Systematic analysis of apoptosis-related genes in the prognosis of lung squamous cell carcinoma: a combined single-cell RNA sequencing study
Highlight box
Key findings
• We constructed a prognostic model based on apoptosis-related genes associated with lung squamous cell carcinoma and integrated single-cell RNA sequencing, providing a fresh perspective on disease understanding.
What is known and what is new?
• Lung squamous cell carcinoma is a type of lung cancer, and apoptosis-related genes play a role in cancer.
• We constructed a prognostic model for lung squamous cell carcinoma based on apoptosis-related genes and integrated single-cell RNA sequencing, providing a fresh perspective on disease understanding.
What is the implication, and what should change now?
• The significance of our study suggests the potential of using our prognostic model to guide personalized treatment for lung squamous cell carcinoma. However, further experimental and clinical research is needed to validate its effectiveness.
Introduction
Lung cancer is the leading cause of cancer-related death, accounting for 19.4% of cancer-related deaths annually (1). Non-small cell lung cancer (NSCLC) constitutes over 80% of all lung cancer cases and includes two main types: non-squamous cell carcinoma (including adenocarcinoma, large cell carcinoma, and other cell types) and squamous cell carcinoma, which accounts for approximately 25–30% of NSCLC cases (2). Squamous cell carcinoma of the lung often develops in the central part of the lung and has the ability to grow to a large size (3). Lung squamous cell carcinoma (LUSC) is a particularly challenging disease to treat (4). Comparative results from relevant clinical trials show that the median survival of patients with advanced squamous cell carcinoma who receive first-line treatment is about 30% shorter than that of patients with other NSCLC subtypes (5,6). The tumor, node, metastasis (TNM) staging system is widely used for LUSC, but it has certain limitations because patients with the same TNM stage can have different survival outcomes (7). A few significant improvements have been achieved in molecular targeted therapy and immunotherapy for NSCLC. However, the vast majority of LUSC cases do not harbour targetable oncogenic mutations or develop resistance to the current treatment methods (8,9). Therefore, it is necessary to identify new biomarkers that can provide diagnostic and prognostic information for LUSC.
Cell apoptosis is an evolutionarily conserved, genetically regulated form of cell suicide that plays an important role in the development and maintenance of tissue homeostasis in multicellular organisms (10). Cell apoptosis is crucial for eliminating cells that have undergone mutations or transformations in the body. Therefore, cancer cells often evolve multiple efficient and diverse mechanisms to evade cell apoptosis (11). Cell apoptosis can be induced through both extrinsic and intrinsic pathways. The extrinsic pathway is stimulated by death receptors, such as Fas, tumor necrosis factor receptors, and tumor necrosis factor-related apoptosis-inducing ligand (TRAIL), while the intrinsic pathway is initiated by DNA damage, energy depletion, and hypoxia, which can lead to dephosphorylation and cleavage of proapoptotic proteins (12). Genetic analysis of NSCLC has revealed inherited and somatic mutations in the EGFR and P53 genes, as well as somatic mutations in the KRAS, BRAF, ERBB2, MET, STK11, PIK3CA, and PARK2 genes. These gene mutations have led to the development of new strategies for targeted treatment (13). Exploring the targeted apoptosis mechanisms in NSCLC represents another approach aimed at selectively killing cancer cells while preserving normal cells. However, related study has shown that there is no correlation between the response to apoptosis-targeted drugs and the histological subtypes of NSCLC. The presence of apoptosis targets, such as TRAIL receptors and death-inducing signaling complex (DISC) compounds, anti-apoptotic B-cell lymphoma 2 (BCL-2) and inhibitor of apoptosis (IAP) family members, is important but cannot predict the response to corresponding targeted therapies (14). Moreover, patients with LUSC often have a poor prognosis. Therefore, identifying key molecules, establishing stable and effective predictive models, and implementing precision treatment are crucial for improving the prognosis of these patients.
In recent years, single-cell RNA sequencing (scRNA-seq) has developed rapidly and is a departure from the conventional “bulk” RNA sequencing (RNA-seq) methods. scRNA-seq captures the transcriptomes of individual cells and allows for unprecedented resolution to evaluate the fundamental biological properties of specific cell populations within complex multicellular ecosystems (15). By exploring the heterogeneity of cells in the tumor microenvironment at the single-cell level, we can greatly improve our understanding of the transcriptional, genetic, metabolic, and other characteristics of thousands of individual cells, thereby deepening our analysis of the cells involved in tumor progression (16). Integrating clinical pathological information and single-cell sequencing data can facilitate the identification of novel diagnostic and prognostic biomarkers as well as potential treatment-related cell types or states (17). However, scRNA-seq is relatively expensive, and therefore the available sample datasets are relatively limited. Nevertheless, the information obtained from scRNA-seq is highly valuable for exploring the characteristics of each cell subpopulation in the sample and the interactions of each cell in the tumor immune microenvironment (TIME) (18). In this study, we aimed to construct an apoptosis prognostic model associated with LUSC survival. Through bioinformatics analysis, we identified four apoptosis-related genes (ARGs) that were associated with LUSC prognosis, using these genes to construct a risk score and prognostic model. The accuracy of this model was validated in a test set, and the potential role of the risk score in guiding immunotherapy for patients with LUSC was investigated. Finally, we further elucidated the role of the prognostic-related ARGs at the cellular level in the occurrence and development of LUSC with the aim of improving the treatment outcomes and prognosis of patients with LUSC at the single-cell level. This study aims to construct a survival prognostic model for LUSC based on the differential expression of ARGs. Through the application of univariate Cox regression, least absolute shrinkage and selection operator (LASSO) regression, and multivariate Cox regression analyses, we intend to assess the correlation of prognostically relevant ARGs in LUSC. Additionally, the objectives of this study include investigating how apoptosis regulatory imbalance is associated with tumorigenesis, exploring the expression differences of apoptosis-related genes in LUSC patients, and evaluating the prognostic value of these genes. The study also aims to identify potential molecular targets to enhance diagnostic and therapeutic strategies for LUSC, ultimately improving patient survival rates. We present this article in accordance with the TRIPOD reporting checklist (available at https://jtd.amegroups.com/article/view/10.21037/jtd-23-1712/rc).
Methods
Data source and preprocessing
RNA-seq data and clinical information of patients with LUSC were obtained from The Cancer Genome Atlas (TCGA) database (https://portal.gdc.cancer.gov/). Overall survival (OS) and other clinical features, including age, gender, T stage, N stage, M stage, and clinical stage, were extracted from the downloaded cases, and missing clinical information was removed. scRNA-seq data were downloaded from the Gene Expression Omnibus (GEO) database (GSE200972) (https://www.ncbi.nlm.nih.gov/geo/), and four tumor tissue samples were selected for analysis. The raw data contained 23,008 genes and 8,452 cells. The “PercentageFeatureSet” function in R version 4.2.1 (The R Foundation for Statistical Computing) was used to calculate the percentage of mitochondria and RNA, and cells with a gene expression greater than 300 and less than 7,000 and with a mitochondrial content of less than 30% were selected as the basis for subsequent analysis. The study was conducted in accordance with the Declaration of Helsinki (as revised in 2013).
Differential expression analysis of ARGs
A series of bioinformatics analyses were performed in R. Specifically, the “limma” R package was used to screen for differentially expressed ARGs between tumor and nontumor tissues in TCGA cohort. For each gene, a Wilcoxon rank-sum test was executed using a for loop to calculate logFC (log2 fold change) and P values. The ultimate criteria for selection are a false discovery rate (FDR) <0.05 and |log2 (fold change)| >1 being the screening criteria.
Development and validation of the prognostic model
In this study, an ARG-related LUSC prognostic model was constructed to predict the OS of patients with LUSC. The TCGA-LUSC cohort was divided into a training set and a testing set using the createDataPartition function, the prognostic features were constructed based on the training set, and then the predictive performance was validated in the validation set. Univariate Cox regression analysis was used to determine the prognostic ARGs in LUSC, and the results were visualized using a forest plot generated by the “forestplot” R package. The LASSO penalized Cox proportional hazards regression (implemented with R package “glmnet”) was used to reduce the genes of the model and limit the complexity of solving the problem of overfitting. Finally, four prognostic ARGs were determined via multivariate Cox regression analysis, and a 4-gene signature was constructed. In the training and testing sets, the risk score of each patient was calculated based on the regression coefficient of the ARGs. The risk score was calculated using the following formula: risk score = expression level of gene a × coefficient a + expression level of gene b × coefficient b + expression level of gene c × coefficient c + … + expression level of gene n × coefficient n. Patients with LUSC were divided into high-risk and low-risk groups based on the median risk value, and Kaplan-Meier survival curves were used to compare the survival between the low-risk and high-risk groups, with P<0.05 being considered statistically significant. Finally, the receiver operating characteristic (ROC) curves and area under the curve (AUC) values for both the training and testing sets were calculated and plotted using the survivalROC package. Time-dependent ROC curves were established to reflect the predictive performance of the prognostic model.
Nomogram development and validation for prognostic risk prediction
To provide a quantitative method for predicting the 3-, 5-, and 7-year survival probabilities of patients with LUSC for clinical doctors, we developed a nomogram integrating various clinical risk factors and apoptosis prognostic models. We then evaluated the calibration curve of the nomogram by plotting the predicted probability of the observed rate using the nomogram. Subsequently, 1,000 repeated samplings were used for cross-validation to construct a concordance-index (C-index) plot to illustrate the predictive ability of the model. The prognostic accuracy of the model was evaluated through sensitivity and specificity using the ROC curve. Finally, a heatmap was constructed to evaluate the correlation between prognostic features and clinical factors.
Functional enrichment analysis
Gene Ontology (GO) and Kyoto Encyclopedia of Genes and Genomes (KEGG) pathway analyses were performed using the Database for Annotation, Visualization and Integrated Discovery (DAVID; https://david.ncifcrf.gov/). First, we obtained pathway enrichment information by inputting ARGs into DAVID and selected the top 10 pathways with the smallest P values for enrichment analysis in both GO and KEGG. The enriched pathways were displayed using the “ggplot” R package.
Gene mutation analysis
Tumor mutational burden (TMB) reflects the number of mutations in cancer. We downloaded mutation data of patients with LUSC from TCGA and performed gene mutation analysis using the “maftools” R package. We calculated the optimal cutoff value for TMB data using the surv_cutpoint function, and then compared the TMB between the high-risk and low-risk groups. Survival analysis was performed based on the TMB score, and the somatic mutations of prognostic genes were identified via the cBioPortal database(http://www.cbioportal.org/).
Relationship of molecular patterns with TME in LUSC
The ESTIMATE algorithm was used to evaluate whether the risk score of patients with LUSC was associated with immune and stromal components. Single-sample gene set enrichment analysis (ssGSEA) was applied to quantify the infiltration levels of 16 immune cells and 13 immune-related pathways between the two risk groups with the “GSVA” package. Immune checkpoint genes with differential expression (with P<0.05 as the threshold) were selected, and the expression differences of immune checkpoint genes between the high-risk and low-risk groups were examined.
Processing of single-cell sequencing data
The NSCLC scRNA-seq dataset, GSE200972, was downloaded from the GEO database, containing 19 tissue samples from four patients with multiple primary lung cancer (1 squamous carcinoma and 3 adenocarcinoma cases). We selected four tumor samples for subsequent analysis. The samples were integrated using the anchors method in the R package “Seurat”. To exclude cell data with genes detectable in only 3 or fewer cells and cell data with fewer than 300 genes detected in 1 cell while retaining those with gene counts between 300 and 7,000, mitochondrial content less than 10%, blood cell content less than 1%, and a total transcript count less than 100,000. The “FindVariableFeatures” function in R was used to identify the top 3,000 highly variable genes, and the top 10 genes were displayed. Principal component analysis (PCA) was performed on single-cell samples, and the top 13 principal components (PCs) were selected for subsequent analysis. The samples were subjected to overall dimensionality reduction analysis via the uniform manifold approximation and projection (UMAP) algorithm. Different cell clusters were manually annotated using the R package “singleR”, the CellMarker database, and references as auxiliary annotations.
Construction of a pseudotemporal analysis trajectory
Pseudotemporal analysis was performed using the Monocle 2 algorithm through branching trajectory analysis. Gene expression levels and dispersion were filtered and sorted to exclude noise and irrelevant genes in the single-cell data. The “orderCells” function in R was used to sort cells and determine their position on the trajectory. Trajectories were constructed for two clusters, and differentiation trajectories of cells in different states were plotted. Subsequently, the expression of prognostic genes in the two clusters was visualized, and the pseudotemporal trajectories of several prognostic genes in T cells were observed.
Statistical analysis
All statistical analyses were performed using R software version 4.2.1. A P value <0.05 was considered statistically significant. Univariate Cox, LASSO regression analysis, and multivariate Cox analyses were used to select key ARGs associated with survival. Survival variations between different groups were compared using Kaplan-Meier curves. The predictive ability of this model was tested using ROC analysis.
Results
Identification of the differentially expressed genes in LUSC
We obtained RNA-seq data and clinical follow-up data from 502 LUSC samples and 51 normal lung tissue samples in TCGA dataset. Differential expression analysis was performed to determine the expression levels of ARGs in tumor and normal samples, and 56 differentially expressed genes were identified (Table S1), most of which were enriched in tumor samples (Figure 1A,1B). Univariate Cox regression was used to screen ARGs, and 8 prognostic-related ARGs were identified (Figure 1C) . LASSO Cox regression analysis and 10-fold cross-validation were used to determine the optimal regularization parameter λ to obtain the best model performance (Figure 1D,1E). Finally, multivariate analysis was performed to identify four ARGs (BMP2, GPX3, JUN, and AIFM3) that were most relevant to the prognosis of patients with LUSC. Kaplan-Meier analysis was used to analyze the impact of the high and low expression of these four genes on prognosis, and the three most significant genes (BMP2, GPX3, and JUN) were identified (Figure S1A-S1C). We found that patients with a high expression of BMP2, GPX3, and JUN had a worse prognosis than did those with low expression. Correlation analysis revealed a close relationship between these genes (Figure S1D-S1F), suggesting a possible synergistic effect on prognosis.
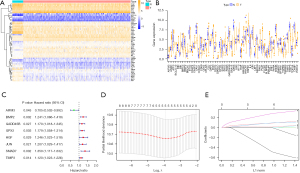
Construction of a risk score based on prognostic ARGs
TCGA cohort was randomly divided into a training set and a testing set, and based on the median risk cutoff value of patients, they were further divided into high-risk and low-risk groups (Figure 2A,2B). The risk score was calculated as follows: risk score = (−0.411 × expression level of AIFM3) + (0.084 × expression level of BMP2) + (0.0838 × expression level of GPX3) + (0.258 × expression level of JUN). The patients in the training and testing sets were sorted from left to right based on their increasing risk scores. The results showed that as the risk score increased, the risk of death increased and the survival time decreased. The trend of survival status and survival time in the testing set was consistent with that in the training set, and the decrease in survival time was significantly correlated with the increase in risk score (Figure 2C,2D). Similarly, Kaplan-Meier survival curves showed that patients in the high-risk group had a lower survival rate than those in the low-risk group (Figure 2E,2F). The predictive performance of the prognostic risk score model was evaluated using time-dependent ROC curves and the AUC (Figure 2G,2H). The results showed that the risk score model had good predictive performance for patients with LUSC, as confirmed by mutual verification between the training and testing sets.
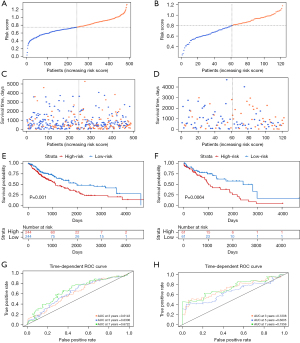
Screening of independent prognostic factors and construction of a nomogram
To identify independent prognostic factors, univariate and multivariate Cox analyses were performed on clinical features and risk scores. We found that risk score and tumor stage were independent prognostic factors for patients, and a nomogram model was constructed to improve its predictive ability (Figure 3A). By constructing calibration curves, it was found that the calibration curves of the 3-, 5-, and 7-year calibration points were relatively well matched with the standard curves, indicating that the model had good predictive performance (Figure 3B). The time-dependent ROC curves and C-index indicated that the nomogram based on the independent prognostic factors had better prognostic predictive performance for LUSC than did the single clinical features (Figure 3C,3D). Finally, we linked the risk score with clinical factors, such as tumor stage, and the results showed that patients with high-risk scores had shorter survival times (Figure 3E). In conclusion, our study results indicated that the risk score of prognostic genes can predict patient prognosis.
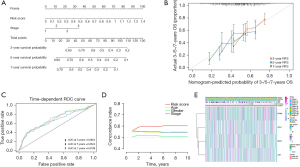
Functional analysis of differentially expressed ARGs
To clarify the biological functions and important pathways of differentially expressed ARGs, we performed GO enrichment analysis to enrich the functions and pathways related to biological process (BP), cell component (CC), and molecular function (MF), and displayed the top 10 pathways with the smallest P values. We found that regulation of the apoptotic process was significantly enriched in BP (Figure 4A), cytoplasmic components such as mitochondrial outer membrane were significantly enriched in CC (Figure 4B), and protein complexes such as cysteine-type endopeptidase were significantly enriched in MF (Figure 4C). Similarly, KEGG enrichment analysis was performed using the same GO enrichment analysis method, and other pathways such as the apoptosis pathway and cancer pathway were enriched (Figure 4D). The detailed results of GO and KEGG enrichment analysis can be found in Table S2.
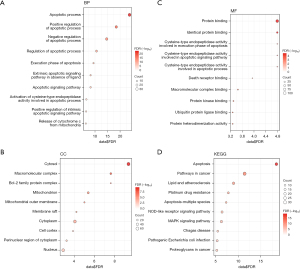
TMB of prognostic biomarkers associated with ARGs in LUSC samples
A high TMB indicates the presence of mutations in tumor cells, which may make them more susceptible to attack by the immune system, thereby increasing the effectiveness of immunotherapy. To investigate the significance of tumor mutations in LUSC, we downloaded LUSC somatic mutation data and calculated TMB scores, comparing the genomic mutation differences between the high-risk and low-risk groups. The mutation rates in TP53, TTN, CSMD3, MUC16, and RYR2 were higher than or equal to 35% in patients with LUSC in both risk groups. Interestingly, the likelihood of these gene mutations was greater in the low-risk group compared to the high-risk group (Figure 5A,5B). To investigate the impact of TMB status on the prognosis of patients with LUSC, survival analysis was performed on different TMB subgroups, and it was found that the prognosis of patients with high TMB was better than that of patients with low TMB (Figure 5C). Subsequently, we combined TMB with risk scores for survival analysis, and the results showed that patients with high TMB and low risk scores had the best prognosis (Figure 5D). Finally, we examined the mutation rates of the four genes in the prognostic model and found that a segment of DNA sequence in the AIFM3 gene was duplicated, leading to an increase in copy number, which may contribute to the occurrence and development of tumors and an increase in the expression of other genes. However, the mutation rates of these genes were all very low (Figure 5E).
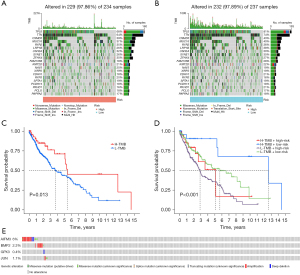
Assessment of tumor immune infiltration and immune checkpoint
The correlation between risk scores and tumor stromal and immune scores was analyzed, and the results showed that as the risk score increased, the abundance of tumor stroma and immune infiltration also increased (Figure 6A,6B). This implied a positive correlation between risk scores and the degree of tumor stromal and immune cell infiltration. Therefore, an increase in risk score may lead to changes in the tumor microenvironment and immune response, promoting tumor stromal and immune cell infiltration. To further understand the characteristics of the tumor microenvironment and its impact on tumor treatment, samples were divided into high-risk and low-risk groups, and the differences in tumor microenvironment score and tumor microenvironment evaluation indicators between different risk groups were compared. We found that both the stromal score and immune score in the high-risk group were higher than those in the low-risk group (Figure 6C). Previous studies have shown that the immune microenvironment has a significant impact on various aspects of tumor occurrence and development, treatment response, and the identification of new therapeutic targets (19,20). Furthermore, our study showed that as the risk score increased, the abundance of immune infiltration also increased. We used the ssGSEA algorithm to demonstrate the enrichment levels of different immune cells in the tumor microenvironment of high- and low-risk groups in order to understand the mechanisms of tumor immune escape and identify potential targets for immunotherapy. We found that compared to the low-risk group, the high-risk group had higher immune cell infiltration and more immune-related functions and pathways (Figure 6D,6E). Next, we evaluated the expression differences of immune checkpoints between the high- and low-risk groups (Figure 6F). The results showed that there were differences in the expression of 35 immune checkpoints between the two risk subgroups, including TNFRSF4, CTLA4, and CD200.
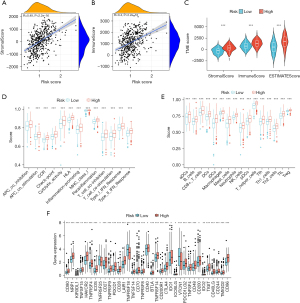
Quality control and filtering of scRNA-seq data
First, we filtered out the unqualified cells and used the filtered cells for subsequent analysis (Figure 7A). We performed PCA on the four single-cell samples and found that the four NSCLC samples had a high degree of aggregation (Figure 7B), which suggested that the transcriptional differences between these four samples were relatively small. We then conducted a correlation analysis of several quality control indicators and found that the proportion of mitochondria and hemoglobin did not increase with the increase of RNA molecules, while the number of transcriptional genes increased with the increase of RNA molecules (Figure 7C), indicating that our quality control was relatively good and removed low-quality cells. Subsequently, we used differential analysis to screen out 3,000 highly variable genes (Figure 7D).
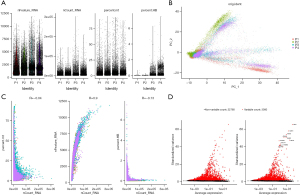
Identification and localization of LUSC cell subtypes
We used the UMAP algorithm to divide the core cells into 16 independent cell clusters (Figure 8A). In order to explore the heterogeneity within the tumor, we calculated the proportion of each cell in the four samples separately (Figure 8B,8C). The results showed that the proportions of different cells were different in each sample, indicating the presence of different cell subpopulations within the tumor, which is consistent with the characteristics of tumor heterogeneity. We identified marker genes using the “singleR” package in R, the CellMarker database, and other references (21) and then annotated the different clusters (Figure 8D). Subsequently, the upregulated, significantly upregulated, downregulated, and significantly downregulated genes in the marker genes of the 16 clusters were displayed (Figure 9A). Similarly, we visualized the marker genes of the annotated 8 cell types (Figure 9B) to better characterize the differences in their functions and characteristics. We localized four apoptosis-related prognostic genes associated with LUSC within individual cell subtypes to understand the biological characteristics and functions of these cell subtypes. Furthermore, this localization could provide clues for further research, such as that exploring the interactions and regulatory mechanisms of these prognostic genes in different cell subtypes and their relationships with other BPs. This localization of cell subtypes can provide insights into the mechanisms of LUSC occurrence and development. We observed the distribution of several apoptosis-related prognostic genes in these 8 cell clusters (Figure 9C), and it was found that BMP2 was significantly upregulated in epithelial cells, GPX3 was expressed at a higher level in smooth muscle cells, and JUN was expressed in all cell subtypes, but relatively higher in T cells. To further understand the specific expression of prognostic genes in the 8 cell clusters, we visualized the gene expression by drawing a heatmap (Figure 9D).
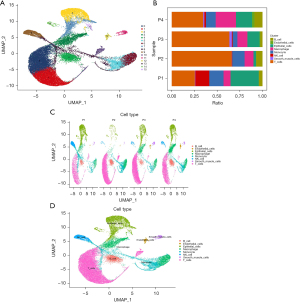
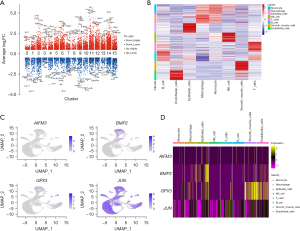
Identification of lung cancer subpopulations via single-cell trajectory analysis
Temporal analysis aims to model the time information of single cells. It can reveal the temporal trends and expression profile differences of different cells and identify biological issues such as cell development, differentiation, and function, which can aid in gaining a deeper understanding of single-cell data and discovering new biological knowledge and biomarkers (22,23). We selected two clusters for temporal analysis, and the trajectory showed the transcriptional states of cell development in the two clusters (Figure 10A). We also visualized the trajectory of the state of cluster cells (Figure 10B) and determined the starting point of cell differentiation (Figure 10C), revealing changes related to tumor progression. Next, we studied the expression changes of four prognostic genes in the temporal analysis (Figure 10D). JUN expression was significantly decreased with cancer progression, while that of AIFM3, BMP2, and GPX3 did not show significant changes. As revealed by previous dimensionality reduction clustering, T cells account for a large proportion in the sample, so we examined the expression changes of the four prognostic genes in the temporal analysis of T cells (Figure 10E).
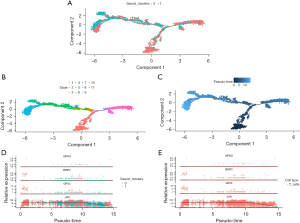
Discussion
LUSC is a histological subtype of NSCLC and is associated with poor prognosis and a high mortality rate mainly due to ineffective, highly challenging treatment (24). Despite a variety of therapies being available for LUSC, including chemotherapy, radiotherapy, and targeted therapy, the prognosis for patients with LUSC remains unsatisfactory (25). Therefore, there is an urgent need to develop new molecular therapeutic targets and prognostic models for the diagnosis and treatment of LUSC. Previous study has shown that apoptosis plays a crucial role in maintaining the balance between cell death and division, and evading apoptosis can lead to uncontrolled cell proliferation, resulting in various diseases such as cancer (26). The mechanism of apoptosis is complex and involves many pathways. Any defect in any of these pathways can lead to malignant transformation of the affected cells, tumor metastasis, and resistance to anticancer drugs. Currently, many new therapeutic strategies targeting apoptosis may be applied (27,28). Although there is a connection between apoptosis and LUSC, no systematic study has been conducted that uses apoptosis-related features as prognostic indicators to predict the prognosis of patients with LUSC.
We thus conducted a systematic analysis based on TCGA-LUSC dataset and ARGs to identify differentially expressed ARGs in LUSC and nontumor tissues. Through multifactor and LASSO Cox regression analysis, we screened for prognostic-related genes and established a risk model to predict the prognosis of LUSC.
Bone morphogenetic proteins (BMPs) are multifunctional cytokines, belonging to members of the transforming growth factor-β superfamily (29). BMP scan trigger the occurrence and progression of tumors through the signaling mediators of ligands and receptors. Meanwhile, BMPs can promote cell differentiation, including via inhibiting the process of epithelial-mesenchymal transition, which can prevent the malignant progression of cancer in the later stages (30). BMP can activate extracellular signal-regulated kinase (ERK), phosphoinositide 3-kinase (PI3K), protein kinase A (PKA), protein kinase C (PKC), and protein kinase D (PKD). Through the initiation of these pathways, BMP can exert its effects on cell survival, apoptosis, migration, and differentiation (31). Sma and Mad-related proteins (SMAD) is a classical pathway mediated by BMP2. A study has shown that BMP2 is highly overexpressed in human NSCLC and is associated with tumor grading and metastasis (32). Through mouse models, BMP2 has been shown to promote lung cancer metastasis. Depletion of BMP2 or its receptor BMPR2 greatly reduces cell migration and invasiveness, and BMP2/BMPR2-mediated cell migration involves the activation of the SMAD1/5/8 signaling pathway. Depletion of SMAD1/5/8 significantly reduces cell migration by inhibiting SMAD1/5/8 (33). Epigenetic silencing of glutathione peroxidase 3 (GPX3), a member of the important antioxidant selenoprotein family (34), maintains genome integrity by inactivating reactive oxygen species (ROS) (35), which are believed to play various roles in cancer development. For example, when the gene expression of important molecules that control cell proliferation, apoptosis, or the cell cycle is abnormal, oxidative stress can lead to persistent DNA damage and may induce cancer (36). Research has shown that GPX3 is associated with ovarian cancer metastasis and cancer progression. In one study, stable OVCAR3 GPX3 knockdown cell line was constructed using lentiviral short hairpin RNA (shRNA), and it was found that reducing GPX3 expression inhibited colony formation and anchorage-independent cell survival (37). In a study on thyroid cancer, methylation-specific polymerase chain reaction (MSP), IHC staining, Transwell experiments, and small interfering RNA (siRNA) knockdown were used to investigate the role of GPX3. It was found that GPX3 inhibits thyroid cancer metastasis by suppressing the Wnt/β-catenin signaling pathway. Silencing GPX3 expression promotes human thyroid cancer metastasis (38). c-Jun has been found to be an oncogenic transcription factor in most cancers, and its overexpression plays an important role in various biological functions such as cell apoptosis, proliferation, invasion, and migration (39,40). One study reported that enforced expression of c-Jun increased anchorage-independent growth of human bronchial epithelial cell lines, and constitutive expression of a significant c-Jun-negative mutant suppressed anchorage-independent but not anchorage-dependent growth of lung cancer cell lines (41). The activity of c-Jun is regulated by post-translational modifications, which are mainly controlled by components of the mitogen-activated protein kinase (MAPK) family of kinases, including c-Jun N-terminal kinase (JNK), ERK, and p38 kinase (42). The significant and unique function of JNK is as an activator of c-Jun. Overexpression or activation of c-Jun has been shown to have antiapoptotic effects in various cancer cell lines, and its targeting may sensitize drug-resistant cancer cells to DNA-damaging agents (43). Therefore, these key apoptotic prognostic-related genes are closely associated with LUSC and its prognosis, demonstrating the validity of our establishment of a prognostic model based on apoptotic prognostic-related genes.
After modeling, we conducted validation by dividing patients with LUSC into different risk groups based on the median risk score. Patients in the high-risk group had significantly worse prognosis. The results of ROC curve analysis showed that the prognostic model had good predictive performance. Furthermore, through analysis of the risk score of the model and other clinical features, the risk score of the model was found to be an independent prognostic indicator, and C-index analysis showed that the risk score had better predictive value than did other traditional clinical parameters. Therefore, the high predictive value of the model was again confirmed.
In addition, we conducted functional enrichment analysis on the apoptotic differentially expressed genes that we screened, identifying the key pathways through which they exert their function. According to GO and KEGG pathway analyses, we found that in cancer, cell apoptosis is mediated by changes in the mitochondrial membrane, which is a multifactorial process involving the BCL-2 family proteins, cysteine proteases, and large molecular complexes. A study has shown that in the preinitiation stage of mitochondria, different proapoptotic signal transduction or damage pathways are activated. When these signals or pathways converge on the mitochondria, the permeability of the inner and outer membranes increases, leading to the execution stage of the apoptotic process (44). The regulation of apoptotic mitochondrial events is achieved through the BCL-2 family of proteins. The BCL-2 family consists of more than 30 proteins and belongs to the BCL-2 superfamily, which includes antiapoptotic proteins, proapoptotic proteins, and homology domain 3 (BH3)-only proteins (45). In the presence of apoptotic stimuli, the expression of BH3 proteins increases, competitively binding to the antiapoptotic protein BCL-2 to release BAX/BAK from inhibition. Free BAX and BAK form oligomers, causing cytochrome C to form a channel through the outer membrane of the mitochondria, releasing it from the intermembrane space of the mitochondria into the cytoplasm. The released cytochrome C activates the caspase cascade reaction to induce cell apoptosis (46,47). Therefore, mitochondria can be considered to be the main integrator of the death pathway. Apoptosis depends on the activation of the above variety of signaling pathways, and these pathways are often dysregulated in cancer, providing a direction for further research on the treatment of apoptosis in LUSC.
Furthermore, in our multifaceted study of the immune microenvironment, we found significant differences in TMB and TP53 between the high- and low-risk groups. Previous research (48) has shown that TMB in blood can be used to evaluate the efficacy of camrelizumab in combination with chemotherapy in patients with advanced LUSC. During treatment, blood TMB levels are positively correlated with treatment efficacy, indicating that higher TMB leads to better treatment efficacy and longer OS and progression-free survival (PFS) for patients. These findings are consistent with the results of our study, in which we found differences in gene mutations between the high- and low-risk groups, with the most common type of gene mutation, that of TP53, showing significant differences in mutation type between the high- and low-risk groups. Previous study has shown that TP53 is one of the most common mutated genes in lung cancer, and its mutation is closely related to the occurrence and development of lung cancer. The type of TP53 mutation is related to prognosis, and patients with nonsense mutations have a poorer prognosis (49). This is consistent with our research, as patients in the high-risk group had a poorer prognosis.
Although a previous study has investigated the relationship between apoptosis and cancer, there is sparse research concerning its relationship with tumor immunity (50). Stromal cells and immune cells are the main elements of the tumor microenvironment, and an important aspect of our research lies in exploring the correlation between the risk model of patients with LUSC and tumor immunity. We used the ESTIMATE algorithm to calculate these scores and found that the high-risk group had higher immune and stromal scores. This indicates that changes in immune status can also affect the process of cell apoptosis. We found that natural killer (NK) cells and macrophages, which are inherently linked with cell apoptosis, were highly expressed in the high-risk group. A study has shown that the mitochondrial apoptosis (mtApoptosis) pathway is crucial for efficient NK killing, and NK cells can preactivate cancer cells for mtApoptosis. Preactivated NK cells bind BH3 mimetics to NK cells, synergistically killing cancer cells in vitro and inhibiting tumor growth in vivo (51). This study suggests that mtApoptosis can enhance NK-based immunotherapy. Interestingly, research has found that macrophages are a heterogeneous group of cells in the innate immune system that are crucial for the initiation, progression, and resolution of inflammation. They have significant functional plasticity and can respond to abnormalities and initiate programs to overcome them and restore normalcy (52,53). The cytokines and intracellular components released by apoptotic cells can activate macrophages, causing them to shift from the M1 to M2 phenotype, thereby promoting tumor growth and metastasis. In addition, apoptotic cells can also induce macrophages to produce growth factors and extracellular matrix components, promoting tumor cell proliferation and invasion. If the interaction between apoptotic cells and macrophages is not disrupted, surviving tumor cells may receive substantial support from the reaction induced by local macrophages in apoptotic tumor cells, thereby enhancing tumor recurrence (54). The consideration of these findings indeed provides valuable ideas and possible strategies for researchers to further explore the antitumor potential of cell apoptosis and tumor immunity.
Tumor heterogeneity is a significant challenge in cancer treatment, as different subpopulations of cells may exhibit varying sensitivity and resistance to therapeutic drugs (55). Therefore, understanding tumor heterogeneity may be considerably valuable for developing personalized treatment plans and predicting treatment outcomes. Compared to traditional “bulk” RNA-seq methods, which average potential differences in cell-specific transcriptomes, scRNA-seq analyzes the gene expression patterns of each individual cell, providing a clear insight into the entire tumor ecosystem, such as the mechanisms of intra- and intertumoral heterogeneity (56). Through scRNA-seq analysis, this study ultimately divided cells into 8 subgroups. To further understand the expression patterns and functions of apoptosis-related prognostic genes in different cell subgroups, we compared the expression of prognostic genes in different cell subgroups to determine their functions in these subgroups. We found that BMP2 was highly expressed in epithelial cells and may thus exert critical functions in these cells. In a study on breast cancer, elevated levels of BMP2 led to excessive activation of the BMPR1B signaling pathway, which is the receptor for BMP2. When BMP2 binds to BMPR1B, it activates the BMPR1B signaling pathway, promoting the transformation of epithelial cells. These transformed epithelial cells have increased proliferation and invasiveness, thereby promoting tumor development (57). Similarly, in a study on lung injury, BMP2 was found to activate the BMP signaling pathway, leading to increased BMP activity and ultimately resulting in the transformation of epithelial cells and the disruption of epithelial barrier function. This study successfully inhibited the increase in BMP activity and the disruption of epithelial barrier function by suppressing the expression of BMP2, thereby avoiding lung injury (58). This provides a good explanation of the role of prognostic genes in tumor development at the cellular level.
Different subpopulations of cells exist within tumors and have different gene expression profiles, which results in heterogeneity within the tumor. Sequencing multiple tumor regions can reveal the evolutionary pattern of the tumor, with tumor cells having different gene mutations and expression profiles at different time points and locations, forming a branched evolutionary tree structure (59). Pseudotime analysis is a method for inferring trajectories from scRNA-seq data. It sorts cells along a trajectory based on the similarity of their expression patterns and determines the lineage structure by identifying branching events (60). In this study, we determined trajectories with different differentiation states based on the scRNA-seq data of NSCLC and located the selected prognostic genes on the cell differentiation trajectories. We observed the changes of prognostic genes in different clusters and the same cell types. Combining pseudotemporal analysis and the localization of cell types in different clusters over time, we were able to intuitively witness the evolution of cells in NSCLC. This provides new insights into the mechanisms and driving factors of tumor evolution.
Our study represents the inaugural attempt to construct a prognostic model based on differentially expressed ARGs to predict the survival rates of LUSC patients. This model holds the potential to introduce novel molecular markers for personalized treatment in LUSC, thereby enhancing treatment efficacy. Our research employs a variety of statistical methods to ensure the stability and reliability of the model. Furthermore, through correlation analysis of prognostic ARGs, we have augmented the biological interpretability of the model. The integrated application of these methods enhances the potential value of our prognostic model in clinical applications.
Although the prognostic model established in this study demonstrated good predictive performance, was proven to be a positive prognostic indicator for patients with LUSC, and provided more accurate information for the treatment and prognostic evaluation of patients with LUSC by incorporating scRNA-seq data, there are still some limitations that need to be considered. The analysis conducted in this study was based on retrospective data from the GEO and TCGA databases, and due to the scarcity of scRNA-seq data in the GEO database, we could not obtain complete single-cell sequencing results for LUSC samples. Our results need to be further functionally validated. Despite these limitations, our findings can serve as a valuable concept-validation study, providing biomarkers and targets for future research and serving as a meaningful reference for the personalized treatment of patients with LUSC.
Conclusions
In summary, based on bioinformatics analysis, this study identified apoptosis-related genes that are associated with prognosis in LUSC, and constructed a risk model based on these prognostic genes that can accurately predict the prognosis of LUSC. Through functional enrichment analysis, we explored the role of mitochondrial membrane changes in mediating apoptosis in LUSC, involving various factors such as BCL-2 family proteins, cysteine proteases, and macromolecular complexes. Apoptosis depends on the activation of different signaling pathways, but these pathways are often dysregulated in LUSC. We also studied the differences in the immune microenvironment between high- and low-risk groups, and found that patients with high TMB had better prognosis than those with low TMB, consistent with previous study showing a positive correlation between TMB and the efficacy and survival of immunotherapy. Finally, single-cell sequencing analysis provided insights into the cellular mechanisms of tumor development, revealing the important biological function of BMP2 in epithelial cells, which, when overexpressed, can lead to the excessive activation of the BMPR1B signaling pathway, promoting the transformation of epithelial cells and increasing the risk of tumor development. Our study provides guidance for the diagnosis and treatment of LUSC patients.
Acknowledgments
Funding: None.
Footnote
Reporting Checklist: The authors have completed the TRIPOD reporting checklist. Available at https://jtd.amegroups.com/article/view/10.21037/jtd-23-1712/rc
Peer Review File: Available at https://jtd.amegroups.com/article/view/10.21037/jtd-23-1712/prf
Conflicts of Interest: All authors have completed the ICMJE uniform disclosure form (available at https://jtd.amegroups.com/article/view/10.21037/jtd-23-1712/coif). The authors have no conflicts of interest to declare.
Ethical Statement: The authors are accountable for all aspects of the work in ensuring that questions related to the accuracy or integrity of any part of the work are appropriately investigated and resolved. The study was conducted in accordance with the Declaration of Helsinki (as revised in 2013).
Open Access Statement: This is an Open Access article distributed in accordance with the Creative Commons Attribution-NonCommercial-NoDerivs 4.0 International License (CC BY-NC-ND 4.0), which permits the non-commercial replication and distribution of the article with the strict proviso that no changes or edits are made and the original work is properly cited (including links to both the formal publication through the relevant DOI and the license). See: https://creativecommons.org/licenses/by-nc-nd/4.0/.
References
- Siegel RL, Miller KD, Fuchs HE, et al. Cancer statistics, 2022. CA Cancer J Clin 2022;72:7-33. [Crossref] [PubMed]
- Bray F, Ferlay J, Soerjomataram I, et al. Global cancer statistics 2018: GLOBOCAN estimates of incidence and mortality worldwide for 36 cancers in 185 countries. CA Cancer J Clin 2018;68:394-424. [Crossref] [PubMed]
- Youlden DR, Cramb SM, Baade PD. The International Epidemiology of Lung Cancer: geographical distribution and secular trends. J Thorac Oncol 2008;3:819-31. [Crossref] [PubMed]
- Socinski MA, Obasaju C, Gandara D, et al. Current and Emergent Therapy Options for Advanced Squamous Cell Lung Cancer. J Thorac Oncol 2018;13:165-83. [Crossref] [PubMed]
- Scagliotti G, Hanna N, Fossella F, et al. The differential efficacy of pemetrexed according to NSCLC histology: a review of two Phase III studies. Oncologist 2009;14:253-63. [Crossref] [PubMed]
- Zinner RG, Novello S, Peng G, et al. Comparison of patient outcomes according to histology among pemetrexed-treated patients with stage IIIB/IV non-small-cell lung cancer in two phase II trials. Clin Lung Cancer 2010;11:126-31. [Crossref] [PubMed]
- Woodard GA, Jones KD, Jablons DM. Lung Cancer Staging and Prognosis. Cancer Treat Res 2016;170:47-75. [Crossref] [PubMed]
- Wang M, Herbst RS, Boshoff C. Toward personalized treatment approaches for non-small-cell lung cancer. Nat Med 2021;27:1345-56. [Crossref] [PubMed]
- Adib E, Nassar AH, Abou Alaiwi S, et al. Variation in targetable genomic alterations in non-small cell lung cancer by genetic ancestry, sex, smoking history, and histology. Genome Med 2022;14:39. [Crossref] [PubMed]
- Shivapurkar N, Reddy J, Chaudhary PM, et al. Apoptosis and lung cancer: a review. J Cell Biochem 2003;88:885-98. [Crossref] [PubMed]
- Hanahan D, Weinberg RA. The hallmarks of cancer. Cell 2000;100:57-70. [Crossref] [PubMed]
- Liu G, Pei F, Yang F, et al. Role of Autophagy and Apoptosis in Non-Small-Cell Lung Cancer. Int J Mol Sci 2017;18:367. [Crossref] [PubMed]
- Gandara DR, Mack PC, Li T, et al. Evolving treatment algorithms for advanced non-small-cell lung cancer: 2009 looking toward 2012. Clin Lung Cancer 2009;10:392-4. [Crossref] [PubMed]
- Pore MM, Hiltermann TJ, Kruyt FA. Targeting apoptosis pathways in lung cancer. Cancer Lett 2013;332:359-68. [Crossref] [PubMed]
- Olsen TK, Baryawno N. Introduction to Single-Cell RNA Sequencing. Curr Protoc Mol Biol 2018;122:e57. [Crossref] [PubMed]
- Lei Y, Tang R, Xu J, et al. Applications of single-cell sequencing in cancer research: progress and perspectives. J Hematol Oncol 2021;14:91. [Crossref] [PubMed]
- Rozenblatt-Rosen O, Regev A, Oberdoerffer P, et al. The Human Tumor Atlas Network: Charting Tumor Transitions across Space and Time at Single-Cell Resolution. Cell 2020;181:236-49. [Crossref] [PubMed]
- Zheng L, Li L, Xie J, et al. Six Novel Biomarkers for Diagnosis and Prognosis of Esophageal squamous cell carcinoma: validated by scRNA-seq and qPCR. J Cancer 2021;12:899-911. [Crossref] [PubMed]
- Shiravand Y, Khodadadi F, Kashani SMA, et al. Immune Checkpoint Inhibitors in Cancer Therapy. Curr Oncol 2022;29:3044-60. [Crossref] [PubMed]
- Thorsson V, Gibbs DL, Brown SD, et al. The Immune Landscape of Cancer. Immunity 2018;48:812-830.e14. [Crossref] [PubMed]
- Ma KY, Schonnesen AA, Brock A, et al. Single-cell RNA sequencing of lung adenocarcinoma reveals heterogeneity of immune response-related genes. JCI Insight 2019;4:e121387. [Crossref] [PubMed]
- La Manno G, Soldatov R, Zeisel A, et al. RNA velocity of single cells. Nature 2018;560:494-8. [Crossref] [PubMed]
- Trapnell C, Cacchiarelli D, Grimsby J, et al. The dynamics and regulators of cell fate decisions are revealed by pseudotemporal ordering of single cells. Nat Biotechnol 2014;32:381-6. [Crossref] [PubMed]
- Lau SCM, Pan Y, Velcheti V, et al. Squamous cell lung cancer: Current landscape and future therapeutic options. Cancer Cell 2022;40:1279-93. [Crossref] [PubMed]
- Perez-Moreno P, Brambilla E, Thomas R, et al. Squamous cell carcinoma of the lung: molecular subtypes and therapeutic opportunities. Clin Cancer Res 2012;18:2443-51. [Crossref] [PubMed]
- Chaudhry GE, Akim AM, Sung YY, et al. Cancer and Apoptosis. Methods Mol Biol 2022;2543:191-210. [Crossref] [PubMed]
- Jan R, Chaudhry GE. Understanding Apoptosis and Apoptotic Pathways Targeted Cancer Therapeutics. Adv Pharm Bull 2019;9:205-18. [Crossref] [PubMed]
- Wong RS. Apoptosis in cancer: from pathogenesis to treatment. J Exp Clin Cancer Res 2011;30:87. [Crossref] [PubMed]
- Wakefield LM, Hill CS. Beyond TGFβ: roles of other TGFβ superfamily members in cancer. Nat Rev Cancer 2013;13:328-41. [Crossref] [PubMed]
- Davis H, Raja E, Miyazono K, et al. Mechanisms of action of bone morphogenetic proteins in cancer. Cytokine Growth Factor Rev 2016;27:81-92. [Crossref] [PubMed]
- Bragdon B, Moseychuk O, Saldanha S, et al. Bone morphogenetic proteins: a critical review. Cell Signal 2011;23:609-20. [Crossref] [PubMed]
- Huang F, Cao Y, Wu G, et al. BMP2 signalling activation enhances bone metastases of non-small cell lung cancer. J Cell Mol Med 2020;24:10768-84. [Crossref] [PubMed]
- Wu CK, Wei MT, Wu HC, et al. BMP2 promotes lung adenocarcinoma metastasis through BMP receptor 2-mediated SMAD1/5 activation. Sci Rep 2022;12:16310. [Crossref] [PubMed]
- Chang C, Worley BL, Phaëton R, et al. Extracellular Glutathione Peroxidase GPx3 and Its Role in Cancer. Cancers (Basel) 2020;12:2197. [Crossref] [PubMed]
- Chen B, Rao X, House MG, et al. GPx3 promoter hypermethylation is a frequent event in human cancer and is associated with tumorigenesis and chemotherapy response. Cancer Lett 2011;309:37-45. [Crossref] [PubMed]
- Ushio-Fukai M. Compartmentalization of redox signaling through NADPH oxidase-derived ROS. Antioxid Redox Signal 2009;11:1289-99. [Crossref] [PubMed]
- Worley BL, Kim YS, Mardini J, et al. GPx3 supports ovarian cancer progression by manipulating the extracellular redox environment. Redox Biol 2019;25:101051. [Crossref] [PubMed]
- Zhao H, Li J, Li X, et al. Silencing GPX3 Expression Promotes Tumor Metastasis in Human Thyroid Cancer. Curr Protein Pept Sci 2015;16:316-21. [Crossref] [PubMed]
- Katiyar S, Jiao X, Wagner E, et al. Somatic excision demonstrates that c-Jun induces cellular migration and invasion through induction of stem cell factor. Mol Cell Biol 2007;27:1356-69. [Crossref] [PubMed]
- Zhao Y, Luo A, Li S, et al. Inhibitor of Differentiation/DNA Binding 1 (ID1) Inhibits Etoposide-induced Apoptosis in a c-Jun/c-Fos-dependent Manner. J Biol Chem 2016;291:6831-42. [Crossref] [PubMed]
- Maeno K, Masuda A, Yanagisawa K, et al. Altered regulation of c-jun and its involvement in anchorage-independent growth of human lung cancers. Oncogene 2006;25:271-7. [Crossref] [PubMed]
- Lopez-Bergami P, Lau E, Ronai Z. Emerging roles of ATF2 and the dynamic AP1 network in cancer. Nat Rev Cancer 2010;10:65-76. [Crossref] [PubMed]
- Vasilevskaya I, O'Dwyer PJ. Role of Jun and Jun kinase in resistance of cancer cells to therapy. Drug Resist Updat 2003;6:147-56. [Crossref] [PubMed]
- Jacotot E, Costantini P, Laboureau E, et al. Mitochondrial membrane permeabilization during the apoptotic process. Ann N Y Acad Sci 1999;887:18-30. [Crossref] [PubMed]
- Cory S, Adams JM. The Bcl2 family: regulators of the cellular life-or-death switch. Nat Rev Cancer 2002;2:647-56.
- Zhao H. Extrinsic and Intrinsic Apoptosis Signal Pathway Review. Apoptosis and Medicine 2012. doi:
10.5772/50129 . - Xiong S, Mu T, Wang G, et al. Mitochondria-mediated apoptosis in mammals. Protein Cell 2014;5:737-49. [Crossref] [PubMed]
- Jiang T, Chen J, Xu X, et al. On-treatment blood TMB as predictors for camrelizumab plus chemotherapy in advanced lung squamous cell carcinoma: biomarker analysis of a phase III trial. Mol Cancer 2022;21:4. [Crossref] [PubMed]
- Fan Z, Zhang Q, Feng L, et al. Genomic landscape and prognosis of patients with TP53-mutated non-small cell lung cancer. Ann Transl Med 2022;10:188. [Crossref] [PubMed]
- Messmer MN, Snyder AG, Oberst A. Comparing the effects of different cell death programs in tumor progression and immunotherapy. Cell Death Differ 2019;26:115-29. [Crossref] [PubMed]
- Pan R, Ryan J, Pan D, et al. Augmenting NK cell-based immunotherapy by targeting mitochondrial apoptosis. Cell 2022;185:1521-1538.e18. [Crossref] [PubMed]
- Lavin Y, Merad M. Macrophages: gatekeepers of tissue integrity. Cancer Immunol Res 2013;1:201-9. [Crossref] [PubMed]
- Wynn TA, Chawla A, Pollard JW. Macrophage biology in development, homeostasis and disease. Nature 2013;496:445-55. [Crossref] [PubMed]
- Weigert A, Mora J, Sekar D, et al. Killing Is Not Enough: How Apoptosis Hijacks Tumor-Associated Macrophages to Promote Cancer Progression. Adv Exp Med Biol 2016;930:205-39. [Crossref] [PubMed]
- Dagogo-Jack I, Shaw AT. Tumour heterogeneity and resistance to cancer therapies. Nat Rev Clin Oncol 2018;15:81-94. [Crossref] [PubMed]
- Baslan T, Hicks J. Unravelling biology and shifting paradigms in cancer with single-cell sequencing. Nat Rev Cancer 2017;17:557-69. [Crossref] [PubMed]
- Chapellier M, Bachelard-Cascales E, Schmidt X, et al. Disequilibrium of BMP2 levels in the breast stem cell niche launches epithelial transformation by overamplifying BMPR1B cell response. Stem Cell Reports 2015;4:239-54. [Crossref] [PubMed]
- Helbing T, Herold EM, Hornstein A, et al. Inhibition of BMP activity protects epithelial barrier function in lung injury. J Pathol 2013;231:105-16. [Crossref] [PubMed]
- Gerlinger M, Rowan AJ, Horswell S, et al. Intratumor heterogeneity and branched evolution revealed by multiregion sequencing. N Engl J Med 2012;366:883-92. [Crossref] [PubMed]
- Wu F, Fan J, He Y, et al. Single-cell profiling of tumor heterogeneity and the microenvironment in advanced non-small cell lung cancer. Nat Commun 2021;12:2540. [Crossref] [PubMed]