Mechanistic analysis of Th2-type inflammatory factors in asthma
Highlight box
Key findings
• Our study revealed that S100A14, KRT6A, S100A2, ABCA13, UBE2C, RASSF10, PSCA, PLAT, and TIMP1 may be the key genes (molecular markers) for airway remodeling. The epithelial-mesenchymal transition-related genes GEM, TPM4, SLC6A8, and SNTB1 may be involved in asthma-induced airway remodeling. IL6 may affect tracheal remodeling by binding to UBE2C protein or by regulating the GEM gene, respectively. IL6 and IL9 may influence the occurrence of tracheal remodeling by regulating the downstream Toll-like receptor (TLR) signaling pathway.
What is known and what is new?
• Extensive chronic airway inflammation and restricted ventilation caused by airway remodeling have long been considered the main pathological features of asthma. Previous literature had reported that T helper type 2-related inflammatory factors, including IL4, IL5, IL1, IL8, and IL25, are involved in airway remodeling, mediating epithelial fibrosis and mucogenesis, and inflammatory infiltration of eosinophils and macrophages; however, the exact mechanism of action remains unelucidated.
• Our study shows that TLR signaling pathway plays an important role in the occurrence of asthma. Therefore, the study of TLR signaling pathway is a new direction for the prevention and treatment of asthma.
What is the implication, and what should change now?
• This study identified key genes and important pathways that affect tracheal remodeling in patients with asthma, providing a new idea for revealing the mechanism of tracheal remodeling induced by asthma and seeking new therapeutic targets.
Introduction
Asthma is a heterogeneous disease characterized by chronic inflammation of the airways and has a wide global impact, with about 300 million asthma patients worldwide (1). There are about 30 million asthma patients in China, and the number of asthma patients has been increasing year by year in recent years due to the increasing environmental problems (2). As a chronic disease of the respiratory system, asthma has a long duration and severe symptoms, which can have a serious impact on the quality of life of patients. If not properly treated, it may be fatal. Asthma ranks 16th among the leading causes of human disability and 28th among the leading causes of economic burden of disease, which not only seriously affects the quality of life of patients but also expends significant medical resources and money. Thus, it is crucial to investigate the pathogenesis of asthma and find effective means of prevention and treatment. The pathogenesis is currently thought to include airway hyperresponsiveness, airway remodeling, and airway inflammation (3,4), however, the exact pathogenesis of asthma is still unclear (5,6).
Widespread chronic inflammation of the airways and airway remodeling leading to airway obstruction have been considered the main pathological features of asthma (7,8). Repeated damage and repair due to chronic inflammation of the airways was originally thought to be the direct cause of airway remodeling. Airway remodeling comprises a combination of multifaceted physiological changes characterized by epithelial detachment, subepithelial fibrosis, smooth muscle hypertrophy, cupped cell proliferation, and angiogenesis, and the cytokines, growth factors, inflammatory mediators, and chemokines released from these cells play an important role in airway reconstruction (9). The signaling pathways leading to this series of changes are also exceptionally complex, and it is now generally accepted that the chronic inflammatory response of the airway due to an imbalance in the immune system is the main initiating factor for this series of changes. Previous literature has reported T helper type 2 (Th2)-associated inflammatory factors including IL4, IL5, IL1, IL8, and IL25 are involved in airway remodeling, mediating epithelial fibrosis, mucus chemotaxis, and inflammatory infiltration of eosinophils and macrophages (10); however, the exact mechanism of action remains elusive.
The primary objective of this study is to gain a deeper understanding of the potential molecular mechanisms of Th2 type airway inflammation and airway remodeling in asthma. To achieve this goal, we will focus on studying Th2 type inflammation-related factors, leveraging bioinformatics techniques and big data mining to delve into their roles in the mechanisms of airway remodeling. By choosing mice exposed to chlorine gas as the experimental model, we aim to further uncover the intricate details of airway remodeling in the pathology of asthma. Our research is anticipated to provide new molecular targets for the prevention and treatment of asthma. We present this article in accordance with the TRIPOD reporting checklist (available at https://jtd.amegroups.com/article/view/10.21037/jtd-23-1628/rc).
Methods
Data sources
The GSE41861, GSE63142, and GSE109365 datasets were downloaded from the Gene Expression Omnibus (GEO) database. GSE63142 contains 56 bronchial epithelial samples from asthmatic patients and 27 bronchial epithelial samples from non-asthmatic patients. GSE41861 contains 91 bronchial epithelial samples from asthmatic patients and 47 bronchial epithelial samples from non-asthmatic patients. The GSE109365 dataset contains five samples of stenotic airways from mice exposed to chlorine for 4 days and five samples of normal airways from mice not exposed to chlorine. All datasets were subjected to background elimination to remove missing, low-expressed, and non-corresponding gene probes (11), with 15,171 genes annotated in the GSE41861 dataset, 19,565 genes annotated in the GSE63142 dataset, and 22,487 genes annotated in the GSE109365 dataset.
Screening of differentially expressed genes (DEGs)
Gene expression analysis was performed using the R language (The R Foundation for Statistical Computing, Vienna, Austria) on the GSE63142 and GSE109365 datasets, which contained gene expression data from the normal control (NC) group (27 cases) and the asthma group (56 cases), and the GSE109365 dataset, which contained gene expression data from the NC group (5) and the treatment group (5 cases). Analysis was performed based on the “limma” package in R software (The R Project for Statistical Computing, Vienna, Austria) to visualize DEGs between the two groups. The DEGs were visualized using the packages “pheatmap”, “ggplot2”, and “tidyverse” in R language. Pearson correlation coefficients were calculated to determine the correlation between genes and genes, and |R| >0.2 and P<0.05 were used to filter the correlation conditions.
Enrichment analysis of DEGs
Gene Ontology (GO) and Kyoto Encyclopedia of Genes and Genomes (KEGG) analyses of DEGs were performed using the org.Hs.eg.db, enrichplot, and clusterProfiler packages in R language. In the KEGG pathway analysis, enrichment pathways were identified based on hypergeometric distribution (P<0.05).
Protein-protein interaction (PPI) network construction
The Search Tool for the Retrieval of Interacting Genes/Proteins (STRING) database is an online database for detecting known or predicted protein interactions. Our screened DEGs were imported into STRING for PPI network analysis, and PPI was obtained by selecting the criterion of medium confidence >0.7.
Feature gene calculation and screening
In this study, the random forest (RF) classifier was used to rank the importance of the target genes and to obtain the genes that were relatively important for the establishment of the classification model. The number of RF decision trees (ntree) =500. The analysis process was implemented by programming in R language (12).
Predicting the proportion of different immune cell infiltrations in samples from asthma patients
The Cell-Type Identification by Estimating Relative Subsets of RNA Transcripts (CIBERSORT) (13) deconvolution algorithm was used to estimate the proportion of different immune cells in asthmatic tissues and to analyze the variability of immune cells in normal and asthmatic bronchial tissues. The CIBERSORT algorithm does not determine the immune cell content solely on the basis of single gene expression; it does so by identifying 547 immune-related genes and subsequently deconvoluting the 547 genes. The algorithm is based on the identification of 547 immune-related genes and the subsequent deconvolution of 547 genes to calculate 22 immune cell contents.
Columnar line graph model construction and validation
The R software was used to obtain the visualized column line graphs, and each variable was given a score according to its level of influence on tracheal remodeling, and then the scores of each variable were summed to obtain the total score, and finally the R software was used to calculate the predicted probability of tracheal remodeling in the patient by converting the relationship between the total score and the probability of tracheal remodeling. The higher the score, the higher the probability of tracheal remodeling.
The internal validation of the column line graph model was achieved using bootstrap self-sampling 1,000 times, and the difference was considered statistically significant at P<0.05. The model was validated in the original cohort and the validation cohort, respectively, and the validation methods included discrimination validation, graph calibration validation, and decision curve analysis (DCA) (14).
Docking prediction based on the ZDOCK server
The Research Collaboratory for Structural Bioinformatics Protein Data Bank (RCSB PDB; https://www.rcsb.org/) database was utilized to retrieve the protein structures of IL6, UBE2C, and TIMP1, which had been selected by X-ray detection for best score and resolution were selected. Thus, protein structures including 1ALU, 4YII, and 3MA2 respectively corresponding to IL6, UBE2C, and TIMP1 in PDB format were downloaded. ZDOCK server (version 3.0.2; https://zdock.umassmed.edu/) is a Fast Fourier Transform-based protein docking program created and managed by Zhiping Weng’s lab (ZLAB) at the University of Massachusetts Medical School (15). It works by searching all possible binding modes in the translational and rotational space between the two proteins and evaluating each pose using an energy-based scoring function. We input the PDB files and conducted two jobs: (I) the 1ALU-4YII pair and (II) the 1ALU-3MA2 pair, of which protein 1ALU remains stationary while others are moved. Further, the ZDOCK server helped to process the top 10 predicted docking files with best ZDOCK scores (15). We uploaded the best docking structure to the online tool Proteins, Interfaces, Structures and Assemblies (PDBePISA; https://www.ebi.ac.uk/msd-srv/prot_int/pistart.html) to visualize the predicted protein complexes and assess the significance of their interfaces. The disulfide bonds, covalent bonds, salt bridges bonds, and hydrogen bonding were detected.
Ethical statement
The study was conducted in accordance with the Declaration of Helsinki (as revised in 2013).
Statistical analysis
R software version 3.6.1 was used for statistical analysis. Count data were expressed as number of cases or percentages using the χ2 test, and Fisher’s exact probability method was used when necessary. Quantitative data were expressed as mean ± standard deviation (SD) if they met normal distribution, and t-test was used for comparison between groups. Spearman correlation analysis was used to evaluate the strength of association between risk factor indicators and the scoring system. The visualized line graphs of the regression model were plotted, and the concordance index (C-index) was calculated to evaluate the effectiveness of the application of the line graphs. Calibration curves were used to analyze the differences between the line graphs and the ideal model. The difference was considered statistically significant at P<0.05.
Results
Identification and enrichment analysis of DEGs
DEGs were analyzed by the “limma” package in R language software for 56 asthmatic and 27 non-asthmatic bronchial epithelial samples in the GSE63142 dataset. A total of 182 DEGs [|log2fold change (FC)| >0.5 and P<0.05] were screened, of which 97 were up-regulated and 85 were down-regulated (Figure 1A).
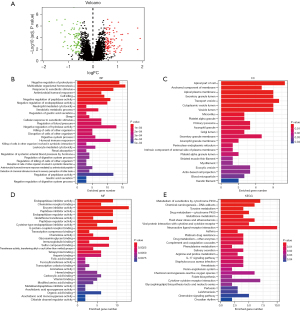
Subsequently, KEGG and GO analysis of these 182 DEGs revealed that they are mainly involved in multicellular organismal homeostasis, negative regulation of proteolysis, apical part of cell transport vesicle, G protein-coupled receptor binding, neuroactive ligand-receptor interaction, cytokine-cytokine receptor interaction, and chemokine signaling pathway (Figure 1B-1E).
Screening of tracheal remodeling-related genes
Similarly, DEGs were analyzed by the “limma” package in the R language software for five mouse stenotic trachea samples exposed to chlorine gas for 4 days and five mouse normal trachea samples not exposed to chlorine gas in the GSE109365 dataset. A total of 381 DEGs (|log2FC| >1.5 and P<0.05) were screened, of which 210 were up-regulated and 171 were down-regulated (Figure 2A).
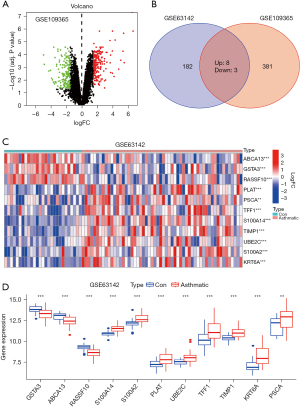
Subsequently, the DEGs in the GSE109365 dataset were intersected using the DEGs in the GSE63142 dataset, with eight up-regulated (UBE2C, TFF1, TIMP1, KRT6A, PSCA, S100A14, S100A2, and PLAT) and 3 down-regulated (GSTA3, ABCA13, and RASSF10) (Figure 2B). The expression of these 11 genes in the GSE109365 dataset was further evaluated (Figure 2C,2D). Based on the above, our study reveals that these are potential candidate targets for further research. However, we acknowledge that further functional studies are required to determine their exact roles in airway remodeling.
Screening of key genes for tracheal remodeling
To further obtain key genes for tracheal remodeling with clinical translational significance, we performed the calculation of signature genes using machine algorithms. First, this study compared the advantages and disadvantages of the support vector machine (SVM) algorithm and RF algorithm, and it was found that the RF algorithm has better accuracy (Figure 3A-3C). Therefore, the RF algorithm was chosen for the calculation of feature genes in this study. The RF algorithm (16) was used for feature gene calculation for 11 DEGs, and 11 genes (S100A14, KRT6A, S100A2, ABCA13, UBE2C, RASSF10, PSCA, PLAT, TIMP1, GSTA3 and TFF1) were selected as candidate feature genes (Figure 3D,3E).
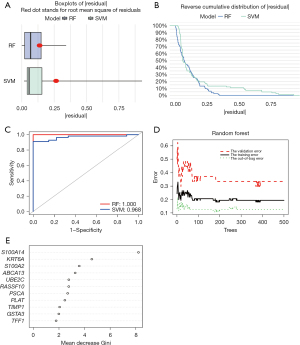
Next, we used S100A14, KRT6A, S100A2, ABCA13, UBE2C, RASSF10, PSCA, PLAT, and TIMP1 to construct the prediction model of tracheal remodeling, and R software was used to visualize the model to obtain the nomogram. The nomogram showed that S100A14, KRT6A, S100A2, ABCA13, UBE2C, RASSF10, PSCA, and PLAT had high score weight, whereas IMP had the lowest predicted score. When the total score reached 180, the risk of tracheal remodeling was indicated, and when the total score reached 280, the probability of tracheal remodeling was as high as 90% (Figure 4A).
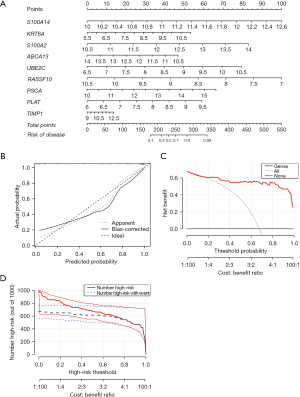
In the next step, the calibration curve was used to evaluate the calibration degree of the nomogram model; “value” indicated the original curve, “ideal” indicated the ideal standard curve, and “bias-corrected” indicated the calibration curve. The results showed that the calibration curve was close to the ideal standard curve in both the original and validation cohorts. This indicated that the nomogram of this study has good calibration ability (Figure 4B).
A total of nine genes were taken as state variables, and the predicted risk values obtained from nomogram were taken as test variables. The “rmda” program package was used to draw the clinical DCA curve of the nomogram model. According to the DCA curve, the nomogram model had high accuracy and good clinical applicability (Figure 4C). Further analysis of the clinical impact curve also suggested that it had good clinical application value (Figure 4D).
Correlation analysis of Th2-type airway inflammatory factors and key genes of tracheal remodeling
We downloaded 10 Th2-type inflammation-related factors from the Molecular Signatures Database (MSigDB; https://www.gsea-msigdb.org/gsea/msigdb/) (Table S1), namely IL1, IL4, IL5, IL6, IL9, IL10, IL13, IL25, IL33, and TSLP. The GSE63142 dataset was used to compare the differential expression of these ten airway inflammatory factors in bronchial epithelial samples from asthmatic patients and non-asthmatic patients. In comparison with non-asthmatic patients, two DEGs were screened, IL6 was down-regulated in bronchial epithelial samples from asthmatic patients, whereas IL9 was up-regulated in bronchial epithelial samples from asthmatic patients (Figure 5A).
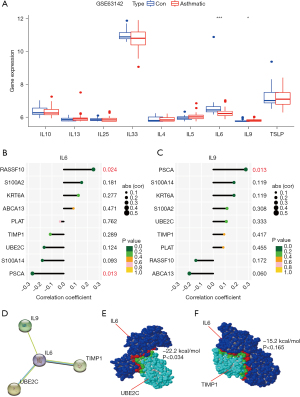
Next, we analyzed the relationship of IL6 and IL9 genes with nine airway remodeling-related genes. The analysis showed that IL6 had mild strength of association (|R| >0.2) with RASSF10 and PSCA (Figure 5B); whereas IL9 had mild strength of association (|R| >0.2) with PSCA (Figure 5C). Based on the above, our data revealed a low correlation between IL6 and IL9 genes and these nine airway remodeling-related genes, suggesting that there may not be a regulatory relationship between them.
Next, we used the PPI website to make binding predictions for IL6, IL9, S100A14, KRT6A, S100A2, ABCA13, UBE2C, RASSF10, PSCA, PLAT, and TIMP1. It was shown that IL6 can bind to IL9, UBE2C, and TIMP1 and is located at the central node of binding (Figure 5D). Further molecular docking also suggested the possible binding of IL6 to UBE2C (Figure 5E), yet IL6 may not bind to TIMP1 (Figure 5F).
Based on the above, our findings revealed that Th2-type airway inflammatory factors may interact with UBE2C to induce airway remodeling induced by asthma.
Correlation analysis of Th2-type airway inflammatory factors IL6 and IL9 with epithelial-mesenchymal transition (EMT)-related genes
Among the series of physiological changes leading to airway remodeling, changes in the airway epithelium are initiating and central (17). EMT is a process in which epithelial cells are de-adhered and transformed into migratory mesenchymal cells. Studies have shown that the expression of c-Myc, Snail, and collagen in the tracheal epithelium also increases significantly during EMT, which leads to basement membrane thickening and abnormal proliferation of myocytes. Therefore, EMT may be involved in airway remodeling during asthma.
Based on the above, this study analyzed the differential expression of EMT genes in bronchial epithelial samples of asthmatic patients and non-asthmatic patients, and a total of 54 DEGs were screened out (Figure 6A). Due to the large number of genes, we used the machine learning algorithm to calculate the characteristic genes. By comparison, the RF algorithm had better accuracy (Figure 6B-6D). The RF algorithm (16) was used to calculate the characteristic genes of 54 DEGs, and four genes (GEM, TPM4, SLC6A8, SNTB1) were selected as candidate characteristic genes (Figure 6E,6F). Next, this study analyzed the relationship between IL6 and IL9 genes and the four EMT-related genes. It was revealed that IL6 and GEM had moderate correlations (|R| >0.6) (Figure 6G); the correlation intensity of IL9 and TPM4 was weak (|R| >0.2) (Figure 6H). Therefore, this study revealed that IL6 can regulate the occurrence of airway remodeling by regulating GEM gene and affecting EMT. Targeting EMT may become a new direction for the treatment of airway remodeling.
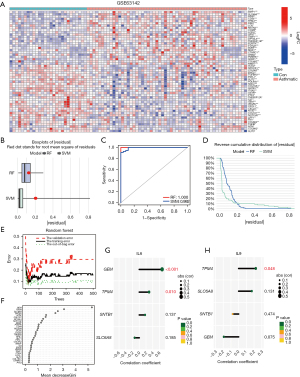
Correlation analysis of Th2-type airway inflammatory factors IL6 and IL9 with the immune microenvironment in asthmatic patients
We applied the GSE41861 dataset to compare the immune cell infiltration in bronchial tissues of asthmatic and non-asthmatic patients. Monocytes, dendritic cells resting, mast cells resting, and mast cells activated showed differences in infiltration distribution compared with epithelial tissue samples from non-asthmatic patients (Figure 7A,7B), and these results suggest that these immune cells may be involved in the immune damage.
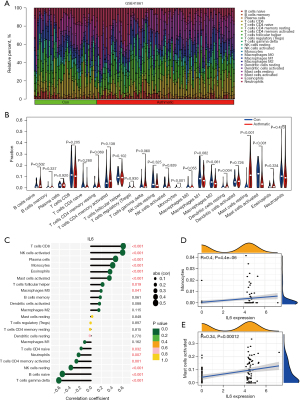
In the next step, Th2-type airway inflammatory factors IL6 and IL9 were correlated with the infiltration distribution of immune cells. The results showed that IL6 correlated significantly (|R| >0.5) with the infiltration distribution of the five immune cells (Figure 7C). Further analysis showed a mild correlation between IL6 and monocytes and mast cells activated (Figure 7D,7E). These data suggest that IL6 may influence the progression of asthma patients by regulating the infiltration distribution of monocytes and mast cells activated, respectively.
Identification and validation of regulatory mechanisms of IL6 and IL9
To confirm the downstream regulatory mechanisms of the IL6 and IL9 genes, this study performed gene set enrichment analysis (GSEA) of the IL6 and IL9 genes using the GSE63142 dataset. Through enrichment analysis, this study revealed that IL6 may regulate cytokine-cytokine receptor interaction, chemokine signaling pathway, Toll-like receptor (TLR) signaling pathway, hematopoietic cell lineage, and antigen processing and presentation affected tracheal remodeling induced by asthma (Figure 8A). IL9 may regulate cytokine-cytokine receptor interaction, the JAK-STAT signaling pathway, chemokine signaling pathway, and natural killer cell-mediated cytotoxicity and TLR signaling pathways affect tracheal remodeling induced by asthma (Figure 8B). These results suggest that the IL6 and IL9 genes may affect airway remodeling by regulating these pathways.
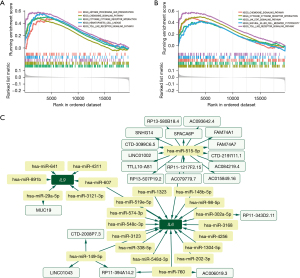
To explore the upstream regulatory mechanisms of the IL6 and IL9 genes, this study applied the TargetScan (https://www.targetscan.org/vert_80/), miRanda (https://www.microrna.org/), and miRDB (https://mirdb.org/) databases to analyze the upstream microRNAs (miRNAs) and long non-coding RNAs (lncRNAs) of IL6 and IL9. A total of 18 IL6-related miRNAs and six IL9-related miRNAs were intersected by the three databases. Next, lncRNA prediction was performed for miRNAs upstream of IL6 and IL9 using the spongeScan database (http://spongescan.rc.ufl.edu/), and a total of 22 lncRNAs were predicted. Competing endogenous RNAs (ceRNAs) network construction was demonstrated for these predicted miRNAs and lncRNAs in combination with Cytoscape software (https://cytoscape.org/) in this study, which showed that miR-515-5p was the core node gene (Figure 8C), and miR-607 was the common upstream regulator of IL6 and IL9. Based on this, we identified miR-515-5p as a core node gene and miR-607 as a common upstream regulator of IL6 and IL9. This implies that the expressions of IL6 and IL9 could be reciprocal, but further research is needed to confirm this.
In order to confirm the downstream regulatory mechanism of IL6 and IL9, GSEA of IL6 and IL9 was performed using GSE63142 dataset. Through enrichment analysis, it was revealed that IL6 may regulate cytokine-cytokine receptor interaction, chemokine signaling pathway, TLR signaling pathway, hematopoietic cell lineage, and antigen processing- and presentation-affected tracheal remodeling induced by asthma (Figure 8A). IL9 may regulate cytokine-cytokine receptor interaction, the JAK-STAT signaling pathway, chemokine signaling pathway, and natural killer cell-mediated cytotoxicity and TLR signaling pathways-affected tracheal remodeling induced by asthma (Figure 8B). These results suggest that IL6 and IL9 may affect airway remodeling by regulating these pathways.
Discussion
Bronchial asthma, as a common disease of the respiratory system, is characterized by chronic inflammation of the airways and airway remodeling as the main pathological features (18,19). Airway remodeling serves as an important pathological cause of airway narrowing and airflow limitation. Studies have shown that airway remodeling occurs in early asthma, when symptoms of airway inflammation are not evident (20). This suggests that mechanisms of airway remodeling due to non-inflammatory factors are also present during the development of asthma. Current studies on mechanisms of airway remodeling have been limited to airway inflammation (21,22), and there are relatively few studies on mechanisms of airway remodeling from non-airway inflammatory aspects (23).
In the present study, we focused on the role and mechanisms of Th2-associated inflammatory factors IL1, IL4, IL5, IL6, IL9, IL10, IL13, IL25, IL33, and TSLP in airway remodeling in bronchial tissues. In this study, we compared the messenger RNA (mRNA) expression of Th2-type inflammatory factors IL1, IL4, IL5, IL6, IL9, IL10, IL13, IL25, IL33, and TSLP in asthmatic and non-asthmatic epithelial tissues and showed that IL6 and IL9 showed differential expression in asthmatic epithelial samples, with IL6 being down-regulated and IL9 being up-regulated, suggesting that these two factors may have opposite functions. However, it is important to note that these inflammatory factors act as secreted proteins on themselves or surrounding cells, and intracellular mRNA expression may not truly reflect their protein levels; further protein-level assays may be required. Previous research has suggested that TGF-β1, IL13, and IL17 are regarded as critical regulatory factors. These factors can modulate the function of airway epithelial cells, smooth muscle cells, fibroblasts, and immune cells, and may lead to structural and functional changes in the airways (24,25). In recent years, the roles of IL6 and IL9 in airway remodeling have received increasing attention. IL6 is believed to promote airway myofibroblast and matrix synthesis through the proliferation of airway smooth muscle cells and the regulation of Th17 cell differentiation (26,27). Meanwhile, IL9 is thought to promote airway myofibroblast and matrix synthesis, as well as the survival and activation of eosinophils, thereby promoting airway myofibrosis, fibrosis, and inflammatory responses (28,29). In this study, an inverse expression of IL6 and IL9 was observed in asthma epithelial samples. This may involve complex biological molecular mechanism differences and needs further experimental validation.
Immunological theory suggests that the development of asthma is associated with an imbalance in the T helper type 1 (Th1)/Th2 factor ratio (30), and that Th cells can be divided into Th1 and Th2 cells depending on the cytokines secreted, with Th1 acting as an inducer of cellular immunity against infections caused by viruses and bacteria, and activating macrophages to cause delayed metaplasia and acting on Th2 cells to avoid over-immunization (31,32); Th1 and Th2 are in a dynamic balance to maintain the stability of the body’s defense function, and the predominance of either of them can lead to immune dysfunction (33,34).
Currently, molecular markers related to tracheal remodeling have not been reported. Therefore, in this study, we analyzed DEGs by using mouse stenosis tracheal samples and mouse normal tracheal samples, and then intersected the asthma-related DEGs to obtain 11 genes. In order to further obtain the key genes of tracheal remodeling with clinical significance, the characteristic genes of tracheal remodeling-related molecules were calculated by machine learning algorithm. This enabled us to screen for clinically transforming tracheal remodeling related molecules, including both inflamed tracheal stenosis and non-inflamed tracheal stenosis.
As the outermost cell in the trachea, the tracheal epithelium assumes a barrier role as well as a role in the presentation and amplification of immune factors in the environment, and its EMT is generally considered the initiating factor in the onset of subsequent tracheal remodeling (35,36). EMT in the tracheal epithelium not only leads to a decrease in the tight junctions of some epithelial cells, thus reducing the epithelial barrier capacity and leading to airway hyperstress, but also leads to airway subepithelial fibrosis, increased extracellular matrix (ECM) secretion and abnormal value added by smooth muscle cells. EMT, as a transitional state between the epithelial and mesenchymal phenotypes, is a key inducer of airway fibrotic remodeling (37,38).
In this study, to clarify whether IL6 and IL9 can influence airway remodeling in asthmatic patients by regulating airway remodeling-related genes and EMT-associated genes, we performed correlation analysis of IL6 and IL9 with airway remodeling-related genes and EMT-associated genes. Our study revealed that IL6 and UBE2C may bind to each other, and IL6 has a regulatory relationship with GEM. These results suggest that Th2-type airway inflammatory factors can influence airway remodeling by binding to or regulating target factors. It is important to note that IL6 is a chemokine (39) that usually binds to the cell membrane or IL6R (40,41), whereas UBE2C is present intracellularly and belongs to the E2 family of enzymes in the ubiquitin-proteasome pathway (42), which are not conditioned to bind in terms of spatial location. We hypothesized that IL6 could enter the cell and bind to UBE2C protein through vesicles during tracheal remodeling in asthmatic patients; or the binding is completed intracellularly before IL6 is secreted out of the cell, forming a protein complex.
To further explain the molecular mechanism of IL6 and IL9 in regulating tracheal remodeling, the ceRNA network of IL6 and IL9 was constructed in this study. In this network, we identified a total of 24 miRNAs and 22 lncRNAs. For the downstream regulatory mechanisms of IL6 and IL9, GSEA was performed in this study. TLRs are a class of relatively conserved transmembrane proteins that exist in nature and are widely found in various cells such as immune cells and epithelial cells in the human body, including airway epithelial cells. It was found that the TLR2/TLR4 signaling pathway abnormally activates neutrophils, monocytes, and CD4+ Th2 lymphocytes in the airways, producing large amounts of IL4, IL5, and IL13, upregulating IgE, and increasing airway hyperresponsiveness, contributing to acute asthma attacks (43,44). Further analysis showed that variants of rs3804099 and Arg753Gln in TLR2 and rs4986791 and Asp299Gly in TLR4 increase the risk of asthma and increase asthma susceptibility in the general population (45). These studies suggest that the TLR signaling pathway plays an important role in asthma development, and therefore, the study of the TLR signaling pathway is a new direction for asthma prevention and treatment.
Conclusions
In summary, this study further mined the asthma gene microarray database through bioinformatics analysis and identified key genes and important pathways affecting airway remodeling in asthma patients, providing new ideas to uncover the mechanism of airway remodeling due to asthma and then seek new therapeutic targets.
Acknowledgments
Funding: This work was supported by
Footnote
Reporting Checklist: The authors have completed the TRIPOD reporting checklist. Available at https://jtd.amegroups.com/article/view/10.21037/jtd-23-1628/rc
Peer Review File: Available at https://jtd.amegroups.com/article/view/10.21037/jtd-23-1628/prf
Conflicts of Interest: All authors have completed the ICMJE uniform disclosure form (available at https://jtd.amegroups.com/article/view/10.21037/jtd-23-1628/coif). The authors have no conflicts of interest to declare.
Ethical Statement: The authors are accountable for all aspects of the work in ensuring that questions related to the accuracy or integrity of any part of the work are appropriately investigated and resolved. The study was conducted in accordance with the Declaration of Helsinki (as revised in 2013).
Open Access Statement: This is an Open Access article distributed in accordance with the Creative Commons Attribution-NonCommercial-NoDerivs 4.0 International License (CC BY-NC-ND 4.0), which permits the non-commercial replication and distribution of the article with the strict proviso that no changes or edits are made and the original work is properly cited (including links to both the formal publication through the relevant DOI and the license). See: https://creativecommons.org/licenses/by-nc-nd/4.0/.
References
- Becker AB, Abrams EM. Asthma guidelines: the Global Initiative for Asthma in relation to national guidelines. Curr Opin Allergy Clin Immunol 2017;17:99-103. [Crossref] [PubMed]
- Jiang W, Ma Z, Zhang H, et al. Efficacy of Jia Wei Yang He formula as an adjunctive therapy for asthma: study protocol for a randomized, double blinded, controlled trial. Trials 2018;19:355. [Crossref] [PubMed]
- Dharmage SC, Perret JL, Custovic A. Epidemiology of Asthma in Children and Adults. Front Pediatr 2019;7:246. [Crossref] [PubMed]
- Papi A, Brightling C, Pedersen SE, et al. Asthma. Lancet 2018;391:783-800. [Crossref] [PubMed]
- Fainardi V, Passadore L, Labate M, et al. An Overview of the Obese-Asthma Phenotype in Children. Int J Environ Res Public Health 2022;19:636. [Crossref] [PubMed]
- Shabestari AA, Imanparast F, Mohaghegh P, et al. The effects of asthma on the oxidative stress, inflammation, and endothelial dysfunction in children with pneumonia. BMC Pediatr 2022;22:534. [Crossref] [PubMed]
- Varricchi G, Ferri S, Pepys J, et al. Biologics and airway remodeling in severe asthma. Allergy 2022;77:3538-52. [Crossref] [PubMed]
- Huang Y, Qiu C. Research advances in airway remodeling in asthma: a narrative review. Ann Transl Med 2022;10:1023. [Crossref] [PubMed]
- Kuwano K, Bosken CH, Paré PD, et al. Small airways dimensions in asthma and in chronic obstructive pulmonary disease. Am Rev Respir Dis 1993;148:1220-5. [Crossref] [PubMed]
- Boulet LP. Airway remodeling in asthma: update on mechanisms and therapeutic approaches. Curr Opin Pulm Med 2018;24:56-62. [Crossref] [PubMed]
- Yang Y, Li Y, Qi R, et al. Constructe a novel 5 hypoxia genes signature for cervical cancer. Cancer Cell Int 2021;21:345. [Crossref] [PubMed]
- Kimura R, Nakata M, Funabiki Y, et al. An epigenetic biomarker for adult high-functioning autism spectrum disorder. Sci Rep 2019;9:13662. [Crossref] [PubMed]
- Newman AM, Liu CL, Green MR, et al. Robust enumeration of cell subsets from tissue expression profiles. Nat Methods 2015;12:453-7. [Crossref] [PubMed]
- Balachandran VP, Gonen M, Smith JJ, et al. Nomograms in oncology: more than meets the eye. Lancet Oncol 2015;16:e173-80. [Crossref] [PubMed]
- Pierce BG, Wiehe K, Hwang H, et al. ZDOCK server: interactive docking prediction of protein-protein complexes and symmetric multimers. Bioinformatics 2014;30:1771-3. [Crossref] [PubMed]
- Wang J, Chen X, Tian Y, et al. Six-gene signature for predicting survival in patients with head and neck squamous cell carcinoma. Aging (Albany NY) 2020;12:767-83. [Crossref] [PubMed]
- Gras D, Bourdin A, Vachier I, et al. An ex vivo model of severe asthma using reconstituted human bronchial epithelium. J Allergy Clin Immunol 2012;129:1259-1266.e1. [Crossref] [PubMed]
- Holgate ST, Arshad HS, Roberts GC, et al. A new look at the pathogenesis of asthma. Clin Sci (Lond) 2009;118:439-50. [Crossref] [PubMed]
- Liu LL, Li FH, Zhang Y, et al. Tangeretin has anti-asthmatic effects via regulating PI3K and Notch signaling and modulating Th1/Th2/Th17 cytokine balance in neonatal asthmatic mice. Braz J Med Biol Res 2017;50:e5991. [Crossref] [PubMed]
- Malmström K, Pelkonen AS, Malmberg LP, et al. Lung function, airway remodelling and inflammation in symptomatic infants: outcome at 3 years. Thorax 2011;66:157-62. [Crossref] [PubMed]
- Dong L, Wang Y, Zheng T, et al. Hypoxic hUCMSC-derived extracellular vesicles attenuate allergic airway inflammation and airway remodeling in chronic asthma mice. Stem Cell Res Ther 2021;12:4. [Crossref] [PubMed]
- Banno A, Reddy AT, Lakshmi SP, et al. Bidirectional interaction of airway epithelial remodeling and inflammation in asthma. Clin Sci (Lond) 2020;134:1063-79. [Crossref] [PubMed]
- Anderson GP. Pharmacology of formoterol: an innovative bronchodilator. Agents Actions Suppl 1991;34:97-115.
- Halwani R, Al-Muhsen S, Hamid Q. Airway remodeling in asthma. Curr Opin Pharmacol 2010;10:236-45. [Crossref] [PubMed]
- Al-Ramli W, Préfontaine D, Chouiali F, et al. T(H)17-associated cytokines (IL-17A and IL-17F) in severe asthma. J Allergy Clin Immunol 2009;123:1185-7. [Crossref] [PubMed]
- Simpson JL, Grissell TV, Douwes J, et al. Innate immune activation in neutrophilic asthma and bronchiectasis. Thorax 2007;62:211-8. [Crossref] [PubMed]
- Bettelli E, Carrier Y, Gao W, et al. Reciprocal developmental pathways for the generation of pathogenic effector TH17 and regulatory T cells. Nature 2006;441:235-8. [Crossref] [PubMed]
- Townsend JM, Fallon GP, Matthews JD, et al. IL-9-deficient mice establish fundamental roles for IL-9 in pulmonary mastocytosis and goblet cell hyperplasia but not T cell development. Immunity 2000;13:573-83. [Crossref] [PubMed]
- Goswami R, Kaplan MH. A brief history of IL-9. J Immunol 2011;186:3283-8. [Crossref] [PubMed]
- Luo W, Hu J, Xu W, et al. Distinct spatial and temporal roles for Th1, Th2, and Th17 cells in asthma. Front Immunol 2022;13:974066. [Crossref] [PubMed]
- Dua B, Upadhyay R, Natrajan M, et al. Notch signaling induces lymphoproliferation, T helper cell activation and Th1/Th2 differentiation in leprosy. Immunol Lett 2019;207:6-16. [Crossref] [PubMed]
- Alhetheel A, Albarrag A, Shakoor Z, et al. Assessment of Th1/Th2 cytokines among patients with Middle East respiratory syndrome coronavirus infection. Int Immunol 2020;32:799-804. [Crossref] [PubMed]
- Li B, Zhang L, Zhao J, et al. The value of cytokine levels in triage and risk prediction for women with persistent high-risk human papilloma virus infection of the cervix. Infect Agent Cancer 2019;14:16. [Crossref] [PubMed]
- Hwang YH, Kim SJ, Kim H, et al. The Protective Effects of 2,3,5,4'-Tetrahydroxystilbene-2-O-β-d-Glucoside in the OVA-Induced Asthma Mice Model. Int J Mol Sci 2018;19:4013. [Crossref] [PubMed]
- Pu Y, Liu Y, Liao S, et al. Azithromycin ameliorates OVA-induced airway remodeling in Balb/c mice via suppression of epithelial-to-mesenchymal transition. Int Immunopharmacol 2018;58:87-93. [Crossref] [PubMed]
- Ortiz-Zapater E, Signes-Costa J, Montero P, et al. Lung Fibrosis and Fibrosis in the Lungs: Is It All about Myofibroblasts? Biomedicines 2022;10:1423. [Crossref] [PubMed]
- He F, Liao B, Pu J, et al. Exposure to Ambient Particulate Matter Induced COPD in a Rat Model and a Description of the Underlying Mechanism. Sci Rep 2017;7:45666. [Crossref] [PubMed]
- Chilosi M, Caliò A, Rossi A, et al. Epithelial to mesenchymal transition-related proteins ZEB1, β-catenin, and β-tubulin-III in idiopathic pulmonary fibrosis. Mod Pathol 2017;30:26-38. [Crossref] [PubMed]
- Chen S, Chen Z, Deng Y, et al. Prevention of IL-6 signaling ameliorates toluene diisocyanate-induced steroid-resistant asthma. Allergol Int 2022;71:73-82. [Crossref] [PubMed]
- Calabrese LH, Rose-John S. IL-6 biology: implications for clinical targeting in rheumatic disease. Nat Rev Rheumatol 2014;10:720-7. [Crossref] [PubMed]
- Garbers C, Aparicio-Siegmund S, Rose-John S. The IL-6/gp130/STAT3 signaling axis: recent advances towards specific inhibition. Curr Opin Immunol 2015;34:75-82. [Crossref] [PubMed]
- Xie C, Powell C, Yao M, et al. Ubiquitin-conjugating enzyme E2C: a potential cancer biomarker. Int J Biochem Cell Biol 2014;47:113-7. [Crossref] [PubMed]
- Gao W, Xiong Y, Li Q, et al. Inhibition of Toll-Like Receptor Signaling as a Promising Therapy for Inflammatory Diseases: A Journey from Molecular to Nano Therapeutics. Front Physiol 2017;8:508. [Crossref] [PubMed]
- Liu CF, Drocourt D, Puzo G, et al. Innate immune response of alveolar macrophage to house dust mite allergen is mediated through TLR2/-4 co-activation. PLoS One 2013;8:e75983. [Crossref] [PubMed]
- Zhao J, Shang H, Cao X, et al. Association of polymorphisms in TLR2 and TLR4 with asthma risk: An update meta-analysis. Medicine (Baltimore) 2017;96:e7909. [Crossref] [PubMed]
(English Language Editor: L. Huleatt)