Bioinformatics and functional experiments reveal that MRC2 inhibits atrial fibrillation via the PPAR signaling pathway
Highlight box
Key findings
• This study identified MRC2 as a novel inhibitory gene in atrial fibrillation (AF). MRC2 downregulation is associated with AF and modulates growth and apoptosis of angiotensin II-induced human cardiac fibroblasts via the PPAR pathway.
What is known and what is new?
• AF involves complex gene networks, but precise mechanisms and implicated genes are not fully understood.
• We discovered a unique role for MRC2 in AF, implying that its downregulation contributes to the disease. Our findings highlight the importance of MRC2 as a diagnostic biomarker and therapeutic target for AF.
What is the implication, and what should change now?
• These findings advance AF molecular mechanisms understanding, informing new diagnostic tools and treatments development.
• Further studies should validate the clinical relevance of MRC2 in AF and understand its disease role.
Introduction
Atrial fibrillation (AF), as a usual arrhythmia phenomenon, refers to severe electrical interference in the atrium, which is more common in the elderly (1). It usually manifests as palpitations, chest tightness, shortness of breath, dizziness, and some patients may also experience syncope, polyuria, angina and so on (2). The pathologic and physiologic mechanisms of AF involve multiple complex electrophysiologic, histologic, and biochemical changes. The interaction between these mechanisms leads to the onset, persistence, and worsening of AF (3). Experimental and clinical data suggest that the pathophysiology of AF is highly complex and involves a large number of important players, including oxidative stress, calcium overload, atrial dilatation, microRNA (miRNA), inflammation, and myofibroblast activation (4). Inflammation contributes to the prothrombotic inflammatory state associated with AF. Inflammatory biomarkers (e.g., C-reactive protein and interleukin-6) contribute to AF-related thrombosis. However, further studies are needed to understand the clinical significance of inflammatory biomarkers in predicting AF-related thromboembolism (5). A study has shown that the disease is closely related to diseases like coronary and congenital heart disease and is regarded as an invisible “killer” of cardiovascular and cerebrovascular diseases (6). AF can also cause cerebral artery embolism, sudden cardiac death, pulmonary embolism, heart failure, peripheral arterial embolism and other complications, which bring certain difficulties to clinical treatment (7). Although some patients can be treated with beta-blockers, calcium channel antagonists, digitalis and other drugs, electrical cardioversion, catheter ablation, and surgical maze surgery to improve the body’s homeostasis and restore normal heart rhythm, the prognosis of most patients is still not optimistic (8). Diabetes mellitus (DM) represents an independent risk factor for chronic AF (9). The prevalence of AF among individuals with DM is notably high, reaching alarming levels in recent years. Emerging evidence suggests that DM acts as a chronic inflammatory disease, contributing to the development and progression of AF (10). The presence of a chronic inflammatory state in DM patients with AF further complicates the disease course. A history of DM was identified as one of the components of high-risk AF, and early reporting of biomarkers facilitates its clinical detection (11). And a study proved that ELABELA (peptide hormone essential for cardiac development) is a protective factor in patients with cardiac arrhythmias and can be used as a prognostic marker for AF and its associated complications (12). Therefore, deep knowledge of the AF mechanism is essential to determine its biological markers and improve its treatment.
To better compile the genetic information of disease patients, the National Center for Biotechnology Information (NCBI) creates the Gene Expression Omnibus (GEO) database (13). The database stores a large number of microarray, next-generation sequencing, and other high-throughput sequencing data, which provides convenience for researchers to retrieve gene expression data of any species or man-made (14). Based on this database, high-throughput sequencing has been widely applied to analyze differentially expressed genes (DEGs) and search for hub genes (15). In addition, the methods of bioinformatics analysis focusing on genomics and proteomics are also widely used in the study of human diseases (16). The potential diagnostic key genes unearthed can also provide a theoretical basis for drug sensitivity analysis and targeted drug development for AF (17). Herein, exploring the pathogenesis of AF based on the above methods will provide a more comprehensively diagnostic direction for the treatment of patients.
In this study, to better explore the pathogenesis of AF and discover new potential clinical indicators, we first downloaded the microarray dataset of GSE143924 from the GEO database and conducted DEGs screening and weighted gene co-expression network analysis (WGCNA) for the overlapping genes. Subsequently, these genes were analyzed by bioinformatics analysis to determine the hub gene. Furthermore, the regulatory mechanisms of the hub gene in AF development were also investigated by cellular experiments. We present this article in accordance with the STREGA and MDAR reporting checklists (available at https://jtd.amegroups.com/article/view/10.21037/jtd-23-1235/rc).
Methods
Microarray data extraction
We searched the GSE143924 microarray data set from the GEO database in the format of series matrix file for subsequent analysis (18). The GSE143924 data set contained 15 patients in sinus rhythm after surgery (SR) and 15 patients developing postoperative AF after surgery (POAF) samples (19). The study was conducted in accordance with the Declaration of Helsinki (as revised in 2013).
Identification and classification of DEGs
Based on the GSE143924 data set, we compared 30 samples in the data set through GEO2R software in the GEO database (20) and set the selection conditions for DEGs, and the results obtained were displayed in a significantly ranked gene table. Fold change (FC) >1 was the standard for up-regulated DEGs, FC <1 for down-regulated DEGs, and they both met P<0.05. Finally, with the help of R software, the cluster distribution of DEGs in 30 samples was drawn into a heat map.
Gene Ontology (GO) and Kyoto Encyclopedia of Genes and Genomes (KEGG) analyses on DEGs
GO integrates the world’s largest source of information about gene functions, and is the basis for large-scale molecular biology and genetics experimental computational analysis in biomedical research (21). It describes the biological functions in three parts: cell component (CC), biological process (BP), and molecular function (MF) (22). The KEGG database has a powerful graphic function, which helps researchers intuitively understand the metabolic pathways and the relationship between the pathways (23). The DAVID database (https://david.ncifcrf.gov/) offers a systematic set of functional annotation tools (24). Herein, we performed an enrichment analysis of DEGs in BP and KEGG based on this database to understand the biological significance behind it.
WGCNA
We performed WGCNA using expression data from GSE143924. In order to conduct this analysis and identify AF-related modules in DEGs, the R package “WGCNA” was employed (25). The adjacency matrix was transformed into a topological overlap matrix using a soft-threshold power with a scale-free R2 near 0.85 and a slope near 1. For the sake of network design and module identification, we set the soft threshold power to 9. A crucial module was defined as one that has a high association with AF. The key module network of WGCNA was generated by Cytoscape (26). Then, area scale Venn diagrams of key module genes and DEGs were created by Venn Diagram Plotter (https://omics.pnl.gov/software/VennDiagramPlotter.php) to screen the overlapping genes (27).
Gene set enrichment analysis (GSEA) on overlapping genes
GSEA is a calculation method used to determine whether a set of priori-defined genes are statistically displayed (28). It is usually used in conjunction with the Molecular Signatures Database (MSigDB) to study the biological functions of genes (29). In this study, we performed enrichment analysis for ten key genes respectively, and P<0.05 was statistically significant.
Expression analysis and receiver operating characteristic (ROC) curve analysis
Peking University created the analytical tool Gene Expression Profiling Interactive Analysis (GEPIA), which includes RNA sequencing (RNA-seq) expression data from 9,736 cancer samples and 8,587 normal tissues (30). Using the “Single Gene Analysis” module of GEPIA, we examined the messenger RNA (mRNA) expressions of overlapping genes in AF for this study. In addition, ROC analysis was used to examine the validity of overlapping genes for AF prognosis prediction. To quantify the ROC, the area under the curve (AUC) was calculated. The accuracy of the prediction increases with AUC values.
Cell culture and transfection
Human cardiac fibroblasts (HCFs) were obtained from the Chinese Cell Resource Bank and cultured in Dulbecco’s modified Eagle’s medium (DMEM) with 10% fetal bovine serum (FBS), and the environment of the medium was set to 37 ℃, 95% air and 5% CO2 humid environment. Subsequently, MRC2 was knocked down [small interfering (si)-MRC2-1 and si-MRC2-2] and over-expressed (over-MRC2), respectively, in HCFs treated with angiotensin II (Ang II)/mock to build the AF model transfected with Lipofectamine 2000 reagent. After a period of time, the next step was performed after transfection.
Quantitative real-time polymerase chain reaction (qRT-PCR) assay
First, total RNA was actually extracted from HCFs cells with Trizol, and then RNA was reverse transcribed by PrimeScriptTM RT reagent kit (TaKaRa, Dalian, China) to synthesize cDNA. Subsequently, qRT-PCR was performed on an ABI7500 system (ABI, FosterCity, CA, USA) using SYBR MAsterMix (ABI). Finally, we applied 2−ΔΔCt method to determine the relative expression.
Cell counting kit-8 (CCK-8) assay
HCFs were added to a 96-well plate at a density of 1×103 per well plate, and then 10 µL of CCK-8 solution was injected into each well plate. Based on the CCK-8, the cell viability was observed at 24, 48, 72, 96 hours, and the optical density (OD) value of the cells at 450 nm was observed.
Flow cytometry assay
For apoptosis, flow cytometry was used here to detect apoptosis of HCFs treated with Ang II in different groups. Specifically, the transfected cells were first suspended in buffer and washed with phosphate buffered saline (PBS), then stained by Annexin V-fluorescein isothiocyanate (FITC)/propidium iodide (PI) assay kit (BD Bioscinge, Carlsbad, CA, USA) and flow cytometry was performed.
Western blotting (WB) assay
First, the protein was acquired from HCFs by radioimmunoprecipitation assay (RIPA) lysis buffer, and the protein concentration was detected and quantified by bicinchoninic acid (BCA) protein. Proteins were then transferred to polyvinylidene fluoride (PVDF) membranes blocked with 5% nonfat milk for 2 hours and detected by primary antibodies at 4 ℃ overnight. Then, PVDF membranes were kept with specific horseradish peroxidase (HRP)-conjugated secondary antibodies for 1 hour at indoor temperature. Finally, the signal was observed by the HyGLO HRP detection kit of Denville (Metuchen, NJ, USA), and the protein level was measured.
Research process
In this study, the GSE143924 microarray dataset was obtained from the GEO database, including 15 samples of patients in SR and 15 samples of patients who developed POAF. First, these 30 samples were compared by GEO2R software to obtain a significantly sorted gene list according to the selection conditions of DEGs, and a heat map of the clustered distribution of DEGs in these 30 samples was plotted using R software. Then, the DEGs were enriched by GO and KEGG using the DAVID database, and then the expression data in GSE143924 were analyzed by WGCNA to identify modules related to AF, and the key module network was obtained by setting appropriate soft thresholds and other parameters using the “WGCNA” package in the R software, and the genes overlapping with DEGs were filtered using the Venn diagram. Genes overlapping with DEGs. The overlapping genes were analyzed by GSEA and combined with the MSigDB to investigate the biological functions of these genes. The mRNA expression of these overlapping genes in AF was investigated using GEPIA, an analytical tool developed by Peking University, and ROC analysis was used to verify the validity of these genes in predicting the prognosis of AF.
At the molecular level, an AF model was constructed using HCFs by cell culture and transfection techniques, and the functions and mechanisms of action of the key genes were investigated by qRT-PCR analysis, CCK-8 experiments, and flow cytometry. The molecular mechanisms and potential therapeutic targets of AF were systematically explored.
Statistical analysis
GraphPadPrismV6 (Prism, San Diego, CA, USA) was used for analysis in all steps of this experiment, and each step was conducted for no less than three times (31). The mean and standard deviation (SD) were used to analyze the data, and statistical significance was inferred when P<0.05, indicating significance in a two-sided manner.
Results
GSE143924-DEGs associated with AF and pertinent enrichment analysis
Based on GEO2R, we identified 182 up-regulated and 228 down-regulated DEGs. The heat map in Figure 1A showed the cluster distribution of these DEGs in the GSE143924 dataset. The up-regulated DEGs in BP were enriched in eosinophil migration, eosinophil chemotaxis, positive regulation of endothelial cell apoptosis, regulation of guanosine triphosphatase (GTPase) activity, antigen receptor-mediated signaling pathways, and regulation of histone methylation (Figure 1B). The enrichment results in KEGG showed the top 8 pathways, namely lipids and atherosclerosis, allograft rejection, insulin secretion, yersinia infection, asthma, chemokine signaling pathway, primary immunity defects and nuclear factor-κB (NF-κB) signaling pathway (Figure 1C). Likewise, down-regulated DEGs were enriched in BP, such as extracellular structural organization, endoderm formation, regulation of ossification, regulation of humoral immune responses, phosphatidylcholine acyl chain remodeling (Figure 1D). These down-regulated DEGs were also enriched in 9 metabolic pathways in KEGG, namely α-linolenic acid metabolism, arachidonic acid, proteoglycans in cancer, focal adhesions, ether lipid metabolism, extracellular matrix (ECM)-receptor interaction, Hippo signaling pathway, Rap1 signaling and the complement and coagulation cascade (Figure 1E).
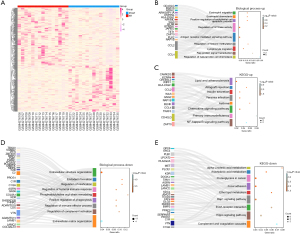
Tan module was the key module in WGCNA
GSE143924 were then analyzed by WGCNA. When the soft threshold power was 9 (unscaled R2=0.85, Figure 2A), we obtained 15 modules (Figure 2B-2D), with non-clustered DEGs in grey modules. From the module-feature correlations, tan module had the highest correlation with AF (correlation coefficient =0.359, P=0.051; Figure 2E).
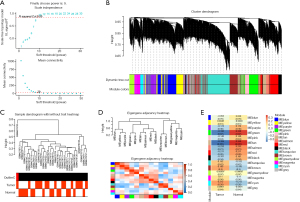
MRC2 was identified as the hub gene associated with AF
Figure 3A was a Venn diagram of tan module genes and GSE143924-DEGs, and 10 overlapping genes were acquired. To investigate potential pathways of these genes, GSEA was first performed (Figure 3B-3K). For example, MRC2 was enriched in primary bile acid biosynthesis [enrichment score (ES) =0.7012, normalized P value (NP) =0.0020], renin-angiotensin system (RAS) (ES =0.6133, NP =0.0145), cytosolic DNA sensing pathway (ES =0.5775, NP =0.0203), and PPAR signaling pathway (ES =0.4419, NP =0.0425; Figure 3G). To strengthen the reliability of the overlapping genes identified from the bioinformatics analysis, we first investigated the expressions of these genes in AF by the online website GEPIA based on The Cancer Genome Atlas (TCGA) data. As shown in Figure 4A-4J, the levels of these genes in the case group were closely lower, indicating that all of them were suppressor genes in AF. According to the AUC values of the genes in ROC curve, only MRC2 had the potential to be diagnostic biomarkers in AF with an AUC value of 0.8 (Figure 4K). Based on these findings, we confirmed that MRC2 was the hub gene.
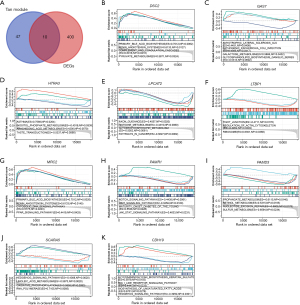
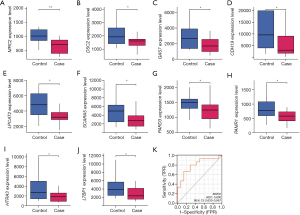
Over-MRC2 inhibited cell proliferation of Ang II-induced HCFs and promoted cell apoptosis
Based on the results in Figure 5A,5B, it appeared that the mRNA and protein expression levels of MRC2 were lower in Ang II-induced HCFs compared to normal controls. The expression of MRC2 was subsequently over-expressed and the efficiency was examined by qRT-PCR and WB (Figure 5C,5D). In the CCK-8 assay, we compared the cell proliferation between different groups and obtained that the proliferation of AF cells in the MRC2 overexpression group was significantly inhibited (Figure 5E). Following this, we found by flow cytometry that overexpressed MRC2 promoted the apoptosis of Ang II-induced HCFs (Figure 5F-5I).
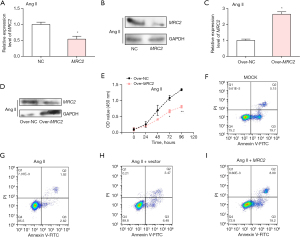
Knockdown of MRC2 promoted the cell proliferation of Ang II-induced HCFs
Compared with normal control, the level of MRC2 in HCFs cells after si-MRC2-1 and si-MRC2-2 transfection was detected by qRT-PCR method, and si-MRC2-1 had a more effective efficiency (Figure 6A). Afterwards, we constructed the AF cells by HCFs treated with Ang II. Among the four groups, negative control (NC), Ang II, Ang II + si-NC and Ang II + si-MRC2, the expression of MRC2 was the lowest in Ang II + si-MRC2 group (Figure 6B). In the CCK-8 experiment, the proliferation of HCFs cells was promoted after Ang II treatment, and MRC2 knockdown promoted Ang II-induced HCFs proliferation (Figure 6C).
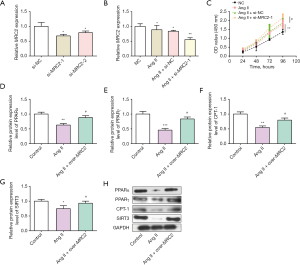
Protein expression levels of PPARα, PPARγ, CPT-1, SIRT3 in HCFs were increased by over-MRC2 in Ang II-induced HCFs
Based on the GSEA results, PPARα, PPARγ, CPT-1, SIRT3 proteins related to PPAR signaling pathway were selected to verify whether it is related to MRC2 in AF. In the WB experiments, we detected that the protein levels of these proteins in HCFs treated with Ang II and over-MRC2. The data demonstrated the protein expressions of them in Ang II-induced HCFs were increased by over-MRC2 (Figure 6D-6H). These results indicate PPAR signaling pathway is associated with the mechanism of MRC2 in AF progression.
Discussion
Although AF itself is not directly life-threatening, its clinical symptoms (such as palpitations, heart failure, etc.) (32) and its complications (such as stroke, etc.) (33) significantly increase disability and mortality possibilities. A study has shown that AF harms the quality of life by limiting physical activity, dying of social interactions and emotions, reducing happiness, increasing dependence and early retirement (34). Due to the above limitations, improving the efficiency of AF diagnosis and standard treatment is important. Further understanding of the molecular mechanism of AF is a basis to determine the molecular biomarkers of AF and develop promising therapies.
According to the gene expression profile GSE143924, the DEGs were identified between 15 POAF and 15 SR groups. The GO annotation and KEGG indicated the DEGs were closely related to the regulation of GTPase activity, NF-κB signaling pathway, Extracellular structure organization and Arachidonic acid metabolism. Bischoff et al. proposed that Rho GTPases operated as a binary switch by cycling between the inactive and active states, and played a vital role in connecting biochemical signals with biophysical cell activity, primarily through the rearrangement of actin and microtubule cytoskeleton (35). Polyakova et al. found that atrial fibrosis was an important factor in inducing and maintaining AF. The occurrence of AF is characterized by changes in the structure of atrial muscle cells, including cell hypertrophy, contractile disintegration, glycogen accumulation, myolysis, and changes in the expression of connexin (36). In addition, AF and its duration are related to atrial fibrosis. Atrial fibrosis can cause abnormal atrial conduction, especially in the case of heart failure (37). A recent study has shown that miRNAs are important regulators of several cardiovascular disease processes associated with the heart, and that atrial fibrosis is a hallmark of cardiac remodeling that perpetuates AF (38). Moreover, a study has revealed that in the blood, liver, muscle, and other organ systems, arachidonic acid functions as a structural lipid linked to phospholipids (39).
Next, we performed WGCNA on GSE143924 database to screen the key module, and identify 10 overlapping genes combined with GSE143924-DEGs.The selection of MRC2 as the hub gene among these 10 overlapping genes was based on the consistency of the results of multiple analyses showing its association with AF. In gene overlap analysis, MRC2 was found to have 10 overlapping genes with tan module genes and GSE143924-DEG, suggesting its relevance in specific biological contexts. By GSEA analysis, MRC2 was enriched in several functional pathways such as bile acid biosynthesis, RAS, cell membrane DNA sensing pathway, and PPAR signaling pathway, implying its important role in AF BPs. Gene expression analysis TCGA data and GEPIA tools showed that MRC2 expression was significantly reduced in AF patients, suggesting that it may have an inhibitory function in AF. It may be related to the pathophysiologic process of AF. In addition, MRC2 showed potential diagnostic value in biomarker analysis, with its ROC curve analysis determining that MRC2 has a high AUC value (0.8), results suggesting that it may be a biomarker for AF. Based on these findings, MRC2 was determined as the target gene in our study. MRC2 is a constitutive recycling endocytic receptor that belongs to the mannose receptor family. The MRC2-encoded protein has a vital function in ECM remodeling by mediating the internalization and lysosomal degradation of collagen ligands. Gai et al. found that MRC2 overexpression predicted poor prognosis in hepatocellular carcinoma (HCC) after hepatectomy, and that MRC2 might contribute to the upregulation of cell migration and invasion in transforming growth factor-driven HCC (40). Yang et al. showed that MRC2 promoted the epithelial-mesenchymal transition (EMT) process associated with ovarian cancer progression by disrupting epithelial cell-cell interactions (41). Study by López-Guisa et al. showed that by slowing the pace of interstitial collagen formation, MRC2 is crucial in the development of solid organ fibrosis, thereby protecting the parenchyma surrounding the kidney from fibrosis-related damage (42). Herein, we conducted functional experiments to explore the role of MRC2 in AF cells. The results demonstrated that MRC2 knockdown promoted the Ang II-induced HCFs cells, which conforms to the finding in expression analysis.
In GSEA, significantly enriched genomes related to MRC2 were primary bile acid biosynthesis, RAS, cytosolic DNA sensing pathway and PPAR signaling pathway. The study by Mascolo et al. suggests that two RAS pathways may counteract the evidence for the beginning of AF by several BPs, including inflammation and the buildup of epicardial adipose tissue (EAT) (43). EAT may cause the start of AF through both direct and indirect pathways, making it a predictor of the condition. In patients with diabetes experiencing AF, a chronic pro-inflammatory state characterized by imbalanced Ang II receptor signaling has been identified. Macrophages, which function as phagocytes and antigen-presenting cells, play a vital role in the secretion of growth factors, immune mediators, effector molecules, as well as the engulfment of pathogens or cellular debris, antigen processing, and presentation (44,45). Diversity and plasticity are hallmarks of macrophages, with two recognized subtypes: classically activated M1 macrophages and alternatively activated M2 macrophages (46,47). Notably, it has been suggested that the long noncoding RNA Gm9866 in RAW264.7 cells contributes to the enhanced expression of M2 markers, namely MRC1 and MRC2. Moreover, the expression of long noncoding RNA Gm9866 was observed to significantly increase after interleukin-4 treatment, while lipopolysaccharide treatment led to a notable decrease (48). The evidence supports the involvement of novel mechanisms in the upregulation of the long non-coding RNA Dnm3os, disruption of its interaction with nucleolin, and epigenetic modifications of target genes, all of which contribute to the promotion of the inflammatory macrophage phenotype in DM (49). In CD14+ monocytes, DRAIR is downregulated by factors like high glucose and palmitic acid but upregulated by anti-inflammatory cytokines and monocyte-to-macrophage differentiation through KLF4. DRAIR overexpression enhances anti-inflammatory and macrophage differentiation genes while inhibiting proinflammatory genes (50). These findings demonstrate the role of DRAIR in modulating the inflammatory phenotype of monocytes/macrophages and its downregulation in promoting chronic inflammation in diabetes.
The study by Zhu et al. shows that ANGPTL4 reverses cardiomyocyte apoptosis, inflammation. At the mechanism level, ANGPTL4 blocked the expression of PPARα and PPARγ proteins in atrial tissues (51). Namely, ANGPTL4 weakens Ang II-induced AF and atrial fibrosis by regulating PPARα and PPARγ signaling pathways. Thus, we speculated that MRC2 might regulate PPAR signaling pathway to suppress AF. In subsequent cellular experiments, we successively confirmed by qRT-PCR, WB, CCK-8 and flow cytometry assays that MRC2 was down-regulated in HCFs treated with Ang II, overexpression of MRC2 significantly inhibited the proliferation of Ang II-treated HCFs and promoted cell apoptosis, whereas knockdown of MRC2 significantly promoted the proliferation of HCFs. Then, we observed the protein levels of four AF-related factors (PPARα, PPARγ, CPT-1, and SIRT3) were higher in Ang II-HCFs cells with over-MRC2. Therefore, we proved that PPAR signaling pathway was involved in the regulatory process of MRC2 in AF.
In the present study, a potential key hub gene, MRC2, was identified by mining DEGs. it should be noted that this study has some limitations. First, AF pathogenesis is caused by a combination of multiple genetic and environmental factors. Second, due to the lack of clinical samples, we were unable to perform gene expression validation, and other basic experiments are needed to validate the function and regulatory mechanisms of these genes. Third, without the added validation of in vivo experiments, there may be limitations in clinical application.
Conclusions
In this study, we have identified the hub gene MRC2 for AF and explored the underlying mechanism of AF through a series of bioinformatic analyses. The results of gene expression analysis based on GEPIA show that MRC2 is a tumor suppressor gene of AF. Over-MRC2 inhibited the proliferation of Ang II-induced HCFs, promoted cell apoptosis. In contrast, knockdown of MRC2 expression could promote the proliferation of Ang II-induced HCFs, and the expression levels of 4 PPAR signaling pathway-related proteins in HCFs were correlated with MRC2 expression. All results show that MRC2 could suppress AF progression by PPAR signaling pathway, which will pave the way for the progression of effective diagnostic indicators and therapies for AF patients.
Acknowledgments
We thank Dr. Francesco Nappi (Centre Cardiologique du Nord, Saint-Denis, France) for the critical comments and valuable advice on this study.
Funding: This work was supported by
Footnote
Reporting Checklist: The authors have completed the STREGA and MDAR reporting checklists. Available at https://jtd.amegroups.com/article/view/10.21037/jtd-23-1235/rc
Data Sharing Statement: Available at https://jtd.amegroups.com/article/view/10.21037/jtd-23-1235/dss
Peer Review File: Available at https://jtd.amegroups.com/article/view/10.21037/jtd-23-1235/prf
Conflicts of Interest: All authors have completed the ICMJE uniform disclosure form (available at https://jtd.amegroups.com/article/view/10.21037/jtd-23-1235/coif). The authors have no conflicts of interest to declare.
Ethical Statement: The authors are accountable for all aspects of the work in ensuring that questions related to the accuracy or integrity of any part of the work are appropriately investigated and resolved. The study was conducted in accordance with the Declaration of Helsinki (as revised in 2013).
Open Access Statement: This is an Open Access article distributed in accordance with the Creative Commons Attribution-NonCommercial-NoDerivs 4.0 International License (CC BY-NC-ND 4.0), which permits the non-commercial replication and distribution of the article with the strict proviso that no changes or edits are made and the original work is properly cited (including links to both the formal publication through the relevant DOI and the license). See: https://creativecommons.org/licenses/by-nc-nd/4.0/.
References
- Barnett AS, Bahnson TD, Piccini JP. Recent Advances in Lesion Formation for Catheter Ablation of Atrial Fibrillation. Circ Arrhythm Electrophysiol 2016;9:e003299. [Crossref] [PubMed]
- Bachmayr-Heyda A, Reiner AT, Auer K, et al. Correlation of circular RNA abundance with proliferation--exemplified with colorectal and ovarian cancer, idiopathic lung fibrosis, and normal human tissues. Sci Rep 2015;5:8057. [Crossref] [PubMed]
- Wijesurendra RS, Casadei B. Mechanisms of atrial fibrillation. Heart 2019;105:1860-7. [Crossref] [PubMed]
- Jalife J, Kaur K. Atrial remodeling, fibrosis, and atrial fibrillation. Trends Cardiovasc Med 2015;25:475-84. [Crossref] [PubMed]
- Guo Y, Lip GY, Apostolakis S. Inflammatory Biomarkers and Atrial Fibrillation: Potential Role of Inflammatory Pathways in the Pathogenesis of Atrial Fibrillation-induced Thromboembolism. Curr Vasc Pharmacol 2015;13:192-201. [Crossref] [PubMed]
- Ebrahim MA, Escudero CA, Kantoch MJ, et al. Insights on Atrial Fibrillation in Congenital Heart Disease. Can J Cardiol 2018;34:1531-3. [Crossref] [PubMed]
- Baopeng T, Ling S, Jianhua M. Prevention of all-cause and sudden cardiac death in atrial fibrillation patients with long RR interval by pacemaker implantation and evaluation of quality of life. Heart 2011;97:A173-4.
- Callans DJ. In the clinic. Atrial fibrillation. Ann Intern Med 2008;149:ITC5-1-15; quiz ITC5-16.
- Staszewsky L, Cortesi L, Baviera M, et al. Diabetes mellitus as risk factor for atrial fibrillation hospitalization: Incidence and outcomes over nine years in a region of Northern Italy. Diabetes Res Clin Pract 2015;109:476-84. [Crossref] [PubMed]
- Huang JK, Lee HC. Emerging Evidence of Pathological Roles of Very-Low-Density Lipoprotein (VLDL). Int J Mol Sci 2022;23:4300. [Crossref] [PubMed]
- Zhong Y, Zhang J, Tang K, et al. Decreased plasma musclin levels are associated with potential atrial fibrillation in non-diabetic patients. Ann Transl Med 2021;9:203. [Crossref] [PubMed]
- Cui C, Zhou H, Xu J. ELABELA acts as a protective biomarker in patients with atrial fibrillation. J Thorac Dis 2021;13:6876-84. [Crossref] [PubMed]
- Demendi C, Börzsönyi B, Pajor A, et al. Abnormal fetomaternal glucocorticoid metabolism in the background of premature delivery: placental expression patterns of the 11β-hydroxysteroid dehydrogenase 2 gene. Eur J Obstet Gynecol Reprod Biol 2012;165:210-4. [Crossref] [PubMed]
- Barrett T, Troup DB, Wilhite SE, et al. NCBI GEO: archive for high-throughput functional genomic data. Nucleic Acids Res 2009;37:D885-90. [Crossref] [PubMed]
- Wang H, Nie X, Li X, et al. Bioinformatics Analysis and High-Throughput Sequencing to Identify Differentially Expressed Genes in Nebulin Gene (NEB) Mutations Mice. Med Sci Monit 2020;26:e922953. [Crossref] [PubMed]
- Monteoliva L, Albar JP. Differential proteomics: an overview of gel and non-gel based approaches. Brief Funct Genomic Proteomic 2004;3:220-39. [Crossref] [PubMed]
- Wei B, Huang X, Lu Y, et al. Screening of atrial fibrillation diagnostic markers based on a GEO database chip and bioinformatics analysis. J Thorac Dis 2022;14:4773-84. [Crossref] [PubMed]
- Robbins KA, Burkhardt C. Intra-and inter-platform renormalization and analysis of microarray data from the NCBI GEO database. 2007. Available online: http://venom.cs.utsa.edu/dmz/techrep/2007/CS-TR-2007-007.pdf
-
Cui Y Wang X Liu Q Integration of Gene Expression Profile Data of Human Epicardial Adipose Tissue From Postoperative Atrial Fibrillation to Verification of Hub Genes. 2021 . doi: . - Guan SF. The expression of glioma-related mRNA and signal pathways were analyzed by integrated bioinformatics analysis. Journal of China Prescription Drug 2019;17:10-2.
- Mayor C, Robinson L. Ontological realism, concepts and classification in molecular biology: development and application of the gene ontology. Journal of Documentation 2014;70:173-93.
- Zhang F, Zhang R, Zhang X, et al. Comprehensive analysis of circRNA expression pattern and circRNA-miRNA-mRNA network in the pathogenesis of atherosclerosis in rabbits. Aging (Albany NY) 2018;10:2266-83. [Crossref] [PubMed]
- Zhang Y, Zhang G, Liu Y, et al. GDF15 Regulates Malat-1 Circular RNA and Inactivates NFκB Signaling Leading to Immune Tolerogenic DCs for Preventing Alloimmune Rejection in Heart Transplantation. Front Immunol 2018;9:2407. [Crossref] [PubMed]
- Wang Y, Shang S, Yu K, et al. Table S2: Function enrichment analyses by the DAVID database. Peer J 2020; [Crossref]
- Langfelder P, Horvath S. WGCNA: an R package for weighted correlation network analysis. BMC Bioinformatics 2008;9:1-13. [Crossref] [PubMed]
- Shannon P, Markiel A, Ozier O, et al. Cytoscape: a software environment for integrated models of biomolecular interaction networks. Genome Res 2003;13:2498-504. [Crossref] [PubMed]
-
Zhang HY Zhou XL Zhang XM Grade-Specific Diagnosis, Prognostic and Therapeutic Biomarkers in Breast Cancer Identified by Integrated Bioinformatics Analysis. Res Sq 2021 . doi: . - Zhou Z, Sun B, Huang S, et al. Roles of circular RNAs in immune regulation and autoimmune diseases. Cell Death Dis 2019;10:503. [Crossref] [PubMed]
- Cine N, Limtrakul P, Sunnetci D, et al. Effects of curcumin on global gene expression profiles in the highly invasive human breast carcinoma cell line MDA-MB 231: A gene network-based microarray analysis. Exp Ther Med 2013;5:23-7. [Crossref] [PubMed]
-
Chen Q Jiang C Li J ALAD Expression and Prognostic Value in Multiple Human Cancers: A Bioinformatics Analysis. Res Sq 2021 . doi: . - Mao X, Chen C, Wang B, et al. A global bibliometric and visualized analysis in the status and trends of subchondral bone research. Medicine (Baltimore) 2020;99:e20406. [Crossref] [PubMed]
- Qin D, Mansour MC, Ruskin JN, et al. Atrial Fibrillation-Mediated Cardiomyopathy. Circ Arrhythm Electrophysiol 2019;12:e007809. [Crossref] [PubMed]
- Zou R, Zhang D, Lv L, et al. Bioinformatic gene analysis for potential biomarkers and therapeutic targets of atrial fibrillation-related stroke. J Transl Med 2019;17:45. [Crossref] [PubMed]
- Dossett ML, Needles EW, Donahue Z, et al. A SMART approach to reducing paroxysmal atrial fibrillation symptoms: Results from a pilot randomized controlled trial. Heart Rhythm O2 2021;2:326-32.
- Bischoff FR, Scheffzek K, Ponstingl H. How Ran is regulated. Results Probl Cell Differ 2002;35:49-66. [Crossref] [PubMed]
- Polyakova V, Miyagawa S, Szalay Z, et al. Atrial extracellular matrix remodelling in patients with atrial fibrillation. J Cell Mol Med 2008;12:189-208. [Crossref] [PubMed]
- Löfsjögård J, Persson H, Díez J, et al. Atrial fibrillation and biomarkers of myocardial fibrosis in heart failure. Scand Cardiovasc J 2014;48:299-303. [Crossref] [PubMed]
- Chen H, Zhang F, Zhang YL, et al. Relationship between circulating miRNA-21, atrial fibrosis, and atrial fibrillation in patients with atrial enlargement. Ann Palliat Med 2021;10:12742-9. [Crossref] [PubMed]
- Broersen K, Ruiperez V, Davletov B. Structural and Aggregation Properties of Alpha-Synuclein Linked to Phospholipase A2 Action. Protein Pept Lett 2018;25:368-78. [Crossref] [PubMed]
- Gai X, Tu K, Lu Z, et al. MRC2 expression correlates with TGFβ1 and survival in hepatocellular carcinoma. Int J Mol Sci 2014;15:15011-25. [Crossref] [PubMed]
- Yang X, Wu G, Yang F, et al. Elevated LINC00909 Promotes Tumor Progression of Ovarian Cancer via Regulating the miR-23b-3p/MRC2 Axis. Oxid Med Cell Longev 2021;2021:5574130. [Crossref] [PubMed]
- López-Guisa JM, Cai X, Collins SJ, et al. Mannose receptor 2 attenuates renal fibrosis. J Am Soc Nephrol 2012;23:236-51. [Crossref] [PubMed]
- Mascolo A, Scavone C, Rafaniello C, et al. Renin-Angiotensin System and Coronavirus Disease 2019: A Narrative Review. Front Cardiovasc Med 2020;7:143. [Crossref] [PubMed]
- Kashem SW, Haniffa M, Kaplan DH. Antigen-Presenting Cells in the Skin. Annu Rev Immunol 2017;35:469-99. [Crossref] [PubMed]
- Hirayama D, Iida T, Nakase H. The Phagocytic Function of Macrophage-Enforcing Innate Immunity and Tissue Homeostasis. Int J Mol Sci 2017;19:92. [Crossref] [PubMed]
- Liu YC, Zou XB, Chai YF, et al. Macrophage polarization in inflammatory diseases. Int J Biol Sci 2014;10:520-9. [Crossref] [PubMed]
- Arora S, Dev K, Agarwal B, et al. Macrophages: Their role, activation and polarization in pulmonary diseases. Immunobiology 2018;223:383-96. [Crossref] [PubMed]
- Liao X, Ruan X, Chen X, et al. The Long Noncoding RNA Gm9866/Nuclear Factor-κB Axis Promotes Macrophage Polarization. Mediators Inflamm 2023;2023:9991916. [Crossref] [PubMed]
- Das S, Reddy MA, Senapati P, et al. Diabetes Mellitus-Induced Long Noncoding RNA Dnm3os Regulates Macrophage Functions and Inflammation via Nuclear Mechanisms. Arterioscler Thromb Vasc Biol 2018;38:1806-20. [Crossref] [PubMed]
- Reddy MA, Amaram V, Das S, et al. lncRNA DRAIR is downregulated in diabetic monocytes and modulates the inflammatory phenotype via epigenetic mechanisms. JCI Insight 2021;6:e143289. [Crossref] [PubMed]
- Zhu X, Zhang X, Cong X, et al. ANGPTL4 Attenuates Ang II-Induced Atrial Fibrillation and Fibrosis in Mice via PPAR Pathway. Cardiol Res Pract 2021;2021:9935310. [Crossref] [PubMed]