Development and validation of a prognostic model based on RNA binding proteins in patients with esophageal cancer
Highlight box
Key findings
• A novel signature based on five RNA-binding proteins (RBPs) can contribute to prognostic evaluation and prediction in patients with esophageal cancer (ESCA).
What is known and what is new?
• RBPs, which are key players in posttranscriptional events, play important roles in maintaining physiological homeostasis. They have been found to be involved in various biological processes of cancer.
• A nomogram for clinical use that integrated the five RBP signature and clinical factors was developed and validated using The Cancer Genome Atlas (TCGA) data set.
What is the implication, and what should change now?
• Identifying the RBP signature could improve our understanding of the molecular mechanisms underlying ESCA progression.
• The utility of the RBP signature should be validated in more data sets or in prospective research for further application.
Introduction
Esophageal cancer (ESCA), comprising squamous cell carcinoma and adenocarcinoma, is one of the most common cancer types worldwide and has the eighth highest incidence among malignancies in the United States, with an estimated 21,560 new cases predicted to be diagnosed in 2023 (1). Moreover, ESCA accounts for 2.6% of cancer deaths, with 16,120 patients predicted to die from this disease in the United States in 2023 (1). Most ESCA patients have progressed to an advanced stage at the time of diagnosis. Despite the improvements in treatment for ESCA over the last decades, it remains one of the most fatal malignancies, with an overall 5-year survival rate of only approximately 21% (1). The high mortality and low survival rate are the major challenges for the treatment of this disease. Therefore, it is still an important task in cancer research to explore novel biomarkers and new therapeutic targets.
RNA-binding proteins (RBPs) play important roles in maintaining physiological homeostasis and are key players in posttranscriptional events (2). RBPs are involved in the development and progression of various diseases, including cardiovascular disease, genetic disease, and neurodegenerative disorders (3,4). Perturbations in RBP-RNA network activity have been regarded as being associated with cancer development (5). According to a previous study, RBPs are abnormally expressed in colorectal cancer, affecting the translation of mRNAs into proteins and leading to carcinogenesis (6). The prognostic model based on RBPs also shows good predictive efficacy in colorectal cancer (7,8). Several studies have established prognostic models for ESCA, such as m6A methylation-associated genes-based model, immune-related gene prognostic model and etc. (9,10). Also, a potential prognostic model was also established through the combination of differentially expressed mRNAs and target genes of differentially expressed microRNAs (11). However, no mature prediction model is being used in clinical practice. Considering the vital role of RBPs in cancer, the construction of prognostic regulatory networks based on RBPs has also gradually attracted attention (12,13).
As far as ESCA is concerned, little is known about the roles of RBPs in the pathology. In the present study, we performed a systematic functional study of RBPs to explore their role in ESCA. We proposed and validated an individualized prognostic model based on RBPs for the overall survival (OS) of ESCA patients. We identified a number of ESCA-related RBPs and thus enhanced our knowledge of the molecular mechanisms underlying the development of ESCA. These RBPs may serve as potential diagnostic and prognostic indicators for ESCA. We present this article in accordance with the TRIPOD reporting checklist (available at https://jtd.amegroups.com/article/view/10.21037/jtd-23-1307/rc).
Methods
Data processing and functional enrichment analysis
RNA-sequencing (RNA-seq) data and clinical information of patients with ESCA (squamous cell carcinoma and adenocarcinoma) were obtained from The Cancer Genome Atlas (TCGA) (https://portal.gdc.cancer.gov/). Patients with incomplete follow-up information were excluded to reduce statistical bias in the subsequent analysis, and 158 primary ESCA and ten normal tissues were ultimately included in our study. The study was conducted in accordance with the Declaration of Helsinki (as revised in 2013).
The “limma” package in R software was used to identify the differentially expressed genes between tumor samples and normal samples from patients with ESCA (14). The differentially expressed RBPs that met the criteria of |log[fold change (FC)]| >0.5 and false discovery rate (FDR)-corrected P value <0.05 were identified as the significantly differentially expressed RBPs. In order to explore the biological functions of these genes, we applied Gene Ontology (GO) and Kyoto Encyclopedia of Genes and Genomes (KEGG) enrichment analysis based on the “clusterProfiler” package of R. GO terms and KEGG pathways with an FDR-adjusted P value <0.05 were retained.
Protein-protein interaction (PPI) network construction and module screening
PPI networks are composed of proteins that interact with each other to participate in various aspects of life processes such as biological signal transmission, gene expression regulation, energy and material metabolism, and cell cycle regulation. To comprehend how proteins function in biological systems, it is crucial to conduct a systematic examination of the interactions of numerous proteins in biological systems (15). To obtain PPI information, the significantly differentially expressed genes were uploaded to the STRING database (http://www.string-db.org/) (16). The PPI network was constructed and visualized using Cytoscape 3.7.2 software (17).
Construction and validation of an RBP-related prognostic signature
The “Caret” package of R was employed to randomly dichotomize the entire cohort of ESCA patients into the training and test data sets (18,19). We performed univariate Cox regression analysis to select RBPs that were significantly associated with ESCA patient OS in the training data set. We then constructed a risk score using multivariate Cox regression analysis to evaluate the prognostic outcomes of patients in the training data set. The risk score formula was established as follows: risk scores = Vi × Ci (Vi is the expression value of a gene, Ci represents the regression coefficient of a gene), summed for each gene considered in the signature (20). All patients in the training data set, test data set, and entire data set were dichotomized into high- and low-risk groups by the median risk cutoff value. The “survivalROC” package of R was utilized to evaluate the value of the prognostic model for 1-year survival through the area under the curve (AUC) value of the receiver operating characteristic (ROC) curve (21). With the AUC ranging from 0 to 1, a higher value of AUC indicates better model prediction performance (AUC =0.5 means random prediction) and AUC >0.7 means that the model has good predictive ability.
Statistical analysis
Cox regression analysis was performed using the “survival” package (22). Normalization and differential expression analysis were carried out using the “limma” package. All statistical analyses were implemented based on R software (version 3.6.3). An FDR-corrected P value <0.05 was considered statistically significant.
Results
Differentially expressed RBPs in ESCA
To obtain the differentially expressed RBPs, we analyzed the expression of 1,542 RBPs (3) in 158 primary ESCA and ten nontumor tissues using the “limma” package in R; the expression patterns of the whole set of RBPs are shown in Figure 1A. After statistical analysis, 255 RBPs, namely, 109 downregulated RBPs and 146 upregulated RBPs, were eventually identified using the criteria of |log(FC)| >0.5 and FDR-corrected P value <0.05 (Figure 1B, tables available at https://cdn.amegroups.cn/static/public/jtd-23-1307-1.xlsx and https://cdn.amegroups.cn/static/public/jtd-23-1307-2.xlsx).
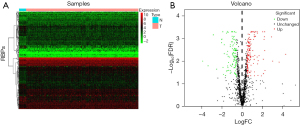
Functional enrichment analysis of the differentially expressed RBPs
To investigate the functions and mechanisms of the identified RBPs, the differentially expressed RBPs were separated into up- and downregulated expression groups and further subjected to functional enrichment analysis. The results of GO functional and KEGG pathway enrichment analyses of these genes are summarized in Table 1. For the upregulated differentially expressed RBPs, the top enriched GO biological process terms were non-coding RNA (ncRNA) processing, ribosome biogenesis, and ribosomal RNA (rRNA) metabolic process; the top enriched GO cellular component terms were spliceosomal complex, U2-type spliceosomal complex, and U2-type precatalytic spliceosome; and the top enriched GO molecular function terms were catalytic activity acting on RNA, ribonuclease activity, and ribonucleoprotein complex binding (Table 1). The downregulated differentially expressed RBPs were significantly enriched in (I) the GO biological process terms related to the regulation of translation, regulation of cellular amide metabolic process, and regulation of mRNA metabolic process; (II) the GO cellular component terms ribosome, ribosomal subunit, and cytoplasmic ribonucleoprotein granule; and (III) the GO molecular function terms translation regulator activity, nucleic acid binding, translation regulator activity, translation repressor activity, and mRNA regulatory element binding (Table 1). Additionally, in the KEGG pathway enrichment analysis for the differentially expressed RBPs, it was discovered that the genes encoding the upregulated RBPs were significantly related to the spliceosome, RNA transport, and ribosome biogenesis in eukaryotes pathways and that those encoding the downregulated RBPs were significantly related to the pathways ribosome and progesterone-mediated oocyte maturation (Table 1). Most of these pathways were involved in RNA-related pathways, which were consistent with the function of these genes themselves.
Table 1
RBPs | Category | ID | Term | P value | FDR |
---|---|---|---|---|---|
Up-regulated RBPs | Biological process | GO:0034470 | ncRNA processing | 3.83e−32 | 5.09e−29 |
Biological process | GO:0042254 | Ribosome biogenesis | 7.04e−27 | 4.68e−24 | |
Biological process | GO:0016072 | rRNA metabolic process | 5.21e−25 | 2.31e−22 | |
Biological process | GO:0031123 | RNA 3'-end processing | 5.71e−24 | 1.90e−21 | |
Biological process | GO:0006913 | Nucleocytoplasmic transport | 9.77e−24 | 2.60e−21 | |
Cellular component | GO:0005681 | Spliceosomal complex | 2.02e−19 | 2.91e−17 | |
Cellular component | GO:0005684 | U2-type spliceosomal complex | 3.01e−18 | 2.17e−16 | |
Cellular component | GO:0071005 | U2-type precatalytic spliceosome | 2.26e−17 | 1.09e−15 | |
Cellular component | GO:0071011 | Precatalytic spliceosome | 3.02e−17 | 1.09e−15 | |
Cellular component | GO:0071013 | Catalytic step 2 spliceosome | 5.78e−17 | 1.67e−15 | |
Molecular function | GO:0140098 | Catalytic activity, acting on RNA | 6.29e−26 | 8.67e−24 | |
Molecular function | GO:0004540 | Ribonuclease activity | 3.77e−13 | 2.60e−11 | |
Molecular function | GO:0043021 | Ribonucleoprotein complex binding | 5.14e−11 | 2.36e−09 | |
Molecular function | GO:0004518 | Nuclease activity | 1.10e−09 | 3.78e−08 | |
Molecular function | GO:0004521 | Endoribonuclease activity | 4.27e−08 | 1.18e−06 | |
KEGG pathway | hsa03040 | Spliceosome | 4.03e−19 | 1.74e−17 | |
KEGG pathway | hsa03013 | RNA transport | 6.10e−15 | 1.24e−13 | |
KEGG pathway | hsa03008 | Ribosome biogenesis in eukaryotes | 8.64e−15 | 1.24e−13 | |
KEGG pathway | hsa03015 | mRNA surveillance pathway | 2.45e−07 | 2.64e−06 | |
KEGG pathway | hsa03010 | Ribosome | 9.46e−04 | 8.17e−03 | |
Down-regulated RBPs | Biological process | GO:0006417 | Regulation of translation | 1.81e−21 | 2.73e−18 |
Biological process | GO:0034248 | Regulation of cellular amide metabolic process | 5.34e−20 | 4.03e−17 | |
Biological process | GO:1903311 | Regulation of mRNA metabolic process | 3.87e−18 | 1.95e−15 | |
Biological process | GO:0043484 | Regulation of RNA splicing | 2.96e−17 | 1.12e−14 | |
Biological process | GO:0006401 | RNA catabolic process | 3.71e−15 | 1.12e−12 | |
Cellular component | GO:0005840 | Ribosome | 2.22e−09 | 2.57e−07 | |
Cellular component | GO:0044391 | Ribosomal subunit | 8.03e−07 | 4.65e−05 | |
Cellular component | GO:0036464 | Cytoplasmic ribonucleoprotein granule | 2.00e−06 | 6.58e−05 | |
Cellular component | GO:0022626 | Cytosolic ribosome | 2.27e−06 | 6.58e−05 | |
Cellular component | GO:0035770 | Ribonucleoprotein granule | 3.02e−06 | 7.00e−05 | |
Molecular function | GO:0090079 | Translation regulator activity, nucleic acid binding | 4.12e−18 | 6.12e−16 | |
Molecular function | GO:0045182 | Translation regulator activity | 2.45e−17 | 1.82e−15 | |
Molecular function | GO:0000900 | Translation repressor activity, mRNA regulatory element binding | 1.40e−12 | 6.71e−11 | |
Molecular function | GO:0030371 | Translation repressor activity | 1.81e−12 | 6.71e−11 | |
Molecular function | GO:0008135 | Translation factor activity, RNA binding | 3.09e−12 | 9.17e−11 | |
KEGG pathway | hsa03010 | Ribosome | 8.98e−06 | 5.39e−04 | |
KEGG pathway | hsa04914 | Progesterone-mediated oocyte maturation | 1.28e−03 | 3.84e−02 |
RBPs, RNA-binding proteins; FDR, false discovery rate; GO, Gene Ontology; ncRNA, non-coding RNA; rRNA, ribosomal RNA; KEEG, Kyoto Encyclopedia of Genes and Genome.
PPIs among the differentially expressed RBPs
To explore the potential interactions among these differentially expressed RBPs in ESCA, we generated a PPI network consisting of 237 nodes and 2,305 edges using the Cytoscape 3.72 software and the STRING database (Figure 2A). The co-expression network was processed via the MCODE tool to identify possible key modules and the first key module acquired, which included 47 hub RBPs (Figure 2B). The RBPs in the first key module were highly enriched in the spliceosome, ribosome biogenesis in eukaryotes, mRNA surveillance pathway, and RNA transport pathways.
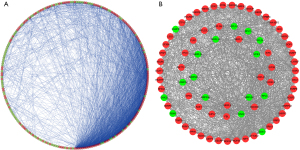
Construction of the RBP prognostic-related risk score model in the training data set
The entire cohort (n=158) of patients with complete survival information and RNA-seq expression profiles was randomly divided into training (n=80) and test (n=78) data sets based on the R package of “caret”. The information of patients with ESCA is summarized in a previous study and no differences in baseline characteristics in patients were found between the two groups (19). In the training set, we performed univariate Cox regression analysis based on the 47 hub RBPs. A total of 18 RBPs significantly associated with OS (P<0.05) were considered prognostic candidate hub RBPs for further analysis (Figure 3A). The five hub RBPs identified from multivariate Cox regression analysis were used to construct the prediction model (Figure 3B, Table 2). The risk scores for predicting the prognostic risk in ESCA patients were calculated with the following formula (see Methods section): risk score = (−0.7174 × ExpTNRC6A) + (0.1323 × ExpCLK1) + (−0.1743 × ExpCIRBP) + (0.2425 × ExpTYW3) + (0.2258 × ExpMRPL13). The expression of these five RBPs is shown in the online table (available at https://cdn.amegroups.cn/static/public/jtd-23-1307-3.xlsx). In addition, the results of testing the proportional hazards (PH) assumption in the Cox regression model demonstrated that all the P values were higher than 0.05, which means that all data satisfied the PH assumption (Table 3). We then dichotomized the ESCA patients into the high- and low-risk groups according to the median risk score. The Kaplan-Meier curves indicated that the high-risk group had poor OS prognoses compared to those of the low-risk group in the training data set (P<0.001; Figure 4A). Time-dependent ROC analysis was executed to further estimate the prognostic ability of the five-RBP signature. The ROC curves of the RBP signature model are shown in Figure 4B, indicating the AUC of 0.789 for 1-year survival, indicating that the model has moderate diagnostic performance. The relative expression standards of the five RBPs for each patient are shown in Figure 4C. The distribution of risk grades between the low- and high-risk groups is depicted in Figure 4D, and the survival status and survival time of patients in the two different risk groups are shown in Figure 4E.
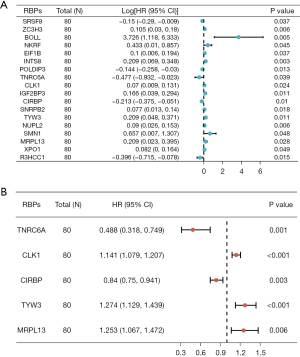
Table 2
RBPs | Coefficient | HR | Lower 95% CI | Upper 95% CI | P value |
---|---|---|---|---|---|
TNRC6A | −0.7174 | 0.4880 | 0.3181 | 0.7488 | 1.02e−03 |
CLK1 | 0.1323 | 1.1414 | 1.0793 | 1.2071 | 3.58e−06 |
CIRBP | −0.1743 | 0.8400 | 0.7498 | 0.9411 | 2.63e−03 |
TYW3 | 0.2425 | 1.2744 | 1.1289 | 1.4386 | 8.84e−05 |
MRPL13 | 0.2259 | 1.2534 | 1.0672 | 1.4722 | 5.93e−03 |
RBPs, RNA binding proteins; HR, hazard ratio; CI, confidence interval.
Table 3
RBP | Chisq | P value |
---|---|---|
TNRC6A | 1.06 | 0.3 |
CLK1 | 2.29 | 0.13 |
CIRBP | 1.15 | 0.28 |
TYW3 | 1.37 | 0.24 |
MRPL13 | 1.3 | 0.26 |
Global | 5.48 | 0.36 |
RBP, RNA-binding protein; Chisq, Chi-square test.
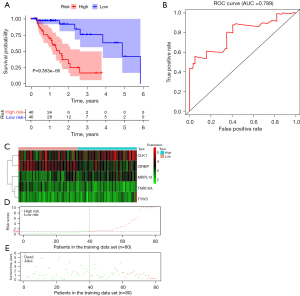
Validation of the RBP prognostic signature
To evaluate the robustness of the five-RBP signature, its prognostic value was further validated in the test data set and the entire data set. The prognostic risk scores of patients in the test cohort and in the entire data set were also calculated based on the expression values of the five prognostic RBPs, according to the previous formula (see Methods section). Moreover, each patient was classified as high- or low-risk by comparing this patient’s risk score with the median risk score calculated in the training data set. The Kaplan-Meier survival curves for patients dichotomized by the risk score also showed significant differences in survival between the two predicted risk groups in the test data set (P=1.792e−02; Figure 5A) and in the entire data set (P=3.131e−06; Figure 5B). The ROC curve of the OS-related predictive signature was further generated in the test data set, in which it had an AUC of 0.753, and in the entire data set, in which it had an AUC of 0.764 (Figure 5C,5D). The distribution of patient risk scores, patient survival statuses, and expression levels of five prognostic RBPs in the test data set (Figure 5E) and the entire data set (Figure 5F) are also shown. CLK1, CIRBP, and MRPL3 were relatively highly expressed in low-risk patients. In addition, patients in high-risk groups had a higher death rate than those in low-risk groups.
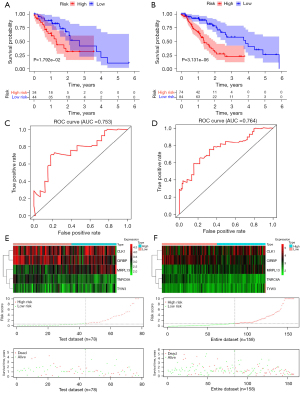
Finally, multivariate Cox regression analyses were performed incorporating age, grade, stage (T, N, M), and risk score as covariables. The results revealed that the five-RBP risk score was significantly associated with OS in training, test and entire data set (Figure 6A-6C, respectively), thus representing a potential prognostic marker for ESCA in the future.
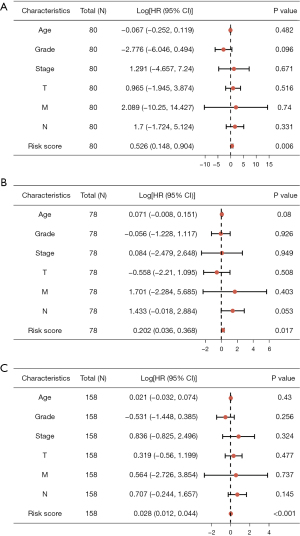
Construction of a nomogram integrating the risk model with clinical parameters
To obtain a quantitative method for ESCA prognosis, we constructed a nomogram by integrating the five-RBP signature and clinical parameters (age, sex, stage T, N, M). Horizontal lines were drawn to determine the points assigned for the five-RBP risk score and each clinical parameter. By calculating the total score of each patient by summing the points of all variables, we could estimate the 1-, 2-, and 3-year survival probability of each ESCA patient (Figure 7). For example, a 60-year-old female patient staged I (T1, N0, M0) and she was high-risk based on the previous formula (see Material and Methods section). Her total score was about 90 points and the 2- and 3-year survival rate was about 86% and 75%, respectively. The establishment of the nomogram made the five-RBP signature more convenient for clinical application.
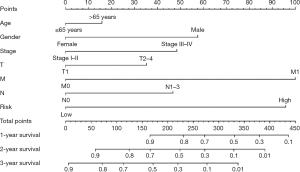
Discussion
RBPs are involved in several aspects of RNA biogenesis, including splicing, localization, stability, and translation efficiency (3). Recent research has shown that RBPs are abnormally expressed in cancer tissues relative to adjacent normal tissues and that their expression is associated with the prognosis of patients (12,13). High-throughput bioinformatic analysis of thousands of paired tumor and normal samples from the TCGA database revealed a consistent pattern of alterations in RBP expression levels across several cancer types (23,24). In recent years, it has become apparent that the control of gene expression by RBPs is of vital importance in the majority of cellular signaling pathways, extending our understanding of its mechanism as clinical therapeutic targets (25). However, only a small fraction of RBPs have been deeply studied during the development of cancer (26-28). In this study, a total of 255 differentially expressed RBPs were identified between ESCA and normal esophageal tissues. We systematically explored the potential biological functional pathways and then constructed a PPI network based on these differentially expressed RBPs. Additionally, univariate Cox regression analysis, Kaplan-Meier survival analysis, multivariate Cox regression analysis, and ROC analysis were further used to explore their biological functions and clinical significance. These findings may contribute to the development of potential biomarkers and therapeutic targets for ESCA.
Our present study showed that the differentially expressed RBPs in ESCA were significantly enriched in the terms ncRNA processing, ribosome biogenesis, rRNA metabolic process, ribonucleoprotein complex binding, regulation of translation, regulation of cellular amide metabolic process, and regulation of mRNA metabolic process. Several studies have shown that the regulation of translation and RNA metabolic processes has been increasingly recognized to be involved in the development of several diseases (29,30). It has been reported that ncRNAs may become novel biomarkers and therapeutic targets for cancer progression (31). RBPs can interact with mRNAs and then form ribonucleoprotein complexes and regulate the expression of the mRNAs by increasing mRNA stability, a process that plays important roles in the development of many diseases. The RBP NONO has recently been found to promote breast cancer proliferation by posttranscriptional regulation of SKP2 and E2F8 (32). In addition, a study indicated that RBPs in the nucleus play key roles in regulating the mRNA alternative splicing process and result in alterations in the expression of tumor-associated genes (33). KEGG pathway analysis suggested that aberrantly expressed RBPs regulate tumorigenesis and the development of ESCA by influencing the spliceosome, RNA transport, and ribosome biogenesis. Our results improved the understanding of the molecular mechanism of ESCA initiation and progression.
After multivariate Cox regression analysis was performed in the training data set, five key RBP genes associated with prognosis were selected: CLK1, CIRBP, MRPL13, TNRC6A, and TYW3. Most of these genes have been shown to play crucial roles in the development and progression of tumors (34,35). CLK1 is involved in the development of gastric cancer and prostate cancer and might be a novel therapeutic target (36,37). CIRBP has been found in cancer and inflammation and is also regarded as a novel oncogene in cancer (38,39). It has been found that CIRBP can directly bind to p53 and then regulate ferroptosis and the growth of pancreatic cancer cells (40). Previous studies have identified MRPL13 as a novel candidate gene associated with breast cancer prognosis (41,42). A recent study indicated that the miR-30/CHD7/TNRC6A pathway is potentially a novel diagnostic biomarker and therapeutic target for cancer (43). TNRC6A is also a downstream target of miR-185-5p and plays an important role in the proliferation and apoptosis of non-small cell lung cancer (44). These results are consistent with our present findings that these hub RBPs play important roles in tumorigenesis and might be new therapeutic targets for ESCA.
Subsequently, a prognostic signature was constructed based on the five key RBPs, namely, CLK1, CIRBP, MRPL13, TNRC6A, and TYW3, and was further validated in the test and entire data sets. ROC curve analysis revealed that the RBP-related signature could improve the diagnosis and assessment of ESCA patients with poor prognosis. Through the nomogram that integrated the risk model with clinical parameters, the 1-, 2-, and 3-year OS probabilities of ESCA patients could be predicted more intuitively. This means that the prognostic model may have a certain value for adjusting the treatment plans of ESCA patients. In addition, the five key RBPs may play important roles in the progression of ESCA. The intervention of these five proteins or the exploration of new targeted drugs is expected to improve the prognosis of patients with ESCA. However, the molecular mechanism by which these RBPs contribute to esophageal carcinogenesis needs to be further explored.
Our present study indicated that the prognostic signature based on RBPs might be applied for the prediction of survival in ESCA patients, thereby potentially being used to assist clinical treatment and improve the outcomes of ESCA patients. However, there are still some limitations to the present study. First, our results were only based on the TCGA database and need to be further verified in prospective studies for clinical use. Second, additional investigations should be conducted to further explore the molecular mechanisms of these RBP hub genes in the development and progression of ESCA. Also, we did not investigate the potential function of RBPs in ESCA with different pathologies due to the limited sample size. We hope to increase the number of patients in future study to explore the application of the RBP-related signature in ESCA patients with different pathologies. Finally, prospective research should be performed to verify the outcomes.
Conclusions
We constructed and validated a novel prognostic signature based on RBPs that could serve as an independent prognostic factor for ESCA and improve individualized outcome predictions. Our results have shown great potential for the identification of new prognostic biomarkers and therapeutic targets for ESCA patients.
Acknowledgments
We would like to thank TCGA project organizers as well as all study participants for providing the publicly available TCGA RNA-seq data and clinical data.
Funding: None.
Footnote
Reporting Checklist: The authors have completed the TRIPOD reporting checklist. Available at https://jtd.amegroups.com/article/view/10.21037/jtd-23-1307/rc
Peer Review File: Available at https://jtd.amegroups.com/article/view/10.21037/jtd-23-1307/prf
Conflicts of Interest: All authors have completed the ICMJE uniform disclosure form (available at https://jtd.amegroups.com/article/view/10.21037/jtd-23-1307/coif). The authors have no conflicts of interest to declare.
Ethical Statement: The authors are accountable for all aspects of the work in ensuring that questions related to the accuracy or integrity of any part of the work are appropriately investigated and resolved. The study was conducted in accordance with the Declaration of Helsinki (as revised in 2013).
Open Access Statement: This is an Open Access article distributed in accordance with the Creative Commons Attribution-NonCommercial-NoDerivs 4.0 International License (CC BY-NC-ND 4.0), which permits the non-commercial replication and distribution of the article with the strict proviso that no changes or edits are made and the original work is properly cited (including links to both the formal publication through the relevant DOI and the license). See: https://creativecommons.org/licenses/by-nc-nd/4.0/.
References
- Siegel RL, Miller KD, Wagle NS, et al. Cancer statistics, 2023. CA Cancer J Clin 2023;73:17-48. [Crossref] [PubMed]
- Shi DL, Cheng XN, Saquet A, et al. Emerging Roles of RNA-Binding Proteins in Inner Ear Hair Cell Development and Regeneration. Int J Mol Sci 2022;23:12393. [Crossref] [PubMed]
- Gerstberger S, Hafner M, Tuschl T. A census of human RNA-binding proteins. Nat Rev Genet 2014;15:829-45. [Crossref] [PubMed]
- Gebauer F, Schwarzl T, Valcárcel J, et al. RNA-binding proteins in human genetic disease. Nat Rev Genet 2021;22:185-98. [Crossref] [PubMed]
- Wang S, Sun Z, Lei Z, et al. RNA-binding proteins and cancer metastasis. Semin Cancer Biol 2022;86:748-68. [Crossref] [PubMed]
- Chatterji P, Rustgi AK. RNA Binding Proteins in Intestinal Epithelial Biology and Colorectal Cancer. Trends Mol Med 2018;24:490-506. [Crossref] [PubMed]
- Luan L, Lu F, Wang X, et al. The predictive value of RNA binding proteins in colon adenocarcinoma. J Gastrointest Oncol 2021;12:1543-57. [Crossref] [PubMed]
- Liu X, Lv Q, Jing Z, et al. Construction of a prognostic risk model of colorectal adenocarcinoma through integrated analysis of RNA-binding proteins. Transl Cancer Res 2021;10:1962-74. [Crossref] [PubMed]
- Guo C, Zeng F, Liu H, et al. Establish immune-related gene prognostic index for esophageal cancer. Front Genet 2022;13:956915. [Crossref] [PubMed]
- Pu Y, Lu X, Yang X, et al. Estimating the prognosis of esophageal squamous cell carcinoma based on The Cancer Genome Atlas (TCGA) of m6A methylation-associated genes. J Gastrointest Oncol 2022;13:1-12. [Crossref] [PubMed]
- Gu B, Zhang S, Fan Z, et al. Prognostic model construction and immune microenvironment analysis of esophageal cancer based on gene expression data and microRNA target genes. Transl Cancer Res 2023;12:1165-74. [Crossref] [PubMed]
- Zhao S, Liu Q, Li J, et al. Construction and Validation of Prognostic Regulation Network Based on RNA-Binding Protein Genes in Lung Squamous Cell Carcinoma. DNA Cell Biol 2021;40:1563-83. [Crossref] [PubMed]
- Zhen J, Song Z, Su W, et al. Integrated analysis of RNA-binding proteins in thyroid cancer. PLoS One 2021;16:e0247836. [Crossref] [PubMed]
- Smyth GK. Linear models and empirical bayes methods for assessing differential expression in microarray experiments. Stat Appl Genet Mol Biol 2004;3:Article3.
- Hu X, Ni S, Zhao K, et al. Bioinformatics-Led Discovery of Osteoarthritis Biomarkers and Inflammatory Infiltrates. Front Immunol 2022;13:871008. [Crossref] [PubMed]
- Szklarczyk D, Franceschini A, Wyder S, et al. STRING v10: protein-protein interaction networks, integrated over the tree of life. Nucleic Acids Res 2015;43:D447-52. [Crossref] [PubMed]
- Shannon P, Markiel A, Ozier O, et al. Cytoscape: a software environment for integrated models of biomolecular interaction networks. Genome Res 2003;13:2498-504. [Crossref] [PubMed]
- Kuhn M. Building Predictive Models in R Using the caret Package. J Stat Softw 2008;28:1-26.
- Du H, Pang S, Li Y, et al. The prognostic value of an immune-related gene signature and infiltrating tumor immune cells based on bioinformatics analysis in primary esophageal cancer. J Gastrointest Oncol 2022;13:1556-70. [Crossref] [PubMed]
- Wang BD, Ceniccola K, Hwang S, et al. Alternative splicing promotes tumour aggressiveness and drug resistance in African American prostate cancer. Nat Commun 2017;8:15921. [Crossref] [PubMed]
- Saha-Chaudhuri P. survivalROC: Time-Dependent ROC Curve Estimation From Censored Survival Data [Internet]. R Package Version 103; 2013.
- Therneau T. A Package for Survival Analysis in R [Internet]. R Package Version 32-10; 2021.
- Yao ZT, Yang YM, Sun MM, et al. New insights into the interplay between long non-coding RNAs and RNA-binding proteins in cancer. Cancer Commun (Lond) 2022;42:117-40. [Crossref] [PubMed]
- Hashimoto S, Kishimoto T. Roles of RNA-binding proteins in immune diseases and cancer. Semin Cancer Biol 2022;86:310-24. [Crossref] [PubMed]
- Wang N, Qiao H, Hao J, et al. RNA-binding protein ENO1 promotes the tumor progression of gastric cancer by binding to and regulating gastric cancer-related genes. J Gastrointest Oncol 2023;14:585-98. [Crossref] [PubMed]
- Ke J, Ni K, Xue H, et al. RBM38 is negatively regulated by miR-320b and enhances Adriamycin resistance in breast cancer cells. Oncol Lett 2022;23:27. [Crossref] [PubMed]
- Loh XY, Sun QY, Ding LW, et al. RNA-Binding Protein ZFP36L1 Suppresses Hypoxia and Cell-Cycle Signaling. Cancer Res 2020;80:219-33. [Crossref] [PubMed]
- Hu X, Harvey SE, Zheng R, et al. The RNA-binding protein AKAP8 suppresses tumor metastasis by antagonizing EMT-associated alternative splicing. Nat Commun 2020;11:486. [Crossref] [PubMed]
- Jain A, Brown SZ, Thomsett HL, et al. Evaluation of Post-transcriptional Gene Regulation in Pancreatic Cancer Cells: Studying RNA Binding Proteins and Their mRNA Targets. Methods Mol Biol 2019;1882:239-52. [Crossref] [PubMed]
- Hinkle ER, Wiedner HJ, Black AJ, et al. RNA processing in skeletal muscle biology and disease. Transcription 2019;10:1-20. [Crossref] [PubMed]
- Yan H, Bu P. Non-coding RNA in cancer. Essays Biochem 2021;65:625-39. [Crossref] [PubMed]
- Iino K, Mitobe Y, Ikeda K, et al. RNA-binding protein NONO promotes breast cancer proliferation by post-transcriptional regulation of SKP2 and E2F8. Cancer Sci 2020;111:148-59. [Crossref] [PubMed]
- Shao Y, Chong W, Liu X, et al. Alternative splicing-derived intersectin1-L and intersectin1-S exert opposite function in glioma progression. Cell Death Dis 2019;10:431. [Crossref] [PubMed]
- Li W, Deng X, Chen J. RNA-binding proteins in regulating mRNA stability and translation: roles and mechanisms in cancer. Semin Cancer Biol 2022;86:664-77. [Crossref] [PubMed]
- Mehta M, Raguraman R, Ramesh R, et al. RNA binding proteins (RBPs) and their role in DNA damage and radiation response in cancer. Adv Drug Deliv Rev 2022;191:114569. [Crossref] [PubMed]
- Babu N, Pinto SM, Biswas M, et al. Phosphoproteomic analysis identifies CLK1 as a novel therapeutic target in gastric cancer. Gastric Cancer 2020;23:796-810. [Crossref] [PubMed]
- Bowler E, Porazinski S, Uzor S, et al. Hypoxia leads to significant changes in alternative splicing and elevated expression of CLK splice factor kinases in PC3 prostate cancer cells. BMC Cancer 2018;18:355. [Crossref] [PubMed]
- He Q, Gao H, Chang YL, et al. ETS-1 facilitates Th1 cell-mediated mucosal inflammation in inflammatory bowel diseases through upregulating CIRBP. J Autoimmun 2022;132:102872. [Crossref] [PubMed]
- Liao Y, Feng J, Sun W, et al. CIRP promotes the progression of non-small cell lung cancer through activation of Wnt/β-catenin signaling via CTNNB1. J Exp Clin Cancer Res 2021;40:275. [Crossref] [PubMed]
- Gao H, Xie R, Huang R, et al. CIRBP Regulates Pancreatic Cancer Cell Ferroptosis and Growth by Directly Binding to p53. J Immunol Res 2022;2022:2527210. [Crossref] [PubMed]
- Wang K, Li L, Fu L, et al. Integrated Bioinformatics Analysis the Function of RNA Binding Proteins (RBPs) and Their Prognostic Value in Breast Cancer. Front Pharmacol 2019;10:140. [Crossref] [PubMed]
- Xu YH, Deng JL, Wang LP, et al. Identification of Candidate Genes Associated with Breast Cancer Prognosis. DNA Cell Biol 2020;39:1205-27. [Crossref] [PubMed]
- Su W, Hong L, Xu X, et al. miR-30 disrupts senescence and promotes cancer by targeting both p16(INK4A) and DNA damage pathways. Oncogene 2018;37:5618-32. [Crossref] [PubMed]
- Bian WX, Xue F, Wang LY, et al. Circular RNA CircCDYL Regulates Proliferation and Apoptosis in Non-Small Cell Lung Cancer Cells by Sponging miR-185-5p and Upregulating TNRC6A. Cancer Manag Res 2021;13:633-42. [Crossref] [PubMed]