during diastole; LVPW-S, left ventricular posterior wall thickness during systole; LVvol-D, left ventricular volume during diastole; LVvol-S, left ventricular volume during systole; LVEF, left ventricular ejection fraction; FS, fractional shortening; BP-S, systolic blood pressure; BP-D, diastolic blood pressure.
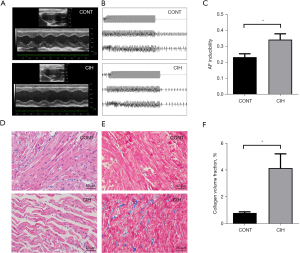
Hematoxylin and eosin staining revealed that, after 8 weeks of CIH, the rat atrial muscle was disrupted, myocardial fibers were thick, cell arrangement was disordered, and intercellular spaces were enlarged (Figure 1D). After Masson’s trichrome staining, the aniline-blue-stained area of the atrial tissue and collagen deposition were significantly greater in the CIH group than the control group (Figure 1E). The collagen volume fraction was significantly higher in the CIH group than the control group (P<0.001; Figure 1F).
Immunohistochemistry and western blotting results revealed that the expression levels of fibrosis-related proteins (Col I, Col III, CTGF, MMP2, MMP9, α-SMA, and TGF-β1) in the CIH group increased significantly compared with those in the control group (Figure 2A-2D, P<0.05 or 0.01). These results suggested that the AF model was successfully established.
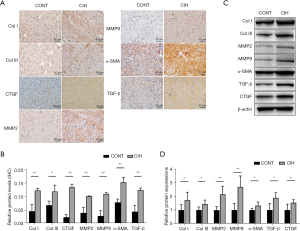
General characteristics of proteins
Atrial tissues from rats in the two groups were subjected to proteome sequencing. The number of proteins corresponding to different molecular weights is shown in Figure 3A. The distribution of the number of peptides for each protein is shown in Figure 3B. The different peptide lengths corresponding to each protein are shown in Figure 3C. Qualitative analysis was used to compare the peptide fragments with those from the background database, and database search software was used to obtain the complete protein sequence based on the coverage index. As shown in Figure 3D, most proteins (46.3%) had a peptide coverage rate of 1–10%.
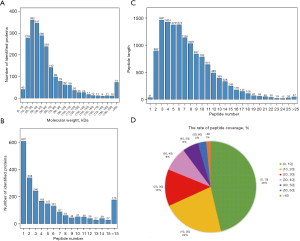
DEP identification
Using thresholds of fold-change >1.2 or <5/6 and P<0.05, 145 DEPs were identified between the CIH and control groups, including 29 up- and 116 down-regulated proteins. The heatmap, volcano map, and histogram of the DEPs are shown in Figure 4A-4C. Pearson’s correlation analysis was performed for the DEPs, and a heatmap of the top 50 DEPs is presented in Figure 4D.
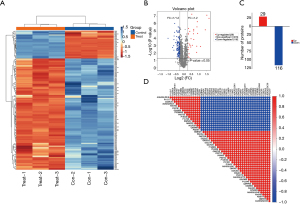
Function and pathway analyses of DEPs
GO and KEGG analyses were performed to understand the functions of the DEPs. The numbers of BP, CC, and MP terms and the KEGG pathways identified are shown in Figure 5A. A GO chord diagram was constructed to represent the relationship between the GO terms and the DEPs (Figure 5B). The top three GO terms were translation, cardiac muscle contraction, and complement activation, with classical pathway for BP; extracellular space, protein-containing complex, and endoplasmic reticulum for CC; and RNA binding, structural constituent of ribosome, and serine-type endopeptidase inhibitor activity for MF (Figure 5C). These DEPs were also involved in some cardiovascular-related pathways, such as hypertrophic cardiomyopathy (HCM), glycerolipid metabolism, cardiac muscle contraction, dilated cardiomyopathy, and adrenergic signaling in cardiomyocytes (Figure 5D).

Protein-pathway interaction network analysis
The interactions between the 10 most significant pathways and DEPs are shown in Figure 6. The adrenergic signaling in cardiomyocytes, HCM, and dilated cardiomyopathy pathways interacted with Myh7, Myl2, and Myl3. Glycerolipid metabolism interacted with Agpat3, Agpat4, and Akr1b10. Cardiac muscle contraction interacted with Atp1a3, Myl2, and Myl3. Additionally, Rps5, Rps28, and ribosomal protein L3 showed high degrees of interaction with other proteins or pathways.
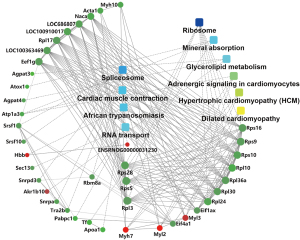
Validation of protein levels
According to the KEGG results, Myh7, Atp1a3, Myl2, and Myl3 were closely correlated with cardiac muscle contraction and adrenergic signaling in cardiomyocytes. Additionally, Myh7, Myl2, and Myl3 were closely related to dilated cardiomyopathy and HCM. Four DEPs were selected for expression-level validation by western blotting and ELISA. As shown in Figure 7A,7B, Myh7, Myl2, and Myl3 were upregulated, whereas Atpla3 was downregulated in the atrial tissue and serum of the CIH group compared with those in the control group (n=15, P<0.05), which is consistent with the sequencing results.
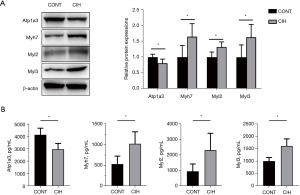
Discussion
We determined the proteins predominantly expressed in an AF model. We identified 145 DEPs between the CIH and control groups. Myh7, Atp1a3, Myl2, and Myl3 have important roles in several cardiovascular-related pathways, such as HCM, glycerolipid metabolism, and dilated cardiomyopathy. The protein levels of Myh7, Myl2, Myl3, and Atpla3, determined by western blotting, were consistent with those determined by sequencing.
Various animal models have been used to investigate the pathophysiology of AF (18,19). AF, which exhibits a high recurrence rate after ablation, is associated with atrial fibrosis (20). We simulated the progression of atrial fibrosis through CIH. Fibrosis manifests as an increased amount of fibrous connective tissue in the cellular matrix and the deposition of collagen fibers, mainly Col I and Col III (21). Additionally, CTGF and TGF-β1 play important roles in tissue fibrosis and can induce collagen production and deposition (22,23). MMPs are extracellular matrix proteolytic enzymes that regulate the extracellular environment and degrade collagen (24). MMPs, particularly MMP2 and MMP9, are associated with diabetic cardiac fibrosis (25,26). We established a rat model of atrial fibrosis, which was verified by assessing biomarkers of fibrosis.
The DEPs between the CIH and control groups were significantly involved in several cardiovascular disease signaling pathways. For instance, the HCM pathway interacted with Myh7, Myl2, and Myl3. AF is a common sequela of HCM (27). Although Myh7 expression is robust in the ventricles, multiple studies have shown an association between the switching of atrial myosin heavy chain protein isoforms and the development of AF (28,29). Additionally, genetic variation in Myh7 is related to high levels of the propeptide of type I collagen in the early stages of HCM, suggesting that fibrosis mediates the association between Myh7 and AF (30). Myl2 is a sarcomeric protein that belongs to the EF-hand calcium-binding protein family. During early embryogenesis, Myl2 plays essential roles in maintaining cardiac morphology and in regulating cardiac contractile function (31,32). Moreover, MLC-2v phosphorylation has been shown to play direct roles in cross-bridge cycling kinetics, calcium-dependent cardiac muscle contraction, cardiac torsion, cardiac function, and various cardiac diseases (31-33). Moreover, variations in Myl2 and Myl3 can cause HCM or restrictive cardiomyopathy and increase the risk of sudden cardiac death (32,34). However, the molecular mechanism still warrants further investigation.
Furthermore, adrenergic signaling in cardiomyocytes and cardiac muscle contraction pathways were correlated with Atp1a3. Atp1a3 maintains the electrochemical gradient of the resting plasma membrane in neurons. Variants in human Atp1a3 can lead to ventricular arrhythmias (35). β-adrenergic receptors belong to the superfamily of G-protein-coupled receptors. Signaling via these receptors plays a key role in regulating cardiovascular system function (36). β-Adrenergic receptor signaling is abnormal in patients with heart failure, and dysfunction of β-adrenergic receptors is an important determinant of age-related cardiac alterations (37). Anti-β1-adrenergic-receptor autoantibodies cause atrial structural remodeling and are associated with the development of AF (38). The cardiac muscle contraction pathway is involved in the pathogenesis of AF (39). Importantly, the expression levels of four DEPs associated with this pathway (Myh7, Atp1a3, Myl2, and Myl3) were consistent between western blotting and sequencing results. These proteins may serve as biomarkers of AF, as they may be involved in the pathogenesis of atrial fibrosis and AF via cardiovascular-related signaling pathways.
This study has some limitations. First, experiments were performed in a rat model, and not in humans. Therefore, the conclusions may be not applicable in humans. Second, the functions of the four proteins differentially expressed in AF were not explored in in vivo and in vitro experiments. Third, as cell sorting was not applied, it was difficult to distinguish between transcriptional regulation and altered cellular composition due to the invasion and proliferation of fibroblasts and immune cells. Therefore, additional experiments should be conducted to obtain more robust conclusions.
Conclusions
We identified DEPs between the CIH and control groups by establishing a rat model of AF. Four proteins that may play a critical role in the progression of AF via cardiovascular-related signaling pathways. These findings improve our understanding of the pathogenesis of AF and provide potential treatment targets for AF.
Acknowledgments
Funding: This work was supported by
Footnote
Reporting Checklist: The authors have completed the ARRIVE reporting checklist. Available at https://jtd.amegroups.com/article/view/10.21037/jtd-23-704/rc
Data Sharing Statement: Available at https://jtd.amegroups.com/article/view/10.21037/jtd-23-704/dss
Peer Review File: Available at https://jtd.amegroups.com/article/view/10.21037/jtd-23-704/prf
Conflicts of Interest: All authors have completed the ICMJE uniform disclosure form (available at https://jtd.amegroups.com/article/view/10.21037/jtd-23-704/coif). The authors have no conflicts of interest to declare.
Ethical Statement: The authors are accountable for all aspects of the work in ensuring that questions related to the accuracy or integrity of any part of the work are appropriately investigated and resolved. Experiments were performed under a project license (No. TMUaMEC2016012) granted by the Animal Care and Ethics Committee of Tianjin Medical University, in compliance with institutional guidelines for the care and use of animals.
Open Access Statement: This is an Open Access article distributed in accordance with the Creative Commons Attribution-NonCommercial-NoDerivs 4.0 International License (CC BY-NC-ND 4.0), which permits the non-commercial replication and distribution of the article with the strict proviso that no changes or edits are made and the original work is properly cited (including links to both the formal publication through the relevant DOI and the license). See: https://creativecommons.org/licenses/by-nc-nd/4.0/.
References
- Aebersold R, Mann M. Mass-spectrometric exploration of proteome structure and function. Nature 2016;537:347-55. [Crossref] [PubMed]
- Chugh SS, Havmoeller R, Narayanan K, et al. Worldwide epidemiology of atrial fibrillation: a Global Burden of Disease 2010 Study. Circulation 2014;129:837-47. [Crossref] [PubMed]
- Ponamgi SP, Siontis KC, Rushlow DR, et al. Screening and management of atrial fibrillation in primary care. BMJ 2021;373: [Crossref] [PubMed]
- Xu J, Luc JG, Phan K. Atrial fibrillation: review of current treatment strategies. J Thorac Dis 2016;8:E886-900. [Crossref] [PubMed]
- Zhao B, Wang W, Liu Y, et al. Establishment of a lncRNA-miRNA-mRNA network in a rat model of atrial fibrosis by whole transcriptome sequencing. J Interv Card Electrophysiol 2022;63:723-36. [Crossref] [PubMed]
- Mann M, Kulak NA, Nagaraj N, et al. The coming age of complete, accurate, and ubiquitous proteomes. Mol Cell 2013;49:583-90. [Crossref] [PubMed]
- Anderson NL, Anderson NG. Proteome and proteomics: new technologies, new concepts, and new words. Electrophoresis 1998;19:1853-61. [Crossref] [PubMed]
- Mayr M, Yusuf S, Weir G, et al. Combined metabolomic and proteomic analysis of human atrial fibrillation. J Am Coll Cardiol 2008;51:585-94. [Crossref] [PubMed]
- Zhang P, Wang W, Wang X, et al. Protein analysis of atrial fibrosis via label-free proteomics in chronic atrial fibrillation patients with mitral valve disease. PLoS One 2013;8:e60210. [Crossref] [PubMed]
- Sühling M, Wolke C, Scharf C, et al. Proteomics and transcriptomics in atrial fibrillation. Herzschrittmacherther Elektrophysiol 2018;29:70-5. [Crossref] [PubMed]
- Wang W, Zhang K, Li X, et al. Doxycycline attenuates chronic intermittent hypoxia‐induced atrial fibrosis in rats. Cardiovasc Ther 2018;36:e12321. [Crossref] [PubMed]
- Ma Z, Zhang K, Wang Y, et al. Doxycycline Improves Fibrosis-Induced Abnormalities in Atrial Conduction and Vulnerability to Atrial Fibrillation in Chronic Intermittent Hypoxia Rats. Med Sci Monit 2020;26:e918883. [Crossref] [PubMed]
- Smith PK, Krohn RI, Hermanson GT, et al. Measurement of protein using bicinchoninic acid. Anal Biochem 1985;150:76-85. [Crossref] [PubMed]
- Candiano G, Bruschi M, Musante L, et al. Blue silver: a very sensitive colloidal Coomassie G-250 staining for proteome analysis. Electrophoresis 2004;25:1327-33. [Crossref] [PubMed]
- Ashburner M, Ball CA, Blake JA, et al. Gene ontology: tool for the unification of biology. Nat Genet 2000;25:25-9. [Crossref] [PubMed]
- Kanehisa M. The KEGG database. Novartis Found Symp 2002;247:91-101; discussion 101-3, 119-28, 244-52.
- Shannon P, Markiel A, Ozier O, et al. Cytoscape: a software environment for integrated models of biomolecular interaction networks. Genome Res 2003;13:2498-504. [Crossref] [PubMed]
- Nishida K, Michael G, Dobrev D, et al. Animal models for atrial fibrillation: clinical insights and scientific opportunities. Europace 2010;12:160-72. [Crossref] [PubMed]
- Allessie M, Ausma J, Schotten U. Electrical, contractile and structural remodeling during atrial fibrillation. Cardiovasc Res 2002;54:230-46. [Crossref] [PubMed]
- Zhao A, Squara F, Marijon E, et al. Two-year clinical outcome after a single cryoballoon ablation procedure: A comparison of first- and second-generation cryoballoons. Arch Cardiovasc Dis 2017;110:543-9. [Crossref] [PubMed]
- Ni H, Li W, Zhuge Y, et al. Inhibition of circHIPK3 prevents angiotensin II-induced cardiac fibrosis by sponging miR-29b-3p. Int J Cardiol 2019;292:188-96. [Crossref] [PubMed]
- Kim KK, Sheppard D, Chapman HA. TGF-β1 Signaling and Tissue Fibrosis. Cold Spring Harb Perspect Biol 2018;10:a022293. [Crossref] [PubMed]
- Gyöngyösi M, Winkler J, Ramos I, et al. Myocardial fibrosis: biomedical research from bench to bedside. Eur J Heart Fail 2017;19:177-91. [Crossref] [PubMed]
- Iyer RP, Patterson NL, Fields GB, et al. The history of matrix metalloproteinases: milestones, myths, and misperceptions. Am J Physiol Heart Circ Physiol 2012;303:H919-30. [Crossref] [PubMed]
- Lindsey ML, Iyer RP, Zamilpa R, et al. A Novel Collagen Matricryptin Reduces Left Ventricular Dilation Post-Myocardial Infarction by Promoting Scar Formation and Angiogenesis. J Am Coll Cardiol 2015;66:1364-74. [Crossref] [PubMed]
- Li G, Xing W, Zhang M, et al. Antifibrotic cardioprotection of berberine via downregulating myocardial IGF-1 receptor-regulated MMP-2/MMP-9 expression in diabetic rats. Am J Physiol Heart Circ Physiol 2018;315:H802-13. [Crossref] [PubMed]
- Garg L, Gupta M, Sabzwari SRA, et al. Atrial fibrillation in hypertrophic cardiomyopathy: prevalence, clinical impact, and management. Heart Fail Rev 2019;24:189-97. [Crossref] [PubMed]
- Consortium G, Ardlie KG, Deluca DS, Segrè AV, Sullivan TJ, Young TR, et al. The Genotype-Tissue Expression (GTEx) pilot analysis: multitissue gene regulation in humans. Science. 2015;348:648-60. [Crossref] [PubMed]
- Belus A, Piroddi N, Ferrantini C, et al. Effects of chronic atrial fibrillation on active and passive force generation in human atrial myofibrils. Circ Res 2010;107:144-52. [Crossref] [PubMed]
- Ho CY, López B, Coelho-Filho OR, et al. Myocardial fibrosis as an early manifestation of hypertrophic cardiomyopathy. N Engl J Med 2010;363:552-63. [Crossref] [PubMed]
- Sheikh F, Lyon RC, Chen J. Functions of myosin light chain-2 (MYL2) in cardiac muscle and disease. Gene 2015;569:14-20. [Crossref] [PubMed]
- Tamamitsu AM, Nakagama Y, Domoto Y, et al. Poor Myocardial Compaction in a Patient with Recessive MYL2 Myopathy. Int Heart J 2021;62:445-7. [Crossref] [PubMed]
- Weterman MA, Barth PG, van Spaendonck-Zwarts KY, et al. Recessive MYL2 mutations cause infantile type I muscle fibre disease and cardiomyopathy. Brain 2013;136:282-93. [Crossref] [PubMed]
- Ingles J, Goldstein J, Thaxton C, et al. Evaluating the Clinical Validity of Hypertrophic Cardiomyopathy Genes. Circ Genom Precis Med 2019;12:e002460. [Crossref] [PubMed]
- Moya-Mendez ME, Ogbonna C, Ezekian JE, et al. ATP1A3-Encoded Sodium-Potassium ATPase Subunit Alpha 3 D801N Variant Is Associated With Shortened QT Interval and Predisposition to Ventricular Fibrillation Preceded by Bradycardia. J Am Heart Assoc 2021;10:e019887. [Crossref] [PubMed]
- Zhu W, Woo AY, Zhang Y, et al. β-adrenergic receptor subtype signaling in the heart: from bench to the bedside. Curr Top Membr 2011;67:191-204. [Crossref] [PubMed]
- de Lucia C, Eguchi A, Koch WJ. New Insights in Cardiac β-Adrenergic Signaling During Heart Failure and Aging. Front Pharmacol 2018;9:904. [Crossref] [PubMed]
- Shang L, Zhang L, Shao M, et al. Elevated β1-Adrenergic Receptor Autoantibody Levels Increase Atrial Fibrillation Susceptibility by Promoting Atrial Fibrosis. Front Physiol 2020;11:76. [Crossref] [PubMed]
- Wu N, Li J, Chen X, et al. Identification of Long Non-Coding RNA and Circular RNA Expression Profiles in Atrial Fibrillation. Heart Lung Circ 2020;29:e157-67. [Crossref] [PubMed]