Derivation and external validation of a nomogram predicting the occurrence of severe illness among hospitalized coronavirus disease 2019 patients: a 2020 Chinese multicenter retrospective study
Highlight box
Key findings
• Hypertension, shortness of breath, days between illness onset and diagnosis, platelet count, alanine aminotransferase, potassium, cardiac troponin I, myohemoglobin and procalcitonin were determinants of progression to severe coronavirus disease 2019 (COVID-19). The nomogram showed good performance.
What is known and what is new?
• Previous studies have reported risk factors associated with poor outcomes in COVID-19 patients. However, many of them incorporated variables that are not routinely available at admission.
• We built a nomogram using easily available indicators at admission to identify COVID-19 patients tending to develop severe disease based on multi-center cohorts. Especially, we found potassium is a determinant of severity and present a dose-response relationship.
What is the implication, and what should change now?
• This model showed excellent performance in predicting the risk of progression to severe COVID-19, thereby helping doctors to identify patients with high risk of developing severe illness at an early stage.
Introduction
The coronavirus disease 2019 (COVID-19) has posed challenge to global emergency response since being openly declared as a worldwide pandemic by the World Health Organization in March 2020 and currently continues to devastate much of the world (1,2). Although most COVID-19 cases manifest mild symptoms or asymptomatic infection and show a relatively good prognosis, COVID-19 can also deteriorate into a severe or critical illness, lead to a distinct surge in need for bed occupation, intensive care units and mechanical ventilation or reach the endpoint of death (3,4). In light of the data, about 20.7% to 31.4% of COVID-19 patients needed to be admitted, 4.9% to 11.5% were characterized as severe and 1.8% to 3.4% were classified as death cases (5). As of February 1, 2023, there have been approximately 754 million reported COVID-19 cases globally and 6.8 million reported deaths attributed to COVID-19, with an average fatality of 0.9% (6). Many countries have suffered from critical medical overstretch caused successively by the original severe acute respiratory coronavirus type 2 (SARS-CoV-2) outbreak in 2020 and the new Alpha, Delta and Omicron variant surges. During the pandemic, early identifying COVID-19 cases with risk of progression to severity is very important and will be in favor of deciding a therapeutic regimen. Previous researches have reported adverse factors relevant to negative consequences in COVID-19 patients such as higher score of Sequential Organ Failure Assessment (SOFA), concurrent diseases, the elderly, lymphocytopenia, neutrophilia, coagulopathy and elevated D-dimer level (7-12). However, many of these studies incorporated variables that were not routinely available, or not easily used indicators at admission, or were not measured consistently across hospitals or were conducted in a single hospital, lacking external validation. Thus, there are concerns about easy implementation and generalizability in regard to predicting the severity risk at admission.
Nomograms allow for evidence-based and individualized risk prediction and have been increasingly used to predict the prognosis of diseases (13). In our study, we aimed to construct a simple nomogram which could early forecast the severity of COVID-19 using readily available indicators at admission from those COVID-19 patients infected with the original SARS-CoV-2 and contribute to clinicians quickly deciding on better treatment strategies and improving survival for this vulnerable population. We present this article in accordance with the STROBE reporting checklist (available at https://jtd.amegroups.com/article/view/10.21037/jtd-23-653/rc).
Methods
Study design and participants
This multicenter, retrospective research enrolled consecutively laboratory-diagnosed COVID-19 cases who were admitted to four designated hospitals in China: Tongji Hospital affiliated with Huazhong University of Science and Technology (HUST), Wuhan Third Hospital of Wuhan University and Wuhan Jinyintan Hospital in Hubei province, and Public Health Clinical Center of Shanghai, between January 1, 2020 and March 8, 2020. Since COVID-19 was an urgent crisis in China at that time, all suspected and confirmed COVID-19 patients must receive treatment in isolation at designated hospitals according to the regulation of the National Health Commission. Meanwhile, discharge criteria should meet negative nucleic acid tests for two consecutive respiratory specimens (sampling interval 1 day), significant improvement of respiratory symptoms and normal body temperature for 3 consecutive days. The inclusion criteria were as follows: age ≥18 years; firstly diagnosed with COVID-19; confirmed infection of original SARS-CoV-2 strains by real-time reverse transcription polymerase chain reaction (RT-PCR) assay using specimens of pharyngeal or nasal swab based on the Guideline on the Prevention and Control for COVID-19 issued by the National Health Commission (Version 5) (14); no severe pneumonia on admission; full baseline, laboratory tests, and follow-up data with a definite endpoint.
Data collection
A self-designed case report form was adopted to extract data from the health information system of each hospital by trained abstracters, and the data were reviewed by research team. Potential predictive variables included baseline data, chest imaging results, laboratory findings, and signs and symptoms on admission. Baseline data, including demographic characteristics and comorbidities, were collected. Laboratory examinations, such as complete blood count, electrolytes, lactate dehydrogenase (LDH), creatine kinase, kidney and liver functions, D-dimer, C-reactive protein (CRP), fibrinogen, procalcitonin (PCT) and myocardial enzymes, were conducted within 24 hours of admission. Two experienced researchers cross-checked all the data.
Definition of outcome
The development of severe conditions after admission was defined as the primary outcome. In our study, the criteria for diagnosing severe COVID-19 pertained to the Guideline on the Diagnosis and Treatment for COVID-19 (Trial Version 6) by the National Health Commission, in which COVID-19 cases were classified into mild, moderate, severe and critical groups (15). The definition of severe COVID-19 encompassed condition deterioration from mild or moderate to severe or critical pneumonia in the period of hospital stay and could be identified as a composite event meeting at least one of the following criteria: a ratio of PaO2 to FiO2 less than or equal to 300 mmHg; oxygen saturations ≤93 percent at rest; respiratory rate ≥30 times per minute; shock; respiratory failure needing mechanical ventilation; and combined with other organ failure requiring intensive care. All patients were followed up until death or discharge.
Statistical analysis
Continuous variables were expressed as the median with interquartile range (IQR) or mean ± standard deviation (SD) and were compared by the Mann-Whitney test or independent group t-tests as appropriate. Categorical variables were presented as the frequency with proportion, and the Fisher’s exact test or Pearson Chi-square test was adopted to compare differences between groups. The patients with COVID-19 from three hospitals in Hubei province were assigned to the training set, and those cases from Shanghai were allocated into the external validation set. Quantitative variables were transformed into qualitative ones based on corresponding reference values. Missing variables at admission were given imputation by expectation-maximization algorithm method if missing rate was less than 10.0%. The correlations of potential predictors and the development of severity were analyzed by univariate logistic regression initially. Then, significant predictors were incorporated into a multivariate logistic model using the backward stepwise selection procedure to determine the independent risk factors for progression to severe COVID-19. Odds ratio (OR) and 95% confidence interval (CI) were exhibited for estimates from logistic models. A 10-fold cross-validation was conducted 400 times on the basis of the training set, and the area under the curve (AUC) of the receiver operating characteristic (ROC) curve was employed to evaluate the predictive competence in differentiating severe COVID-19 cases from non-severe cases. Based on the determinants identified in the multivariate model, a predictive nomogram was constructed to produce a combined indicator for assessing the individual probability of the development of severity of COVID-19 using the RMS package of the R project (The R Foundation for Statistical Computing, Austria). The nomogram performance was tested by discrimination and calibration. We used the concordance index (C-index) to evaluate discrimination, in which perfect predictive competence was expressed by an absolute value approximately equal to 1. Visual calibration curves were plotted based on 1,000 bootstrap resamplings to evaluate the concordance between observed and forecasted severity probability. To validate the generalizability, the nomogram performance was further validated in the external validation set that was not included in the model training, using the method described earlier. All statistical analyses were carried by R project v.4.1.2 and SAS v.9.2 (SAS Institute Inc., Arlington, VA, USA). All tests were performed 2-sided at the 5% significance level.
Ethical statement
The study was conducted in accordance with the Declaration of Helsinki (as revised in 2013). This study was approved by the Ethics Committee of Wuhan Third Hospital of Wuhan University (approval No. KY2020-007) and individual informed consent for this retrospective analysis was waived.
Results
Patient characteristics
A total of 610 cases were diagnosed with confirmed COVID-19 in these four hospitals till March 8, 2020, and 12 non-hospitalized cases were excluded. Ultimately, 598 eligible COVID-19 cases were included in our study, with 437 in the training set and 161 in the external validation set. Table 1 describes the demography and clinical characteristics in the training and validation sets. Among all 598 patients, the mean age was 54.68±15.59 years, and 347 (58.03%) were males. The top three symptoms at admission were fever (454 cases, 75.92%), dry cough (188 cases, 31.44%), and sputum production (172 cases, 28.76%). The most frequent comorbidity was hypertension (198 cases, 33.11%), followed by diabetes (77 cases, 12.88%). Eventually, 519 cases were cured and discharged after meeting discharge criteria, and 79 cases died from multi-organ failure or acute respiratory distress syndrome caused by COVID-19 in the hospital, with an average mortality of 13.21%. In the set of training and validation, 144 (32.95%) and 54 (33.54%) patients, respectively, progressed to severe COVID-19 during the hospitalization. As described in Table 1, the baseline characteristics between the two cohorts were well-balanced.
Table 1
Variables | Training cohort (n=437) | External validation cohort (n=161) | t/Z/χ2 | P |
---|---|---|---|---|
Demographics | ||||
Age, years | 55.16±15.44 | 53.40±15.97 | 1.219 | 0.223 |
Sex | 1.085 | 0.297 | ||
Male | 248 (56.8) | 99 (61.5) | ||
Female | 189 (43.2) | 62 (38.5) | ||
Smoking | 0.838 | 0.658 | ||
Never | 391 (89.5) | 148 (91.9) | ||
Current | 34 (7.8) | 10 (6.2) | ||
Former | 12 (2.7) | 3 (1.9) | ||
Symptoms and signs | ||||
Fever (≥37.3 ℃) | 337 (77.1) | 117 (72.7) | 1.272 | 0.259 |
Dry cough | 138 (31.6) | 50 (31.1) | 0.015 | 0.903 |
Sputum production | 131 (30.0) | 41 (25.5) | 1.169 | 0.280 |
Pharyngodynia | 26 (5.9) | 12 (7.5) | 0.447 | 0.504 |
Chest pain | 20 (4.6) | 8 (5.0) | 0.041 | 0.840 |
Shortness of breath | 113 (25.9) | 36 (22.4) | 0.769 | 0.380 |
Bilateral lung lobes involved in chest CT | 400 (91.5) | 145 (90.1) | 0.315 | 0.574 |
Days from illness onset to diagnosis confirmed | 4 [2–7] | 5 [2–8] | 1.333 | 0.183 |
Days from illness onset to admission | 7 [4–11] | 7 [4–11] | 0.674 | 0.501 |
Comorbid conditions | ||||
Any comorbidity | 220 (50.3) | 81 (50.3) | 0.000 | 0.994 |
Hypertension | 138 (31.6) | 60 (37.3) | 1.719 | 0.190 |
Cardiovascular disease | 25 (5.7) | 13 (8.1) | 1.095 | 0.295 |
Diabetes | 57 (13.0) | 20 (12.4) | 0.040 | 0.841 |
Carcinoma | 18 (4.1) | 3 (1.9) | 1.767 | 0.219 |
Cerebrovascular disease | 13 (3.0) | 6 (3.7) | 0.216 | 0.642 |
COPD | 7 (1.6) | 4 (2.5) | 0.508 | 0.498 |
Laboratory findings | ||||
C-reactive protein, mg/L | 31.30 [8.00–48.85] | 32.50 [6.03–46.01] | 0.587 | 0.558 |
White blood cell count, ×109/L | 5.60 [4.44–7.79] | 5.28 [4.19–7.07] | 1.744 | 0.081 |
Neutrophil count, ×109/L | 3.56 [2.75–5.32] | 3.56 [2.59–5.04] | 0.920 | 0.358 |
Lymphocyte count, ×109/L | 1.04 [0.69–1.48] | 1.02 [0.46–1.48] | 0.078 | 0.938 |
Haemoglobin, g/L | 132.0 [122.0–141.0] | 132.5 [124.0–147.0] | 1.968 | 0.049 |
Platelet count, ×109/L | 203.0 [151.0–238.0] | 196.5 [148.0–232.0] | 0.176 | 0.860 |
ALT >50 U/L | 100 (22.9) | 36 (22.4) | 0.018 | 0.892 |
AST >40 U/L | 127 (29.1) | 47 (29.2) | 0.001 | 0.975 |
Total bilirubin, μmol/L | 9.6 [7.2–12.5] | 9.45 [7.33–12.65] | 0.822 | 0.411 |
Direct bilirubin, μmol/L | 3.9 [3.1–5.5] | 3.9 [3.2–5.25] | 0.068 | 0.946 |
Albumin, g/L | 37.00 [32.60–40.85] | 37.00 [32.84–41.66] | 1.081 | 0.280 |
Blood urea nitrogen, mmol/L | 4.80 [3.82–5.80] | 4.70 [3.75–5.68] | 1.155 | 0.248 |
Creatinine, μmol/L | 68.30 [55.00–81.55] | 67.68 [55.52–80.14] | 0.375 | 0.707 |
Sodium, mmol/L | 139.0 [137.0–141.0] | 139.4 [138.0–141.3] | 1.491 | 0.136 |
Potassium, mmol/L | 3.94 [3.70–4.30] | 3.94 [3.70–4.20] | 0.196 | 0.845 |
Calcium, mmol/L | 2.07 [2.01–2.14] | 2.07 [1.99–2.14] | 0.353 | 0.724 |
LDH, U/L | 264.0 [207.0–371.0] | 261.0 [210.5–372.5] | 0.065 | 0.948 |
Creatine kinase, U/L | 82.0 [56.0–138.5] | 82.0 [56.0–128.0] | 0.094 | 0.925 |
CK-MB, U/L | 12.00 [11.00–15.17] | 13.00 [11.33–14.27] | 0.139 | 0.890 |
Myohemoglobin, ng/mL | 24.51 [9.23–50.15] | 24.51 [5.22–44.95] | 1.717 | 0.086 |
cTnI, ng/mL | 0.07 [0.02–3.75] | 0.07 [0.02–4.15] | 0.191 | 0.849 |
PCT, μg/L | 0.05 [0.02–0.10] | 0.05 [0.02–0.07] | 1.140 | 0.254 |
ESR, mm/h | 55.0 [34.0–78.0] | 49.0 [25.1–75.5] | 1.080 | 0.280 |
BNP, pg/mL | 46.00 [29.00–83.30] | 46.00 [26.50–67.00] | 0.567 | 0.571 |
Fibrinogen, g/L | 5.26 [4.03–5.49] | 5.08 [3.92–5.55] | 0.456 | 0.648 |
D-dimer, μg/L | 0.65 [0.36–1.51] | 0.57 [0.35–1.30] | 0.743 | 0.457 |
Data are presented as mean ± standard deviation, n (%), or median [interquartile range]. COVID-19, coronavirus disease 2019; CT, computerized tomography; COPD, chronic obstructive pulmonary disease; ALT, alanine aminotransferase; AST, aspartate aminotransferase; LDH, lactate dehydrogenase; CK-MB, creatine kinase isoenzymes; cTnI, cardiac troponin I; PCT, procalcitonin; ESR, erythrocyte sedimentation rate; BNP, brain natriuretic peptide.
Independent predictors for severity of COVID-19
The predictors correlated with the occurrence of severe conditions in the univariable analysis are shown in Table 2. Twenty-nine variables were significantly associated with progression to severe illness, in which included age, hypertension, diabetes, carcinoma, fever, shortness of breath, bilateral lung lobes involved in chest computerized tomography (CT), interval from onset to admission, interval from onset to diagnosis, CRP, white blood cell (WBC) count, platelet, lymphocyte, neutrophil, hemoglobin, aspartate aminotransferase (AST), alanine aminotransferase (ALT), albumin, blood urea nitrogen, creatinine, PCT, D-dimer, LDH, potassium, brain natriuretic peptide (BNP), cardiac troponin I (cTnI), myohemoglobin, creatine kinase, and erythrocyte sedimentation rate (ESR). Then, the significant predictors mentioned above were included in a multivariate model. Table S1 exhibits the ranges of normal values for laboratory examinations. As illustrated in Table 3, nine variables in the ultimate model were significant determinants of occurrence of severity. The determinants included were hypertension (OR: 3.320, 95% CI: 1.880–5.862), shortness of breath (OR: 2.220, 95% CI: 1.247–3.953), platelet count >350×109/L (OR: 4.235, 95% CI: 1.159–15.465), ALT >50 U/L (OR: 2.803, 95% CI: 1.521–5.164), potassium (3.5–5.3 mmol/L: OR: 4.096, 95% CI: 1.320–12.707; >5.3 mmol/L: OR: 6.831, 95% CI: 1.208–38.631), cTnI >0.0 4 ng/mL (OR: 6.740, 95% CI: 3.453–13.153), myohemoglobin >48.8 ng/mL (OR: 2.079, 95% CI: 1.149–3.760), PCT >0.05 µg/L (OR: 2.173, 95% CI: 1.253–3.769), and days from onset to diagnosis (OR: 1.106, 95% CI: 1.050–1.165).
Table 2
Variable | Level | Progression to severe COVID-19 | Unadjusted OR | P | |
---|---|---|---|---|---|
No (n=293) | Yes (n=144) | ||||
Age, years | <40 | 72 (24.6) | 10 (6.9) | Ref | |
40–69 | 184 (62.8) | 94 (65.3) | 3.678 | <0.0001 | |
≥70 | 37 (12.6) | 40 (27.8) | 7.784 | <0.0001 | |
Gender | male | 157 (53.6) | 91 (63.2) | Ref | |
female | 136 (46.4) | 53 (36.8) | 0.672 | 0.057 | |
Smoking status | Never | 260 (88.7) | 131 (91.0) | Ref | |
Current | 25 (8.5) | 9 (6.3) | 0.715 | 0.404 | |
Former | 8 (2.7) | 4 (2.8) | 0.992 | 0.990 | |
Hypertension | Absent | 228 (77.8) | 71 (49.3) | Ref | |
Present | 65 (22.2) | 73 (50.7) | 3.607 | <0.0001 | |
Cardiovascular disease | Absent | 276 (94.2) | 136 (94.4) | Ref | |
Present | 17 (5.8) | 8 (5.6) | 0.955 | 0.917 | |
Diabetes | Absent | 265 (90.4) | 115 (79.9) | Ref | |
Present | 28 (9.6) | 29 (20.1) | 2.387 | 0.002 | |
Carcinoma | Absent | 285 (97.3) | 134 (93.1) | Ref | |
Present | 8 (2.7) | 10 (6.9) | 2.659 | 0.037 | |
COPD | Absent | 288 (98.3) | 142 (98.6) | Ref | |
Present | 5 (1.7) | 2 (1.4) | 0.811 | 0.804 | |
Cerebrovascular disease | Absent | 283 (96.6) | 141 (97.9) | Ref | |
Present | 10 (3.4) | 3 (2.1) | 0.602 | 0.442 | |
Fever | Absent | 54 (18.4) | 46 (31.9) | Ref | |
Present | 239 (81.6) | 98 (68.1) | 0.481 | 0.002 | |
Dry cough | Absent | 205 (70.0) | 94 (65.3) | Ref | |
Present | 88 (30.0) | 50 (34.7) | 1.239 | 0.322 | |
Sputum production | Absent | 199 (67.9) | 107 (74.3) | Ref | |
Present | 94 (32.1) | 37 (25.7) | 0.732 | 0.171 | |
Pharyngodynia | Absent | 273 (93.2) | 138 (95.8) | Ref | |
Present | 20 (6.8) | 6 (4.2) | 0.593 | 0.269 | |
Chest pain | Absent | 279 (95.2) | 138 (95.8) | Ref | |
Present | 14 (4.8) | 6 (4.2) | 0.866 | 0.774 | |
Shortness of breath | Absent | 244 (83.3) | 80 (55.6) | Ref | |
Present | 49 (16.7) | 64 (44.4) | 3.984 | <0.0001 | |
Bilateral lung lobes involved in chest CT | No | 33 (11.3) | 4 (2.8) | Ref | |
Yes | 260 (88.7) | 140 (97.2) | 4.442 | 0.003 | |
Interval between illness onset and diagnosis | 4 [2–6] | 6 [2–10] | 1.098 | <0.0001 | |
Interval between illness onset and admission | 6 [3–10] | 10 [7–15] | 1.117 | <0.0001 | |
CRP, mg/L | <5 | 66 (22.5) | 19 (13.2) | Ref | |
5–100 | 202 (68.9) | 77 (53.5) | 1.324 | 0.338 | |
>100 | 25 (8.5) | 48 (33.3) | 6.669 | <0.0001 | |
WBC count, ×109/L | <4 | 53 (18.1) | 16 (11.1) | Ref | |
4–10 | 215 (73.4) | 92 (63.9) | 1.417 | 0.262 | |
>10 | 25 (8.5) | 36 (25.0) | 4.770 | <0.0001 | |
Neutrophil count, ×109/L | <1.8 | 18 (6.1) | 5 (3.5) | Ref | |
1.8–6.3 | 230 (78.5) | 88 (61.1) | 1.377 | 0.539 | |
>6.3 | 45 (15.4) | 51 (35.4) | 4.080 | 0.010 | |
Lymphocyte count, ×109/L | <0.5 | 152 (51.9) | 59 (41.0) | Ref | |
0.5–1.1 | 121 (41.3) | 68 (47.2) | 1.448 | 0.086 | |
≥1.1 | 20 (6.8) | 17 (11.8) | 2.190 | 0.031 | |
Haemoglobin, g/L | <130 | 122 (41.6) | 75 (52.1) | 1.524 | 0.040 |
≥130 | 171 (58.4) | 69 (47.9) | Ref | ||
Platelet count, ×109/L | <125 | 41 (14.0) | 17 (11.8) | Ref | |
125–350 | 242 (82.6) | 113 (78.5) | 1.126 | 0.702 | |
>350 | 10 (3.4) | 14 (9.7) | 3.376 | 0.016 | |
ALT, U/L | ≤50 | 248 (84.6) | 89 (61.8) | Ref | |
>50 | 45 (15.4) | 55 (38.2) | 3.406 | <0.0001 | |
AST, U/L | ≤40 | 232 (79.2) | 78 (54.2) | Ref | |
>40 | 61 (20.8) | 66 (45.8) | 3.218 | <0.0001 | |
Total bilirubin, mmol/L | ≤20.5 | 262 (89.4) | 136 (94.4) | Ref | |
>20.5 | 31 (10.6) | 8 (5.6) | 0.497 | 0.089 | |
Direct bilirubin, mmol/L | ≤8.6 | 265 (90.4) | 131 (91.0) | Ref | |
>8.6 | 28 (9.6) | 13 (9.0) | 0.939 | 0.859 | |
Albumin, g/L | <40 | 176 (60.1) | 128 (88.9) | 5.318 | <0.0001 |
≥40 | 117 (39.9) | 16 (11.1) | Ref | ||
Blood urea nitrogen, mmol/L | ≤9.5 | 278 (94.9) | 124 (86.1) | Ref | |
>9.5 | 15 (5.1) | 20 (13.9) | 2.989 | 0.002 | |
Creatinine, μmol/L | ≤111 | 280 (95.6) | 129 (89.6) | Ref | |
>111 | 13 (4.4) | 15 (10.4) | 2.504 | 0.016 | |
Sodium, mmol/L | ≤147 | 291 (99.3) | 140 (97.2) | Ref | |
>147 | 2 (0.7) | 4 (2.8) | 4.157 | 0.102 | |
Potassium, mmol/L | <3.5 | 44 (15.0) | 7 (4.9) | Ref | |
3.5–5.3 | 243 (82.9) | 130 (90.3) | 3.363 | 0.004 | |
>5.3 | 6 (2.0) | 7 (4.9) | 7.333 | 0.004 | |
Calcium, mmol/L | <2.55 | 281 (95.9) | 137 (95.1) | Ref | |
≥2.55 | 12 (4.1) | 7 (4.9) | 1.196 | 0.136 | |
LDH, U/L | <245 | 146 (49.8) | 40 (27.8) | Ref | |
≥245 | 147 (50.2) | 104 (72.2) | 2.582 | <0.0001 | |
Creatine kinase, U/L | ≤200 | 247 (84.3) | 104 (72.2) | Ref | |
>200 | 46 (15.7) | 40 (27.8) | 2.065 | 0.003 | |
CK-MB, U/L | ≤24 | 259 (88.4) | 133 (92.4) | Ref | |
>24 | 34 (11.6) | 11 (7.6) | 0.63 | 0.200 | |
Myohemoglobin, ng/mL | ≤48.8 | 226 (77.1) | 49 (34.0) | Ref | |
>48.8 | 67 (22.9) | 95 (66.0) | 6.540 | <0.0001 | |
cTnI, ng/mL | ≤0.04 | 184 (62.8) | 19 (13.2) | Ref | |
>0.04 | 109 (37.2) | 125 (86.8) | 11.106 | <0.0001 | |
PCT, μg/L | ≤0.05 | 198 (67.6) | 56 (38.9) | Ref | |
>0.05 | 95 (32.4) | 88 (61.1) | 3.275 | <0.0001 | |
ESR, mm/h | ≤15 | 41 (14.0) | 10 (6.9) | Ref | |
>15 | 252 (86.0) | 134 (93.1) | 2.180 | 0.031 | |
BNP, pg/mL | ≤250 | 271 (92.5) | 108 (75.0) | Ref | |
>250 | 22 (7.5) | 36 (25.0) | 4.106 | <0.0001 | |
Fibrinogen, g/L | ≤4 | 82 (28.0) | 33 (22.9) | Ref | |
>4 | 211 (72.0) | 111 (77.1) | 1.307 | 0.258 | |
D-dimer, μg/L | ≤0.5 | 143 (48.8) | 30 (20.8) | Ref | |
>0.5 | 150 (51.2) | 114 (79.2) | 3.623 | <0.0001 |
Data are presented as n (%) or median (interquartile range). COVID-19, coronavirus disease 2019; COPD, chronic obstructive pulmonary disease; CT, computerized tomography; CRP, C-reactive protein; WBC, white blood cell; ALT, alanine aminotransferase; AST, aspartate aminotransferase; LDH, lactate dehydrogenase; CK-MB, Creatine kinase isoenzymes; cTnI, cardiac troponin I; PCT, procalcitonin; ESR, erythrocyte sedimentation rate; BNP, brain natriuretic peptide.
Table 3
Variable | Level | β | Wald χ2 | OR (95% CI) | P |
---|---|---|---|---|---|
Hypertension | Absent | Ref | |||
Present | 1.200 | 17.105 | 3.320 (1.880–5.862) | <0.0001 | |
Shortness of breath | Absent | Ref | |||
Present | 0.798 | 7.348 | 2.220 (1.247–3.953) | 0.007 | |
Platelet count, ×109/L | <125 | Ref | |||
125–350 | 0.054 | 0.017 | 1.056 (0.467–2.386) | 0.896 | |
>350 | 1.443 | 4.769 | 4.235 (1.159–15.465) | 0.029 | |
ALT, U/L | ≤50 | Ref | |||
>50 | 1.031 | 10.919 | 2.803 (1.521–5.164) | 0.001 | |
Potassium, mmol/L | <3.5 | Ref | |||
3.5–5.3 | 1.410 | 5.957 | 4.096 (1.320–12.707) | 0.015 | |
>5.3 | 1.921 | 4.724 | 6.831 (1.208–38.631) | 0.030 | |
cTnI, ng/mL | ≤0.04 | Ref | |||
>0.04 | 1.908 | 31.230 | 6.740 (3.453–13.153) | <0.0001 | |
Myohemoglobin, ng/mL | ≤48.8 | Ref | |||
>48.8 | 0.732 | 5.859 | 2.079 (1.149–3.760) | 0.016 | |
PCT, μg/L | ≤0.05 | Ref | 1.056 | ||
>0.05 | 0.776 | 7.636 | 2.173 (1.253–3.769) | 0.006 | |
Days between illness onset and diagnosis | 0.101 | 14.377 | 1.106 (1.050–1.165) | 0.0001 |
COVID-19, coronavirus disease 2019; ALT, alanine aminotransferase; cTnI, cardiac troponin I; PCT, procalcitonin.
Construction of predictive nomogram in training cohort
A nomogram incorporating the nine independent determinants was constructed following the outcomes of multivariate model (Figure 1). Firstly, the score of each determinant was obtained according to the points scale at the top of the figure. Then, individual scores were summarized to compute the total score. Finally, a patient’s probability of developing into severe illness was determined by matching the total score vertically downward to the point scale beneath the figure. The AUC of the ROC was 0.887 (95% CI: 0.854–0.919) within the training set, and the ROC of this model exhibited a sensitivity and specificity of 80.6% and 86.0% (Figure 2A). After bias correction with 10-fold cross-validation, the mean AUC was reduced to 0.774, which was still robust. This nomogram showed outstanding discrimination in predicting the possibility of developing into severe conditions with a C-index being 0.887 (95% CI: 0.854–0.919). Meanwhile, the calibration curve showed excellent agreement between the actual observations and the predictions by the nomogram, as referred to the probability of severity (Figure 2B).
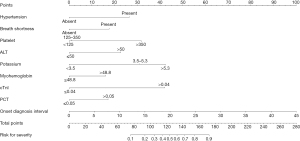
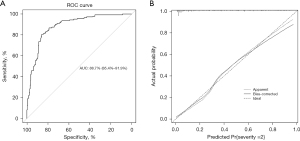
Validation of nomogram performance in external validation cohort
An external validation was conducted and our nomogram showed good discrimination for severity prediction with a C-index being 0.850 (95% CI: 0.815–0.885) within the external validation cohort. The sensitivity and specificity of the ROC in the validation set were 86.7% and 73.8%, respectively (Figure 3A). Furthermore, calibration plot of validation set suggested optimal concordance between the observed deterioration and the risk predicted by the nomogram (Figure 3B).
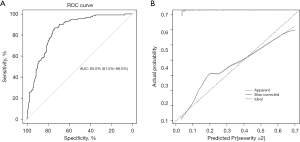
Discussion
Predictably, the COVID-19 pandemic remains a major global threat, and it is vital to identify the determinants early to forecast the risk of developing into severity (16). In the present research, we built a nomogram using easily available indicators at admission to distinguish the cases tending to progress to severe conditions based on multi-center cohorts. Our nomogram incorporated nine indicators, including hypertension, shortness of breath, days between illness onset and diagnosis, platelet count, ALT, potassium, cTnI, myohemoglobin, and PCT. COVID-19 deteriorates rapidly compared with other respiratory infectious diseases; thus, early identification of severity is crucial. Early prediction of severity is beneficial for determining cases at high risk and properly distributing crucial healthcare resources. Some models of deep-learning algorithms were reported to have reliable accuracy in predicting the severity of COVID-19 (17-19). However, these models are hard to construct owing to needing complicated algorithms, making them not easily available for all physicians and hospitals. By contrast, this nomogram using clinical data easily obtained at admission is a user-friendly and convenient predicting system for estimating the COVID-19 severity. To address the bias in variable selection in our study, the resampling technique of 10-fold cross-validation on the fitting cohort was adopted and then validated on a withheld validation set. Our nomogram has been proven to be a powerful and robust model by internal validation with a C-index being 0.887 and external validation with a C-index being 0.850. It maintained adequate discrimination capacity by internal 10-fold cross-validation with a mean AUC of 0.774. Besides, this nomogram also exhibited good performance in both internal and external calibration by achieving perfect consistency between real observations and predicted values. Therefore, it can serve as a quantitative tool for assessing the individual probability of developing into severe disease for clinicians and allow them to determine proper treatment at an early stage.
Early studies have demonstrated age is a predictor for progression of severe COVID-19 (20-22). Although the percentage of cases over the age of 70 in the severe group was greater than that in the non-severe group in our study, age did not emerge as an independent predictive indicator of severe COVID-19 in our study. This may be because all individuals were inpatients, and a majority of patients who needed to be hospitalized were older. There is a growing awareness that extra-pulmonary involvement of COVID-19 plays a crucial role in progression of conditions. Freund et al. found that the higher the proportion of concurrent involvement of COVID-19 patients, the higher the likelihood of severe conditions (23). As reported by previous studies, more prevalent comorbidities existed in cases with severe conditions than in mild cases, and comorbidity of hypertension was demonstrated as a significant predictor of critical COVID-19 (24,25). In agreement with previous studies, our study confirmed that hypertension was an independent predictor for progression of severe illness. At the early stage of COVID-19, it manifested diverse symptoms, which were unbeneficial to the diagnosis. Our study showed that fever was not a risk factor in predicting severity, and the prevalence of patients with fever at early stage of illness in the severe group was lower than that in the controls. The reason lies in the fact that fever may prompt patients to seek medical interventions early. Shortness of breath was thought to be more likely to lead poor clinical outcomes in COVID-19 cases (26). The percentage of shortness of breath was greater in severe cases in our study, and shortness of breath was proven to be a determinant of severe illness, as described before (27,28). Our study also showed that days from onset to diagnosis was a determinant of occurrence of severe COVID-19. Considering the huge case volume during the pandemic period and the limited health resources, public health strategies should be taken into account to optimize the accessibility to emergency healthcare service to reduce diagnostic delay.
Our nomogram included several easily accessible laboratory markers. This study showed that platelet count >350×109/L was an independent predictor for progression to severe disease. Major pathologic events in severe COVID-19 include hypercoagulable state, and thromboembolic complications have been listed among life-threatening complications of the disease, accelerating the negative outcome in COVID-19 patients (29). Al-Samkari et al. found that COVID-19 patients with platelet count >450×109/L were more likely to develop severe thrombosis (30). Low platelet count implies being under severe conditions according to SOFA score. However, the proportion of low platelet count in the non-severe group was higher than that in the severe group in our study, probably explaining why low platelets did not enhance the progression to the severity of COVID-19 compared to those with normal platelet count. As for laboratory indicators in our nomogram, the inclusion of ALT, cTnI, and myohemoglobin suggested that the manifestation of multi-organ dysfunction represented the crucial determinant of identifying occurrence of severity. Prior studies have revealed that high ALT in COVID-19 cases was exceedingly correlated with developing into severity (31). ALT in our study also served as a well-recognized determinant for illness progression. Acute myocardial injury resulting from viral myocarditis is a commonly reported cardiac complication among COVID-19 and may indicate potentially life-threatening prognosis. Studies have demonstrated that higher cTnI and myohemoglobin were allied to the increased risk of disease severity (32,33). Our results indicated that cTnI together with myohemoglobin were recognized as reliable and effective predictors of severe COVID-19. Electrolyte disturbances, especially potassium disorder, are usual in COVID-19 cases. Hyperkalemia may increase the chances of progression to severe illness and even death (34). To the best of our knowledge, none of previous COVID-19 researches about predicting models exclusively assessed the association between serum potassium and illness severity. In this research, we discovered that elevated potassium was an independently risk factor of severity, and a dose-response relationship was presented. Considering the potential interference effect of impaired renal function on hyperkalemia, Kendall correlation analysis was conducted, and results showed that both blood urea nitrogen and creatinine had no correlation with potassium. As an inflammatory biomarker, serum PCT levels are usually undetectable or low. Severe COVID-19 patients usually manifested a more significant increase in PCT compared with non-severe patients (35). Any substantial increase of PCT in COVID-19 cases reflects that patients may have concomitant bacterial infections, and development of a severe illness. Our study demonstrated that higher PCT at admission obviously enhanced the risk of developing severe COVID-19.
Some limitations of our research should be acknowledged. Firstly, the retrospective design of this multi-center study restricted the strength of evidence. This study did not include all possible indicators of severity, such as oxygen saturation and comorbidities like acute kidney injury (22). Additional prospective studies are required to verify the reliability of the results. Secondly, our nomogram was tailored for COVID-19 cases infected with the original SARS-CoV-2 strains and may not be applicable to other variant strains, especially the Omicron strains. Future nomogram studies using recent SARS-CoV-2 variants and more comprehensive parameters are needed. The sample size of this multi-center study was not extremely large, particularly for the external validation cohort, which might affect the extrapolation and generalization of conclusions. Meanwhile, this study primarily included objective predictors associated with the development of severe COVID-19; thus, our results may stand against scrutiny. Thirdly, our study constructed and validated the predictive model taking merely admitted COVID-19 cases as objects. Therefore, this nomogram is not applicable to non-hospitalized patients. Moreover, our analysis was restricted to predict the progression to severe COVID-19 using information obtained at admission, without considering the dynamic changes in the indicators.
Conclusions
As a whole, our study has established an early predicting nomogram model that incorporates hypertension, shortness of breath, platelet count, ALT, potassium, cTnI, myohemoglobin, PCT, and days between illness onset and diagnosis. These factors are all easily available at admission. It’s soberly to note that although several studies on nomogram to predict the severity of COVID-19 were conducted in China during the same periods, the results were not quite the same. Our findings show some differences compared to other nomograms, likely due to variations in sample size and choice of predictors. Therefore, our model should be interpreted with caution. Even so, our model has demonstrated excellent performance in predicting the likelihood of progression to severity, which can assist doctors in determining highly risky cases at an early stage and making optimal medical decisions.
Acknowledgments
We thank Yun Ling, the chief of Shanghai Public Health Clinical Center, Tao Bai, the chief of Wuhan Jinyintan Hospital, and Kui Liu, the chief of Wuhan Tongji Hospital for their kind support of data. We thank all healthcare workers involved in the diagnosis and treatment of patients in 4 sites.
Funding: None.
Footnote
Reporting Checklist: The authors have completed the STROBE reporting checklist. Available at https://jtd.amegroups.com/article/view/10.21037/jtd-23-653/rc
Data Sharing Statement: Available at https://jtd.amegroups.com/article/view/10.21037/jtd-23-653/dss
Peer Review File: Available at https://jtd.amegroups.com/article/view/10.21037/jtd-23-653/prf
Conflicts of Interest: All authors have completed the ICMJE uniform disclosure form (available at https://jtd.amegroups.com/article/view/10.21037/jtd-23-653/coif). The authors have no conflicts of interest to declare.
Ethical Statement: The authors are accountable for all aspects of the work in ensuring that questions related to the accuracy or integrity of any part of the work are appropriately investigated and resolved. The study was conducted in accordance with the Declaration of Helsinki (as revised in 2013). This study was approved by the Ethics Committee of Wuhan Third Hospital of Wuhan University (approval No. KY2020-007) and individual informed consent for this retrospective analysis was waived.
Open Access Statement: This is an Open Access article distributed in accordance with the Creative Commons Attribution-NonCommercial-NoDerivs 4.0 International License (CC BY-NC-ND 4.0), which permits the non-commercial replication and distribution of the article with the strict proviso that no changes or edits are made and the original work is properly cited (including links to both the formal publication through the relevant DOI and the license). See: https://creativecommons.org/licenses/by-nc-nd/4.0/.
References
- Huang C, Wang Y, Li X, et al. Clinical features of patients infected with 2019 novel coronavirus in Wuhan, China. Lancet 2020;395:497-506. [Crossref] [PubMed]
- Chen N, Zhou M, Dong X, et al. Epidemiological and clinical characteristics of 99 cases of 2019 novel coronavirus pneumonia in Wuhan, China: a descriptive study. Lancet 2020;395:507-13. [Crossref] [PubMed]
- Grasselli G, Zangrillo A, Zanella A, et al. Baseline Characteristics and Outcomes of 1591 Patients Infected With SARS-CoV-2 Admitted to ICUs of the Lombardy Region, Italy. JAMA 2020;323:1574-81. [Crossref] [PubMed]
- Baj J, Karakuła-Juchnowicz H, Teresiński G, et al. COVID-19: Specific and Non-Specific Clinical Manifestations and Symptoms: The Current State of Knowledge. J Clin Med 2020;9:1753. [Crossref] [PubMed]
- Liang W, Liang H, Ou L, et al. Development and Validation of a Clinical Risk Score to Predict the Occurrence of Critical Illness in Hospitalized Patients With COVID-19. JAMA Intern Med 2020;180:1081-9. [Crossref] [PubMed]
- Weekly epidemiological update on COVID-19. 1 February 2023. Available online: https://www.who.int/publications/m/item/weekly-epidemiological-update-on-covid-19---1-february-2023
- Wu Z, McGoogan JM. Characteristics of and Important Lessons From the Coronavirus Disease 2019 (COVID-19) Outbreak in China: Summary of a Report of 72 314 Cases From the Chinese Center for Disease Control and Prevention. JAMA 2020;323:1239-42. [Crossref] [PubMed]
- Wu C, Chen X, Cai Y, et al. Risk Factors Associated With Acute Respiratory Distress Syndrome and Death in Patients With Coronavirus Disease 2019 Pneumonia in Wuhan, China. JAMA Intern Med 2020;180:934-43. [Crossref] [PubMed]
- Livingston E, Bucher K. Coronavirus Disease 2019 (COVID-19) in Italy. JAMA 2020;323:1335. [Crossref] [PubMed]
- Guan WJ, Liang WH, Zhao Y, et al. Comorbidity and its impact on 1590 patients with COVID-19 in China: a nationwide analysis. Eur Respir J 2020;55:2000547. [Crossref] [PubMed]
- Huang R, Zhu L, Xue L, et al. Clinical findings of patients with coronavirus disease 2019 in Jiangsu province, China: A retrospective, multi-center study. PLoS Negl Trop Dis 2020;14:e0008280. [Crossref] [PubMed]
- Wang D, Hu B, Hu C, et al. Clinical Characteristics of 138 Hospitalized Patients With 2019 Novel Coronavirus-Infected Pneumonia in Wuhan, China. JAMA 2020;323:1061-9. [Crossref] [PubMed]
- Balachandran VP, Gonen M, Smith JJ, et al. Nomograms in oncology: more than meets the eye. Lancet Oncol 2015;16:e173-80. [Crossref] [PubMed]
- Feng Y, Ling Y, Bai T, et al. COVID-19 with Different Severities: A Multicenter Study of Clinical Features. Am J Respir Crit Care Med 2020;201:1380-8. [Crossref] [PubMed]
- Guideline on the Diagnosis and Treatment for COVID-19 (trial V6). 19 February 2022. Available online: http://www.nhc.gov.cn/yzygj/s7653p/202002/8334a8326dd94d329df351d7da8aefc2.shtml
- Zeng Z, Wu C, Lin Z, et al. Development and validation of a simple-to-use nomogram to predict the deterioration and survival of patients with COVID-19. BMC Infect Dis 2021;21:356. [Crossref] [PubMed]
- Xiao LS, Li P, Sun F, et al. Development and Validation of a Deep Learning-Based Model Using Computed Tomography Imaging for Predicting Disease Severity of Coronavirus Disease 2019. Front Bioeng Biotechnol 2020;8:898. [Crossref] [PubMed]
- Shi W, Peng X, Liu T, et al. A deep learning-based quantitative computed tomography model for predicting the severity of COVID-19: a retrospective study of 196 patients. Ann Transl Med 2021;9:216. [Crossref] [PubMed]
- Chen Y, Ouyang L, Bao FS, et al. A Multimodality Machine Learning Approach to Differentiate Severe and Nonsevere COVID-19: Model Development and Validation. J Med Internet Res 2021;23:e23948. [Crossref] [PubMed]
- Zhang H, Zhong F, Wang B, et al. A nomogram predicting the severity of COVID-19 based on initial clinical and radiologic characteristics. Future Virol 2022;
- Martin S, Fuentes S, Sanchez C, et al. Development and validation of a laboratory-based risk score to predict the occurrence of critical illness in hospitalized patients with COVID-19. Scand J Clin Lab Invest 2021;81:282-9. [Crossref] [PubMed]
- Freund O, Weiss TE, Tau L, et al. Safety and outcomes of an early discharge strategy with oxygen home therapy in stable severe COVID-19 patients. Infect Dis (Lond) 2023;55:292-8. [Crossref] [PubMed]
- Freund O, Azolai L, Sror N, et al. Diagnostic delays among COVID-19 patients with a second concurrent diagnosis. J Hosp Med 2023;18:321-8. [Crossref] [PubMed]
- Zhou Y, He Y, Yang H, et al. Development and validation a nomogram for predicting the risk of severe COVID-19: A multi-center study in Sichuan, China. PLoS One 2020;15:e0233328. [Crossref] [PubMed]
- Zhou F, Yu T, Du R, et al. Clinical course and risk factors for mortality of adult inpatients with COVID-19 in Wuhan, China: a retrospective cohort study. Lancet 2020;395:1054-62. [Crossref] [PubMed]
- Li L, Fang X, Cheng L, et al. Development and validation of a prognostic nomogram for predicting in-hospital mortality of COVID-19: a multicenter retrospective cohort study of 4086 cases in China. Aging (Albany NY) 2021;13:3176-89. [Crossref] [PubMed]
- Shi L, Wang Y, Wang Y, et al. Dyspnea rather than fever is a risk factor for predicting mortality in patients with COVID-19. J Infect 2020;81:647-79. [Crossref] [PubMed]
- Allali G, Marti C, Grosgurin O, et al. Dyspnea: The vanished warning symptom of COVID-19 pneumonia. J Med Virol 2020;92:2272-3. [Crossref] [PubMed]
- Hottz ED, Azevedo-Quintanilha IG, Palhinha L, et al. Platelet activation and platelet-monocyte aggregate formation trigger tissue factor expression in patients with severe COVID-19. Blood 2020;136:1330-41. [Crossref] [PubMed]
- Al-Samkari H, Karp Leaf RS, Dzik WH, et al. COVID-19 and coagulation: bleeding and thrombotic manifestations of SARS-CoV-2 infection. Blood 2020;136:489-500. [Crossref] [PubMed]
- Parohan M, Yaghoubi S, Seraji A. Liver injury is associated with severe coronavirus disease 2019 (COVID-19) infection: A systematic review and meta-analysis of retrospective studies. Hepatol Res 2020;50:924-35. [Crossref] [PubMed]
- Inciardi RM, Adamo M, Lupi L, et al. Characteristics and outcomes of patients hospitalized for COVID-19 and cardiac disease in Northern Italy. Eur Heart J 2020;41:1821-9. [Crossref] [PubMed]
- Gaze DC. Clinical utility of cardiac troponin measurement in COVID-19 infection. Ann Clin Biochem 2020;57:202-5. [Crossref] [PubMed]
- Noori M, Nejadghaderi SA, Sullman MJM, et al. Epidemiology, prognosis and management of potassium disorders in Covid-19. Rev Med Virol 2022;32:e2262. [Crossref] [PubMed]
- Liu F, Li L, Xu M, et al. Prognostic value of interleukin-6, C-reactive protein, and procalcitonin in patients with COVID-19. J Clin Virol 2020;127:104370. [Crossref] [PubMed]