Development and validation of an immune-related gene prognostic index for lung adenocarcinoma
Highlight box
Key findings
• Our research results suggested that the immune-related gene (IRG) prognostic index (IRGPI) is a prospective biomarker based on IRGs that can distinguish high- and low-risk groups to predict patient prognosis, help characterize the tumor immune microenvironment (TIME), and evaluate the benefit of immune checkpoint inhibitor (ICI) therapy in lung adenocarcinoma (LUAD).
What is known and what is new?
• The method of constructing prognostic models based on various genomes has been widely accepted.
• Prognostic models based on IRGs in LUAD have not been reported yet.
What is the implication, and what should change now?
• IRGPI can distinguish high- and low-risk groups to predict patient prognosis, help characterize the TIME, and evaluate the benefit of ICI therapy in LUAD. The genes involved in building the model still need further study.
Introduction
Lung cancer is the most common malignancy worldwide, and it caused nearly a quarter of the cancer-related deaths in 2021 (1). It is estimated that between 2015 and 2030, the median age-standardized mortality rates (ASMR) of lung cancer will rise by about 40% (2). The high morbidity and mortality of lung cancer make it one of the most serious public health problems globally. The most common subtype of lung cancer is lung adenocarcinoma (LUAD) (3). Although comprehensive treatment plans such as surgery and chemoradiotherapy have been adopted, especially in the last few years, the individual application of targeted drugs has significantly improved the objective response rate (ORR) of treatment, but the prognosis of LUAD is still not optimistic.
Immunotherapy is an effective therapy that has emerged in the area of cancer treatment in recent years, and has changed the treatment landscape of multiple solid malignancies (4), the most impressive of which is immune checkpoint inhibitor (ICI) therapy (5). The clinical advantages of ICI include a sustained anti-tumor immune response with a low rate of recurrence, and even be complete mitigation in some advanced malignancies (6). Therefore, ICI has been approved for the first-line treatment of certain cancers.
ICI shows powerful antitumor effects in some non-small cell lung cancer (NSCLC) patients. The treatment of metastatic and advanced NSCLC was revolutionized by immunotherapy with programmed cell death 1 (PD-1) or programmed cell death-ligand 1 (PD-L1) antibodies (7-9). Currently, pembrolizumab and atezolizumab monotherapy are the preferred therapeutic options for LUAD patients with expression levels of PD-L1 ≥50% and negative for actionable molecular markers (10). The potential of these drugs to produce durable clinical responses to therapy has led to their rapid adoption as a standard of therapy (11,12). Nevertheless, in current clinical practice, the use of ICI incurs two key challenges; ICIs can cause serious side effects in many organs (13-15); ICI therapy is completely ineffective for many patients. Therefore, the development of a gold-standard biomarker to identify patients likely to benefit from ICI is challenging (16).
With the development of bioinformatics analysis, gene signatures identified from different types of genomes are increasingly accepted as new tumor marker candidates. For example, a prognostic model based on genes related to ferroptosis and metabolism is expected to provide new insights into the development and treatment of cancer (17-21). In the present study, we attempted to explore a prognostic marker for LUAD based on immune-related genes (IRGs). It has practical application value by detecting the expression of model genes in LUAD tissues and converting genes expression level into risk scores to predict patient prognosis. An IRG prognostic index (IRGPI) of LUAD was established by exploiting weighted gene co-expression network analysis (WGCNA) using transcriptomic data and clinical outcomes. Univariate and multivariate Cox regression analysis were utilized to recognize differentially expressed immune-related genes (DEIRGs) associated with survival, and then to build IRGPI, a quantitative score that distinguishes between low and high risk of prognosis. At the same time, we use the GSE72094 cohort to verify the results, which showed that the results are consistent with those of The Cancer Genome Atlas (TCGA). Finally, the molecular and tumor microenvironment (TME) characterization of IRGPI was verified and its prognostic predictive ability in patients with immunotherapy was validated, and contrasted with other immunotherapy biomarkers, angiogenesis-related genes (ARGs), tumor immune dysfunction and exclusion (TIDE), and tumor inflammation signature (TIS). We present this article in accordance with the TRIPOD reporting checklist (available at https://jtd.amegroups.com/article/view/10.21037/jtd-23-1374/rc).
Methods
Data collection
Transcriptome RNA-sequencing data (FPKM normalized), mutation information, and corresponding clinicopathological characteristics of LUAD patients were downloaded from TCGA’s Genomic Data Commons Data Portal (https://portal.gdc.cancer.gov/), which includes 522 patients and 594 samples (535 cancer samples and 59 normal samples) (accessed February 26, 2022) (table available at https://cdn.amegroups.cn/static/public/10.21037jtd-23-1374-1.xls). To further validate the reliability of the IRGPI risk score, an independent cohort of 442 LUAD simples, GSE72094, was obtained from the Gene Expression Omnibus (GEO) database (https://www.ncbi.nlm.nih.gov/geo/), along with transcriptomic data and clinical outcomes (accessed March 18, 2022). All available data downloaded from the TCGA and GSE72094 cohorts were analyzed to maximize the power and generalizability of the results. The clinicopathological characteristics are summarized in Table 1.
Table 1
Patient characteristics | Training cohorts | Test cohorts (GSE72094) |
---|---|---|
No. of patients | 522 | 442 |
Status, n (%) | ||
Alive | 334 (64.0) | 298 (67.4) |
Dead | 188 (36.0) | 122 (27.6) |
Unknown | 0 | 22 (5.0) |
Survival time, days, median [range] | 820 [3–2,077] | 653 [0–7,248] |
Age, years, median [range] | 66 [33–88] | 70 [38–89] |
Age (years), n (%) | ||
≤65 years | 241 (41.2) | 127 (28.7) |
>65 years | 262 (51.2) | 294 (66.5) |
Unknown | 19 (3.6) | 21 (4.8) |
Sex, n (%) | ||
Male | 280 (53.6) | 240 (54.3) |
Female | 242 (46.4) | 202 (45.7) |
Stage, n (%) | ||
I | 279 (53.4) | 265 (60.0) |
II | 124 (23.8) | 69 (16.5) |
III | 85 (16.3) | 63 (14.3) |
IV | 26 (5.0) | 17 (3.8) |
Unknown | 8 (1.5) | 28 (6.3) |
T, n (%) | ||
1 | 172 (33.0) | NA |
2 | 281 (53.8) | NA |
3 | 47 (9.0) | NA |
4 | 19 (3.6) | NA |
Unknown | 3 (0.6) | NA |
N, n (%) | ||
0 | 335 (64.2) | NA |
1 | 98 (18.8) | NA |
2 | 75 (14.4) | NA |
3 | 2 (0.4) | NA |
Unknown | 12 (2.3) | NA |
M, n (%) | ||
0 | 353 (67.6) | NA |
1 | 25 (4.8) | NA |
Unknown | 144 (27.6) | NA |
IRGs were obtained from the InnateDB database (https://www.innatedb.ca/) and the immunology database and analysis portal (ImmPort; https://www.immport.org/home) (accessed July 31, 2021). 1,226 immune genes in the InnateDB database and 1,793 immune genes in the ImmPort database were screened for further analysis.
A total of 48 ARGs were downloaded from the Molecular Signatures Database (MSigDB; http://www.broad.mit.edu/gsea/msigdb) to analyze the correlation with immune infiltration (accessed April 14, 2021).
The data used in this study are publicly available, and thus informed consent or ethical approval was not required. The study was conducted in accordance with the Declaration of Helsinki (as revised in 2013).
IRGs differential expression analysis
The differentially expressed genes (DEGs) was evaluated by the limma package in R (R Foundation for Statistical Computing, Vienna, Austria) with a cutoff criterion |log2 fold-change| >1 and false discovery rate (FDR) <0.05. Based on the ImmPort database and the InnateDB database, we identified 3,019 IRGs. We defined DEIRGs as overlapping genes between DEGs and IRGs. Gene Ontology (GO) and Kyoto Encyclopedia of Genes and Genomes (KEGG) analyses were employed to identify the potential functions and pathways that the DEIRGs, and the functions and pathways significantly enriched in DEIRGs were visualized by the GOPlot R package.
Assessment of hub genes
The hub genes were identified by WGCNA. The topological overlap measure (TOM) was set to 1-TOM as distance of clustered genes and the optimal soft-thresholding power was set to 4. Significantly different expression pattern modules between tumor and normal samples were selected for further analysis. Then, the intersection between the modules and DEIRGs was chosen.
Construction of IRGPI
To build a prognostic index, a univariate Cox regression analysis was performed to identify the relationship between IRGs expression and overall survival (OS). The genes that significantly affected OS were utilized to build the IRGPI by multivariate Cox regression analysis. risk score = (expIRG1 × coef1) + (expIRG2 × coef2) +... + (expIRGn × coefn). High- and low-risk groups of LUAD patients were divided by the median risk score (0.997). A Kaplan-Meier (KM) survival analysis was performed to compare OS between the two groups. Furthermore, univariate and multivariate Cox regression analyses were utilized on IRGPI risk score and clinicopathological features including age, sex, and stage to evaluate the independent prognostic value of IRGPI. Knots for age were at 65. Samples with missing clinical data in univariate and multivariate Cox regression analyses were removed.
Characteristics analysis of TME and ARGs in different IRGPI subgroups
To evaluate the potential functions and pathways of the two IRGPI groups, gene set enrichment analysis (GSEA) was performed by the ClusterProfile R package (P value <0.05). Maftools R package was used to develop the somatic mutation landscape in two IRGPI groups.
The Cell-type Identification by Estimating Relative Subsets of RNA Transcripts algorithm (CIBERSORT; HTTPS://cibersort.stanford.edu/) was performed to identify immune characteristics of LUAD and appraise the relative proportions of 22 types of immune cells. The associated proportions of clinicopathological factors and these immune cells were compared between the two IRGPI groups. Correlation analysis was carried out between IRGPI risk score and conventional or potential immunotherapy biomarkers, such as PD-1, cytotoxic T-lymphocyte-associated protein 4 (CTLA-4), PD-L1, AUNIP, NPM1, EXO1, CBX7, SFTPA1, and tumor mutation burden (TMB).
To clarify whether there is a correlation between ARGs and IRGPI risk scores, correlation analysis was performed between IRGPI risk score and 48 ARGs obtained from MSigDB.
Comprehensive analysis of clinicopathological characteristics and ICI efficacy in different IRGPI subgroups
Distribution of clinicopathological features were compared in the two IRGPI subgroups and visualized by the ComplexHeatmap package. In addition, the distribution of immune subtypes was compared in the two IRGPI groups; the immune subtypes were based on extensive immune genomic analysis of more than 10,000 tumors using data aggregated by TCGA, which identified and characterized 6 immune subtypes across multiple tumor types.
To explore the prognostic value of ICI therapy in different IRGPI groups, TIDE scores were computed online (http://tide.dfci.harvard.edu/) in two IRGPI groups. TIS is an 18-gene signature that demonstrates prospective results in predicting response to anti-PD-1/PD-L1 agents (Table S1). The capability of the IRGPI risk score was appraised by receiver operating characteristic (ROC) curves and area under the curve (AUC) values, which compared with the TIDE and TIS scores.
Statistical analysis
R (4.2.0 version; R Foundation for Statistical Computing) was employed for all statistical analyses. Continuous variables were compared between two IRGPI subgroups using an independent t-test and categorical data analysis was conducted using the χ2 test. The Wilcoxon test was used to verify TIDE scores groups. Univariate and multivariate survival analysis was performed using KM survival analysis with the log-rank test and Cox regression model, respectively. The effect of IRGPI and clinical features on prognosis were analyzed by univariate and multivariate Cox regression analysis. A P value <0.05 was considered statistically significant.
Results
Immune-related hub DEGs
A total of 8,109 DEGs were obtained by differential expression analysis (535 tumor and 59 normal samples), of which 6,245 genes were up-regulated and 1,864 genes were down-regulated (Figure 1A, table available at https://cdn.amegroups.cn/static/public/10.21037jtd-23-1374-2.xls). By intersecting these DEGs with the IRG downloaded from the InnateDB database and the ImmPort database, we obtained 681 DEIRGs, among which 423 genes were up-regulated and 258 genes were down-regulated in tumor samples compared with normal samples (Figure 1B, table available at https://cdn.amegroups.cn/static/public/10.21037jtd-23-1374-3.xls).
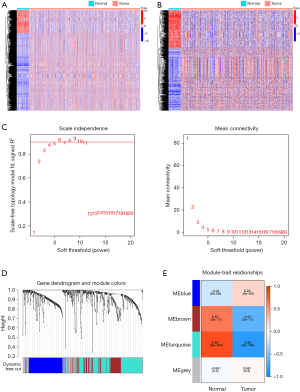
WGCNA showed that the DEIRGs were strongly associated with LUAD. The scale-free topology criterion was used to determine the soft threshold. In the present study, the correlation coefficient was greater than 0.85. The optimal soft-thresholding power was 4 based on the scale-free network (Figure 1C). According to the average link hierarchical clustering and optimal soft threshold capability, we identified four modules (Figure 1D,1E). A total of 681 genes were assigned to the four modules, three of which were selected as significant modules (the blue, brown, and turquoise) (P<0.05). The genes contained in the three significant modules were obtained and intersected with the DEIRGs. Finally, 426 DEIRGs strongly associated with LUAD were obtained for further analysis.
Functional enrichment analysis of the IRG in the pathogenesis of LUAD
In order to further understand the role of the IRGs in the pathogenesis of LUAD, functional and pathway enrichment analyses of IRGs were performed. A total of 681 DEIRGs were conducted to determine 1,808 GO terms and 59 KEGG pathways. All top ten terms of biological processes (BP), cellular components (CC), and molecular function (MF) were illustrated (Figure 2A). Terms such as immunoglobulin production, humoral immune response, and the production of molecular mediators of the immune response were enriched in the BP category; immunoglobulin complex, external side of plasma membrane, and immunoglobulin complex, circulating were enriched in the CC category; signaling receptor-activator activity, receptor-ligand activity, and antigen-binding were enriched in the MF category. The full results of GO are listed in table available at https://cdn.amegroups.cn/static/public/10.21037jtd-23-1374-4.xls. As for KEGG pathway analysis, the set of IRGs was mainly enriched in cytokine-cytokine receptor interaction, viral protein interaction with cytokine and cytokine receptor, and the chemokine signaling pathway (Figure 2B). The full results of GO are listed in table available at https://cdn.amegroups.cn/static/public/10.21037jtd-23-1374-5.xls. The results indicated that the DEIRGs were significantly associated with immune response.
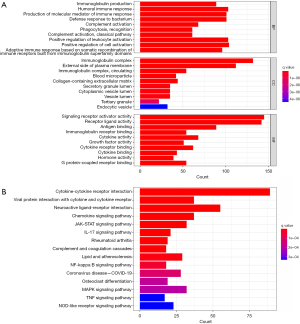
Performance of IRGPI risk score in predicting the prognosis of LUAD
Univariate Cox regression analysis was used to screen 426 immune-related hub genes in the three WGCNA-derived modules described above. Some 54 genes showed statistical significance for OS (P<0.01 were selected) (Figure 3A, Table S2). After narrowing the scope of OS-related hub genes by multivariate Cox regression analysis, 13 genes were picked for the construction of a prognostic index. The 13 genes included CD79A, F2RL1, GMFG, INHA, NLRC4, AGER, GPI, ANGPTL4, LIFR, TRIM6, PLK1, C7, and C6. Subsequently, the IRGPI risk score was calculated using the weights of the 13 genes multiplied by the corresponding gene expression level (Table 2). Risk scores were calculated for each patient in the TCGA and GSE72094 cohorts, and patients were divided into high- and low-risk subgroups using the TCGA median of 0.997. Through t-distributed stochastic neighbor embedding (tSNE) and principal component analysis (PCA) analysis, we determined that the model divided the TCGA and GSE72094 samples well (Figure 3B,3C).
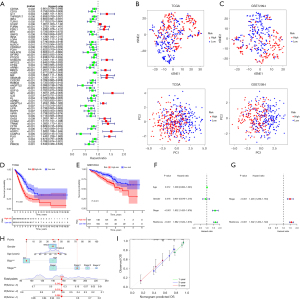
Table 2
ID | Coef |
---|---|
CD79A | −0.23544897047258 |
F2RL1 | 0.164033293483382 |
GMFG | 0.229663457926312 |
INHA | 0.0854504370539527 |
NLRC4 | −0.379763670758073 |
AGER | −0.106632985781127 |
GPI | 0.290658464320619 |
ANGPTL4 | 0.142341274528789 |
LIFR | −0.189665876861247 |
TRIM6 | 0.250033316508902 |
PLK1 | 0.249729746881706 |
C7 | 0.246389600680723 |
C6 | −0.140902095752421 |
IRGPI, immune-related gene prognostic index.
KM survival analysis showed that OS was significantly related with IRGPI risk score, the IRGPI low-risk patients had better OS than IRGPI high-risk patients (P<0.001, log-rank test; Figure 3D). Next, to assess the reliability of this risk model, we performed a survival analysis on an independent cohort (GSE72094, n=442). Of note, similar results to the TCGA cohort were achieved (P<0.001, log-rank test; Figure 3E). The result illustrates that the IRGPI risk model has applicability for predicting the prognosis of LUAD patients and it could be a prospective indicator to evaluate the prognosis of LUAD patients. This result was also confirmed by univariate analysis (Figure 3F, Table S3). Furthermore, IRGPI is an independent prognostic factor adjusted by stage of LUAD patients via multivariate Cox regression analysis (Figure 3G, Table S4).
Then, to better apply the risk score model to the clinic, we integrated the risk score and other clinicopathological risk factors, and constructed a nomogram to predict the 1-, 3-, and 5-year survival of patients (Figure 3H). The calibration curve showed that it has not deviated from the reference line and does not require recalibration (Figure 3I).
Molecular characteristics of two IRGPI subgroups
GSEA was performed to investigate the gene sets enriched in two IRGPI groups. The gene sets of the IRGPI-low group were enriched in systemic lupus erythematosus, asthma, and intestinal immune network for IGA production pathways (Figure 4A), whereas the gene sets of the IRGPI-high group were enriched in the proteasome, DNA replication, cell cycle, pyrimidine metabolism, ribosome, and other related pathways (Figure 4B). The full results of GSEA are listed in table available at https://cdn.amegroups.cn/static/public/10.21037jtd-23-1374-6.xlsx.
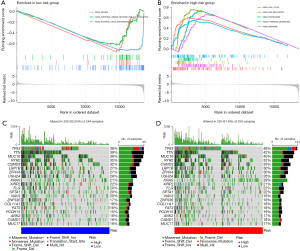
Subsequently, the somatic mutation landscapes of the two IRGPI subgroups were plotted to gain further insight into the tumorigenesis of LUAD. Compared with the IRGPI low-risk group, the mutation counts in the IRGPI high-risk group were significantly higher. The most common type of mutation was missense mutation, followed by nonsense deletion. The top 20 genes with the highest mutation rates in the two IRGPI subgroups were illustrated, and most of them in the IRGPI high-risk group had a higher mutation rate, except for RYR2, USH2A, XIRP2, NAV3, and COL11A1 (Figure 4C,4D).
Immune characteristics of two IRGPI subgroups
To explore the constitution of immune cells in the two IRGPI subgroups, the CIBERSORT algorithm was performed to compare the distribution of 22 types of immune cells in different IRGPI groups. The results illustrated that plasma cells, resting dendritic cells (DC), resting mast cells, memory B cells, and resting memory CD4 T cells were more plentiful in the IRGPI-low groups whereas M0 macrophages, activated DC, and resting natural killer (NK) cells were more abundant in the IRGPI-high groups (Figure 5A, table available at https://cdn.amegroups.cn/static/public/10.21037jtd-23-1374-7.xls). Next, we performed the single-sample GSEA (ssGSEA) score to investigate the enrichment level of 29 immune characteristics in each LUAD sample of different IRGPI groups. As a result, we found that there were more B cells, human leukocyte antigen (HLA), iDCs, mast cells, neutrophils, T helper cells, tumor-infiltrating lymphocytes (TIL), type II IFN response, CD8+ T cells, T-cell co-inhibition, T-cell co-stimulation, activated DCs (aDCs), cytolytic activity, inflammation-promoting, plasmacytoid DCs (pDCs), and follicular helper T (Tfh) in the low-risk groups (Figure 5B). The results revealed that the IRGPI low-risk group had more active immune-related functions. Subsequently, we further identified the survival relationship between immune and molecular function and IRGPI subgroups, and the results demonstrated that patients with more aDCs, B cells, CD8+ T cells, cytolytic activity, HLA, iDCs, inflammation-promoting, mast cells, pDCs, T-cell co-inhibition, T helper cells, Tfh, TIL, and type II IFN response correlation signals had a better prognosis (Figure 5C-5P).
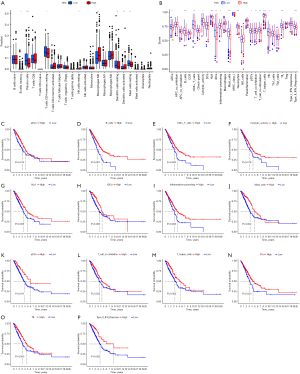
Association between IRGPI subgroups and immunotherapy biomarkers
Some biomarkers such as PD-1, PD-L1, and CTLA-4, as well as TMB, have been used in clinical immunotherapy. In addition, an increasing number of potential biomarkers are being discovered, such as AUNIP, NPM1, EXO1, CBX7, and SFTPA1.
We investigated the association between IRGPI scores and these biomarkers. The Pearson correlation coefficients between IRGPI risk scores and PDCD1 (PD-1) was −0.1 with P=0.025 [CTLA-4: r=−0.2, P=9.5e−06; CD274 (PD-L1): r=−0.023, P = 0.61; AUNIP: r=0.39, P<2.2e−16; NPM1: r=0.26, P=2e−09; EXO1: r=0.43, P<2.2e−16; CBX7: r=−0.47, P<2.2e−16; SFTPA1: r=−0.27, P=1e−09; TMB: r=0.13, P=0.0036] (Figure 6A-6I). The full results of TMB are listed in table available at https://cdn.amegroups.cn/static/public/10.21037jtd-23-1374-8.xls. We found that, except for PD-L1, the correlation trends between the IRGPI scores and other biomarkers were the same as in previous studies, and the P value of the correlation was less than 0.05, indicating that the IRGPI score is strongly associated with this biomarker.
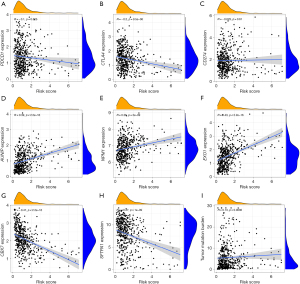
Association between IRGPI subgroups and ARGs
The growth of lung cancer is dependent on angiogenesis, and a large amount of angiogenesis is correlated to the invasion and poor outcome of lung cancer. In addition, angiogenesis was found to play an important role in immunosuppression, leading to primary and secondary resistance to ICI. Therefore, combining analysis of angiogenesis and immunity can deepen our understanding of ICI. Based on our research, we sought to clarify whether there is a correlation between ARGs expression and IRGPI risk score. A total of 48 ARGs were obtained from MSigDB and we investigated the relationship between IRGPI scores and the expression of these genes. Pearson correlation coefficients and P values between IRGPI risk scores and these genes are presented in Table S5. Among them, 24 genes were negatively correlated with IRGPI scores, 9 were positively correlated, and the remaining genes had P values >0.05. The 9 ARGs with the smallest P value are listed in Figure 7A-7I (EMCN: r=−0.36, P<2.2e−16; SPHK1: r=0.35, P=1.2e−15; COL4A3: r=−0.33, P=1.6e−14; HTATIP2: r=0.29, P<2.1e−11; TNFSF12: r=−0.26, P=2.4e−09; SHH: r=−0.25, P<2.2e−08; FOXO4: r=−0.25, P<2.4e−08; NCL: r=0.23, P=3.6e−07; IL17F: r=−0.22, P=4e−07) (Figure 6A-6I). We found that most of the ARGs were strongly associated with IRGPI risk scores.
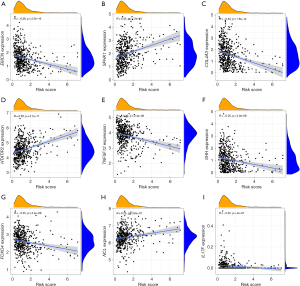
Analyses of clinicopathological characteristics and other immune subtypes in different IRGPI subgroups
Figure 8A illustrates the distribution of clinicopathological characteristics of 535 LUAD patients in the TCGA cohort in two risk groups (table available at https://cdn.amegroups.cn/static/public/10.21037jtd-23-1374-9.xls). We found a significant relationship in tumor stages and grades between the two IRGPI groups. We further observed tumor staging and found that the stage I patient count in the IRGPI-low group was significantly higher than that in the IRGPI-high group, whereas in stages II and III, the opposite was true (Figure 8B).
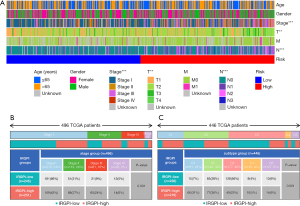
According to extensive immune genomic analysis, six immune subtypes were identified, which included wound healing (c1), interferon-γ (IFN-γ) dominant (c2), inflammatory (c3), lymphocyte depleted (c4), immunologically quiet (c5), and transforming growth factor-β (TGF-β) dominant (c6). The six immune subtypes have latent therapeutic and prognostic implications for cancer. In the present study, we found that the C3 had more IRGPI-low group patients than the other four subtypes (Figure 8C), which probably suggested that patients in C3 group had the best prognosis.
Response analysis of ICI therapy in different IRGPI subgroups
We performed a TIDE score to evaluate the latent clinical curative effect of ICI treatment between low- and high-risk groups. In our results, we discovered that the low-risk group had higher TIDE scores than the high-risk group (Figure 9A), which implies that the high-risk group could benefit more from ICI treatment than the low-risk group. Moreover, we found that the high-risk group had higher microsatellite instability (MSI-H) score (Figure 9B), higher T-cell exclusion score, and lower T-cell dysfunction score (Figure 9C,9D).
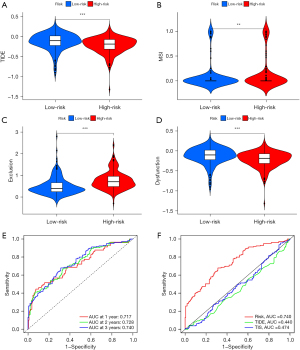
Based on the TCGA cohort, we validated the predictive capability of the IRGPI score for ICI therapy effect. Our data showed that the AUC of the ROC curve of the prognostic model reached 0.717 for 1-year OS, 0.728 for 2-year OS, and 0.740 for 3-year OS (Figure 9E). In addition, compared with TIDE and TIS scores, IRGPI scores had more accurate prediction power (Figure 9F). The results suggest that the IRGPI score probably plays an important role on predicting immunotherapy response.
Discussion
Immunotherapy is one of the most significant breakthroughs in cancer therapy, and it has been shown to be a valid therapy for patients with advanced, recurrent, and metastatic malignancy (22,23). However, merely a minority of patients can benefit from ICI treatment. Thus, a priority is to explore reliable biomarkers to select who can benefit most from immunotherapy.
In our study, WGCNA and regression analysis were used to determine candidate 13 core IRGs affecting patient OS to build a IRGPI based on the DEIRGs in LUAD. The IRGPI score, calculated by the weighted level (Table 2), was a valid and independent prognostic factor. In short, LUAD patients with low IRGPI scores have better survival, whereas those with high IRGPI scores are associated with a worse survival in the TCGA and GEO cohort. We sought to summarize the functions of the 13 genes and find a mechanistic interpretation for the IRGPI. The CD79A (CD79a molecule) is encoded by the MB-1 gene, and its mutation results in a complete arrest of B-cell development (24). F2R like trypsin receptor 1 (F2RL1) is a G-protein-coupled receptor (25) that is significantly upregulated in ovarian, cervical, and lung cancers, and enhances tumor cell proliferation, migration, and invasion (26-29). Glia maturation factor gamma (GMFG) is a 17 kDa protein, which mediates monocyte, neutrophil, and T-lymphocyte migration (30-32). Inhibin subunit alpha (INHA) may suppress tumor activity in normal epithelial cells and switch to tumor-facilitating activity in tumor, similar to TGF-β, and inhibin-α expression is elevated in most cancers (33,34). NLR family CARD domain containing 4 (NLRC4) is an essential component of the NLRC4 inflammasome, but the independent action of NLRC4-expressed inflammasome in macrophages is required to produce inhibition of melanoma progression (35). Furthermore, it was found from NLRC4-mediated antitumor immunity that IL-1β is released from DCs after activation of the nucleotide-binding oligomerization domain (NOD)-like receptor family pyrin domain containing 3 (NLRP3) inflammasome could promote antitumor immune responses during chemotherapy (36). Advanced glycosylation end-product specific receptor (RAGE) is a multiligand receptor of the immunoglobulin superfamily that is related to innate and adaptive immune reaction, immune cell migration, and chemotaxis and cytokine generation by interacting with ‘alarmins’ HMGB1 and S100B (37). Furthermore, recent findings have demonstrated that targeting RAGE might offset the damage of muscle strength and mass and extend survival in tumor patients (38). Glucose-6-phosphate isomerase (GPI) is a member of the glucose phosphate isomerase protein family, which may be involved in cancer invasion and metastasis (39,40). Moreover, the expression of GPI was significantly upregulated in LUAD patients, which was closely related to poor prognosis, and its mechanisms affecting LUAD progression may affect LUAD progression through regulation of cell cycle and immune infiltration (41). Angiopoietin like 4 (ANGPTL4) is an adipokine that adjusts lipid metabolism and influences tumor progression (42-44), and its triggering of tumor metastasis might occur through activation of integrin signaling (45). LIF receptor subunit alpha (LIFR) is a metastasis suppressor that inhibits invasion and metastasis in many kinds of tumors including hepatocellular carcinoma, lung cancer, and gastric cancer (46-48). Tripartite motif containing 6 (TRIM6) is a member of the TRIM family proteins. An improving number of studies have demonstrated its role in regulating invasion and metastasis (49,50), among which TRIM6 may promote CRC migration and invasion by regulating STAT3 activation status (51). Polo like kinase 1 (PLK1) is a highly conserved serine-threonine kinase that has been verified to be closely related to tumorigenesis and aberrantly overexpressed in many tumors, correlating with poor prognosis in some human cancers (52-54). In NSCLC, PLK1 overexpression induces epithelial to mesenchymal transition (EMT) and increases cell motility, and PLK1 is a powerful predictor of worse survival in patients with metastatic NSCLC (55). Although complement C7 is a significant part of the innate immune system, high expression of C7 may promote the development of breast cancer (56). Furthermore, plasma C7 levels have been shown to be able to accurately predict treatment response to pembrolizumab in NSCLC patients, suggesting that plasma C7 is a surrogate and supportive biomarker (57). Decreased complement C6 expression in hepatocellular carcinoma is related to poor outcome and increased immune cell infiltration. This finding indicates that C6 may be involved in immune cell infiltration (58). Consistent with previous studies, we found that F2RL1, GMFG, INHA, GPI, ANGPTL4, TRIM6, PLK1, and C7 had positive weights, whereas CD79a, NLRC4, AGER, LIFR, and C6 had negative weights (Table 2). Thus, IRGPI was positively correlated with F2RL1, GMFG, INHA, GPI, ANGPTL4, TRIM6, PLK1, and C7, whereas IRGPI was negatively correlated with CD79a, NLRC4, AGER, LIFR, and C6. In conclusion, IRGPI is a biomarker closely related to active immunity and tumor promotion or suppression.
To comprehend the immunological properties of IRGPI subgroups in-depth, we subsequently studied genetic mutations in two IRGPI groups. Missense mutations were the most common mutation type, followed by nonsense deletion, as in a previous study (59). In our study, universally higher mutation rates in the IRG high-risk group were found. The largest differences in mutations between subgroups were in the TP53 and KRAS genes, which were more common in IRGPI-high samples than in IRGPI-low samples (48% vs. 39% and 29% vs. 21%, respectively). TP53 is the most commonly mutated gene in human tumors and is associated with aggressiveness and poor prognosis in many cancers (60). KRAS mutations may contribute to a worse prognosis via strengthening tumor cell proliferation (61). It has been reported that TP53 mutation induced the expression of immune checkpoints and activated T-effector and IFN-γ signature in LUAD. Furthermore, the TP53/KRAS co-mutated subgroup exhibited a unique increase in PD-L1 expression and the highest proportion of PDL1+/CD8A+, demonstrating that patients with TP53 and KRAS mutations would be more responsive to ICI (62). This factor maybe a foundation for the response to ICI treatment for patients with high IRGPI score.
Next, we investigated the TME of two IRGPI groups. The component of some immune cells differs between two IRGPI groups. We found that memory B cells, plasma cells, resting memory CD4 T cells, resting DCs, and resting Mast cells were more enriched in the IRGPI-low group, whereas resting NK cells, M0 macrophages, aDCs, and resting mast cells were more enriched in the IRGPI-high group. Plasma cells have been reported to be related to improved survival in NSCLC (63). Dense infiltration of T cells, particularly resting memory CD4 T cells, has been shown to predict a good prognosis (64). In many tumors, M0 macrophages are associated with chronic inflammation and contribute to tumor growth and increased aggressiveness, and it is related to its prognosis (65-67).
Among known immunotherapy predictive biomarkers such as PD-L1, PD-1, CTLA-4, and TMB, as well as potential biomarkers predicted by the current studies, such as AUNIP, NPM1, EXO1, CBX7, and SFTPA1, increased AUNIP, NPM1, and EXO1 expression predicted worse OS in LUAD patients (68-70). In contrast, higher SFTPA1 and CBX7 expression predicted better outcome in LUAD patients (71,72). Comparing them with the IRGPI risk score, we found that the IRGPI score significantly correlated with these biomarkers, except for PD-L1. In general, PD-L1, a predictive biomarker for PD-1/PD-L1 inhibitor, is commonly used to predict the prognosis of immunotherapy, and patients with PD-L1 positivity are more likely to benefit from anti-PD-1/PD-L1 treatments than patients with PD-L1 negativity (73). However, the implementation of PD-L1 is restricted by a large number of PD-L1 antibodies, assays, positivity thresholds, and scoring systems currently in use (74). Of these, lack of consistency in testing and changeability in the thresholds used to delimit PD-L1 positivity are probably the predominant causes. In addition, some researchers believe that the PD-L1 expression value detected by immunohistochemistry is more valuable than the intension and location of the PD-L1 expression value tested by transcriptome data (75). Therefore, in-depth studies are required to clarify the association between PD-L1 and IRGPI. In addition, since the growth of lung cancer is dependent on angiogenesis, which is associated with the invasion and poor prognosis of lung cancer. Furthermore, angiogenesis was found to play an important role in immunosuppression, leading to primary and secondary resistance to ICI (76). So, we also analyzed the correlation of ARGs and the IRGPI risk score. We found that 24 ARGs were correlated positively with the IRGPI score, and 9 ARGs were negatively correlated, demonstrating that most ARGs were significantly associated with the IRGPI score. Therefore, the relationship between ARGs and IRGPI deserves further study.
Further observing the clinicopathological characteristics of two risk groups, we found a significant relationship between tumor stage and grade between the two risk groups. Tumor staging was further looked at, and it was found that the number of patients with stage I was significantly higher in the IRGPI-low group than in the IRGPI-high group, whereas in stages II and III, the opposite was true, indicating a better prognosis in the low-risk group. To further verify this conclusion, we compared six immune subtypes based on pooled TCGA data, as previously described. The six immune subtypes have potential therapeutic and prognostic implications for cancer. A previous study has shown that C3 has the best prognosis, followed by C1 and C2, and C4 and C6 have the worst prognosis (77). In this study, we found that low-risk patients were significantly clustered in the C3, which supported our conclusion.
Finally, comparison with other diagnostic criteria of ICI treatment, including the TIDE and TIS scores (78,79), demonstrated that IRGPI has better predictive performance in many solid tumors. The TIDE was associated with T-cell dysfunction in high infiltration of cytotoxic T lymphocytes (CTLs) cancers and T-cell exclusion in low infiltration of CTLs cancers, thus representing two potential mechanisms of tumor immune escape (79). For ICI-treated patients, the TIDE has higher accuracy in predicting survival outcomes than current clinically commonly used biomarkers such as PD-L1 expression and TMB. In our research, IRGPI-low patients had higher CTL infiltration, TIDE, and T-cell dysfunction scores than the IRGPI-high patients. Therefore, their higher prognosis and lower ICI response may be due to higher CTL infiltration and T-cell dysfunction score (79). In contrast, the IRGPI-high subgroup had a higher MSI and T-cell exclusion score and a lower TIDE and T-cell dysfunction score. Although a higher T-cell exclusion score implies a higher likelihood of immune evasion, a lower T-cell dysfunction score may improve ICI responses (79). Overall, the high-risk group with lower TIDE score has a better immune microenvironment. In addition, it was found that the IRGPI score had reliable predictive performance for ICI treatment effect in the TCGA cohort and was better than the predictive capability of TIDE and TIS scores.
The model we constructed has certain advantages. By comparing with other recently released models, our model has higher reliability and stronger pertinence. ROC analysis shows that the predictive value of our prognostic model is better than other models, and for the predictive ability of 1-, 3-, and 5-year survival times, our calibration curve showed high accuracy between actual incidence and predicted incidence better than other models (80,81). And the deep combination of this model with immunity has significant advantages in predicting the efficacy of immunotherapy.
Although the IRGPI score had so many advantages, it still has certain limitations. First, the analysis was based on a public database, such as TCGA and GEO databases, which are valuable resource but has limitations, including potential heterogeneity in data quality and patient characteristics. secondly, the effect of ICI was evaluated using score, not clinical data, because it was based on a public database. Finally, the experiments were not conducted for in vitro or in vivo functional analysis of gene expression. In view of the deficiencies mentioned above, we will clinically measure the expression of 13 model genes in patients with LUAD to predict patient prognosis, and verify the predictive ability of the prognostic model in practice through long-term follow-up.
Conclusions
The IRGPI is a promising immune-related prognostic biomarker. It can distinguish high- and low-risk groups to predict patient prognosis, help characterize the tumor immune microenvironment (TIME). Our study revealed that the high-risk subgroup may be more responsive to ICI therapy, but further research is warranted to clarify this point.
Acknowledgments
We sincerely thank the China Postdoctoral Science Foundation, Yunnan Health System of Leading Talents, The Ministry of Education International Cooperation Joint Laboratory of Plateau Regional High Incidence Tumors, Science and Technology Innovation Team of Diagnosis and Treatment for Glucolipid Metabolic Diseases in Kunming Medical University, Cancer Clinical Medical Center of Yunnan Province, The Joint Special Key Project Funds for the Department of Science and Technology of Yunnan Province-Kunming Medical University, and the Joint Special Funds for the Department of Science and Technology of Yunnan Province-Kunming Medical University for their financial support, which made it possible to cover the article processing fee of the present study. We thank LetPub (www.letpub.com) for its linguistic assistance during the preparation of this manuscript.
Funding: This work was supported by
Footnote
Reporting Checklist: The authors have completed the TRIPOD reporting checklist. Available at https://jtd.amegroups.com/article/view/10.21037/jtd-23-1374/rc
Peer Review File: Available at https://jtd.amegroups.com/article/view/10.21037/jtd-23-1374/prf
Conflicts of Interest: All authors have completed the ICMJE uniform disclosure form (available at https://jtd.amegroups.com/article/view/10.21037/jtd-23-1374/coif). The authors have no conflicts of interest to declare.
Ethical Statement: The authors are accountable for all aspects of the work in ensuring that questions related to the accuracy or integrity of any part of the work are appropriately investigated and resolved. The study was conducted in accordance with the Declaration of Helsinki (as revised in 2013).
Open Access Statement: This is an Open Access article distributed in accordance with the Creative Commons Attribution-NonCommercial-NoDerivs 4.0 International License (CC BY-NC-ND 4.0), which permits the non-commercial replication and distribution of the article with the strict proviso that no changes or edits are made and the original work is properly cited (including links to both the formal publication through the relevant DOI and the license). See: https://creativecommons.org/licenses/by-nc-nd/4.0/.
References
- Siegel RL, Miller KD, Fuchs HE, et al. Cancer Statistics, 2021. CA Cancer J Clin 2021;71:7-33. [Crossref] [PubMed]
- Martin-Sanchez JC, Lunet N, Gonzalez-Marron A, et al. Projections in Breast and Lung Cancer Mortality among Women: A Bayesian Analysis of 52 Countries Worldwide. Cancer Res 2018;78:4436-42. [Crossref] [PubMed]
- Ferlay J, Colombet M, Soerjomataram I, et al. Cancer incidence and mortality patterns in Europe: Estimates for 40 countries and 25 major cancers in 2018. Eur J Cancer 2018;103:356-87. [Crossref] [PubMed]
- Kirkwood JM, Butterfield LH, Tarhini AA, et al. Immunotherapy of cancer in 2012. CA Cancer J Clin 2012;62:309-35. [Crossref] [PubMed]
- Riley RS, June CH, Langer R, et al. Delivery technologies for cancer immunotherapy. Nat Rev Drug Discov 2019;18:175-96. [Crossref] [PubMed]
- Khalil DN, Smith EL, Brentjens RJ, et al. The future of cancer treatment: immunomodulation, CARs and combination immunotherapy. Nat Rev Clin Oncol 2016;13:394. [Crossref] [PubMed]
- Antonia SJ, Villegas A, Daniel D, et al. Overall Survival with Durvalumab after Chemoradiotherapy in Stage III NSCLC. N Engl J Med 2018;379:2342-50. [Crossref] [PubMed]
- Gandhi L, Rodriguez-Abreu D, Gadgeel S, et al. Pembrolizumab plus Chemotherapy in Metastatic Non-Small-Cell Lung Cancer. N Engl J Med 2018;378:2078-92. [Crossref] [PubMed]
- Horita N, Fukuda N, Kaneko T. Atezolizumab for PD-L1-Selected Patients with NSCLC. N Engl J Med 2021;384:583-4. [Crossref] [PubMed]
- Ettinger DS, Wood DE, Aisner DL, et al. NCCN Guidelines Insights: Non-Small Cell Lung Cancer, Version 2.2021. J Natl Compr Canc Netw 2021;19:254-66. [Crossref] [PubMed]
- Reck M, Rodriguez-Abreu D, Robinson AG, et al. Pembrolizumab versus Chemotherapy for PD-L1-Positive Non-Small-Cell Lung Cancer. N Engl J Med 2016;375:1823-33. [Crossref] [PubMed]
- Herbst RS, Giaccone G, de Marinis F, et al. Atezolizumab for First-Line Treatment of PD-L1-Selected Patients with NSCLC. N Engl J Med 2020;383:1328-39. [Crossref] [PubMed]
- Byun DJ, Wolchok JD, Rosenberg LM, et al. Cancer immunotherapy - immune checkpoint blockade and associated endocrinopathies. Nat Rev Endocrinol 2017;13:195-207. [Crossref] [PubMed]
- Naidoo J, Wang X, Woo KM, et al. Pneumonitis in Patients Treated With Anti-Programmed Death-1/Programmed Death Ligand 1 Therapy. J Clin Oncol 2017;35:709-17. [Crossref] [PubMed]
- June CH, Warshauer JT, Bluestone JA. Is autoimmunity the Achilles' heel of cancer immunotherapy? Nat Med 2017;23:540-7. [Crossref] [PubMed]
- Ancevski Hunter K, Socinski MA, Villaruz LC. PD-L1 Testing in Guiding Patient Selection for PD-1/PD-L1 Inhibitor Therapy in Lung Cancer. Mol Diagn Ther 2018;22:1-10. [Crossref] [PubMed]
- Hu J, Lai C, Shen Z, et al. A Prognostic Model of Bladder Cancer Based on Metabolism-Related Long Non-Coding RNAs. Front Oncol 2022;12:833763. [Crossref] [PubMed]
- Yu Z, Qiu B, Zhou H, et al. Characterization and application of a lactate and branched chain amino acid metabolism related gene signature in a prognosis risk model for multiple myeloma. Cancer Cell Int 2023;23:169. [Crossref] [PubMed]
- Wang X, Xia G, Xiao S, et al. A ferroptosis-related gene signature associated with immune landscape and therapeutic response in osteosarcoma. Front Oncol 2022;12:1024915. [Crossref] [PubMed]
- Wang Y, Yang J, Chen S, et al. Identification and Validation of a Prognostic Signature for Thyroid Cancer Based on Ferroptosis-Related Genes. Genes (Basel) 2022;13:997. [Crossref] [PubMed]
- Li C, Liang H, Bian S, et al. Construction of a Prognosis Model of the Pyroptosis-Related Gene in Multiple Myeloma and Screening of Core Genes. ACS Omega 2022;7:34608-20. [Crossref] [PubMed]
- Borghaei H, Paz-Ares L, Horn L, et al. Nivolumab versus Docetaxel in Advanced Nonsquamous Non-Small-Cell Lung Cancer. N Engl J Med 2015;373:1627-39. [Crossref] [PubMed]
- Garon EB, Rizvi NA, Hui R, et al. Pembrolizumab for the treatment of non-small-cell lung cancer. N Engl J Med 2015;372:2018-28. [Crossref] [PubMed]
- Minegishi Y, Coustan-Smith E, Rapalus L, et al. Mutations in Igalpha (CD79a) result in a complete block in B-cell development. J Clin Invest 1999;104:1115-21. [Crossref] [PubMed]
- Antoniak S, Mackman N. Multiple roles of the coagulation protease cascade during virus infection. Blood 2014;123:2605-13. [Crossref] [PubMed]
- Jahan I, Fujimoto J, Alam SM, et al. Role of protease activated receptor-2 in tumor advancement of ovarian cancers. Ann Oncol 2007;18:1506-12. [Crossref] [PubMed]
- Michel N, Heuze-Vourc'h N, Lavergne E, et al. Growth and survival of lung cancer cells: regulation by kallikrein-related peptidase 6 via activation of proteinase-activated receptor 2 and the epidermal growth factor receptor. Biol Chem 2014;395:1015-25. [Crossref] [PubMed]
- Yang L, Ma Y, Han W, et al. Proteinase-activated receptor 2 promotes cancer cell migration through RNA methylation-mediated repression of miR-125b. J Biol Chem 2015;290:26627-37. [Crossref] [PubMed]
- Wu K, Xu L, Cheng L. PAR2 Promoter Hypomethylation Regulates PAR2 Gene Expression and Promotes Lung Adenocarcinoma Cell Progression. Comput Math Methods Med 2021;2021:5542485. [Crossref] [PubMed]
- Aerbajinai W, Liu L, Zhu J, et al. Glia Maturation Factor-gamma Regulates Monocyte Migration through Modulation of beta1-Integrin. J Biol Chem 2016;291:8549-64. [Crossref] [PubMed]
- Aerbajinai W, Liu L, Chin K, et al. Glia maturation factor-gamma mediates neutrophil chemotaxis. J Leukoc Biol 2011;90:529-38. [Crossref] [PubMed]
- Lippert DN, Wilkins JA. Glia maturation factor gamma regulates the migration and adherence of human T lymphocytes. BMC Immunol 2012;13:21. [Crossref] [PubMed]
- Derynck R, Akhurst RJ, Balmain A. TGF-beta signaling in tumor suppression and cancer progression. Nat Genet 2001;29:117-29. [Crossref] [PubMed]
- Risbridger GP, Shibata A, Ferguson KL, et al. Elevated expression of inhibin alpha in prostate cancer. J Urol 2004;171:192-6. [Crossref] [PubMed]
- Janowski AM, Colegio OR, Hornick EE, et al. NLRC4 suppresses melanoma tumor progression independently of inflammasome activation. J Clin Invest 2016;126:3917-28. [Crossref] [PubMed]
- Yu X, Liu W, Chen S, et al. Immunologically programming the tumor microenvironment induces the pattern recognition receptor NLRC4-dependent antitumor immunity. J Immunother Cancer 2021;9:e001595. [Crossref] [PubMed]
- Hudson BI, Lippman ME. Targeting RAGE Signaling in Inflammatory Disease. Annu Rev Med 2018;69:349-64. [Crossref] [PubMed]
- Chiappalupi S, Sorci G, Vukasinovic A, et al. Targeting RAGE prevents muscle wasting and prolongs survival in cancer cachexia. J Cachexia Sarcopenia Muscle 2020;11:929-46. [Crossref] [PubMed]
- Knight AL, Yan X, Hamamichi S, et al. The glycolytic enzyme, GPI, is a functionally conserved modifier of dopaminergic neurodegeneration in Parkinson's models. Cell Metab 2014;20:145-57. [Crossref] [PubMed]
- Gallardo-Perez JC, Adan-Ladron de Guevara A, Marin-Hernandez A, et al. HPI/AMF inhibition halts the development of the aggressive phenotype of breast cancer stem cells. Biochim Biophys Acta Mol Cell Res 2017;1864:1679-90. [Crossref] [PubMed]
- Han J, Deng X, Sun R, et al. GPI Is a Prognostic Biomarker and Correlates With Immune Infiltrates in Lung Adenocarcinoma. Front Oncol 2021;11:752642. [Crossref] [PubMed]
- Georgiadi A, Lichtenstein L, Degenhardt T, et al. Induction of cardiac Angptl4 by dietary fatty acids is mediated by peroxisome proliferator-activated receptor beta/delta and protects against fatty acid-induced oxidative stress. Circ Res 2010;106:1712-21. [Crossref] [PubMed]
- Zhu P, Goh YY, Chin HF, et al. Angiopoietin-like 4: a decade of research. Biosci Rep 2012;32:211-9. [Crossref] [PubMed]
- Shen CJ, Chan SH, Lee CT, et al. Oleic acid-induced ANGPTL4 enhances head and neck squamous cell carcinoma anoikis resistance and metastasis via up-regulation of fibronectin. Cancer Lett 2017;386:110-22. [Crossref] [PubMed]
- Shen CJ, Chang KY, Lin BW, et al. Oleic acid-induced NOX4 is dependent on ANGPTL4 expression to promote human colorectal cancer metastasis. Theranostics 2020;10:7083-99. [Crossref] [PubMed]
- Luo Q, Wang C, Jin G, et al. LIFR functions as a metastasis suppressor in hepatocellular carcinoma by negatively regulating phosphoinositide 3-kinase/AKT pathway. Carcinogenesis 2015;36:1201-12. [Crossref] [PubMed]
- Zhang F, Li K, Pan M, et al. miR-589 promotes gastric cancer aggressiveness by a LIFR-PI3K/AKT-c-Jun regulatory feedback loop. J Exp Clin Cancer Res 2018;37:152. [Crossref] [PubMed]
- Liu Q, Cao G, Wan Y, et al. Hsa_circ_0001073 targets miR-626/LIFR axis to inhibit lung cancer progression. Environ Toxicol 2021;36:1052-60. [Crossref] [PubMed]
- Hatakeyama S. TRIM Family Proteins: Roles in Autophagy, Immunity, and Carcinogenesis. Trends Biochem Sci 2017;42:297-311. [Crossref] [PubMed]
- Hatakeyama S. TRIM proteins and cancer. Nat Rev Cancer 2011;11:792-804. [Crossref] [PubMed]
- Zhao H, Huang J, Chen M, et al. Tripartite Motif Protein 6 Promotes Colorectal Cancer Cell Migration and Metastasis via SOCS2-STAT3 Signaling. Front Oncol 2021;11:695525. [Crossref] [PubMed]
- Montaudon E, Nikitorowicz-Buniak J, Sourd L, et al. PLK1 inhibition exhibits strong anti-tumoral activity in CCND1-driven breast cancer metastases with acquired palbociclib resistance. Nat Commun 2020;11:4053. [Crossref] [PubMed]
- Shin CH, Lee H, Kim HR, et al. Regulation of PLK1 through competition between hnRNPK, miR-149-3p and miR-193b-5p. Cell Death Differ 2017;24:1861-71. [Crossref] [PubMed]
- Wang B, Huang X, Liang H, et al. PLK1 Inhibition Sensitizes Breast Cancer Cells to Radiation via Suppressing Autophagy. Int J Radiat Oncol Biol Phys 2021;110:1234-47. [Crossref] [PubMed]
- Shin SB, Jang HR, Xu R, et al. Active PLK1-driven metastasis is amplified by TGF-beta signaling that forms a positive feedback loop in non-small cell lung cancer. Oncogene 2020;39:767-85. [Crossref] [PubMed]
- Zhang H, Zhao Y, Liu X, et al. High Expression of Complement Component C7 Indicates Poor Prognosis of Breast Cancer and Is Insensitive to Taxane-Anthracycline Chemotherapy. Front Oncol 2021;11:724250. [Crossref] [PubMed]
- Park JG, Choi BK, Lee Y, et al. Plasma complement C7 as a target in non-small cell lung cancer patients to implement 3P medicine strategies. EPMA J 2021;12:629-45. [Crossref] [PubMed]
- Mu D, Qin F, Li B, et al. Identification of the Sixth Complement Component as Potential Key Genes in Hepatocellular Carcinoma via Bioinformatics Analysis. Biomed Res Int 2020;2020:7042124. [Crossref] [PubMed]
- Shen H, Zhu M, Wang C. Precision oncology of lung cancer: genetic and genomic differences in Chinese population. NPJ Precis Oncol 2019;3:14. [Crossref] [PubMed]
- Vousden KH, Prives C. P53 and prognosis: new insights and further complexity. Cell 2005;120:7-10. [Crossref] [PubMed]
- McCuaig S, Barras D, Mann EH, et al. The Interleukin 22 Pathway Interacts with Mutant KRAS to Promote Poor Prognosis in Colon Cancer. Clin Cancer Res 2020;26:4313-25. [Crossref] [PubMed]
- Dong ZY, Zhong WZ, Zhang XC, et al. Potential Predictive Value of TP53 and KRAS Mutation Status for Response to PD-1 Blockade Immunotherapy in Lung Adenocarcinoma. Clin Cancer Res 2017;23:3012-24. [Crossref] [PubMed]
- Lohr M, Edlund K, Botling J, et al. The prognostic relevance of tumour-infiltrating plasma cells and immunoglobulin kappa C indicates an important role of the humoral immune response in non-small cell lung cancer. Cancer Lett 2013;333:222-8. [Crossref] [PubMed]
- Jin Y, Qin X. Profiles of immune cell infiltration and their clinical significance in head and neck squamous cell carcinoma. Int Immunopharmacol 2020;82:106364. [Crossref] [PubMed]
- Jairath NK, Farha MW, Srinivasan S, et al. Tumor Immune Microenvironment Clusters in Localized Prostate Adenocarcinoma: Prognostic Impact of Macrophage Enriched/Plasma Cell Non-Enriched Subtypes. J Clin Med 2020;9:1973. [Crossref] [PubMed]
- Liu X, Wu S, Yang Y, et al. The prognostic landscape of tumor-infiltrating immune cell and immunomodulators in lung cancer. Biomed Pharmacother 2017;95:55-61. [Crossref] [PubMed]
- Le T, Su S, Shahriyari L. Immune classification of osteosarcoma. Math Biosci Eng 2021;18:1879-97. [Crossref] [PubMed]
- Zhou CS, Feng MT, Chen X, et al. Exonuclease 1 (EXO1) is a Potential Prognostic Biomarker and Correlates with Immune Infiltrates in Lung Adenocarcinoma. Onco Targets Ther 2021;14:1033-48. [Crossref] [PubMed]
- Liu XS, Zhou LM, Yuan LL, et al. NPM1 Is a Prognostic Biomarker Involved in Immune Infiltration of Lung Adenocarcinoma and Associated With m6A Modification and Glycolysis. Front Immunol 2021;12:724741. [Crossref] [PubMed]
- Ma C, Kang W, Yu L, et al. AUNIP Expression Is Correlated With Immune Infiltration and Is a Candidate Diagnostic and Prognostic Biomarker for Hepatocellular Carcinoma and Lung Adenocarcinoma. Front Oncol 2020;10:590006. [Crossref] [PubMed]
- Yuan L, Wu X, Zhang L, et al. SFTPA1 is a potential prognostic biomarker correlated with immune cell infiltration and response to immunotherapy in lung adenocarcinoma. Cancer Immunol Immunother 2022;71:399-415. [Crossref] [PubMed]
- Yang Y, Hu Z, Sun H, et al. CBX7, a Potential Prognostic Biomarker in Lung Adenocarcinoma. Onco Targets Ther 2021;14:5477-92. [Crossref] [PubMed]
- Chlopik A, Selim MA, Peng Y, et al. Prognostic role of tumoral PDL1 expression and peritumoral FoxP3+ lymphocytes in vulvar melanomas. Hum Pathol 2018;73:176-83. [Crossref] [PubMed]
- Sacher AG, Gandhi L. Biomarkers for the Clinical Use of PD-1/PD-L1 Inhibitors in Non-Small-Cell Lung Cancer: A Review. JAMA Oncol 2016;2:1217-22. [Crossref] [PubMed]
- Chen Y, Li ZY, Zhou GQ, et al. An Immune-Related Gene Prognostic Index for Head and Neck Squamous Cell Carcinoma. Clin Cancer Res 2021;27:330-41. [Crossref] [PubMed]
- Jenkins RW, Barbie DA, Flaherty KT. Mechanisms of resistance to immune checkpoint inhibitors. Br J Cancer 2018;118:9-16. [Crossref] [PubMed]
- Thorsson V, Gibbs DL, Brown SD, et al. The Immune Landscape of Cancer. Immunity 2018;48:812-30 e14. [Crossref] [PubMed]
- Ayers M, Lunceford J, Nebozhyn M, et al. IFN-gamma-related mRNA profile predicts clinical response to PD-1 blockade. J Clin Invest 2017;127:2930-40. [Crossref] [PubMed]
- Jiang P, Gu S, Pan D, et al. Signatures of T cell dysfunction and exclusion predict cancer immunotherapy response. Nat Med 2018;24:1550-8. [Crossref] [PubMed]
- Zhu P, Teng Z, Yang W, et al. Construction of a prognostic model for lung adenocarcinoma based on membrane-tension-related genes. J Thorac Dis 2023;15:2098-115. [Crossref] [PubMed]
- Zhang M, Xiao Z, Xie Y, et al. A cuproptosis-related lncRNA signature-based prognostic model featuring on metastasis and drug selection strategy for patients with lung adenocarcinoma. Front Pharmacol 2023;14:1236655. [Crossref] [PubMed]