High-resolution transcriptomics analysis of CXCL13+ EPSTI1+ CDK1+ cells with a specific focus on lung adenocarcinoma
Highlight box
Key findings
• Integrated analyses have revealed a strong correlation among CXCL13, CDK1, and EPSTI1. These three genes supplied prognostic information and could help identify immuno-sensitive populations with lung adenocarcinoma (LUAD).
What is known and what is new?
• CXCL13, EPSTI1, and CDK1 has been described in meta-analyses and systematic review.
• We presented an integrated overview of the CXCL13+ EPSTI1+ CDK1+ subpopulation, with a specific focus on LUAD.
What is the implication, and what should change now?
• The CXCL13, EPSTI1, and CDK1 positive subpopulation formed the niche that might offer additional targets for immune checkpoint blockade therapies.
Introduction
Immune checkpoint inhibitors (ICIs) have revolutionized cancer treatment for a variety of solid tumors, including non-small cell lung cancer (NSCLC) (1). Although biomarkers associated with efficacy have been identified, such as programmed cell death ligand 1 (PD-L1) expression and tumor mutational burden (TMB), most patients with NSCLC do not achieve positive outcomes with ICIs. Targeted therapies have significantly improved prognosis in oncogenic-driven subgroups, but also pose challenges for immunotherapy (1,2). Thus, there is a need to identify intratumoral immune infiltration related to ICIs response.
Single-cell RNA (scRNA) analysis has recently been utilized to clarify high-resolution tumor microenvironment (TME) profiles (3,4). Paradoxically, CXCL13, considered one of the best ICIs markers, is highly expressed in neoantigen-associated T cells but is strongly linked to pathologically unresponsive NSCLC patients receiving ICIs (5). At the same time, widespread expression of CXCL13 improves antitumor immune responses in NSCLC, implying that fluorescence-activated cell sorting (FACS)-sorted CXCL13 subpopulations are promising candidates (5,6).
Recent transient single-cell kinetic results have demonstrated the enrichment of PD-L1 blockade-induced proliferative CD8+ T exhausted cells in cancer nests (7). CDK1 plays a critical role in regulating T cell proliferation, making it a potential candidate for screening the CXCL13 subpopulation (8). However, a challenge arises as both CDK1 and CXCL13 are expressed in cancer cells (6). Further studies are needed to obtain a more immunologically characterized subpopulation. Based on previous data and the immunotherapy-related website ICBatlas (9,10), EPSTI1 has been identified as a favorable indicator of ICI response, and may have the potential to describe complex subpopulations. Furthermore, it is more practical to use streamlined signatures in clinical settings. To prioritize tumor-type applications, we represented three genes in the pan-cancer profile and compared them with pathways that determined response to pembrolizumab (11).
In this study, we have defined the immuno-sensitive niche as CXCL13, EPSTI1, and CDK1 positivity. Our hypothesis suggests that high expression of the three-gene signature may predict the presence of a subpopulation. After identifying subgroups according to the signature, our analysis focused on prognosis, pan-cancer, and high-dimensional transcriptome. Meanwhile, the signature-pathway association was established by correlation analysis. We present this article in accordance with the TRIPOD reporting checklist (available at https://jtd.amegroups.com/article/view/10.21037/jtd-23-1164/rc).
Methods
Data acquisition and processing
The study was conducted in accordance with the Declaration of Helsinki (as revised in 2013). We collected four publicly released scRNA datasets with primary NSCLC (Table S1) (12-15). Among these datasets, E-MTAB-6149 and the West China Hospital dataset were specifically referred to as NSCLC, whereas the others were regarded as lung adenocarcinoma (LUAD). We allocated E-MTAB-6149 as the training set, and used the remaining datasets for validation. In addition, four datasets, namely GSE154826, GSE179994, GSE111907, and GSE184398, which contained FACS information, were utilized for further analysis (14-17). Compound perturbation datasets including Cancer Cell Line Encyclopedia (CCLE), Genomics of Drug Sensitivity in Cancer (GDSC), ConnectivityMap (CMAP), and our data regarding the dinaciclib/alvocidib-treated NCI-H1944 cell line, were described in detail in our previous study (9). For the comprehensive analysis of bulk RNA sequencing, we included the stage IB–IIIA naive cohort The Cancer Genome Atlas (TCGA)-LUAD (n=320) and the ICIs-treated cohort called Stand Up to Cancer-Mark Foundation (SU2C-MARK) LUAD (n=47) (9,18).
We gathered the information of 102 LUAD cases that passed the quality test and 47 of them contained mutations and overall survival data in the SU2C-MARK data. For TCGA-LUAD, common clinical features were incorporated, including TMB, global methylation, estimated tumor purity, and fraction genome altered (FGA). In addition, expression profiles, genomic, and clinical information were downloaded from Lung Cancer Explorer (LCE; https://lce.biohpc.swmed.edu/lungcancer/), Xena (https://xena.ucsc.edu/), and cBioPortal (https://www.cbioportal.org/) websites (19-21). We used our re-analyzed data, which included only stage IB–IIIA patients and excluded those who underwent preoperative chemotherapy. To perform a meta-analysis, we obtained 12 separate cohorts from LCE, each consisting of three genes, from the pool of 27 previously described cohorts with more than 100 samples. Here, the TCGA-LUAD data came from LCE website to maintain consistency. Using the maftools R package, we analyzed and visualized the whole-exome sequencing data from the SU2C-MARK cohort (22). Frequent and differential mutations between the two groups were compared.
Seurat R package (23) was used to load and visualize the scRNA datasets. Quality control measures were implemented to retain only cells that met the criterion of having unique molecular identifier of more than 200. T-distributed stochastic neighbor embedding (t-SNE) was used to depict annotated clusters and marker genes. Specifically, “None” is for unidentifiable clusters from other studies. Moreover, we used the CellPhoneDB python package to infer intercellular ligand-receptor pairing from scRNA (24). The type labels of all cells were randomly shuffled 1,000 times to establish the mean expression levels of ligands and receptors within each interaction cluster. Differentially expressed genes (DEGs) in the bulk data were considered as those with a greater than two-fold change, within the scRNA FindMarkers function settings as follows: only.pos = TRUE, min.pct =0.35, logfc.threshold =0.5, test.use = ‘MAST’.
Identification and abundance assessment of CXCL13+ EPSTI1+ CDK1+ subpopulation
We defined subpopulation positivity as the concurrent expression of CXCL13, EPSTI1, and CDK1 in a single sample, with values exceeding 0. In other words, a positive cell needed to express all three markers. Then, the relative abundance of each subpopulation was evaluated on each sample using the above three genes and by the R package gene set variation analysis (GSVA) (function: method = ‘ssgsea’, kcdf = ‘Gaussian’, abs.ranking = TRUE) (25). Supplemental explanation: Gaussian model was applied to logarithmic scale data, setting the absolute ranking to obtain the maximum variation of the score. For the application of the single-sample gene set enrichment analysis (ssGSEA) algorithm, each data needed to be implemented separately, and the scores were not directly comparable across queues. Rating groupings were ranked by median value unless otherwise noted.
Survival and meta-analysis
We performed Cox proportional hazards model for survival analysis and calculated 95% confidence intervals (CIs). The R package survminer was conducted to plot the Kaplan-Meier survival by the log-rank test. The receiver operating characteristic (ROC) curve and cross-queue prognosis were visualized via R package pROC and meta, respectively. Only overall survival was considered in this study.
Comprehensive analysis from online website
We explored the correlation of genes in the TME in terms of bulk and scRNA through sites Tumor-Immune System Interactions and Drug Bank (TISIDB; http://cis.hku.hk/TISIDB/) and Tumor Immune Single Cell Hub (TISCH; http://tisch.comp-genomics.org/), respectively (26,27). The ICBatlas (http://bioinfo.life.hust.edu.cn/ICBatlas/) and SpatialDB (http://www.spatialomics.org/SpatialDB/) websites were used to explore the transcriptome in the context of pan-cancer ICIs and spatial distribution, respectively (10,28). In addition, gene expression in cancer and adjacent normal tissues, and signature correlation were stored in Gene Expression Profiling Interactive Analysis (GEPIA2; http://gepia2.cancer-pku.cn/) (29). DEGs were used to access potential upstream transcription factors through ChEA3 (https://maayanlab.cloud/chea3/), which was based on experimental data (30). Finally, the pathology and sublocalization at the protein level were analyzed using the Human Protein Atlas (HPA) website (https://www.proteinatlas.org/) (31). Note that antibodies including anti-CXCL13 (HPA052613), anti-EPSTI1 (HPA017362), and anti-CDK1 (CAB003799) in lung tumors and normal tissues have been studied.
Statistical analysis
The R software (The R Foundation for Statistical Computing, Vienna, Austria) was used to write all codes for the analyses. A heatmap was created to show the degree of correlation and difference. The variables between groups were compared using the non-parametric test (Wilcoxon test or Kruskal-Wallis test). Statistical significance was determined at a P value <0.05 and adjusted for Bonferroni testing.
Results
EPSTI1 is co-expressed with CXCL13 and CDK1
The clinical significance of proliferating T cells is unclear. For the CXCL13+ CDK1+ subpopulation, which contained proliferating T cells and other components, we are primarily concerned with immunogenicity, which is also similar to the concern of lymph nodes (6). The flowchart of our search for markers that could further delineate the CXCL13+ CDK1+ subpopulation is displayed in Figure 1A. According to Lambrechts et al.’s descriptions, T cells with high CDK1 expression are typically proliferative, leading to the conclusion that CXCL13+ CDK1+ T cells are also highly proliferative (Figure 1B) (12). Then, several markers including CXCL13, IFNG, CDK1, and interferon (IFN)-stimulated genes (ISGs) (e.g., MX1, IRF7, and EPSTI1) were examined (Figure 1C) (32). We screened candidate genes associated with the CXCL13+ CDK1+ subpopulation according to the following two criteria: significant enrichment in immune-related function and down-regulation of cyclin-dependent kinase (CDK) inhibitors (table available at https://cdn.amegroups.cn/static/public/jtd-23-1164-1.xls, Figure 1D). The final two genes identified were HAVCR2 (a known checkpoint) and EPSTI1.
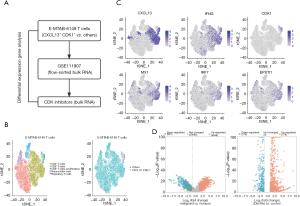
Identification of EPSTI1 as an indicator of immunosensitivity
We aimed to identify markers relevant to ICIs, CXCL13, and EPSTI1 for the top 35 genes regarding immuno-sensitivity according to the ICBatlas website analysis (10). However, the role of EPSTI1 in cancer immunity compared to that of CXCL13 is not yet fully understood. To investigate further, we utilized pan-cancer data and observed that EPSTI1 exhibited fewer genetic alterations in NSCLC (Figure 2A) (21). Importantly, when we separated the immunotherapy samples into responders and non-responders, the ICBatlas website demonstrated that the discrepancy in EPSTI1 expression among NSCLC type was the most significant (10); EPSTI1 expression could effectively distinguish between responders and non-responders [area under the curve (AUC) =0.8182; Figure 2B]. Thus, we delved deeper into the intrinsic mechanism by which EPSTI1 facilitates immune sensitivity. Firstly, EPSTI1 expression was positively correlated with most immune cells in pan-cancer (Figure 2C). Meanwhile, EPSTI1 was more likely to activate the immune through the antigen presentation process (Figure S1A-S1C). Additionally, previous literature revealed that EPSTI1 was regulated by IFN-α (33), which aligns with our finding that EPSTI1 was positively linked to the type I IFN pathway member (e.g., MX1; Figure 2D) (32). Overall, our analysis showed that EPSTI1 is an immuno-sensitive marker, especially in NSCLC.
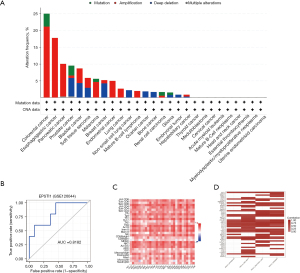
Pan-cancer profile of the CXCL13+ EPSTI1+ CDK1+ subpopulation
Based on the E-MTAB-6149 T cells dataset, we classified the subpopulation order into CXCL13, EPSTI1, and CDK1 (Figure 1C) (12). The expression of CXCL13, EPSTI1, and CDK1 can partially indicate the abundance of the CXCL13, EPSTI1, and CDK1 positive subpopulation. In one-third of all cancer types, such as breast carcinoma, the tumor tissues had notably higher gene expression levels compared to adjacent normal tissues (Figure S2A-S2C) (29). Therefore, our next analysis focused on the tumor tissue. Transcriptional features have been evaluated in the pembrolizumab trials; the three-gene signature has exhibited a robust correlation with IFN-γ, T-cell-inflamed, proliferation, and glycolysis in pan-cancer (Figure 3A) (11). This means that the generated signature is a key measure of previous pathways. Furthermore, we utilized the GSVA R package to estimate the CXCL13, EPSTI1, and CDK1 positive subpopulation’s relative abundance (25). Theoretically, populations with pathology-associated regions have a higher gene signature. Our analysis demonstrated that LUAD had a high abundance, ranking 7th across all cancer types (Figure 3B). This finding was further supported by the GSE184398 cohort (Figure 3C). In contrast to whole cells, diminished scores were observed in the cancer portion of LUAD (Figure 3C), signifying that the CXCL13, EPSTI1, and CDK1 positive subpopulation may be related to immune rather than cancer cells. But in T myeloid-derived suppressor cells (MDSCs), and Treg cells, the subpopulations were not particularly significant (Figure S3A-S3C). The results of FACS sequencing implied that the CXCL13, EPSTI1, and CDK1 positive subpopulation was mainly present in the non-epithelial fraction of LUAD, which we further confirmed in the high-resolution scRNA datasets (Figure S4A-S4D). As expected, the CXCL13, EPSTI1, and CDK1 positive subpopulation was mainly composed of proliferating T cells (48–82%; Figure S4B-S4D). Notably, the proportion of epithelium was higher in lung squamous carcinoma (LUSC) than in LUAD [basal: 11.5% vs. alveolar type II (ATII): 1.6%; Figure S4A], which was consistent with recent descriptions of proliferative cancer epithelium in LUSC (13). Overall, we demonstrated three-signature associated pathways, and identified tumor categories that prioritized the validation of niche, a TME that influenced prognosis and drug resistance (1).
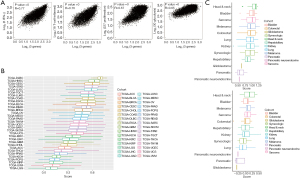
Comprehensive analysis from the three-gene signature in LUAD
Given the possible confounding effect of the proliferative features of LUSC, our analysis was changed to LUAD. Using the GSVA R package, we found that high score of three-gene signature was associated with a better prognosis for ICIs after selecting optimal the cut-off point [hazard ratio (HR) =0.423; 95% CI: 0.188–0.952; Figure 4A] (25). Separated by the high frequency of mutations in the high- vs. low-scoring group, STK11 mutations were mainly in the low-scoring group (Figure 4B). Meanwhile, SYCP2 mutations were predominantly detected in the low-scoring group, whereas CSMD3, KIAA1109, USH2A, ANKRD30B, CPXM2, NBEA, TG, TRPM1, and TP53 mutations were significantly enriched in the high-scoring group (Figure 4C). We speculated that it may be related to genes with increased TMB, such as CSMD3 and USH2A (34). Meanwhile, the score may reflect the immunogenicity of the mutation, as lower scores have a higher proportion of STK11 (2).
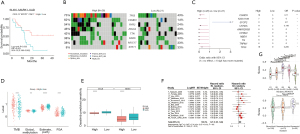
Furthermore, in stage IB–IIIA TCGA-LUAD, the high-scoring group had higher TMB, and global methylation, but lower tumor purity and FGA than the low-scoring group (Figure 4D). Also, only dasatinib was significant in cross-validated by CCLE and GDSC datasets (log fold change >0.7; Figure 4E). Next, the correlation between scores and factors was studied. Importantly, the prognostic significance of LUAD was consistent across multiple datasets, indicating that the results of our meta-analysis could be considered reliable (19). We used the random effects model to validate that high scores were a factor in unfavorable prognosis, after detecting no significant heterogeneity across the datasets (HR =1.21; 95% CI: 1.05–1.4; Figure 4F). Immune-related S3 and squamous clusters with a poorer prognosis were found to have a higher score (Figure 4G) (9,35). Combined with our results, a subset of the population with a poorer prognosis may be suitable for ICIs.
CXCL13+ EPSTI1+ CDK1+ spatial presentation, cellular communication and regulation
We assumed that a high abundance of the signature population contained the CXCL13, EPSTI1, and CDK1 positive subpopulation, and therefore would benefit from ICIs. The human protein database suggested that CXCL13 and EPSTI1 would be suitable for cytoplasmic staining in 1 LUAD sample (Figure S5A) (31). Meanwhile, CDK1 and EPSTI1 were both positively expressed in normal lung tissue (Figure S5B). According to the spatial transcriptome results, CXCL13, EPSTI1, and CDK1 were co-localized in breast carcinoma (Figure 5A) (28). Considering that CDK1 expression was widespread, we had detected the presence of the CXCL13, EPSTI1, and CDK1 positive subpopulation in at least two tumor species, which had been supported by pan-cancer profiles (Figure 3B,3C, Figure S3).
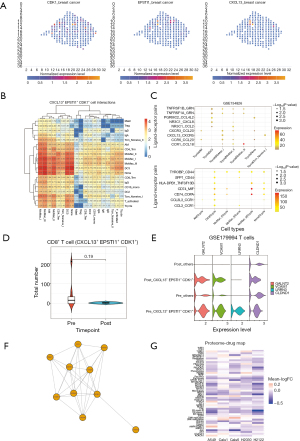
CITE-seq is the technology to simultaneously measure cell surface proteome and the transcriptome from the single-cell level (14). We supposed that there would be an immune-specific subpopulation that was highly matched to the CD45-positive sorted dataset. The subpopulation was defined as described in the Methods section, and the CXCL13, EPSTI1, and CDK1 positive subpopulation showed active communication of myeloid cells (Figure 5B), despite that half of the subpopulation was proliferating T cells (Figure S4C). Moreover, the ligand-receptor pairing analysis showed that TNF, CD74, and CD44 signals were the most significant, and that DC3 regulated proliferating T cells through multiple pathways (table available at https://cdn.amegroups.cn/static/public/jtd-23-1164-2.xls, Figure 5C).
We wanted to explore the role of the CXCL13, EPSTI1, and CDK1 positive subpopulation in the context of both immune and T-cell FACS sequencing. Although not statistically significant, we observed a decrease in the proportion of proliferating T cells in the GSE179994 cohort after treatment, which supported the previous description (P=0.19; Table S2, Figure 5D) (15). A comparison of the CXCL13, EPSTI1, and CDK1 positive subpopulation before and after treatment revealed that GALNT2, VCAM1, LRRN3, and CLDND1 were significantly reduced (Figure 5E). Importantly, smoking-associated characteristics involving LRRN3 and CLDND1 were decreased post-treatment, and LRRN3 was predominantly expressed in the CXCL13, EPSTI1, and CDK1 positive subpopulation (36). Then, we performed transcription factor and CMAP enrichment analyses using 121 highly-expressed genes from the CXCL13, EPSTI1, and CDK1 positive subpopulation before chemo-immunotherapies (table available at https://cdn.amegroups.cn/static/public/jtd-23-1164-3.xls) (37). Among the ChEA3 predicted top 10 transcription factors, FOXM1 was validated by CMAP analysis (table available at https://cdn.amegroups.cn/static/public/jtd-23-1164-4.xls, Figure 5F) (30,37). Meanwhile, CDK inhibitors were found to reduce the above features (table available at https://cdn.amegroups.cn/static/public/jtd-23-1164-4.xls). Similarly, we used the proteome from lung cancer cell lines to infer compound perturbations (38). Since only a small number of target genes were considered significant, the fold change related to compound treating was averaged to simplify. Most compounds tended to reduce the above subpopulation-related characteristics, and compounds PD184352 (MEK I), Staurosporine (Pan Kinase), and BrefeldinA (ER Stress II) were noted for all five cell lines (Figure 5G).
Discussion
In this study, we showed that EPSTI1 is a positive target of CDK inhibitors, which is one of the characteristics of IFN, and further elucidated the role of EPSTI1 in the cancer immunity. Importantly, the CXCL13, EPSTI1, and CDK1 positive subpopulation can be used as a marker to screen for patients who will benefit from ICIs for LUAD.
The positive expression of CXCL13, EPSTI1, and CDK1 positive samples in LUAD may lead to poor prognosis, but patients can benefit from ICIs. Populations with high score of the three-gene signature have high TMB but low chromosomal variation, and high methylation levels but low tumor purity. A recent study has shown that tumor-infiltrating lymphocyte patterns are inconsistent with checkpoint profile, and our results may be useful in finding ICIs-sensitive populations (39). For the CXCL13, EPSTI1, and CDK1 positive subpopulation in LUAD, only one cell was found to express all three of these markers simultaneously in the stroma, and there was a rare number detected in unsorted sequencing or peripheral blood tissues (data not shown). Of course, there are challenges with scRNA data, such as drop-out events and measurement noise (4). Since the CXCL13, EPSTI1, and CDK1 positive subpopulation was rarely expressed in cancer cells fractions, and was verified with bulk and scRNA (Figure 3C, Figure S4A), immune-enrichment sequencing was recommended.
We hypothesized that pre-existing cells could help to identify sensitive populations susceptible to ICIs. However, therapy-guided TME differs from the naïve state. In NSCLC, ICIs therapies have been found to induce proliferating CD8+ T cells infiltration into the cancer nest, but a durable response may require a reduction in this subpopulation (40). Similarly, therapies have been shown to result in a decrease in proliferating T cells in hepatocellular carcinoma (41). Proliferating T cells being in a state of memory but not in exhaustion may contribute to long-term responses (42). It is of interest to further investigate of the transition from memory to exhaustion. Recently, Chu et al. characterized the pan-cancer T cell atlas and identified CXCL13+ as a subset of proliferating T cells (43). However, we found that proliferating T cells in NSCLC were not coordinated with the overall profile. Despite the presence of proliferating T cells, it remains unclear whether this group is biologically significant. Associated with our results, RBPJ may be the regulator of the CXCL13, EPSTI1, and CDK1 positive subpopulation (44), which suggests that the validation of the 121 therapeutically relevant subgroup genes is meaningful (table available at https://cdn.amegroups.cn/static/public/jtd-23-1164-3.xls).
The proliferation markers are widely expressed and not cell-specific. Although subpopulations in T cells are rare, key targets and regulatory networks are depicted (Figure 5E,5F). A recent study showed that CXCL13 is widely expressed, and similarly, the CXCL13, EPSTI1, and CDK1 positive subpopulation is not restricted to T cells (6). Thus, we explored cellular communication in the CXCL13, EPSTI1, and CDK1 positive subpopulation, where the CD74-MIF/COPA pathway was significant (Figure 5C). It can be speculated that maintenance of CD74 signals may be associated with better immune responses (45). Taken together, the CXCL13, EPSTI1, and CDK1 positive subpopulation may help to explore cellular communication, such as DC3 activation of T cells via signaling. In this study, we found that at least two tumor species contained this subpopulation and that it is immunospecific in LUAD. Thus, our data are mainly based on LUAD, but pan-cancer validation may be profitable. We believe that the identification of the CXCL13, EPSTI1, and CDK1 positive subpopulation will help to obtain high-resolution information related to ICIs. This cannot be evaluated with a deconvolution-based algorithm (4).
It is not surprising that CDK1 and ISG are co-expressed. Previous studies have shown that CDK1 and CDK inhibitors could regulate the IFN pathway (46,47). Emerging evidence also suggests that CDK4, another member of the CDK family may affect T-cell survival (48). In this study, we have identified relevant targets of CDK1, such as GALNT2 and VCAM1. Previous study has shown that O-glycosylation can be modified by proliferative factors (e.g., FOXM1), and it is plausible that transcription factor synergist mediators may regulate the activity of the downstream enzyme GALNT2 (49). In addition, VCAM1 and CDK1 are considered key molecules in the progression of idiopathic pulmonary fibrosis (50). In conclusion, we believe that the concept based on co-expression allows the discovery of additional targets and may provide insights into the underlying mechanisms of CDK-applicable diseases.
Meta-analyses and systematic review have demonstrated the prognostic role of CXCL13, EPSTI1, and CDK1, but the CXCL13, EPSTI1, and CDK1 positive subpopulation has not been studied (51-53). CDK1 is associated with cancer stemness and proliferation, whereas CXCL13 has previously been considered a promoter of tertiary lymphoid structures (6,53). EPSTI1 is mainly expressed in the immune and stromal fractions and its immunological role of EPSTI1 in colon cancer has been described (52). We described the pan-tumor spectrum of EPSTI1, in which EPSTI1 was once highly amplified in colon cancer, but was ignored (Figure 2A) because EPSTI1 plays a tumor suppressor role in LUSC, and it was necessary to determine whether EPSTI1 enhances antitumor immunity in LUAD (54). Meanwhile, despite the importance of CDK1 as a member of proliferation, our score differs from the past pan-cancer proliferation index (55). Again, squamous-lineage scores were higher compared to those of other cancers (Figure 3B,3C). In addition, TP53 mutations have been found to have higher scores and it is interesting to explore patterns of co-alteration (2). Importantly, we highlighted co-localization of EPSTI1, CXCL13, and CDK1 by spatial transcriptome and HPA immunohistochemistry databases. From the perspective of antibody validation, prioritizing CXCL13 was necessary because CDK1 and EPSTI1 could be expressed in the cytoplasm of most tumors. In addition, we hypothesize that the high RNA expression enrichment of CXCL13 and EPSTI1 in human lymph nodes may lead to enhanced immuno-sensitivity.
Immune checkpoint levels are higher in LUSC than in LUAD, which exhibits activated MHC-II (56). Our previous findings also indicated that different TME profiles are associated with distinct subtypes, such as the squamous subtype having an increased CXCL13, EPSTI1, and CDK1 positive subpopulation compared to the bronchioid subtype (9). Apart from this, populations previously negative for NKX2-1 and with solid pathology exhibited higher proportions of PD-L1. The CXCL13, EPSTI1, and CDK1 positive subpopulation reflected all three features of neoantigen activation, ISG, and proliferation simultaneously. This subpopulation might identify patients with poor prognosis who would benefit from ICIs and dasatinib. Combined with our results, dasatinib reshapes specific subgroups in the TME to complement ICIs. Indeed, the results with dasatinib in some datasets and cell lines were consistent with our previous observations, such as in the H2030 cell line (38). Our results suggest that the multi-omics data are meaningful, and indicated the potential usefulness of CMAP analysis in providing information on gene knockdown and compound perturbation (37). Major limitations include the fact that our study primarily involved data analysis and that the definition of cell types was dependent on other studies. The deficiencies of sequencing should also be considered.
Conclusions
Our study discovered the signature comprising CXCL13, EPSTI1, and CDK1 was indicative of prognosis and drug sensitivity. Pan-cancer analyses not only revealed the distribution of the three-gene signature but also supported its association with the previously identified pathways. Additionally, we verified the existence of subpopulations and demonstrated the cellular communication within the immuno-sensitive niche. High-resolution and multi-omics analyses suggested subpopulations of associated targets and guided treatment.
Acknowledgments
We appreciate the data from the TCGA, SU2C-MARK, LCE and GEO datasets.
Funding: This study was supported by the National Natural Science Foundation of China (Nos. 82172670, 81972858, 82202951 and 81773245), the Technology Innovation and Application Development Project of Chongqing (Nos. 2023DBXM002, CSTB2022NSCQ-MSX1356 and CSTB2022TIAD-KPX0176), the Cultivation Program for Clinical Research Talents of Army Medical University (Nos. 2018XLC1010 and 2019XQN10), and the Red Medical Talents Development Program of Army Medical University.
Footnote
Reporting Checklist: The authors have completed the TRIPOD reporting checklist. Available at https://jtd.amegroups.com/article/view/10.21037/jtd-23-1164/rc
Peer Review File: Available at https://jtd.amegroups.com/article/view/10.21037/jtd-23-1164/prf
Conflicts of Interest: All authors have completed the ICMJE uniform disclosure form (available at https://jtd.amegroups.com/article/view/10.21037/jtd-23-1164/coif). The authors have no conflicts of interest to declare.
Ethical Statement: The authors are accountable for all aspects of the work in ensuring that questions related to the accuracy or integrity of any part of the work are appropriately investigated and resolved. The study was conducted in accordance with the Declaration of Helsinki (as revised in 2013).
Open Access Statement: This is an Open Access article distributed in accordance with the Creative Commons Attribution-NonCommercial-NoDerivs 4.0 International License (CC BY-NC-ND 4.0), which permits the non-commercial replication and distribution of the article with the strict proviso that no changes or edits are made and the original work is properly cited (including links to both the formal publication through the relevant DOI and the license). See: https://creativecommons.org/licenses/by-nc-nd/4.0/.
References
- Otano I, Ucero AC, Zugazagoitia J, et al. At the crossroads of immunotherapy for oncogene-addicted subsets of NSCLC. Nat Rev Clin Oncol 2023;20:143-59. [Crossref] [PubMed]
- Skoulidis F, Heymach JV. Co-occurring genomic alterations in non-small-cell lung cancer biology and therapy. Nat Rev Cancer 2019;19:495-509. [Crossref] [PubMed]
- Binnewies M, Roberts EW, Kersten K, et al. Understanding the tumor immune microenvironment (TIME) for effective therapy. Nat Med 2018;24:541-50. [Crossref] [PubMed]
- Zeng Z, Li Y, Li Y, et al. Statistical and machine learning methods for spatially resolved transcriptomics data analysis. Genome Biol 2022;23:83. [Crossref] [PubMed]
- Hanada KI, Zhao C, Gil-Hoyos R, et al. A phenotypic signature that identifies neoantigen-reactive T cells in fresh human lung cancers. Cancer Cell 2022;40:479-493.e6. [Crossref] [PubMed]
- Sorin M, Karimi E, Rezanejad M, et al. Single-cell spatial landscape of immunotherapy response reveals mechanisms of CXCL13 enhanced antitumor immunity. J Immunother Cancer 2023;11:e005545. [Crossref] [PubMed]
- Pai JA, Hellmann MD, Sauter JL, et al. Lineage tracing reveals clonal progenitors and long-term persistence of tumor-specific T cells during immune checkpoint blockade. Cancer Cell 2023;41:776-790.e7. [Crossref] [PubMed]
- Legut M, Gajic Z, Guarino M, et al. A genome-scale screen for synthetic drivers of T cell proliferation. Nature 2022;603:728-35. [Crossref] [PubMed]
- Zeng L, Zhang L, Li L, et al. RNA sequencing identifies lung cancer lineage and facilitates drug repositioning. bioRxiv 2023. Available online: https://doi.org/
10.1101/2023.01.18.524544 - Yang M, Miao YR, Xie GY, et al. ICBatlas: A Comprehensive Resource for Depicting Immune Checkpoint Blockade Therapy Characteristics from Transcriptome Profiles. Cancer Immunol Res 2022;10:1398-406. [Crossref] [PubMed]
- Cristescu R, Nebozhyn M, Zhang C, et al. Transcriptomic Determinants of Response to Pembrolizumab Monotherapy across Solid Tumor Types. Clin Cancer Res 2022;28:1680-9. [Crossref] [PubMed]
- Lambrechts D, Wauters E, Boeckx B, et al. Phenotype molding of stromal cells in the lung tumor microenvironment. Nat Med 2018;24:1277-89. [Crossref] [PubMed]
- Zhang L, Zhang Y, Wang C, et al. Integrated single-cell RNA sequencing analysis reveals distinct cellular and transcriptional modules associated with survival in lung cancer. Signal Transduct Target Ther 2022;7:9. [Crossref] [PubMed]
- Leader AM, Grout JA, Maier BB, et al. Single-cell analysis of human non-small cell lung cancer lesions refines tumor classification and patient stratification. Cancer Cell 2021;39:1594-1609.e12. [Crossref] [PubMed]
- Liu B, Hu X, Feng K, et al. Temporal single-cell tracing reveals clonal revival and expansion of precursor exhausted T cells during anti-PD-1 therapy in lung cancer. Nat Cancer 2022;3:108-21. [Crossref] [PubMed]
- Gentles AJ, Hui AB, Feng W, et al. A human lung tumor microenvironment interactome identifies clinically relevant cell-type cross-talk. Genome Biol 2020;21:107. [Crossref] [PubMed]
- Combes AJ, Samad B, Tsui J, et al. Discovering dominant tumor immune archetypes in a pan-cancer census. Cell 2022;185:184-203.e19. [Crossref] [PubMed]
- Ravi A, Hellmann MD, Arniella MB, et al. Genomic and transcriptomic analysis of checkpoint blockade response in advanced non-small cell lung cancer. Nat Genet 2023;55:807-19. [Crossref] [PubMed]
- Cai L, Luo D, Yao B, et al. Systematic Analysis of Gene Expression in Lung Adenocarcinoma and Squamous Cell Carcinoma with a Case Study of FAM83A and FAM83B. Cancers (Basel) 2019;11:886. [Crossref] [PubMed]
- Goldman MJ, Craft B, Hastie M, et al. Visualizing and interpreting cancer genomics data via the Xena platform. Nat Biotechnol 2020;38:675-8. [Crossref] [PubMed]
- Cerami E, Gao J, Dogrusoz U, et al. The cBio cancer genomics portal: an open platform for exploring multidimensional cancer genomics data. Cancer Discov 2012;2:401-4. [Crossref] [PubMed]
- Mayakonda A, Lin DC, Assenov Y, et al. Maftools: efficient and comprehensive analysis of somatic variants in cancer. Genome Res 2018;28:1747-56. [Crossref] [PubMed]
- Butler A, Hoffman P, Smibert P, et al. Integrating single-cell transcriptomic data across different conditions, technologies, and species. Nat Biotechnol 2018;36:411-20. [Crossref] [PubMed]
- Efremova M, Vento-Tormo M, Teichmann SA, et al. CellPhoneDB: inferring cell-cell communication from combined expression of multi-subunit ligand-receptor complexes. Nat Protoc 2020;15:1484-506. [Crossref] [PubMed]
- Hänzelmann S, Castelo R, Guinney J. GSVA: gene set variation analysis for microarray and RNA-seq data. BMC Bioinformatics 2013;14:7. [Crossref] [PubMed]
- Ru B, Wong CN, Tong Y, et al. TISIDB: an integrated repository portal for tumor-immune system interactions. Bioinformatics 2019;35:4200-2. [Crossref] [PubMed]
- Sun D, Wang J, Han Y, et al. TISCH: a comprehensive web resource enabling interactive single-cell transcriptome visualization of tumor microenvironment. Nucleic Acids Res 2021;49:D1420-30. [Crossref] [PubMed]
- Fan Z, Chen R, Chen X. SpatialDB: a database for spatially resolved transcriptomes. Nucleic Acids Res 2020;48:D233-7. [Crossref] [PubMed]
- Tang Z, Kang B, Li C, et al. GEPIA2: an enhanced web server for large-scale expression profiling and interactive analysis. Nucleic Acids Res 2019;47:W556-60. [Crossref] [PubMed]
- Keenan AB, Torre D, Lachmann A, et al. ChEA3: transcription factor enrichment analysis by orthogonal omics integration. Nucleic Acids Res 2019;47:W212-24. [Crossref] [PubMed]
- Uhlen M, Zhang C, Lee S, et al. A pathology atlas of the human cancer transcriptome. Science 2017;357:eaan2507. [Crossref] [PubMed]
- Zheng L, Qin S, Si W, et al. Pan-cancer single-cell landscape of tumor-infiltrating T cells. Science 2021;374:abe6474. [Crossref] [PubMed]
- Li T, Lu H, Shen C, et al. Identification of epithelial stromal interaction 1 as a novel effector downstream of Krüppel-like factor 8 in breast cancer invasion and metastasis. Oncogene 2014;33:4746-55. [Crossref] [PubMed]
- Tian Y, Xu J, Chu Q, et al. A novel tumor mutational burden estimation model as a predictive and prognostic biomarker in NSCLC patients. BMC Med 2020;18:232. [Crossref] [PubMed]
- Roh W, Geffen Y, Cha H, et al. High-Resolution Profiling of Lung Adenocarcinoma Identifies Expression Subtypes with Specific Biomarkers and Clinically Relevant Vulnerabilities. Cancer Res 2022;82:3917-31. [Crossref] [PubMed]
- Beineke P, Fitch K, Tao H, et al. A whole blood gene expression-based signature for smoking status. BMC Med Genomics 2012;5:58. [Crossref] [PubMed]
- Subramanian A, Narayan R, Corsello SM, et al. A Next Generation Connectivity Map: L1000 Platform and the First 1,000,000 Profiles. Cell 2017;171:1437-1452.e17. [Crossref] [PubMed]
- Ruprecht B, Di Bernardo J, Wang Z, et al. A mass spectrometry-based proteome map of drug action in lung cancer cell lines. Nat Chem Biol 2020;16:1111-9. [Crossref] [PubMed]
- Rakaee M, Andersen S, Giannikou K, et al. Machine learning-based immune phenotypes correlate with STK11/KEAP1 co-mutations and prognosis in resectable NSCLC: a sub-study of the TNM-I trial. Ann Oncol 2023;34:578-88. [Crossref] [PubMed]
- Spiliotaki M, Neophytou CM, Vogazianos P, et al. Dynamic monitoring of PD-L1 and Ki67 in circulating tumor cells of metastatic non-small cell lung cancer patients treated with pembrolizumab. Mol Oncol 2023;17:792-809. [Crossref] [PubMed]
- Magen A, Hamon P, Fiaschi N, et al. Intratumoral dendritic cell-CD4(+) T helper cell niches enable CD8(+) T cell differentiation following PD-1 blockade in hepatocellular carcinoma. Nat Med 2023;29:1389-99. [Crossref] [PubMed]
- Mizukoshi E, Nakagawa H, Tamai T, et al. Peptide vaccine-treated, long-term surviving cancer patients harbor self-renewing tumor-specific CD8(+) T cells. Nat Commun 2022;13:3123. [Crossref] [PubMed]
- Chu Y, Dai E, Li Y, et al. Pan-cancer T cell atlas links a cellular stress response state to immunotherapy resistance. Nat Med 2023;29:1550-62. [Crossref] [PubMed]
- Wang F, Long J, Li L, et al. Single-cell and spatial transcriptome analysis reveals the cellular heterogeneity of liver metastatic colorectal cancer. Sci Adv 2023;9:eadf5464. [Crossref] [PubMed]
- Wang J, Hong J, Yang F, et al. A deficient MIF-CD74 signaling pathway may play an important role in immunotherapy-induced hyper-progressive disease. Cell Biol Toxicol 2023;39:1169-80. [Crossref] [PubMed]
- Wu L, Qin Y, Xia S, et al. Identification of Cyclin-Dependent Kinase 1 as a Novel Regulator of Type I Interferon Signaling in Systemic Lupus Erythematosus. Arthritis Rheumatol 2016;68:1222-32. [Crossref] [PubMed]
- Cingöz O, Goff SP. Cyclin-dependent kinase activity is required for type I interferon production. Proc Natl Acad Sci U S A 2018;115:E2950-9. [Crossref] [PubMed]
- Cheng D, Qiu K, Rao Y, et al. Proliferative exhausted CD8(+) T cells exacerbate long-lasting anti-tumor effects in human papillomavirus-positive head and neck squamous cell carcinoma. Elife 2023;12:e82705. [Crossref] [PubMed]
- Itkonen HM, Loda M, Mills IG. O-GlcNAc Transferase - An Auxiliary Factor or a Full-blown Oncogene? Mol Cancer Res 2021;19:555-64. [Crossref] [PubMed]
- Kim SK, Jung SM, Park KS, et al. Integrative analysis of lung molecular signatures reveals key drivers of idiopathic pulmonary fibrosis. BMC Pulm Med 2021;21:404. [Crossref] [PubMed]
- Litchfield K, Reading JL, Puttick C, et al. Meta-analysis of tumor- and T cell-intrinsic mechanisms of sensitization to checkpoint inhibition. Cell 2021;184:596-614.e14. [Crossref] [PubMed]
- Wang X, Cheng W, Zeng X, et al. EPSTI1 as an immune biomarker predicts the prognosis of patients with stage III colon cancer. Front Immunol 2022;13:987394. [Crossref] [PubMed]
- Hamid ARAH, Syadza YZ, Yausep OE, et al. The expression of stem cells markers and its effects on the propensity for recurrence and metastasis in bladder cancer: A systematic review. PLoS One 2023;18:e0269214. [Crossref] [PubMed]
- Fan M, Arai M, Tawada A, et al. Contrasting functions of the epithelial-stromal interaction 1 gene, in human oral and lung squamous cell cancers. Oncol Rep 2022;47:5. [Crossref] [PubMed]
- Ramaker RC, Lasseigne BN, Hardigan AA, et al. RNA sequencing-based cell proliferation analysis across 19 cancers identifies a subset of proliferation-informative cancers with a common survival signature. Oncotarget 2017;8:38668-81. [Crossref] [PubMed]
- Rocha P, Rodrigo M, Moliner L, et al. Pre-existing tumor host immunity characterization in resected non-small cell lung cancer. Lung Cancer 2023;181:107257. [Crossref] [PubMed]
(English Language Editor: J. Jones)