Utilizing residential histories to assess environmental exposure and socioeconomic status over the life course among mesothelioma patients
Highlight box
Key findings
• We found that assessment of exposure to non-asbestos air toxics based solely on addresses at cancer diagnosis was underestimated for mesothelioma patients, and the misclassification increased at time points further back from the cancer diagnosis year.
What is known and what is new?
• Exposure misclassification resulting from estimates solely based on a single address has been widely recognized though not commonly assessed in cancer research.
• We examined the effect size of the misclassification of exposure to air toxics and socioeconomic status across the life course utilizing residential histories of 1,015 mesothelioma patients.
What is the implication, and what should change now?
• With geospatial data becoming more readily available, incorporating cancer patients’ residential history will lead to improved exposure and health risk estimates, and should be part of the modern cancer surveillance system.
Introduction
Mesothelioma is a rare cancer [incidence rate is about 1 per 100,000 persons per year in the United States (U.S.)] with long latency period of 20 to 30 years and poor prognosis (1-3). The median age of diagnosis is 72 years for the malignant pleural mesothelioma, the major form of mesothelioma, and the 5-year relative survival rate is only 12% (4). Workplace asbestos exposure is the dominant contributor to mesothelioma, though para-occupational (or take-home) exposure and environmental exposure to asbestos (e.g., residing in close proximity to asbestos sources) also play a critical role of mesothelioma development (5-9). Studies from mesothelioma registries in many countries have been instrumental in providing rich information, including specifically residential histories, to understand the impact of asbestos exposure at scale, and to investigate known and unknown asbestos exposure sources (8,10-12). A considerable portion (14–59%) of the mesothelioma cases lacks an identifiable source of asbestos exposure (13). While other non-asbestos exposures (e.g., tobacco smoke exposure), as well as lower socioeconomic status (SES), also contribute to the poor prognosis and survival (2,13-16), little is known about the epidemiology of non-asbestos related environmental exposure and SES factors among mesothelioma patients. Moreover, no study has reconstructed exposure history to non-asbestos related air toxics and SES based on residential history for mesothelioma patients.
It is well-recognized that the places we live throughout our lifetime are closely connected to our health (17-19). For cancer, exposures that are important to disease development may occur long before the manifestation of clinical symptoms and diagnosis (3,20,21). However, the conventional approach has been to link the exposure at the address at cancer diagnosis with cancer outcomes to study potential clustering of incidence cases, as well as to examine the potential risk factors (22,23). While this snapshot of address serves as a reasonable proxy for patient’s present socioeconomic, demographic, and environmental circumstances, it is subject to exposure misclassification, due to the underlying assumption of a constant exposure level throughout the entire time prior to patient’s cancer diagnosis.
To overcome this limitation, there has been a call for collecting and incorporating residential history in cancer epidemiological investigations (17,22,24-26). Researchers who have undertaken this task have typically done so through patient interviews or questionnaires (26-30). However, methodological and practical issues such as small sample size and recall bias have proven to be significant challenges (17). Some researchers were able to take advantage of the comprehensive national-level cancer registry and population health databases to obtain partial or complete residential history information (7,8,12,31-33), while others have used non-street-level address geographic information [e.g., coarse geography such as a county or zone improvement plan (ZIP) code] to estimate residential mobility (34-36).
In recent decades, the parallel advancement in electronic records keeping, geographic information system technology, and increasing computational power have accelerated the utilization of detailed residential history data (36-39). Indeed, studies have demonstrated the feasibility of using commercial databases to obtain multiple addresses of patients at scale (38-46). For example, residential history information has been used to increase the accuracy in estimating pesticide exposure among children linked to cancer in a population-based case-control study (42). Recently, studies have also suggested differential impact of SES on cancer survivorship between using the address at cancer diagnosis and the whole residential history post-cancer diagnoses (44,45).
Despite of these advances, few studies have systematically detailed methods on reconstructing a chronological exposure profile linked to residential history by combining multiple administrative and commercial data sources. In addition, extensive comparisons between alternative exposure measures estimated from patient’s residential history and that at cancer diagnosis address have been lacking. We addressed these research gaps in the current study using mesothelioma patients as an example. Specifically, we aimed to assess environmental exposure and SES across the life course utilizing residential histories of mesothelioma patients and to estimate the effect size of the exposure misclassification. We hypothesized that exposure to air toxics and SES at cancer diagnosis address would differ from those estimated from patients’ prior residential history. Quantification of the direction and magnitude of the exposure misclassification adds to the growing field of incorporating residential histories into cancer surveillance and epidemiological research. We present this article in accordance with the STROBE reporting checklist (available at https://jtd.amegroups.com/article/view/10.21037/jtd-23-533/rc).
Methods
Study population
In this retrospective observational case-only study, our population consisted of 1,015 mesothelioma cases diagnosed in 2011–2015 from the New York State (NYS) Cancer Registry. We used three databases to reconstruct patient’s residential history. Patient’s street-level address at the time of cancer diagnosis was collected in the registry database along with patient demographic and cancer characteristics as part of the routine cancer surveillance. We also used address information at patient’s hospitalizations collected in the health insurance claims for the years 1982–2019 available in the New York Statewide Planning and Research Cooperative System (SPARCS) (47). Finally, we used the commercial database from LexisNexis to obtain multiple street-level addresses for the same individual over time. Previous studies have shown LexisNexis’s ability to provide reliable residential history information (38,42,43,45).
The study was conducted in accordance with the Declaration of Helsinki (as revised in 2013). The study was approved by the Institution Review Board at the NYS Department of Health (#1498055-1) and that at the Icahn School of Medicine at Mount Sinai (IRB-19-02514). Informed consent was not required, as NYS Cancer Registry collects data under the Public Health Law Section 2401 and uses them for cancer surveillance, public health planning and evaluation, and research.
Address processing
Out of 5,795 unique address texts [excluding addresses containing ZIP code only or postal office (PO) box], we successfully geocoded 5,696 (98.3%) valid address texts using three geocoders: the Automated Geospatial Geocoding Interface Environment system, which is a powerful geocoding platform for open use by cancer registries in the U.S. (48,49), Google maps, and the Census Geocoder. Then we deduplicated the geocoded address texts. That is when two unique address texts were geocoded to the same latitude and longitude coordinates (or within 30 m), we regarded them as one address location. In addition, we included only geolocations where patients had resided up to their cancer diagnoses. Because the exposure data (details below) were only available at census tract level, we then mapped up each address to the corresponding census tract.
We used the first known date associated with a unique address as the starting time of this address, and used the start time of the next address in chronologically order as the end of the previous address. If only a single point address was available as the last address, we assumed a duration of 2.2 years, which was the median length of residency at an address among the study population. We conducted this process separately for each address data source, which allowed the direct comparison of residential history information (e.g., the total number of unique addresses) within each data source. We then compiled addresses from all three data sources into one, and updated the starting and ending time of an address based on the earliest and latest dates available, respectively.
Exposure data
For environmental exposure, we used estimates from the National Air Toxics Assessment (NATA) provided by the U.S. Environmental Protection Agency, which is a modeled lifetime cancer risk from inhalation of non-asbestos air toxins, and takes into account emission source types, meteorological conditions, and human activity patterns (50). In addition, the NATA data have the advantage of being a summary measure of exposure to a mixture of air toxics, which may better reflect the lifetime cancer risk. The national percentile ranking was available at census tract level for calendar years 1996, 1999, 2002, 2005, 2011, and 2014. We matched the time of census tracts lived with the closest NATA years available (Figure S1).
For SES exposure, we used the Yost index percentile ranking at census tract level. Yost index is a composite SES indicator commonly used in cancer epidemiology. It is consisted of seven census variables, including measures of income, house value, rent, poverty, education, employment, and occupation (51,52). We calculated four sets of Yost index according to the published methods using the 1990 and 2000 decennial census data, as well as the 5-year estimates from the 2009–2013 and 2014–2018 U.S. Census American Community Survey (51-53). Similar to air toxic exposure estimates, we matched the time of census tracts lived to the closest Yost index years available (Figure S1).
Approximately 2.4% of the tracts (n=125) had missing data for either air toxic exposure or SES. We ran an imputation analysis with all available cancer and socioeconomic data to fill in these missing data points (54).
Relative exposure (RE)
As NATA methods (e.g., the number and types of pollutants and models used) have changed overtime, a direct comparison of the NATA estimates (including the metric of cancer risk) across years was not appropriate (50). To overcome this inherent limitation of the NATA data, we exploited the concept of relative change, specifically, a relative measure in reference to the NYS average. The RE for non-asbestos air toxics (REcan) was calculated by dividing the NATA percentile ranking of individual census tracts by the average percentile ranking for NYS and subtracting one. Similarly, we calculated the RE for SES (REses), where a positive or negative value indicated a lower or higher SES than the NYS average, respectively. In addition, both NATA estimates and Yost index for SES served as composite indicators, rather than a specific air pollutant or a particular socioeconomic factor. For brevity, we referred to these two RE measures as REcan and REses, respectively, thereafter.
REcan and REses across patient’s residential history
We calculated the time-weighted-average (TWA) for REcan and REses based on the durations and RE levels associated with individual census tracts lived. If a patient lived simultaneously at multiple tracts (e.g., those who maintained multiple living quarters for various reasons), we evenly split the overlapping time among these tracts. We calculated the overall TWA and simple average REcan and REses from all tracts lived, as well as REcan and REses at the first known tract, tract with the longest residency, tract with the minimum RE, and the tract with the maximum RE, respectively. In addition, we calculated the yearly TWA REcan and REses for observational look-back windows spanning 5, 10, 15, 20, and 30 years prior to the patient’s cancer diagnosis year. For patients who lived in a single address during an entire year, the weight used in calculating the yearly TWA was essentially 1, while for patients who lived in multiple addresses in a year, the sum of the weights from these addresses summed to 1. Thus, the yearly TWA was based on overall tracts lived within a specific 1-year time frame, while the overall TWA was calculated using all tracts lived throughout patient’s residential history.
Statistical analyses
We summarized REcan and REses by patient characteristics with frequency and proportion for categorical variables and with median [interquartile range (IQR)] for continuous variables. Using Wilcoxon signed-rank tests, we compared REcan or REses estimated at diagnosis tract with six alternative measures estimated using (I) the first known tract; (II) tract with the longest residency; (III) tract with the minimum RE; (IV) tract with the maximum RE; (V) the simple-average from all tracts; and (VI) the overall TWA. In addition, standard mean differences (SMDs), a measure of effect size, were calculated. The relationships among REcan, REses, and other continuous variables were also examined using the Spearman’s rank correlation.
We compared the yearly TWA during the cancer diagnosis year with those during individual years prior to cancer diagnosis for a given look-back window. We used a generalized linear model with general estimated equation (GEE) to take into account the autocorrelation within individuals across repeated measures. Separate models were used for REcan and REses. The dependent variable was the yearly TWA, while the main independent or explanatory variable was years prior to cancer diagnosis. We treated time as a categorical variable, and the alpha level for statistical significance was Bonferroni adjusted to account for multiple testing. Alternatively, we also treated years to cancer diagnosis as a continuous variable in the model in order to estimate the annual change in REcan and REses. All models were adjusted for patient’s age at cancer diagnosis, sex, race, Hispanic ethnicity, cancer stage, and tobacco use status. The analyses were conducted using SAS (V9.4) and R (V4.0.2) in RStudio (V2022.02.03).
Results
Overall patient characteristics
As shown in Table 1, the majority of the 1,015 mesothelioma patients were male (75.0%) and White (91.4%). At the time of cancer diagnosis, patients had a median age of 75 (IQR, 65–81) years, and the majority (64.9%) of them were diagnosed with a distant-stage tumor.
Table 1
Variables | Overall | Years prior to cancer diagnosis | ||||
---|---|---|---|---|---|---|
5-year | 10-year | 15-year | 20-year | 30-year | ||
Total | 1,015 (100.0) | 974 (96.0) | 952 (93.8) | 913 (90.0) | 839 (82.7) | 444 (43.7) |
Age at diagnosis (years) | 75 [65–81] | 75 [66–82] | 75 [66–82] | 75 [66–82] | 76 [67–82] | 76 [68–82] |
Sex | ||||||
Male | 761 (75.0) | 734 (75.4) | 716 (75.2) | 690 (75.6) | 635 (75.7) | 343 (77.3) |
Female | 254 (25.0) | 240 (24.6) | 236 (24.8) | 223 (24.4) | 204 (24.3) | 101 (22.7) |
Race | ||||||
White | 928 (91.4) | 894 (91.8) | 878 (92.2) | 853 (93.4) | 789 (94.0) | 417 (93.9) |
Black | 51 (5.0) | 49 (5.0) | 47 (4.9) | 42 (4.6) | 39 (4.6) | 22 (5.0) |
Other | 36 (3.5) | 31 (3.2) | 27 (2.8) | 18 (2.0) | 11 (1.3) | 5 (1.1) |
Non-Hispanic ethnicity | 958 (94.4) | 924 (94.9) | 905 (95.1) | 872 (95.5) | 804 (95.8) | 423 (95.3) |
Stage | ||||||
Local | 97 (9.6) | 93 (9.5) | 92 (9.7) | 87 (9.5) | 78 (9.3) | 41 (9.2) |
Regional | 161 (15.9) | 153 (15.7) | 149 (15.7) | 145 (15.9) | 129 (15.4) | 72 (16.2) |
Distant | 659 (64.9) | 636 (65.3) | 620 (65.1) | 593 (65.0) | 552 (65.8) | 287 (64.6) |
Unstaged/unknown | 98 (9.7) | 92 (9.4) | 91 (9.6) | 88 (9.6) | 80 (9.5) | 44 (9.9) |
Tobacco product use | ||||||
Current | 116 (11.4) | 111 (11.4) | 105 (11.0) | 95 (10.4) | 86 (10.3) | 42 (9.5) |
Former | 482 (47.5) | 468 (48.0) | 459 (48.2) | 446 (48.8) | 424 (50.5) | 230 (51.8) |
Never | 334 (32.9) | 319 (32.8) | 312 (32.8) | 298 (32.6) | 263 (31.3) | 131 (29.5) |
Unknown | 83 (8.2) | 76 (7.8) | 76 (8.0) | 74 (8.1) | 66 (7.9) | 41 (9.2) |
Number of unique addresses | 4,602 | 1,817 | 2,365 | 2,782 | 2,959 | 2,027 |
Unique addresses lived per patient | 4 [2–6] | 1 [1–2] | 2 [1–3] | 2 [1–4] | 3 [2–5] | 4 [2–6] |
Duration lived at each address in years | 2.2 [1.0–8.3] | 2.2 [1.0–8.5] | 2.2 [1.0–8.7] | 2.2 [1.0–8.9] | 2.3 [1.1–6.7] | 2.6 [1.1–9.8] |
Lived only in one address | 140 (13.8) | 122 (12.0) | 115 (11.3) | 106 (10.4) | 84 (8.3) | 35 (3.4) |
Average Euclidean distance (km) moved between addresses lived | 12.1 [1.7–193.9] |
12.5 [2.1–199.8] |
12.7 [2.3–202.0] |
13.3 [2.6–214.4] |
14.9 [2.9–238.5] |
19.4 [4.4–238.5] |
TWA REcan | −0.11 [−0.50 to 0.30] |
−0.11 [−0.50 to 0.29] |
−0.11 [−0.49 to 0.29] |
−0.13 [−0.50 to 0.27] |
−0.12 [−0.49 to 0.26] |
−0.01 [−0.41 to 0.33] |
TWA REses | −0.28 [−0.65 to 0.25] |
−0.28 [−0.65 to 0.24] |
−0.28 [−0.65 to 0.23] |
−0.30 [−0.66 to 0.21] |
−0.33 [−0.66 to 0.19] |
−0.47 [−0.74 to −0.004] |
Data are presented as n, median [IQR], or n (%). REcan was based on the cancer risk from the NATA data, and REses was based on Yost index derived from the Census data. To match NATA and Yost index data at census tract level, we coded each address by its census tract. RE was used for comparability across time, which was calculated by dividing the NATA percentile ranking or Yost index percentile ranking at individual census tracts by the average percentile ranking for NYS and subtracting one. The relative measure was compared to the average level of exposures in NYS. We calculated TWA of REcan and REses for observational look-back windows spanning 5, 10, 15, 20, and 30 years prior to the patient’s cancer diagnosis year. TWA, time-weighted-average; REcan, relative exposure for non-asbestos air toxics; REses, relative exposure for socioeconomic status; IQR, interquartile range; NATA, National Air Toxics Assessment; RE, relative exposure; NYS, New York State.
We identified 4,602 unique address points among the entire study population from all three data sources (Table 1), of which 860 addresses (18.9%) were in common for all three data sources (Figure S2). We found that 3,793 (82.4%) addresses were in NYS, which spanned 2,140 census tracts. The remaining addresses were in other 41 states, encompassing 705 census tracts. The residential history of the study sample covered the period from 1953 to 2015. The median number of unique addresses lived by the patients was 4 (IQR, 2–6) addresses, and the median time lived in an address was 2.2 (IQR, 1.0–8.3) years. Approximately 14% of the patients only lived in one address (i.e., non-movers), and the median distance moved among the entire study population was 12.1 (IQR, 1.7–193.9) km (Table 1).
The overall TWA REcan of patients had a median of −0.11 (IQR, −0.50 to 0.30), and the overall TWA REses had a median of −0.28 (IQR, −0.65 to 0.25) (Table 1). There was a weak inverse correlation between the two TWA measures according to the Spearman’s correlation coefficient (ρ=−0.09, P=0.012, Figure S3). The total residential time was positively associated with the overall TWA REcan (ρ=0.12, P<0.001), while negatively associated with overall TWA REses (ρ=−0.39, P<0.001, Figure S3).
When the look-back time window increased from 5 to 30 years prior to the cancer diagnosis, the proportion of patients with available residential history data decreased from 96.0% (n=974) to 43.7% (n=444) of the entire sample, while the number of unique addresses identified increased from 1,817 to 2,027 (Table 1). The distribution of patient-level characteristics across subgroups defined by the retrospective observation time window remained largely similar to those found in the entire study sample (Table 1), with some notable differences. With a longer residential history available spanning from 5- to 30-year, the average moving distance increased from 12.5 to 19.4 km, while the proportion of non-movers decreased from 13.8% to 3.4%. In addition, those with up to a 30-year residential history had lower REcan and REses.
Comparisons between REcan and REses at the cancer diagnosis address and alternative measures from patient residential histories
REcan at cancer diagnosis tract differed significantly from those measured by the six alternative indicators (P<0.01, Figure 1). However, the effect size as measured by SMD ranged from small (0.04, Table S1) to moderate (0.50). Similar patterns were found for REses in nearly all comparisons (P<0.01, Figure 1), with small (0.03, Table S1) to moderate (0.77) SMD. We found moderate (ρ=0.77, Figure S4) to strong (0.92) positive correlations between REcan at diagnosis tracts and the other six alternative measures. A similar but slightly attenuated correlation were found for REses with ρ ranging from 0.63 to 0.86 (Figure S4).
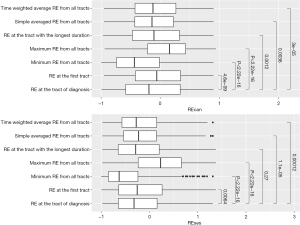
Comparisons between yearly REcan and REses at cancer diagnosis and proceeding years
Figure 2 presents the comparisons of yearly TWA measures within a retrospective observation window ranging from 5 to 30 years prior to the year of cancer diagnosis. REcan in the preceding years tended to be higher than that of the diagnosis year, as indicated by the positive beta coefficients [and their 95% confidence interval (CI)] from the regression model. However, the effect size was relatively small, with the upper 95% CI below 0.15% or 15% (Figure 2). In addition, REcan was not significantly elevated for all individual years prior to cancer diagnosis. We also found that the magnitude of the differences in the yearly TWA at cancer diagnosis and proceeding years tended to be larger at time points further away from the cancer diagnosis year (Figure 2). Finally, in the subgroup of patients (n=444) who had 30-year residential history prior to cancer diagnosis, significantly higher yearly REcan was only observed in the 21–30 years prior to the cancer diagnosis year.
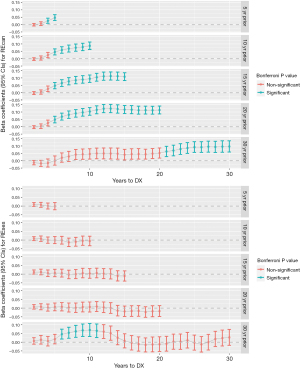
The trend of yearly REses over different observational time windows were similar in general, which showed limited differences (not statistically significant) over time (Figure 2). One exception was the subgroup of patients with 30-year residential history, where yearly REses during the 6–11 years prior to cancer diagnosis was found to be significantly higher than that during the diagnosis year.
When time was treated as a continuous variable, we found a similar trend of higher yearly REcan in years prior to patient’s cancer diagnosis (Figure 3). For example, for the 5-year prior to cancer diagnosis time window (n=974), with each increase year proceeding the diagnosis year, the REcan increased by 1.24% (95% CI: 0.71–1.77%), and by 0.36% (95% CI: 0.25–0.48%) for the 30-year look-back window (n=444). It is important to note that because of the sample size difference (e.g., only 43.7% of the patients had a 30-year residential history), a direct comparison of the findings across different look-back time windows (e.g., 1.24% vs. 0.36%) may not be appropriate. The differences of yearly REses were not statistically significant for any of the five retrospective-observational-window scenarios.
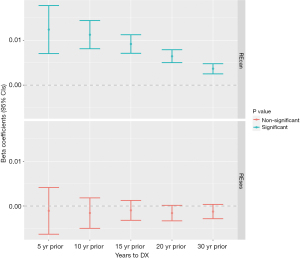
Discussion
Using residential histories from multiple data sources, we reconstructed exposure history associated with non-asbestos related air toxics and sociodemographic status among 1,015 mesothelioma cases reportable to NYS Cancer Registry between 2011 and 2015. We compared RE measures (REcan and REses) at the cancer diagnosis address with six other alternative measures from patient residential histories. Further, we investigated the longitudinal changes of patient’s RE measures and quantified the extent of exposure misclassification using cross-sectional and longitudinal estimates.
We found that the study population tended to have a higher REcan in earlier addresses than that at the addresses at cancer diagnosis. This main finding confirmed the long-suspected concern about misclassification of environmental exposure based only on a single address at the time of cancer diagnosis, though the direction and the magnitude of the misclassification have not been well studied. It is unsurprising that the assumption of constant exposure was violated, as our data showed that mesothelioma patients had lived in a median number of 4 unique addresses, and residential mobility increased with longer residential history studied. Residential mobility in the general U.S. population and cancer population before and after diagnosis have been previously documented (34-36,42,45,55). For example, in a population-based case-control study of bladder cancer patients diagnosed between 2000 and 2004 in Michigan, participants lived 9 addresses during an average of 65-year long residential history (27). In the Iowa Women’s Health Study, 32% of the participants moved at least once during 19 years of follow-up between 1986 and 2004 (56).
Extending the existing literature, we further quantified the degree of the exposure misclassification between approaches with and without considering residential histories. Our analysis suggests that the identified misclassification may be limited, as the observed differences in REcan during years preceding the cancer diagnosis were no more than 15% higher than the RE during the diagnosis year, and yearly increment ranged from 0.2% to 1.8%, based on the 95% CIs. In addition, we found the effect size, measured by SMD, ranged from small to moderate, when comparing REcan and REses at cancer diagnosis address and six alternative measures.
We also found moderate to strong positive correlations between REcan or REses at the diagnosis tract and alternative measures based on residential histories. This result was consistent with other findings. For example, Ling et al. [2019] found moderate to strong Spearman’s correlations (ρ=0.76–0.83) between pesticide exposures based on cancer diagnosis address, birth address, and residential addresses during the first year of life among a population-based case-control pediatric cancer population in California (42). Strong correlations between exposure estimates in successive addresses, with full residential histories or a single address were also reported for childhood leukemia cases in a nationwide Finnish case-control study (33). In a large cohort study examining the association between cancer and nutrition in Sweden, the investigators found that the correlation between NOx concentrations at the enrollment address and the average concentration over the entire follow-up period (an average of 14.6 years) was 0.80 (57).
Our analysis showed that not all earlier residential times prior to the cancer diagnosis had statistically significant higher REcan than that at the cancer diagnosis year, and the magnitude of the difference in the yearly REcan at cancer diagnosis and proceeding years tended to be larger at time points further away from the cancer diagnosis year. Similar findings were reported by a simulation study based on data from the Health-AARP Diet and Health Study (58). Interestingly, among the subset of our study population with 30-year residential history records, we found that significantly elevated REcan occurred in the earliest decade, which was equivalent to an age group of 46–55 years (assuming a median age at diagnosis of 76 years). Meanwhile, significantly elevated REses occurred in the 6–13 years prior to cancer diagnosis, which was equivalent to an age group of 65–70 years. These results suggest that the time window of high exposure for REcan may differ from that for REses. One explanation of this discrepancy is likely their differences in the spatial and temporal variability, as we would expect exposures that have a larger variation across space and time would suffer a larger misclassification bias when a single address rather than multiple addresses was used to estimate exposures. Indeed, studies have found that different exposure measures (e.g., different pollutants, greenspace, or agriculture land) with different spatiotemporal characteristics exhibited varied magnitudes of exposure misclassification associated with residential mobility (56,58,59).
Our results should be viewed in light of their limitations. First, our findings may be unique to the mesothelioma patients studied. In general, we found the current study population tended to live in areas where both REcan and REses were lower than the NYS average. As NYS is a large multicultural populous state, it remains to be confirmed that if the same trend would hold true for mesothelioma patients diagnosed in a different period in NYS or in other states, since migration patterns may differ over time and/or among people residing in another state. In addition, patients with different cancer types may also have a different REcan and REses pattern than mesothelioma patients. Second, while we were able to identify multiple addresses for individual patients, their residential history may still be incomplete due to the inherent limitations in the mechanisms through which an organization/company collects the data. In addition, studies have shown that the availability of residential history varied by patient’s sociodemographic characteristics (39,40,42,46). Inclusion of other address data sources may improve the completeness of residential history, thus improve the estimate of the duration at each address and the subsequent exposure estimates. Third, our exposure assessment was limited to the availability of exposure data in terms of the time and geographic resolution. It is also important to note that we used a relative rather than an absolute measure of exposure to examine the exposure misclassification. In addition, Yost index was a composite measure based on seven individual census variables, and may not be a comprehensive measure of neighborhood SES (51,53). For two addresses, where one lived by a patient in 1996 and the other in 2014, while both RE may be 10% higher than the corresponding NYS average level, the earlier address may have a higher absolute exposure, as the NYS average ranking was higher in 1996 than in 2014 (69th percentile vs. 51st percentile). The choice of using REcan in the current analysis was due to the incomparability of NATA data over time. Such a constraint can be potentially avoided by using exposure measures that are generated consistently over time. However, this kind of measure may not be readily available, especially if it has to be consistently measured over a long period spanning from the 1950s to the current decade as seen in our study. These imprecisions may have obscured the estimate of the effect size of the exposure misclassification to some degree. These issues may be potentially mitigated among younger patients or patients diagnosed in more recent years, as data for environmental pollutants and socioeconomic risk factors, as well as the available residential history records, have become increasingly available with refined spatiotemporal resolutions.
Conclusions
We found that the assessment of exposure to non-asbestos air toxic based solely on the address at cancer diagnosis was underestimated among mesothelioma patients from a large population-based central cancer registry, and the misclassification increased at time points further away from the cancer diagnosis year. In addition, the type of exposure (e.g., air-toxic exposure vs. SES exposure) and the length of the residential history (e.g., 5- vs. 30-year) may affect the exposure misclassification. Overall, we found a relatively small effect size in exposure misclassification between estimates with and without incorporating patient’s residential history information. In addition, the availability of longitudinal exposure patterns based on patient residential history may have important implications, such as tracking the inducement and survival conditions for mesothelioma patients, investigating susceptible time window and latency period, studying disease development of mesothelioma and different cancer types. While the current study was a case-only design and specific to mesothelioma which has a known etiology, our methods can be applied to future studies with comparison groups to further study mesothelioma patients, as have been shown in other studies based on population-level mesothelioma registries where residential history information is available, as well as other cancer types (7-9,11,12,31-33). With increasingly available geocoding and geospatial analytical tools, as well as refined data on environmental and socioeconomic factors, utilization of patients’ residential history as opposed to single address at diagnosis will lead to improved exposure and health risk estimates. Moreover, the augmentation of residential history information into current cancer epidemiological studies will facilitate the investigation of the potential critical time window that are important to the natural history of cancer development. Such research advancement can benefit and should become an integral part of the modern cancer surveillance system.
Acknowledgments
The authors would like to thank Dr. Francis Boscoe (Pumphandle, LLC, Camden, ME, USA) for his contribution to the project.
Funding: This work was supported in part by a grant from
Footnote
Reporting Checklist: The authors have completed the STROBE reporting checklist. Available at https://jtd.amegroups.com/article/view/10.21037/jtd-23-533/rc
Data Sharing Statement: Available at https://jtd.amegroups.com/article/view/10.21037/jtd-23-533/dss
Peer Review File: Available at https://jtd.amegroups.com/article/view/10.21037/jtd-23-533/prf
Conflicts of Interest: All authors have completed the ICMJE uniform disclosure form (available at https://jtd.amegroups.com/article/view/10.21037/jtd-23-533/coif). All authors report that this work was supported in part by a grant from the National Cancer Institute (1R21CA235153). F.F.L. reports that the NYS Cancer Registry was supported in part by the Centers for Disease Control and Prevention’s National Program of Cancer Registries through cooperative agreement 6NU58DP006309 awarded to the New York State Department of Health and by Contract 75N91018D00005 (Task Order 75N91018F00001) from the National Cancer Institute, National Institutes of Health. The authors have no other conflicts of interest to declare.
Ethical Statement: The authors are accountable for all aspects of the work in ensuring that questions related to the accuracy or integrity of any part of the work are appropriately investigated and resolved. The study was conducted in accordance with the Declaration of Helsinki (as revised in 2013). The study was approved by the Institution Review Board at the New York State (NYS) Department of Health (#1498055-1) and that at the Icahn School of Medicine at Mount Sinai (IRB-19-02514). Informed consent was not required, as NYS Cancer Registry collects data under the Public Health Law Section 2401 and uses them for cancer surveillance, public health planning and evaluation, and research.
Open Access Statement: This is an Open Access article distributed in accordance with the Creative Commons Attribution-NonCommercial-NoDerivs 4.0 International License (CC BY-NC-ND 4.0), which permits the non-commercial replication and distribution of the article with the strict proviso that no changes or edits are made and the original work is properly cited (including links to both the formal publication through the relevant DOI and the license). See: https://creativecommons.org/licenses/by-nc-nd/4.0/.
References
- Centers for Disease Control and Prevention. Incidence of Malignant Mesothelioma, 1999-2018. USCS Data Brief, No. 27. 2022. Available online: https://www.cdc.gov/cancer/uscs/about/data-briefs/no27-incidence-malignant-mesothelioma-1999-2018.htm
- Selikoff IJ, Hammond EC, Churg J. Asbestos exposure, smoking, and neoplasia. JAMA 1968;204:106-12.
- Selikoff IJ, Hammond EC, Seidman H. Latency of asbestos disease among insulation workers in the United States and Canada. Cancer 1980;46:2736-40. [Crossref] [PubMed]
- American Cancer Society. Malignant Mesothelioma. 2020. Available online: https://www.cancer.org/cancer/types/malignant-mesothelioma.html
- Vimercati L, Cavone D, Delfino MC, et al. Asbestos Air Pollution: Description of a Mesothelioma Cluster Due to Residential Exposure from an Asbestos Cement Factory. Int J Environ Res Public Health 2020;17:2636. [Crossref] [PubMed]
- Vimercati L, Cavone D, Lovreglio P, et al. Environmental asbestos exposure and mesothelioma cases in Bari, Apulia region, southern Italy: a national interest site for land reclamation. Environ Sci Pollut Res Int 2018;25:15692-701. [Crossref] [PubMed]
- Marinaccio A, Corfiati M, Binazzi A, et al. The epidemiological surveillance of malignant mesothelioma in Italy (1993-2015): methods, findings, and research perspectives. Epidemiol Prev 2020;44:23-30. [Crossref] [PubMed]
- Magnani C, Mensi C, Binazzi A, et al. The Italian Experience in the Development of Mesothelioma Registries: A Pathway for Other Countries to Address the Negative Legacy of Asbestos. Int J Environ Res Public Health 2023;20:936. [Crossref] [PubMed]
- Kitamura Y, Zha L, Liu R, et al. Association of mesothelioma deaths with neighborhood asbestos exposure due to a large-scale asbestos-cement plant. Cancer Sci 2023;114:2973-85. [Crossref] [PubMed]
- Lysaniuk B, Cely-García MF, Mazzeo A, et al. Where are the landfilled zones? Use of historical geographic information and local spatial knowledge to determine the location of underground asbestos contamination in Sibaté (Colombia). Environ Res 2020;191:110182. [Crossref] [PubMed]
- Gaitens JM, Culligan M, Friedberg JS, et al. Laying the Foundation for a Mesothelioma Patient Registry: Development of Data Collection Tools. Int J Environ Res Public Health 2023;20:4950. [Crossref] [PubMed]
- Petrof O, Neyens T, Nuyts V, et al. On the impact of residential history in the spatial analysis of diseases with a long latency period: A study of mesothelioma in Belgium. Stat Med 2020;39:3840-66. [Crossref] [PubMed]
- Liu B, van Gerwen M, Bonassi S, et al. Epidemiology of Environmental Exposure and Malignant Mesothelioma. J Thorac Oncol 2017;12:1031-45. [Crossref] [PubMed]
- McDonald JC, McDonald AD. The epidemiology of mesothelioma in historical context. Eur Respir J 1996;9:1932-42. [Crossref] [PubMed]
- Freudenberger DC, Shah RD. A narrative review of the health disparities associated with malignant pleural mesothelioma. J Thorac Dis 2021;13:3809-15. [Crossref] [PubMed]
- Alnajar A, Kareff SA, Razi SS, et al. Disparities in Survival Due to Social Determinants of Health and Access to Treatment in US Patients With Operable Malignant Pleural Mesothelioma. JAMA Netw Open 2023;6:e234261. [Crossref] [PubMed]
- Boscoe FP. The use of residential history in environmental health studies. Geospatial Analysis of Environmental Health 2011;4:93-110.
- Diez Roux AV, Mair C. Neighborhoods and health. Ann N Y Acad Sci 2010;1186:125-45. [Crossref] [PubMed]
- Marmot M. Social justice, epidemiology and health inequalities. Eur J Epidemiol 2017;32:537-46. [Crossref] [PubMed]
- Jacquez GM, Meliker J, Kaufmann A. In search of induction and latency periods: space-time interaction accounting for residential mobility, risk factors and covariates. Int J Health Geogr 2007;6:35. [Crossref] [PubMed]
- Rodgers KM, Udesky JO, Rudel RA, et al. Environmental chemicals and breast cancer: An updated review of epidemiological literature informed by biological mechanisms. Environ Res 2018;160:152-82. [Crossref] [PubMed]
- Schootman M, Gomez SL, Henry KA, et al. Geospatial Approaches to Cancer Control and Population Sciences. Cancer Epidemiol Biomarkers Prev 2017;26:472-5. [Crossref] [PubMed]
- Bell BS, Hoskins RE, Pickle LW, et al. Current practices in spatial analysis of cancer data: mapping health statistics to inform policymakers and the public. Int J Health Geogr 2006;5:49. [Crossref] [PubMed]
- Jacquez GM, Essex A, Curtis A, et al. Geospatial cryptography: enabling researchers to access private, spatially referenced, human subjects data for cancer control and prevention. J Geogr Syst 2017;19:197-220. [Crossref] [PubMed]
- Pickle LW, Szczur M, Lewis DR, et al. The crossroads of GIS and health information: a workshop on developing a research agenda to improve cancer control. Int J Health Geogr 2006;5:51. [Crossref] [PubMed]
- Meliker JR, Jacquez GM. Space-time clustering of case-control data with residential histories: insights into empirical induction periods, age-specific susceptibility, and calendar year-specific effects. Stoch Environ Res Risk Assess 2007;21:625-34. [Crossref] [PubMed]
- Meliker JR, Slotnick MJ. Lifetime exposure to arsenic in drinking water and bladder cancer: a population-based case-control study in Michigan, USA. Cancer Causes Control 2010;21:745-57. [Crossref] [PubMed]
- Miller A, Siffel C, Correa A. Residential mobility during pregnancy: patterns and correlates. Matern Child Health J 2010;14:625-34. [Crossref] [PubMed]
- Pronk A, Nuckols JR, De Roos AJ, et al. Residential proximity to industrial combustion facilities and risk of non-Hodgkin lymphoma: a case-control study. Environ Health 2013;12:20. [Crossref] [PubMed]
- Wheeler DC, De Roos AJ, Cerhan JR, et al. Spatial-temporal analysis of non-Hodgkin lymphoma in the NCI-SEER NHL case-control study. Environ Health 2011;10:63. [Crossref] [PubMed]
- Hystad P, Carpiano RM, Demers PA, et al. Neighbourhood socioeconomic status and individual lung cancer risk: evaluating long-term exposure measures and mediating mechanisms. Soc Sci Med 2013;97:95-103. [Crossref] [PubMed]
- Sloan CD, Nordsborg RB, Jacquez GM, et al. Space-time analysis of testicular cancer clusters using residential histories: a case-control study in Denmark. PLoS One 2015;10:e0120285. [Crossref] [PubMed]
- Nikkilä A, Kendall G, Raitanen J, et al. Effects of incomplete residential histories on studies of environmental exposure with application to childhood leukaemia and background radiation. Environ Res 2018;166:466-72. [Crossref] [PubMed]
- Liu B, Lee FF, Boscoe F. Residential mobility among adult cancer survivors in the United States. BMC Public Health 2020;20:1601. [Crossref] [PubMed]
- Muralidhar V, Nguyen PL, Tucker-Seeley RD. Recent relocation and decreased survival following a cancer diagnosis. Prev Med 2016;89:245-50. [Crossref] [PubMed]
- Namin S, Zhou Y, McGinley E, et al. Residential history in cancer research: Utility of the annual billing ZIP code in the SEER-Medicare database and mobility among older women with breast cancer in the United States. SSM Popul Health 2021;15:100823. [Crossref] [PubMed]
- Sahar L, Foster SL, Sherman RL, et al. GIScience and cancer: State of the art and trends for cancer surveillance and epidemiology. Cancer 2019;125:2544-60. [Crossref] [PubMed]
- Stinchcomb DG, Roeser A. NCI/SEER Residential History Project Technical Report. 2016. Available online: https://www.westat.com/wp-content/uploads/legacy/NCISAS/NCI_Res_Hist_Proj_Tech_Rpt_v2sec.pdf
- Hurley S, Hertz A, Nelson DO, et al. Tracing a Path to the Past: Exploring the Use of Commercial Credit Reporting Data to Construct Residential Histories for Epidemiologic Studies of Environmental Exposures. Am J Epidemiol 2017;185:238-46. [Crossref] [PubMed]
- Hurley SE, Reynolds P, Goldberg DE, et al. Residential mobility in the California Teachers Study: implications for geographic differences in disease rates. Soc Sci Med 2005;60:1547-55. [Crossref] [PubMed]
- Jacquez GM, Barlow J, Rommel R, et al. Residential mobility and breast cancer in Marin County, California, USA. Int J Environ Res Public Health 2013;11:271-95. [Crossref] [PubMed]
- Ling C, Heck JE, Cockburn M, et al. Residential mobility in early childhood and the impact on misclassification in pesticide exposures. Environ Res 2019;173:212-20. [Crossref] [PubMed]
- Wheeler DC, Wang A. Assessment of Residential History Generation Using a Public-Record Database. Int J Environ Res Public Health 2015;12:11670-82. [Crossref] [PubMed]
- Wiese D, Stroup AM, Maiti A, et al. Socioeconomic Disparities in Colon Cancer Survival: Revisiting Neighborhood Poverty Using Residential Histories. Epidemiology 2020;31:728-35. [Crossref] [PubMed]
- Wiese D, Stroup AM, Maiti A, et al. Residential Mobility and Geospatial Disparities in Colon Cancer Survival. Cancer Epidemiol Biomarkers Prev 2020;29:2119-25. [Crossref] [PubMed]
- Brooks MS, Bennett A, Lovasi GS, et al. Matching participant address with public records database in a US national longitudinal cohort study. SSM Popul Health 2021;15:100887. [Crossref] [PubMed]
- New York State Department of Health. Statewide Planning and Research Cooperative System (SPARCS). 2022. Available online: https://www.health.ny.gov/statistics/sparcs/
- IMS. SEER*DMS Users Manual, Overview of SEER*DMS Geocoding. 2018. Available online: https://www.imsusersupport.com/seerdms/users-manual/overview-of-seerdms/geocoding
- Goldberg DW, Wilson JP, Knoblock CA, et al. An effective and efficient approach for manually improving geocoded data. Int J Health Geogr 2008;7:60. [Crossref] [PubMed]
- United States Environmental Protection Agency. National Air Toxics Assessment. 2022. Available online: https://www.epa.gov/national-air-toxics-assessment
- Yu M, Tatalovich Z, Gibson JT, et al. Using a composite index of socioeconomic status to investigate health disparities while protecting the confidentiality of cancer registry data. Cancer Causes Control 2014;25:81-92. [Crossref] [PubMed]
- Yost K, Perkins C, Cohen R, et al. Socioeconomic status and breast cancer incidence in California for different race/ethnic groups. Cancer Causes Control 2001;12:703-11. [Crossref] [PubMed]
- Boscoe FP, Liu B, Lee F. A comparison of two neighborhood-level socioeconomic indexes in the United States. Spat Spatiotemporal Epidemiol 2021;37:100412. [Crossref] [PubMed]
- Su YS, Gelman A, Hill J, et al. Multiple imputation with diagnostics (mi) in R: Opening windows into the black box. J Stat Softw 2011;45:1-31.
- Census Declining Mover Rate Driven by Renters, Census Bureau Reports. 2017. Accessed March 30, 2019. Available online: https://census.gov/newsroom/press-releases/2017/mover-rates.html
- Medgyesi DN, Fisher JA, Cervi MM, et al. Impact of residential mobility on estimated environmental exposures in a prospective cohort of older women. Environ Epidemiol 2020;4:e110. [Crossref] [PubMed]
- Oudin A, Forsberg B, Strömgren M, et al. Impact of residential mobility on exposure assessment in longitudinal air pollution studies: a sensitivity analysis within the ESCAPE project. ScientificWorldJournal 2012;2012:125818. [Crossref] [PubMed]
- Joseph AC, Fuentes M, Wheeler DC. The impact of population mobility on estimates of environmental exposure effects in a case-control study. Stat Med 2020;39:1610-22. [Crossref] [PubMed]
- Brokamp C, LeMasters GK, Ryan PH. Residential mobility impacts exposure assessment and community socioeconomic characteristics in longitudinal epidemiology studies. J Expo Sci Environ Epidemiol 2016;26:428-34. [Crossref] [PubMed]