Development and validation of a DNA damage repair-related gene-based prediction model for the prognosis of lung adenocarcinoma
Highlight box
Key findings
• A nine-gene risk signature associated with DNA damage repair (DDR) in lung adenocarcinoma (LUAD) was effectively developed, demonstrating its potential as a robust and reliable classification tool for clinical practice.
What is known and what is new?
• Lung cancer is the leading cause of morbidity and mortality among all cancer types, with LUAD being the most prevalent subtype. DDR-related genes are closely associated with cancer progression and treatment, with emerging evidence highlighting their correlation with tumor development. However, the relationship between LUAD prognosis and DDR-related genes remains unclear.
• We successfully developed a nine-gene risk signature associated with DDR in LUAD, demonstrating its potential as an effective and stable classification tool for clinical practice.
What is the implication, and what should change now?
• This study has significant implications for LUAD patient care and highlights the importance of considering DDR subtypes in personalized treatment approaches.
Introduction
Lung cancer, particularly non-small cell lung carcinoma (NSCLC), is the leading cause of morbidity and mortality among all cancer types (1,2). NSCLC is commonly classified into histological subtypes, with lung adenocarcinoma (LUAD) being the most prevalent subtype (3). The development of LUAD is influenced by various factors, including smoking, alcohol consumption, and metabolic disorders. Despite significant advancements in multimodal treatment approaches, such as immunotherapy, radiotherapy, and noninvasive surgical resection, the outcomes for patients with lung cancer remain unsatisfactory, with a 5-year relative overall survival (OS) rate of approximately 18% (3,4). This can be attributed to the limitations of the traditional histological classification of LUAD, given its high heterogeneity and complexity (5,6). Additionally, the existing staging system fails to accurately predict the prognosis of lung cancer, which can result in some patients with early-stage disease failing to receive appropriate adjuvant therapy after surgery, leading to cancer recurrence or metastasis (7,8). Therefore, there is a crucial need to identify more effective prognostic indicators for patients with LUAD.
DNA damage repair (DDR) genes play a pivotal role in maintaining the stability of the human genome, while the loss of DDR function can contribute to the initiation and progression of cancer (9,10). As a result, there is a growing appreciation for treatment strategies that target aberrant DDR function. One such example is poly (ADP-ribose) polymerase (PARP), a nuclear enzyme involved in recognizing DNA damage, which has emerged as a therapeutic target for cancer treatment (11). DDR genes can be categorized into specific functional pathways based on their roles in DNA damage response (12). This categorization has provided valuable insights into underlying mechanisms and therapeutic analyses. DDR-related genes are closely associated with cancer progression and treatment, with emerging evidence highlighting their correlation with tumor development (13,14). However, the prognostic significance of these genes in LUAD has not been thoroughly investigated. In this study, we conducted a comprehensive evaluation and developed a novel signature and nomogram based on DDR genes to predict the outcomes of LUAD. Despite this progress, there remains a limited understanding of the dysregulation and heterogeneity of DDR genes in LUAD, particularly in terms of transcriptomic and proteomic analysis. Recent studies by Gu et al. demonstrated the prognostic value of a 15-feature gene signature in improving outcomes for patients with LUAD (15). Wu et al. also investigated the survival benefits associated with high tumor mutational burden (TMB) or DDR gene mutations in patients with LUAD with high stromal or immune scores (16). These findings underscore the potential of DDR genes as not only oncogenes but also promising biomarkers for prognosis prediction and treatment in patients with LUAD.
In this study, we analyzed a dataset of gene expression in LUAD obtained from The Cancer Genome Atlas (TCGA) and identified nine DDR genes through screening. The identified DDR gene signature proved to be a reliable predictor of survival prognosis in patients with LUAD. Moreover, our DDR subtype signature demonstrated its potential as a robust and clinically applicable classification tool in LUAD. These DDR genes hold additional promise as biomarkers for guiding immunotherapies in patients with LUAD. We present this article in accordance with the TRIPOD reporting checklist (available at https://jtd.amegroups.com/article/view/10.21037/jtd-23-1746/rc).
Methods
Data collection
RNA sequencing (RNA-seq) data along with clinical information including age, clinical stage, mutations, copy number variations, days to death, vital status, and more, were obtained from TCGA database (https://portal.gdc.cancer.gov/). Samples lacking clinical information were excluded from the analysis. Additionally, the GSE13213 dataset retrieved containing 117 LUAD samples from the Gene Expression Omnibus (GEO) database (https://www.ncbi.nlm.nih.gov/geo/) was utilized for external validation. To analyze the expression levels, count per million (CPM) read values were calculated using the edgeR software package (17). Ethics approval was deemed unnecessary for this phase of the study given that TCGA and GEO databases are publicly accessible resources. The study was conducted in accordance with the Declaration of Helsinki (revised in 2013).
Identification of differentially expressed DDR genes
Initially, the list of DDR-associated genes was retrieved from the Molecular Signatures Database (https://www.gsea-msigdb.org/gsea/msigdb). Ensemble IDs were transformed into gene symbols, selecting median values in cases where a gene had multiple symbols. To assess the expression levels of DDR genes in each sample, we conducted cluster analysis using the ConsensusClusterPlus package. This analysis facilitated the grouping of samples based on the expression patterns exhibited by DDR genes (18).
Establishment and validation of a prognostic model
The cancer samples of messenger RNA (mRNA)-seq in LUAD were randomly divided into two equal groups: a training group and a test group. A regression model was built according to the training group, and the test group and the total samples were used to verify the model results. The glmnet R package (The R Foundation for Statistical Computing, Vienna, Austria) was used to perform least absolute shrinkage and selection operator (LASSO) Cox regression for the purpose of identifying prognostic genes. To prevent overfitting, 10-fold cross-validation was employed to determine the penalized regularization parameter λ in the model. Based on the constructed model, the risk score for each LUAD sample was calculated. Additionally, the survival R package was used to conduct univariate Cox regression analysis of OS (19). Using the median of the risk score, we divided the patients with LUAD into two groups: a high-risk and low-risk group. Subsequently, receiver operating characteristic (ROC) curve analysis was conducted separately in the training dataset, testing dataset, and the entire dataset to assess the accuracy of the DDR signature. The differences between the high-risk and low-risk groups were evaluated through Kaplan-Meier (KM) curve analysis and the log-rank test. A P value of less than 0.05 was considered statistically different.
Functional and pathway enrichment analysis
Gene set enrichment analysis (GSEA) is a computational method used to identify sets of genes that are statistically enriched for a specific observable variable. In this study, we conducted GSEA using gene expression data obtained from TCGA and the Gene Oncology (GO) or Kyoto Encyclopedia of Genes and Genomes (KEGG) databases (20,21). The objective was to determine if particular gene sets exhibited enrichment based on their expression levels. Additionally, GSEA was performed using the gsva R package (22). We analyzed the differentially regulated genes between the high-risk and low-risk groups. A P value of less than 0.05 was considered statistically significant.
Relationship between the DDR signature and immune infiltration
The wilcox.test function in R was used to compare the differential expression of immune checkpoint genes between different groups of patients with LUAD. To visualize the mutational profiles of the low-risk and high-risk groups, the maftools package in R was employed (23). Cancer-testicular antigen (CTA) levels were obtained from the CTdatabase (http://www.cta.lncc.br/) (24), and the number of CTAs in each patient was calculated. Differential analysis of CTA numbers was performed using the wilcox.test function in R. Additionally, the response of patients with LUAD to immune checkpoint blockade (ICB) was predicted on pretreatment genomics using the tumor immune dysfunction and exclusion (TIDE) program (http://tide.dfci.harvard.edu/). A P value of less than 0.05 was considered statistically significant.
Statistical analysis
The RNA-seq data, along with clinical information pertaining to LUAD samples, were acquired from the Genomic Data Commons Portal or the GEO database. Statistical analyses were performed using GraphPad Prism 9.0 and R packages. Differences between the high-risk and low-risk groups were evaluated through KM curve analysis and the log-rank test. ROC curve analysis was separately conducted on the training, testing, and entire datasets to assess the accuracy of the DDR signature. All statistical procedures were carried out using R software (v4.0; The R Foundation for Statistical Computing). The Student’s t-test and paired t-test were applied for independent and paired groups, respectively. Continuous variables were presented as mean ± standard deviation. Statistical significance was established for a P value below 0.05.
Results
DDR gene alteration profiles in LUAD
To investigate the heterogeneity of DDR gene expression in LUAD, a total of 514 patients with LUAD were included in the analysis. Based on the expression profiles of 429 DDR genes, these patients were divided into distinct subtypes to characterize the diversity within the DDR pathway. Through consensus clustering and consideration of clinical features, two DDR subgroups were identified (Figure 1). Cluster 1 consisted of 227 patients, accounting for 44.2% of all LUAD cases and was designated as the DDR-suppressed subgroup, exhibiting comparative downregulation of DDR genes. In contrast, cluster 2 comprised 287 patients, representing 55.8% of all LUAD cases and was designated as the DDR-activated subgroup, showing significant upregulation of most DDR-related genes.
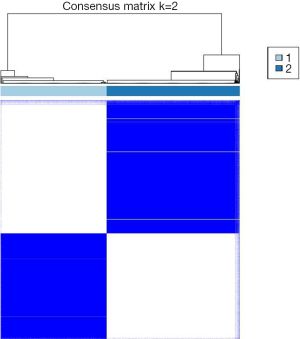
DDR gene-based subtypes exhibited distinct clinical characteristics
The two subgroups exhibited contrasting clinical outcomes. KM plots revealed that patients classified into the DDR-activated subtypes had poorer OS [hazard ratio (HR) =1.516, P=5.9e−03; Figure 2A] and disease-free survival (DFS) (HR =1.312, P=6.92e−02; Figure 2B). Furthermore, we investigated clinical parameters between the two subgroups and found that the DDR-activated subgroup was associated with more diverse factors. Specifically, we observed a higher incidence of advanced M stage (0.01≤P<0.05, Figure 2C), higher grade N stage (0.01≤P<0.05, Figure 2D), advanced pathologic stage (P<0.01, Figure 2E), and progressive T stage (0.01≤P<0.05, Figure 2F) in the DDR-activated subgroup. Additionally, we observed a higher frequency of females and older individuals in the DDR-suppressed subgroups (P<0.01, Figure 2G), while patients in the DDR-activated subgroup were significantly younger compared to those in cluster 1 (P<0.01, Figure 2H). These results indicated that the expression level of the DDR gene has a significant impact on the clinical parameters and prognosis of patients with LUAD.

DDR genes-based subtypes show distinct molecular characteristics
We conducted gene set variation analysis (GSVA) analysis to explore DDR-related pathways. The results revealed that the DDR-suppressed subgroups exhibited a higher frequency of involvement in the base excision repair pathway (P<0.01), Fanconi anemia pathway (P<0.01), homologous recombination pathway (P<0.01), and mismatch repair pathway (P<0.05). However, no significant difference was observed between the two groups in the non-homologous end-joining and nucleotide excision repair pathways (Figure 3).
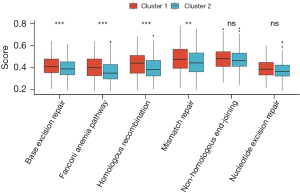
When examining genomic alterations, we conducted a comparison of gene mutation differences between the two DDR subtypes using maftools. The analysis revealed a tumor median mutation burden of 3.39 mutations per megabase (MB) (Figure 4A), with a significantly higher TMB observed in the DDR-activated subgroups (P<0.01, Figure 4B). This finding suggests a potentially enhanced response to immunotherapy in the DDR-activated subgroups. Among the top 10 genes with the highest frequencies of driver mutational genes in patients from the training cohort were TP53, TTN, CSMD3, RP1L1, XIRP2, STAB2, MMRN1, DCHS2, MTCL1, and LRP2 (Figure 4C). Notably, given the significance of TP53, we further investigated and found a higher frequency of TP53 mutations in the DDR-activated subgroup, which correlated with a poor prognosis (189/283 vs. 52/225, P<0.01) (Figure 4C). Missense mutations were the most prevalent type among these mutations (Figure 4D).
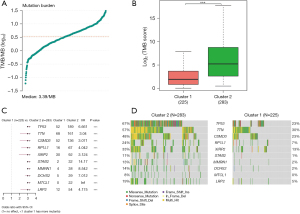
DDR subtypes were characterized by different immune profiles
Immune cell infiltration has a significant impact on tumor progression and the response to immunotherapy. Therefore, we investigated the differences in immune cell infiltration between the DDR-activated and DDR-suppressed subgroups. Our analysis revealed that naive B cells (P<0.01), resting dendritic cells (P<0.01), resting mast cells (P<0.01), monocytes (P<0.01), plasma cells (P<0.05), and resting memory CD4 T cells (0.01≤P<0.05) were significantly upregulated in the DDR-suppressed subgroup (Figure 5A). On the other hand, M0 macrophages, M1 macrophages, activated memory CD4 T cells, CD8 T cells, follicular helper T cells, resting natural killer (NK) cells (all P values <0.01), and neutrophils (0.01≤P<0.05) were significantly upregulated in the DDR-activated subgroup (Figure 5A). Furthermore, we observed a significant upregulation of programmed death-ligand 1 (PD-L1; CD274) in the DDR-activated subgroup [log2fold change (log2FC) =−1.161, P=4.36e−22<0.01] (Figure 5B). This result is consistent with the prediction of TMB for immunotherapy mentioned above.
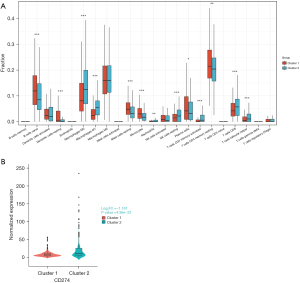
Construction and validation of the prognostic DDR-related gene pair signature
Differentially expressed DDR-related genes were identified based on the mRNA-seq count data and sample clustering information in LUAD. We observed 604 upregulated genes in the DDR-activated subgroup and 933 downregulated genes in the DDR-suppressed subgroup (Figure 6A,6B). Furthermore, a comparison of differentially expressed DDR-related genes between the two subgroups was performed, with the results being presented in Figure 6C.
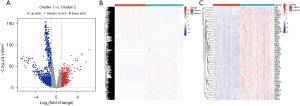
After the differentially expressed genes were screened, univariate Cox regression analysis was performed for each gene according to its expression level in LUAD the mRNA-seq expression data (CPM) and clinical information of the samples. Finally, a total of 223 differentially expressed DDR-related genes were found to be significantly associated with the OS of patients with LUAD in the training set (P<0.001; table available at https://cdn.amegroups.cn/static/public/jtd-23-1746-1.xlsx). To mitigate overfitting, LASSO regression was applied, and lambda.min (λ.min) was selected as the optimal regularization parameter, ensuring a more accurate model. Figure 7A,7B show the results of LASSO regression and cross-validation. The genes whose regression coefficient was not equal to 0 in LASSO regression analysis were then selected as marker genes. We observed that λ.min =0.095. From this analysis, nine genes were identified as hub DDR-related genes: CASP14, DKK1, ECT2, FLNC, HMMR, IGFBP1, KRT6A, TYMS, and FCER2 (Figure 7C). These hub genes were considered independent prognostic indicators of tumor prognosis. By using the expression levels of these selected genes, the corresponding risk scores for each sample was predicted (Figure 7D). Higher risk scores were associated with worse survival outcomes for the patients (Figure 7E,7F).
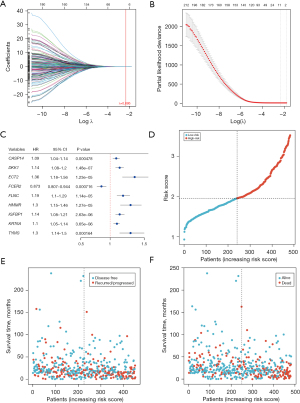
To validate the robustness of the constructed model, the patients were divided into high-risk and low-risk groups based on the median cutoff (Figure 8). In the training group, KM survival analysis revealed that the high-risk group exhibited significantly poorer OS compared to the low-risk group (HR =3.341, P=1.38e−08, Figure 8A). The area under the curve (AUC) values for the 1-, 3-, and 5-year follow-up periods were 0.767, 0.699, and 0.665, respectively, indicating the predictive ability of the model (Figure 8B). To further assess the prognostic performance of the model, individual risk scores were calculated using the aforementioned method, and patients in TCGA testing set and the entire TCGA set were classified accordingly. The predictions of the signature in these datasets were consistent with the previous findings. Specifically, the high-risk patients in all cohorts and the test group exhibited a significantly shorter OS compared to those in the low-risk group (Figure 8C,8E). The AUCs of the ROC curves for the 1-, 3-, and 5-year OS are presented in Figure 8D,8F and further supported the predictive ability of the model. The clustering heat map of marker genes (Figure 8G) indicated that patients with higher risk scores of FCER2 showed lower risk score status and superior survival. However, CASP14, DKK1, ECT2, FLNC, HMMR, IGFBP1, KRT6A, and TYMS exhibited opposing results.
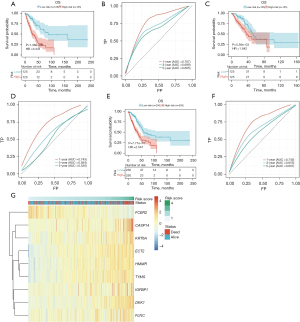
In the external validation cohort (GSE13213), consistent with the previous findings, patients with higher risk scores exhibited significantly shorter OS compared to those with lower risk scores (Figure 9A). The AUC values of the ROC curves for the 1-year OS in the validation cohort were 0.87, respectively, indicating the favorable predictive ability of our model (Figure 9B). In addition, our verification results revealed significant correlations between the risk score and gender, T stage, age, and pathologic stage (Figure 9C-9E).
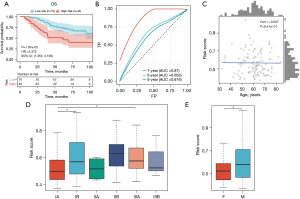
Advanced DDR risk score was correlated with poor clinical factors and lower OS in patients with LUAD
We investigated the association between the DDR risk score and clinical factors in patients with LUAD. The high-risk group exhibited poor clinical characteristics, including advanced M stage (P<0.01, Figure 10A), advanced N stage (P<0.01, Figure 10B), lower pathologic stage (P<0.01, Figure 10C,10D), and advanced T stage (P<0.01, Figure 10E,10F). Additionally, higher risk scores were observed in male patients (P<0.01, Figure 10G) and showed a negative correlation with age (correlation =−0.028, P=0.531). Tumor purity was found to have a positive correlation with the risk values (correlation =0.098, P=0.0277, Figure 10H,10I). Univariate Cox regression analysis revealed significant associations between the risk score and various clinical variables, including risk (HR =2.1, P=4.23e−15), age (HR =1.01, P=0.299), sex (HR =1.05, P=0.753), T stage (HR =1.52, P=8.91e−06); N stage (HR =1.7, P=1.39e−09), M stage (HR =2.13, P=0.00583), and pathologic stage (HR =1.66, P=8.08e−13, Figure 10J). Subsequently, multivariate Cox regression analysis revealed that the DDR risk score could serve as an independent prognostic factor (HR =1.96, P=1.33e−15, Figure 10K).
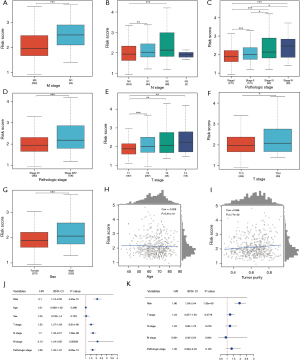
DDR signature is a promising predictor for immunotherapy
Firstly, the differential expression of immune checkpoint genes was demonstrated. Notably, ADORA2A, BTLA, CD200, LAIR1, and TIGIT were significantly up-regulated in the low-risk score subgroup. CD274, CD276, IDO1, and PVR were significantly up-regulated in the high-risk score subgroup (Figure 11). The TMB of each sample in TCGA-LUAD was then calculated by maftools. The median TMB was 3.4/MB (Figure 12A). TMB scores were significantly higher in the high-risk group (P<0.01, Figure 12B), indicating a better response to immunotherapy. The top 10 genes with the highest frequencies of driver mutations are shown in Figure 12C,12D. TP53 had a more frequent mutation in the high-risk group, indicating a poorer prognosis. The difference in the number of CTA between different risk groups was tested using wilcox.test. The result showed a higher CTA number in the high-risk group than the low-risk group (P<0.01, Figure 13). Moreover, the low-risk group had a higher TIDE score compared to the high-risk group (P<0.01, Figure 14). Taken together, patients with a high-risk score may have a better immunotherapy effect.
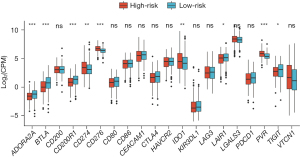
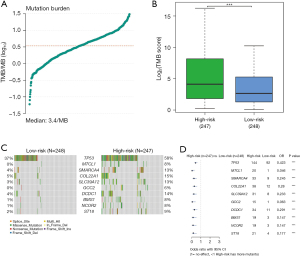
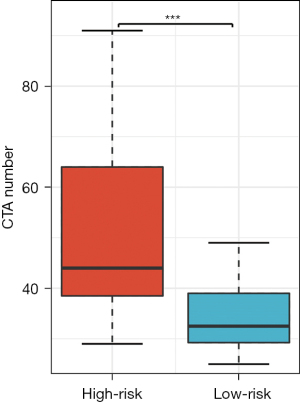
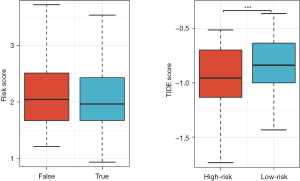
Discussion
DDR plays a crucial role in the development of various cancers by regulating multiple pathways involved in the interaction between tumor and immune cells (25-28). DNA damage can occur through endogenous events, such as oxidative damage, replication fork collapse, or errors that naturally occur during DNA replication or immune cell maturation, as well as exogenous factors such as ultraviolet rays, ionizing radiation, or chemical reagents. DNA repair is a pivotal mechanism for preserving genome stability and repairing DNA lesions. Deficiencies in the DNA repair pathway can influence tumor development, metastasis, and prognosis (14,29,30). The objective of this study was to investigate the predictive function of a DDR gene-related signature in the prognosis and immunotherapy response of LUAD.
Distinct clinical and molecular characteristics were observed in association with different DDR signatures. Specifically, patients belonging to the DDR-activated subgroup exhibited aggressive clinical manifestations, including advanced stage, poor differentiation, and an unfavorable prognosis. In contrast, the DDR-suppressed subgroup showed a higher frequency of alterations in base excision repair, Fanconi anemia pathway, homologous recombination, and dislocation repair. TMB is an important indicator that affects the treatment response and prognosis of lung cancer. Our results demonstrated that the DDR-activated subgroup had a significantly higher amount of variations in TMB compared to the DDR-suppressed subgroup. The TP53 gene, a critical DNA repair factor implicated in various cancers, has been reported to be more frequently mutated in the DDR-activated subtype (31,32). Notably, we found a higher frequency of troponin (TNN) variants in the DDR-activated subgroup, which has been associated with poor OS, increased immunogenicity, and altered immunotherapy prognosis in LUAD (33). Furthermore, CSMD3 was identified as being higher expressed in the DDR-activated subgroup. Previous studies have highlighted the involvement of CSMD3 gene mutations in immune response regulation and tumor prognosis (34,35).
We further investigated the immune microenvironment in LUAD and discovered that different DDR subtypes were associated with distinct immune profiles. In the DDR-suppressed subgroup, there was a notable increase in plasma cells, T lymphocytes, and activated memory CD4 cells, with mast cells being particularly abundant. Tumor-invading mast cells have been linked to resistance to anti-PD-1 therapy (36). Conversely, the DDR-activated subgroups exhibited heightened expression of M0 macrophages, M1 macrophages, activated memory CD4 T cells, CD8 T cells, follicular helper T cells, resting NK cells, and neutrophils. This immune cell composition could potentially enhance immune responses and improve patient outcomes (37-39). For certain patients with lung cancer, the use of immune checkpoint inhibitors (ICIs) has demonstrated improved long-term efficacy. Currently, several drugs are available to delay or prevent resistance to ICIs, and a high expression of PD-L1 serves as a significant indicator for ICI treatment (40-43). Notably, in our study, PD-L1 (CD274) expression was significantly elevated in the DDR-activated subgroup. These findings suggest that DDR subtypes exhibit distinct differences in immune cell infiltration, indicating potential variations in immunotherapy responses between the subtypes.
According to previous research, DDR genes have prognostic potential in various cancer types (44-46). In our study, we selected nine DDR-related genes (CASP14, DKK1, ECT2, FLNC, HMMR, IGFBP1, KRT6A, TYMS, and FCER2) to create a signature for identifying DDR subtypes in patients with LUAD, with the aim of clinical application. Patients were classified into different DDR types based on this signature in both the training and validation cohorts. To assess the accuracy of the model, patients were further divided into high-risk and low-risk groups. KM survival analysis demonstrated that the high-risk group had a poorer prognosis compared to the low-risk group. Moreover, univariate and multivariate Cox regression analyses indicated a significant negative correlation between the risk score derived from the DDR signature and other clinicopathological parameters in predicting survival. Additionally, the high-risk group exhibited lower TIDE scores, higher numbers of CTAs, and higher TMB scores, suggesting that patients with a high-risk score may have a more favorable response to immunotherapy (47,48). However, further randomized trials involving patients with LUAD receiving immunotherapy are necessary to validate the predictive performance of the DDR signature in terms of immunotherapy response. In summary, the risk score derived from the DDR signature can serve as a stable and independent indicator for prognosis and potential immunotherapy effect. It holds important clinical significance as an effective tool for classifying patients with LUAD. However, further research and validation are required to fully elucidate the clinical implications and utility of the risk score in guiding treatment decisions for patients with LUAD.
Conclusions
Our study contributes to the understanding of DDR heterogeneity and the identification of DDR subtypes in patients with LUAD. The distinct characteristics of DDR subtypes offer valuable insights into the clinical management and decision-making of LUAD. We have successfully developed a nine-gene risk signature associated with DDR in LUAD, which demonstrated its potential as an effective and stable classification tool for clinical practice. Moreover, our DDR subtype signature holds promise as a biomarker for guiding immunotherapy in patients with LUAD. Overall, these findings have significant implications for LUAD patient care and highlight the importance of considering DDR subtypes in personalized treatment approaches.
Acknowledgments
Funding: This study was funded by
Footnote
Reporting Checklist: The authors have completed the TRIPOD reporting checklist. Available at https://jtd.amegroups.com/article/view/10.21037/jtd-23-1746/rc
Peer Review File: Available at https://jtd.amegroups.com/article/view/10.21037/jtd-23-1746/prf
Conflicts of Interest: All authors have completed the ICMJE uniform disclosure form (available at https://jtd.amegroups.com/article/view/10.21037/jtd-23-1746/coif). A.A. receives consulting fees from Amgen, AstraZeneca, Roche, Astellas, Takeda, BMS, MSD, Pfizer, Merck, Novartis and payment for lectures, presentations from Amgen and Novartis. The other authors have no conflicts of interest to declare.
Ethical Statement: The authors are accountable for all aspects of the work in ensuring that questions related to the accuracy or integrity of any part of the work are appropriately investigated and resolved. The study was conducted in accordance with the Declaration of Helsinki (revised in 2013).
Open Access Statement: This is an Open Access article distributed in accordance with the Creative Commons Attribution-NonCommercial-NoDerivs 4.0 International License (CC BY-NC-ND 4.0), which permits the non-commercial replication and distribution of the article with the strict proviso that no changes or edits are made and the original work is properly cited (including links to both the formal publication through the relevant DOI and the license). See: https://creativecommons.org/licenses/by-nc-nd/4.0/.
References
- Hirsch FR, Scagliotti GV, Mulshine JL, et al. Lung cancer: current therapies and new targeted treatments. Lancet 2017;389:299-311. [Crossref] [PubMed]
- Wahla AS, Zoumot Z, Uzbeck M, et al. The Journey for Lung Cancer Screening where we Stand Today. Open Respir Med J 2022;16:e187430642207060. [Crossref] [PubMed]
- Duma N, Santana-Davila R, Molina JR. Non-Small Cell Lung Cancer: Epidemiology, Screening, Diagnosis, and Treatment. Mayo Clin Proc 2019;94:1623-40. [Crossref] [PubMed]
- Park CK, Cho HJ, Choi YD, et al. A Phase II Trial of Osimertinib in the Second-Line Treatment of Non-small Cell Lung Cancer with the EGFR T790M Mutation, Detected from Circulating Tumor DNA: LiquidLung-O-Cohort 2. Cancer Res Treat 2019;51:777-87. [Crossref] [PubMed]
- Hensing T, Chawla A, Batra R, et al. A personalized treatment for lung cancer: molecular pathways, targeted therapies, and genomic characterization. Adv Exp Med Biol 2014;799:85-117. [Crossref] [PubMed]
- Li H, Sha X, Wang W, et al. Identification of lysosomal genes associated with prognosis in lung adenocarcinoma. Transl Lung Cancer Res 2023;12:1477-95. [Crossref] [PubMed]
- Nieder C, Mehta MP, Geinitz H, et al. Prognostic and predictive factors in patients with brain metastases from solid tumors: A review of published nomograms. Crit Rev Oncol Hematol 2018;126:13-8. [Crossref] [PubMed]
- Niewada M, Macioch T, Konarska M, et al. Immune checkpoint inhibitors combined with tyrosine kinase inhibitors or immunotherapy for treatment-naïve metastatic clear-cell renal cell carcinoma-A network meta-analysis. Focus on cabozantinib combined with nivolumab. Front Pharmacol 2022;13:1063178. [Crossref] [PubMed]
- Jeggo PA, Pearl LH, Carr AM. DNA repair, genome stability and cancer: a historical perspective. Nat Rev Cancer 2016;16:35-42. [Crossref] [PubMed]
- Filippi L, Palumbo B, Bagni O, et al. DNA Damage Repair Defects and Targeted Radionuclide Therapies for Prostate Cancer: Does Mutation Really Matter? A Systematic Review. Life (Basel) 2022;13:55. [Crossref] [PubMed]
- Sonnenblick A, de Azambuja E, Azim HA Jr, et al. An update on PARP inhibitors--moving to the adjuvant setting. Nat Rev Clin Oncol 2015;12:27-41. [Crossref] [PubMed]
- Knijnenburg TA, Wang L, Zimmermann MT, et al. Genomic and Molecular Landscape of DNA Damage Repair Deficiency across The Cancer Genome Atlas. Cell Rep 2018;23:239-254.e6. [Crossref] [PubMed]
- Yu Z, Vyungura O, Zhao Y. Molecular subtyping and IMScore based on immune-related pathways, oncogenic pathways, and DNA damage repair pathways for guiding immunotherapy in hepatocellular carcinoma patients. J Gastrointest Oncol 2022;13:3135-53. [Crossref] [PubMed]
- Shao C, Wang Y, Pan M, et al. The DNA damage repair-related gene PKMYT1 is a potential biomarker in various malignancies. Transl Lung Cancer Res 2021;10:4600-16. [Crossref] [PubMed]
- Gu L, Xu Y, Jian H. Identification of a 15 DNA Damage Repair-Related Gene Signature as a Prognostic Predictor for Lung Adenocarcinoma. Comb Chem High Throughput Screen 2022;25:1437-49. [Crossref] [PubMed]
- Wu D, Huang L, Mao J, et al. Combination of Tumor Mutational Burden and DNA Damage Repair Gene Mutations with Stromal/Immune Scores Improved Prognosis Stratification in Patients with Lung Adenocarcinoma. J Oncol 2022;2022:6407344. [Crossref] [PubMed]
- Robinson MD, McCarthy DJ, Smyth GK. edgeR: a Bioconductor package for differential expression analysis of digital gene expression data. Bioinformatics 2010;26:139-40. [Crossref] [PubMed]
- Wilkerson MD, Hayes DN. ConsensusClusterPlus: a class discovery tool with confidence assessments and item tracking. Bioinformatics 2010;26:1572-3. [Crossref] [PubMed]
- Groeneveld CS, Chagas VS, Jones SJM, et al. RTNsurvival: an R/Bioconductor package for regulatory network survival analysis. Bioinformatics 2019;35:4488-9. [Crossref] [PubMed]
- Ashburner M, Ball CA, Blake JA, et al. Gene ontology: tool for the unification of biology. The Gene Ontology Consortium. Nat Genet 2000;25:25-9. [Crossref] [PubMed]
- Kanehisa M, Goto S. KEGG: kyoto encyclopedia of genes and genomes. Nucleic Acids Res 2000;28:27-30. [Crossref] [PubMed]
- Hänzelmann S, Castelo R, Guinney J. GSVA: gene set variation analysis for microarray and RNA-seq data. BMC Bioinformatics 2013;14:7. [Crossref] [PubMed]
- Mayakonda A, Lin DC, Assenov Y, et al. Maftools: efficient and comprehensive analysis of somatic variants in cancer. Genome Res 2018;28:1747-56. [Crossref] [PubMed]
- Almeida LG, Sakabe NJ, deOliveira AR, et al. CTdatabase: a knowledge-base of high-throughput and curated data on cancer-testis antigens. Nucleic Acids Res 2009;37:D816-9. [Crossref] [PubMed]
- Chabanon RM, Rouanne M, Lord CJ, et al. Targeting the DNA damage response in immuno-oncology: developments and opportunities. Nat Rev Cancer 2021;21:701-17. [Crossref] [PubMed]
- Huang R, Zhou PK. DNA damage repair: historical perspectives, mechanistic pathways and clinical translation for targeted cancer therapy. Signal Transduct Target Ther 2021;6:254. [Crossref] [PubMed]
- Lu Z, Priya Rajan SA, Song Q, et al. 3D scaffold-free microlivers with drug metabolic function generated by lineage-reprogrammed hepatocytes from human fibroblasts. Biomaterials 2021;269:120668. [Crossref] [PubMed]
- Triozzi PL, Stirling ER, Song Q, et al. Circulating Immune Bioenergetic, Metabolic, and Genetic Signatures Predict Melanoma Patients' Response to Anti-PD-1 Immune Checkpoint Blockade. Clin Cancer Res 2022;28:1192-202. [Crossref] [PubMed]
- Li W, Zhang M, Huang C, et al. Genetic variants of DNA repair pathway genes on lung cancer risk. Pathol Res Pract 2019;215:152548. [Crossref] [PubMed]
- Liu T, Hu A, Chen H, et al. Comprehensive analysis identifies DNA damage repair-related gene HCLS1 associated with good prognosis in lung adenocarcinoma. Transl Cancer Res 2023;12:2613-28. [Crossref] [PubMed]
- Zhu Y, Guo YB, Xu D, et al. A computed tomography (CT)-derived radiomics approach for predicting primary co-mutations involving TP53 and epidermal growth factor receptor (EGFR) in patients with advanced lung adenocarcinomas (LUAD). Ann Transl Med 2021;9:545. [Crossref] [PubMed]
- Li S, Wang L, Wang Y, et al. The synthetic lethality of targeting cell cycle checkpoints and PARPs in cancer treatment. J Hematol Oncol 2022;15:147. [Crossref] [PubMed]
- Wang Z, Wang C, Lin S, et al. Effect of TTN Mutations on Immune Microenvironment and Efficacy of Immunotherapy in Lung Adenocarcinoma Patients. Front Oncol 2021;11:725292. [Crossref] [PubMed]
- Lu N, Liu J, Xu M, et al. CSMD3 is Associated with Tumor Mutation Burden and Immune Infiltration in Ovarian Cancer Patients. Int J Gen Med 2021;14:7647-57. [Crossref] [PubMed]
- Weng W, Yu L, Li Z, et al. The immune subtypes and landscape of sarcomas. BMC Immunol 2022;23:46. [Crossref] [PubMed]
- Somasundaram R, Connelly T, Choi R, et al. Tumor-infiltrating mast cells are associated with resistance to anti-PD-1 therapy. Nat Commun 2021;12:346. [Crossref] [PubMed]
- Melssen M, Slingluff CL Jr. Vaccines targeting helper T cells for cancer immunotherapy. Curr Opin Immunol 2017;47:85-92. [Crossref] [PubMed]
- Kennedy R, Celis E. Multiple roles for CD4+ T cells in anti-tumor immune responses. Immunol Rev 2008;222:129-44. [Crossref] [PubMed]
- Zhong R, Chen D, Cao S, et al. Immune cell infiltration features and related marker genes in lung cancer based on single-cell RNA-seq. Clin Transl Oncol 2021;23:405-17. [Crossref] [PubMed]
- Passaro A, Brahmer J, Antonia S, et al. Managing Resistance to Immune Checkpoint Inhibitors in Lung Cancer: Treatment and Novel Strategies. J Clin Oncol 2022;40:598-610. [Crossref] [PubMed]
- Hiley CT, Le Quesne J, Santis G, et al. Challenges in molecular testing in non-small-cell lung cancer patients with advanced disease. Lancet 2016;388:1002-11. [Crossref] [PubMed]
- Ichiki Y, Fukuyama T, Ueno M, et al. Immune profile analysis of peripheral blood and tumors of lung cancer patients treated with immune checkpoint inhibitors. Transl Lung Cancer Res 2022;11:2192-207. [Crossref] [PubMed]
- Moran JA, Adams DL, Edelman MJ, et al. Monitoring PD-L1 Expression on Circulating Tumor-Associated Cells in Recurrent Metastatic Non-Small-Cell Lung Carcinoma Predicts Response to Immunotherapy With Radiation Therapy. JCO Precis Oncol 2022;6:e2200457. [Crossref] [PubMed]
- Zhan J, Wu S, Zhao X, et al. A Novel DNA Damage Repair-Related Gene Signature for Predicting Glioma Prognosis. Int J Gen Med 2021;14:10083-101. [Crossref] [PubMed]
- Li N, Zhao L, Guo C, et al. Identification of a novel DNA repair-related prognostic signature predicting survival of patients with hepatocellular carcinoma. Cancer Manag Res 2019;11:7473-84. [Crossref] [PubMed]
- Jinjia C, Xiaoyu W, Hui S, et al. The use of DNA repair genes as prognostic indicators of gastric cancer. J Cancer 2019;10:4866-75. [Crossref] [PubMed]
- Salmaninejad A, Zamani MR, Pourvahedi M, et al. Cancer/Testis Antigens: Expression, Regulation, Tumor Invasion, and Use in Immunotherapy of Cancers. Immunol Invest 2016;45:619-40. [Crossref] [PubMed]
- Jiang P, Gu S, Pan D, et al. Signatures of T cell dysfunction and exclusion predict cancer immunotherapy response. Nat Med 2018;24:1550-8. [Crossref] [PubMed]