A decreased impact of air pollution on hospital pneumonia visits during COVID-19 outbreak in northeastern Thailand
Highlight box
Key findings
• The effect of PM2.5 and NO2 modeled significantly related to pneumonia. The PM2.5 model showed that the pneumonia risks from air pollution displayed declined and RRs of pneumonia and NO2 decreased significantly during the coronavirus disease 2019 (COVID-19) period.
What is known and what is new?
• The results suggested that the decreased relative risk (RR) of pneumonia could be achieved with reduced air pollutants.
• Air pollutant improvements and public health policies during the COVID-19 outbreak were evidence to support the risk reduction of respiratory illness.
What is the implication, and what should change now?
• This study’s result reinforces the current knowledge that air pollution has damaged human health, which could support air quality policies and measures to reduce respiratory health illnesses as witnessed during the COVID-19 period.
Introduction
Background
Respiratory diseases affect the lives of more than one billion people worldwide and are the principal cause of mortality and morbidity. Clinical pneumonia is usually caused by various infectious agents (viruses, bacteria, and other pathogens) but the etiology of pneumonia states that it is a complex disease caused by a combination of host, infection, and environmental factors (1). Other than the meteorological factors, such as temperature, humidity, sunshine, and wind speed, the study of Lam et al. [2018] have linked air pollutants to pneumonia (2). Many cities had serious air pollution situations that reported the effect of fine particulate matter (PM2.5) and O3 on hospital visits for pneumonia (3-5).
When coronavirus disease 2019 (COVID-19) started spreading in late 2019, it brought about changes in people’s behaviors, healthcare, and the environment. There was an increase in active protective measures and some of the regulations such as lockdowns, work from home, and the suspension of events had a direct impact on public health in many cities. Especially, the impact of lockdown periods significantly reduced hospital admissions, emergency department visits, and Intensive care unit (ICU) admission of many diseases (6,7). Moreover, in countries imposing lockdowns, a better quality of air pollutants along with particulate matter (PM10 and PM2.5) and gaseous pollutants (NO2, SO2, CO) was significantly witnessed (8,9). The positive effect of the environmental changes on human health during the COVID-19 pandemic has also been seen in many cities. The improvements in air quality since the lockdown may be an actual cause of the reduction in admissions of asthma and chronic obstructive pulmonary disease (COPD) (10,11). Additionally, Ambika et al. [2021] observed that a significant decrease in particulate matter and health risk (cancer risk, acute, and chronic health index) during the lockdown is due to a corresponding decrement in air pollution in India (12).
Rationale and knowledge gap
Changes in the ambient air pollutants provided a rare opportunity to investigate the change in health risk. Interestingly, no study has evaluated the air pollution effect on respiratory diseases, especially pneumonia before and during the COVID-19 outbreak in Thailand. Khon Kaen is a province to keep an eye on in Thailand. This province and its vicinity have had air pollution problems and a high level of PM2.5 during harvesting season every year. Between 2018 and 2019, the annual average of PM2.5 was higher than Bangkok (capital) and Chiang Mai (13). Parallel to the incidence of pneumonia in this province, pneumonia incidence was in the top five leading rates in Thailand from 2017–2019 (14). Therefore, this was a unique research opportunity to assess the effects on the risk of pneumonia resulted from the changes in air pollution before vs. during the COVID-19 outbreak.
Objective
The aim of this study is to evaluate the association between short-term exposure to air pollutants and the count of hospital visits with pneumonia through the generalized additive model (GAM) method. We comprehensively explained the changes in air pollutants during the COVID-19 outbreak in 2020–2021 compared to those before the outbreak in 2018–2019 and estimated the relative risk (RR) attributable to the air pollutant variation in Khon Kaen, one of the most polluted provinces in Thailand. The findings of this study provided details on the dangers of air pollution and can be utilized as epidemiological evidence to demonstrate the risk factors for respiratory ailments. We present this article in accordance with the STROBE reporting checklist (available at https://jtd.amegroups.com/article/view/10.21037/jtd-23-1051/rc).
Methods
Study area
Khon Kaen, a center of the mid-northeastern region of Thailand, is connected to 9 provinces with an area of 10,886 sq.km. In 2021, its population was 1.79 million, with a higher proportion of females than males (50.9% vs. 49.1%). Classifications of age group were as follows: 0–12 years old (12.8%), 13–24 years old (14.6%), 25–44 years old (29.5%), 45–64 years old (30.2%), and above 65 years old (12.9%). The province is known as a great educational province surrounded by business districts resulting in the traffic congestion in large areas. The major industries were found in the non-metallic, metal, food, and transportation. There were some air pollution sources from productions of power, ethanol, natural gas, industrial rock, mineral deposit extraction, and a center for the distribution of fuel and gas for northeastern region of Thailand. Moreover, this city has a large agricultural industry with an agricultural area of 6,752 km2 (62% of the total area). There have been problems with burning agricultural waste. Therefore, the province has had air pollution problems and high level of PM2.5 during harvesting season for decades (13). Overall, the area of this study covered 50 kilometers around a selected air quality monitoring station as shown in Figure 1. People who stayed within 50 km of 14 districts in Khon Kaen province and 4 districts in Maha Sarakham province around the station were enrolled in this study.
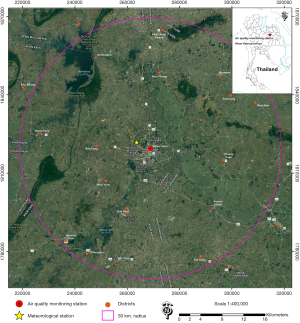
Data collection
Air pollution data were retrieved from the Thai Pollution Control Department (PCD). The daily average calculation, hourly measurements of each pollutant must be available more than 75% each day for the whole 4-year period of analysis otherwise it was excluded. Estimated daily air pollutant concentrations of PM10, PM2.5, and NO2 were calculated for the 24-hour mean and daily O3 was calculated for the maximum 8-hour rolling mean regarding PCD standard metrics. The ambient temperature and relative humidity were provided by Thai Meteorological Department, and the station is located in Khon Kaen center as shown in Figure 1. Their daily averages were also used for statistical analysis to control weather effects. Lastly, daily pneumonia hospital admitted cases were obtained from the Information and Communication Technology Center, Ministry of Public Health from 1st January 2017 to 31st December 2021. The diagnosis of pneumonia was mainly based on codes of International Classification of Disease, 10th edition (ICD-10: J10-18) together with the demographic information of gender, address, date of birth, and date of diagnosis.
Statistical analysis
Descriptive analysis and regression models were used to test different hypotheses. To characterize cases and exposure, Mann-Whitney U test was used to compare differences of variables of hospital visits with pneumonia together with air pollution concentration and meteorological factors before the COVID-19 outbreak and during the COVID-19 outbreak. Spearman’s correlation was used to present the degree of correlation between air pollutants and meteorological variables.
Poison with GAM was employed to examine the association between the counts of hospitalization for pneumonia and a vector of air pollution variables pertaining to each day t (15). The confounder control time strata in the model specifications, nonlinear independent variables (temperature, humidity, and time) were fitted by smoothing spline function to control seasonality and long-term effects [temperature (3 degrees of freedom; df), relative humidity (3 df), and time (7 df per year)]. Also, a day of the week (DOW) and public holidays were the dummy variable to control for the short-term trend. A specified regression model and df to control the time-varying covariates, each variable was set in accordance with previous literature (3,5).
First, the single-pollutant model was performed to include one pollutant (PM10, PM2.5, NO2, and O3) as a predictor in the model for the hospital visits with pneumonia.
Second, as for effects of co-pollutants, to adjust for the potential confounding effect of interactions among air pollutants on pneumonia, the multi-pollutant model used the same model as the single-pollutant models to control the influence effect modification of various pollutants.
Third, to determine the acute, delay, and potential prolonged effects between the increase in air pollution and hospital visits with pneumonia, the models with different lag structures, including both single-day lag (e.g., lag 0 was the same day of air pollutant concentration and hospital visit, lag 1 was air pollutant concentration of the day before hospital visit) and cumulative-day lag (e.g., lag 0–1 was the 2-day averages of current and previous day concentrations of air pollutants) were fitted in both single- and multi-pollutant models. The results were expressed in terms of RR and 95% confidence interval (95% CI) with a 10 µg/m3 increase in the concentrations of air pollutants.
Finally, this study used the Z-test to compare the RR of pneumonia associated with air pollution before vs. during the COVID-19 outbreak for lockdown interaction testing (16). The mathematical was explained by , where and are natural logarithms of RRs before and during the COVID-19 outbreak, and and are their respective standard errors. All the analyses were conducted using mgcv, dlnm, and splines packages in R 4.1.3 (R Foundation for Statistical Computing, Vienna, Austria).
Ethical statement
The study was conducted in accordance with the Declaration of Helsinki (as revised in 2013). This study was reviewed by the graduate program, Faculty of Science, Chulalongkorn University, and was considered exempt from institutional review board approval, since this study used secondary data without any personal identifiers and did not contain confidential patient data. For this type of study, informed consent from the subjects is not required.
Results
Statistic summary of data
Total pneumonia cases, air pollutant levels, and meteorological variables were characterized in Table 1. For the air pollutant status, we noticed that during the outbreak, concentrations of PM10, PM2.5, and NO2 were lower as compared to those before the COVID-19 period. Especially PM2.5 was decreased significantly (P<0.001). Nevertheless, the 8-hour running average of O3 was quite higher during the COVID-19 outbreak (103.25 µg/m3) than before the outbreak (78.15 µg/m3) (P<0.001). The weather conditions were comparable in two periods, with the daily mean temperature and the relative humidity before the outbreak vs. during the outbreak (27.43 vs. 27.14 ℃ and 70.09% vs. 70.87% respectively). Both of the weather variables were no significant difference between the two periods. The daily average number of pneumonia cases was about 69 cases/day within a range of 15–165 cases/day. During the outbreak, it decreased to 60 [15–150] cases/day. Compared with that before the COVID-19 period, the average daily pneumonia case was significantly lower during the COVID-19 outbreak (P<0.001). However, in the adult group (18–64 years old), it increased during the outbreak period.
Table 1
Variables | Before COVID-19 outbreak | During COVID-19 outbreak | P value† | |||||||
---|---|---|---|---|---|---|---|---|---|---|
Mean (SD) | Min | Median | Max | Mean (SD) | Min | Median | Max | |||
Air pollutants (µg/m3) | ||||||||||
PM10 (24-hour mean) | 55.59 (26.09) | 17.17 | 48.62 | 163.67 | 54.71 (24.62) | 19.30 | 48.24 | 137.79 | 0.630 | |
PM2.5 (24-hour mean) | 32.61 (17.75) | 10.33 | 26.83 | 109.29 | 29.44 (15.99) | 9.042 | 24.23 | 87.50 | <0.001 | |
NO2 (24-hour mean) | 18.24 (10.22) | 2.22 | 16.69 | 68.97 | 17.63 (9.68) | 2.32 | 15.53 | 61.69 | 0.373 | |
O3 (8-hour mean) | 78.15 (59.60) | 3.27 | 64.75 | 242.21 | 103.25 (38.47) | 34.78 | 101.84 | 192.39 | <0.001 | |
Meteorological factors | ||||||||||
Temperature (℃) | 27.43 (2.57) | 17.54 | 27.66 | 34.20 | 27.14 (2.93) | 14.84 | 27.35 | 34.08 | 0.452 | |
Relative humidity (%) | 70.09 (11.72) | 30.50 | 70.10 | 99.12 | 70.87 (11.92) | 38.62 | 70.38 | 96.46 | 0.292 | |
Total pneumonia (cases/day) | 68.81 (23.01) | 15 | 68 | 165 | 60.27 (25.26) | 15 | 57 | 150 | <0.001 | |
Sex | ||||||||||
Male | 37.12 (12.67) | 8 | 37 | 86 | 33.53 (13.64) | 9 | 32 | 87 | <0.001 | |
Female | 31.71 (11.86) | 7 | 30 | 79 | 26.75 (12.99) | 4 | 25 | 74 | <0.001 | |
Age (years) | ||||||||||
0–17 | 18.69 (8.37) | 1 | 18 | 63 | 9.53 (7.88) | 0 | 8 | 51 | <0.001 | |
18–64 | 23.89 (9.47) | 2 | 24 | 60 | 26.61 (15.97) | 4 | 23 | 100 | 0.759 | |
≥65 | 26.25 (9.64) | 2 | 26 | 55 | 24.15 (9.56) | 5 | 23 | 64 | <0.001 |
†, Mann-Whitney U test. COVID-19, coronavirus disease 2019; SD, standard deviation.
Spearman correlation coefficient
Considering the correlation between air pollutants and weather measurements, in Figure 2 we found a strong correlation among PM10, PM2.5 and NO2 in both two periods. Spearman corresponding coefficients of PM10 and PM2.5 (r=0.98), PM10 and NO2 (r=0.80), NO2 and PM2.5 (r=0.79) during the COVID-19 outbreak were slightly higher than those before the COVID-19 period (r=0.96, 0.75, and 0.74, respectively). Moreover, less active emission sources and fuel change during the outbreak regarding changes in transportation mode and industry plants made PM10, PM2.5 and NO2 correlations with O3 greater positive levels (r=0.78, 0.79, and 0.61 respectively (P<0.001).
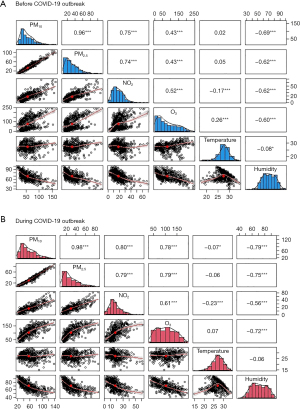
Single-pollutant analysis
With single-pollutant model analysis, a 10-µg/m3 increase in PM10, PM2.5, and NO2 except O3 showed increases in pneumonia effect in the period before the outbreak, and it was noticeable that their RRs were all significant as early as at single-day lag 0, RR =1.016 (95% CI: 1.003 to 1.030), RR =1.036 (95% CI: 1.014 to 1.058), and RR =1.046 (95% CI: 1.015 to 1.078), respectively (Figure 3A). No association was found during the outbreak. A similar result was seen with cumulative-day exposure lag as no increased air pollutants-pneumonia association was found during the outbreak (Figure 3B). However, PM10, PM2.5, and NO2 except O3 showed increasingly pronounced risks detected through all cumulative-day lags. RRs kept increasing from lag 0–1 to lag 0–5 then dropping at lag 0–6 (P<0.05) only in the period before the outbreak. The greatest pneumonia effect was associated with PM2.5 at lag 0–5 (P<0.05). Although O3 had positive correlation coefficients with PM10, PM2.5, and NO2 in both periods, it did not affect pneumonia while other pollutants did.
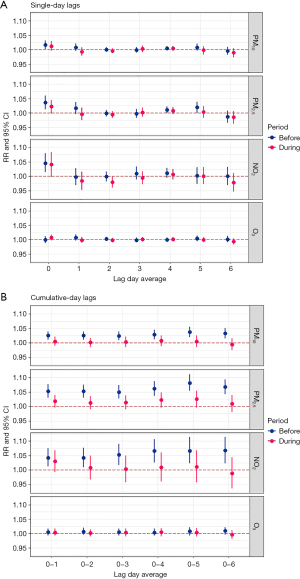
Multi-pollutant analysis
In multi-pollutant analyses (Figure 4), PM2.5 significantly increased pneumonia risk in both periods, before the COVID-19 outbreak and during the COVID-19 outbreak. RRs before the outbreak were higher than those during the outbreak although they were not statistically higher regarding lockdown interaction testing. For single-day exposure lag, the pneumonia risk was statistically significant at lag 0 (RR =1.078, 95% CI: 1.004 to 1.157), lag 4 (RR =1.054, 95% CI: 1.011 to 1.098) and lag 5 (RR =1.090, 95% CI: 1.021 to 1.165) before the outbreak vs. lag 1 (RR =1.064, 95% CI: 1.002 to 1.130) during the outbreak. Similarly, greater PM2.5-induced pneumonia RRs were observed before the outbreak vs. during the outbreak through continuous cumulative exposure as following, lag 0–1 (RR =1.132, 95% CI: 1.056 to 1.214 vs. RR =1.098, 95% CI: 1.011 to 1.192), lag 0–2 (RR =1.138, 95% CI: 1.059 to 1.222 vs. RR =1.119, 95% CI: 1.021 to 1.225 ), lag 0–3 (RR =1.144, 95% CI: 1.062 to 1.232 vs. RR =1.113, 95% CI: 1.010 to 1.226), lag 0–4 (RR =1.205, 95% CI: 1.114 to 1.304 vs. RR =1.134, 95% CI: 1.023 to 1.257), lag 0–5 (RR =1.314, 95% CI: 1.200 to 1.439 vs. RR =1.201, 95% CI: 1.073 to 1.344), lag 0–6 (RR =1.298, 95% CI: 1.191 to 1.414 vs. RR =1.217, 95% CI: 1.087 to 1.363). Furthermore, we observed that NO2 daily exposure did not post pneumonia risk with single-day exposure lags, but it significantly posted an increased risk for those inhaling NO2 cumulative day exposure before the COVID-19 outbreak at lag 0–3 (RR =1.050, 95% CI: 1.001 to 1.100). It was significantly greater than that risk during the outbreak per lockdown interaction P=0.016. Additionally, NO2 in cumulative-day lags (lag 0–4, lag 0–5, and lag 0–6) was a statistical difference in risk estimates between the two periods.
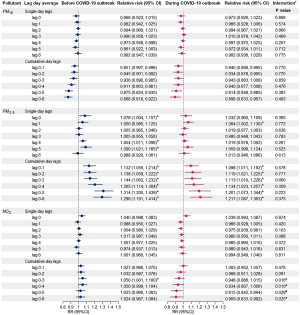
Discussion
Key findings
This time-series study evaluated the association between short-term exposure to air pollutants (PM10, PM2.5, NO2, and O3) and pneumonia before vs. during the COVID-19 outbreak using GAM. The finding indicated that the effect of PM10, PM2.5, and NO2 were associated with hospital pneumonia visits. As highlighted in the multi-pollutant model, this study showed that the RRs of pneumonia associated with PM2.5 increased during the COVID-19 outbreak and the pneumonia risk from NO2 was significantly greater during before-COVID-19 outbreak than during the outbreak.
Explanations of findings
Air pollutant concentrations
The coronavirus has spread in the world and has an enormous impact on not only people but also the environment. In this regard, we observed that the concentrations of PM2.5, PM10, and NO2 during the COVID-19 period were lower than those before the COVID-19 period. Especially PM2.5 was statistically significantly lower, similar to previous studies (17,18). During the full lockdown, residents were restricted to go outside and reduced outdoor activities, leading to a substantial decrease in vehicle and public transportation usage. PM came from car exhaust fumes, coal combustion, industrial chimneys, dust from construction sites, and the interaction of SOX and NOX in the air. When transportation and industrial production were reduced due to epidemic control actions, followed are reductions in air pollutants (19). While, a high concentration of O3 also increased in Khon Kaen, similar to other recent studies (20,21). The mechanisms of the increase in O3 were correlated between weather conditions, restrictive measures and reduction of air pollution (NOx and VOC) (22,23).
Hospital visits with pneumonia
Thailand was the first nation to report a verified COVID-19 case outside of China. Thailand’s government enacted the Public Administration in Emergency Situation. A national lockdown policy, almost all hospitals had limited health care service, for example, by solely opening some wards, sending medication to the patient’s house rather than having them picked it up at the hospital, and use the phone to contact a doctor, which resulted in a drop in the daily number of patients during an early lock down phase but alleviating for following phases. The result of this study found that the number of pneumonia cases in Khon Kaen from 2020 to 2021 during the outbreak was lower than in the earlier 2 years [2018–2019]. Similarly, in countries imposing lockdowns, a reduction in hospitalization for many diseases has presented. In Korea, the investigation of Huh et al. [2021] highlighted the decrease in hospital admissions for respiratory diseases during the COVID-19 pandemic. Hospital admissions reduced by 0.47 times for pneumonia, pneumonia (patients with COPD) reduced by 0.54 times, and pneumonia (patients with asthma) reduced by 0.38 times when comparison with 2016–2019 (24). Additionally, the study of Bodilsen et al. [2021] and Dias et al. [2022] showed a decrease in hospitalizations and mortality due to pneumonia during the lockdown intervention period (25,26). These studies also suggested that a decrease in pneumonia may be related to the prevention and control measures during the COVID-19 pandemic. Because of the difficulty accessing hospital services due to strict quarantine measures, together with hospital avoidance behavior during COVID-19, many patients preferred staying more at home and may have delayed treatment resulted from concerns about being infected by the COVID-19 virus in the community and hospitals.
The association between air pollution and pneumonia in single-pollutant analysis
Single-pollutant models showed that the short-term effect of PM10, PM2.5, and NO2 in single-day lags was associated with hospital visits with pneumonia at lag day 0 before the COVID-19 period. Similarly, the study in Turkey, Tasci et al. [2018] showed that atmospheric PM10, and NOX concentrations were associated with the number of elderly patients admitted to the hospital due to pneumonia on day 0 and the previous 1–4 days (27). Another report had also highlighted the changes in PM2.5 and PM10 that were associated with the increase in the hospitalization of childhood pneumonia at lag day 0 and lag 0–2 (28). For all analyses of PM10, PM2.5, and NO2 in single-day lags, the strongest effects were observed on the same day as the day going for a hospital visit. Although pneumonia is a type of lower respiratory tract infection, these studies support that the effect of air pollution on pneumonia tends to be short-term risk. It shows harmful to human health and this further implies the need of protecting short-term risk of respiratory health.
The association between air pollution and pneumonia in multi-pollutant analysis
RRs derived by multi-pollutant analyses of the combinations of PM10, PM2.5, NO2, and O3 based on the positive single-pollutant model provided different results for these pollutants. PM10, PM2.5, and NO2 in single-pollutant model was significantly associated with pneumonia. Nevertheless, only PM2.5 and NO2 had a significant effect on pneumonia in multi-pollutant model. In general, fine particulate matter is not a single pollutant, but rather is a mixture of many chemical species. PM2.5, after adjusting for PM10, NO2, and O3, was strongly significantly associated with pneumonia. Similarly, Wang et al. [2021] found that PM2.5 had an adverse effect on pneumonia after adjusting for meteorological factors and other pollutants (28). Epidemiological confirmation, the impact of PM2.5 cause damage to the respiratory epithelial cells. The evidence presented that PM2.5 exposure has adverse consequences for precipitate inflammatory and tissue remodeling changes in human lungs (29). Additionally, Schulze et al. [2017] tried to identify the mechanism of PM2.5 that interferes with alveolar macrophages and pulmonary epithelial cells and stimulates the release of cytokines that the respiratory tract could also activate inflammatory (30).
NO2 showed a result similar to PM2.5, the significance of NO2 remained strong after adjusting for PM10, PM2.5, and O3 at lag 0–3 before the COVID-19 outbreak. Another study highlighted that NO2 was significantly associated with an increased risk of hospital admissions for pneumonia after adjusting for PM10 and SO2 (31). In terms of toxicology, NO2 might damage macrophages, and natural killer cells in the respiratory tract related to decreased mucociliary clearance and vulnerable respiratory epithelium (32). Laboratory studies have shown that exposure to PM and NO2 significantly affects the susceptibility of respiratory epithelial and increased susceptibility to both bacterial and viral infection (33,34). These studies were evidence to support our finding that PM2.5 and NO2 were significantly associated with the number of hospital visits with pneumonia in Khon Kaen.
Comparison between two periods
During the COVID-19 outbreak, single- and multi-pollutant model results showed that the RR of air pollution displayed declined. As a result of this study, there was a lower RR of PM2.5 as compared to pre-COVID-19 period in Khon Kaen, Thailand. Supportively, a study by Bherwani et al. [2021], PM2.5 was found to decrease in 2020 for Brazil, India, Iran, Kenya, Malaysia, Mexico, Pakistan, Peru, Sri Lanka, and Thailand and was compared with year 2019 to calculate the reduced health burden during lockdown period. Interestingly, total morbidity (respiratory diseases, cardiovascular diseases, and COPD) cases showed a reduction of 4.7% for Thailand (35). In China, the spread of the COVID-19 pandemic brought about changes in air quality and its health impacts. Chen et al. [2021] also observed health burden attributable to a significant decrease air pollutant and the positive values of mortality of all causes, cardiovascular, and respiratory disease indicated the health gains from the PM2.5 improvement in 2020 compared with same period in 2018–2019 (36).
NO2 daily exposure before the COVID-19 outbreak was significantly greater than that risk during the outbreak in cumulative-day lags (lag 0–3, lag 0–4, lag 0–5, and lag 0–6). A previous study in Grenoble, France, Aix et al. [2022] tried to determine the lower concentrations of NO2, O3, PM2.5, and PM10 and how they affected human health compared to the 2020 period with a reference period 2015–2019. Aix et al. found that PM2.5 and NO2 associated with the highest health risk reductions and reductions in short-term risks were related to reductions in NO2 (−1.5% in hospitalizations for respiratory causes) (20). Many above studies investigated the effect of air pollutants and concluded that PM2.5 and NO2 associated with the risk reduction of hospitalizations for respiratory causes. Researchers have agreed for the reason that the decreased sensitivity of respiratory illness might be the response to air pollution changes during the COVID-19 lockdown.
Another critical aspect, different effect sizes in the two periods may also be attributed to the different population behavior and intervention measures due to the spread of COVID-19 disease. Limiting social interaction, hand hygiene, and self-protective equipment such as mask-wearing during the pandemic could have helped prevent some of the incidence of respiratory diseases (37,38). Some of these regulations (e.g., stay-at-home measure, lockdowns or curfews, limiting travel, and restrictions on social gatherings) which restricted the behaviors of individuals and reduced human activity had a direct impact on public health. Since people spent less time outdoors, it could reduce or make people avoid exposing to ambient air pollution. As with previous findings, Ko et al. [2023] tried to clarify the impact of the COVID-19 on a marked reduction in non-COVID pneumonia in Hong Kong. PM and influenza rates were mediators for changes in hospital admissions of non-COVID pneumonia before and during the COVID-19 pandemic (11% and 52%, respectively). The study provided supplementary evidence that mask-wearing rates were associated with respiratory illness. However, the report of Ko et al. did not assess the mask-wearing rates that would contribute to the pneumonia in the study (39). Most literatures in this study did not include the patient’s factors in the model analyses. Some studies suggested that an increase in these factors (mask-wearing rate and social distancing measures) might be contributed for reduced risk of diseases. Principally, it may be assumed that interventions to prevent COVID-19, in conjunction with improved air quality and changes in human behavior have reduced the risk of pneumonia.
Strengths and limitations
To the best of our knowledge, this is the first study that evaluated the association between air pollution and pneumonia comparing before vs. during the COVID-19 outbreak in Thailand. Using a time-series design, the effect of air pollutants modeled had significantly related to pneumonia. As the result, we identified decreased RR of pneumonia and reduced PM2.5, and NO2 concentrations during the COVID-19 outbreak. The additional explanations for this study comprehensively explained that the environmental improvements and public health policies were effective in lowering associated pneumonia risk.
The limitations of this study should be acknowledged. First, the modelling parameters were limited because of the integrity of each air pollution data as it is possible that other air pollutants might have influenced pneumonia illness. Second, we directly applied the ambient air pollutant concentrations to represent people’s exposure levels. In fact, we cannot truly quantify the decreased numbers of hospital visits with pneumonia associated with the reduced air pollution because of the difference of human health behaviors between the two periods. Nevertheless, the national lockdown was associated with the limitation of human flow. Protective measures started to rise sharply during the COVID-19 outbreak and stayed at a high level during the entire epidemic period, impacted respiratory protection in two periods that were independent. Due to the collinearity in modelling the hospitalization with mask-wearing rate and other factors such as behavior changes in the period of before and during the COVID-19 outbreak, we could not conclude what factors mediate the change in pneumonia hospitalization. Moreover, as a limitation of the time-series model purely based on variable counts or values per day, and thus individual patient characteristics cannot be fitted. Therefore, we could not consider changes in people’s behaviors and community hygienic measures in the model. Future study design such as case-cross over modelling should be considered for the patient’s factors or existing respiratory diseases, as well as for other regions of Thailand where air pollution sources are different.
Implications and actions needed
As mentioned above, the key finding of this study has shown that the respiratory health impacts were related to air pollutants. PM and NO2 model showed the association between hospital visits with pneumonia. Additionally, pneumonia risks of air pollution displayed declined during the COVID-19 period. A better quality of air pollutants along with public health policies during the pandemic was evidence to support the changes in risks of pneumonia. The findings of this study support raising awareness about measures and policies to preserve the air quality to decrease respiratory ailments.
Conclusions
The effect of PM and NO2 modeled significantly related to pneumonia in Khon Kaen, Thailand. Specifically, our findings showed that the influence of air pollutants on pneumonia was higher through cumulative exposure days before the COVID-19 outbreak than during the outbreak. This was consistent with several studies showing greater decreases in air pollutants concentrations because of the control of the COVID-19 measures, which could provide more useful information for environmental policies and measurements to reduce the emission sources of air pollution. The human health benefits can be achieved if control measures are taken to reduce air pollution.
Acknowledgments
The authors would like to acknowledge the Thai PCD, Thai Meteorological Department, and Information and Communication Technology Center, Ministry of Public Health for providing the data for this study.
Funding: This work was supported by
Footnote
Reporting Checklist: The authors have completed the STROBE reporting checklist. Available at https://jtd.amegroups.com/article/view/10.21037/jtd-23-1051/rc
Data Sharing Statement: Available at https://jtd.amegroups.com/article/view/10.21037/jtd-23-1051/dss
Peer Review File: Available at https://jtd.amegroups.com/article/view/10.21037/jtd-23-1051/prf
Conflicts of Interest: Both authors have completed the ICMJE uniform disclosure form (available at https://jtd.amegroups.com/article/view/10.21037/jtd-23-1051/coif). The authors have no conflicts of interest to declare.
Ethical Statement: The authors are accountable for all aspects of the work in ensuring that questions related to the accuracy or integrity of any part of the work are appropriately investigated and resolved. The study was conducted in accordance with the Declaration of Helsinki (as revised in 2013). This study was reviewed by the graduate program, Faculty of Science, Chulalongkorn University, and was considered exempt from institutional review board approval, since this study used secondary data without any personal identifiers and did not contain confidential patient data. For this type of study, informed consent from the subjects is not required.
Open Access Statement: This is an Open Access article distributed in accordance with the Creative Commons Attribution-NonCommercial-NoDerivs 4.0 International License (CC BY-NC-ND 4.0), which permits the non-commercial replication and distribution of the article with the strict proviso that no changes or edits are made and the original work is properly cited (including links to both the formal publication through the relevant DOI and the license). See: https://creativecommons.org/licenses/by-nc-nd/4.0/.
References
- Quinton LJ, Walkey AJ, Mizgerd JP. Integrative Physiology of Pneumonia. Physiol Rev 2018;98:1417-64. [Crossref] [PubMed]
- Lam HC, Chan EY, Goggins WB 3rd. Comparison of short-term associations with meteorological variables between COPD and pneumonia hospitalization among the elderly in Hong Kong-a time-series study. Int J Biometeorol 2018;62:1447-60. [Crossref] [PubMed]
- Qiu H, Chuang KJ, Bai CH, et al. Association of ambient ozone with pneumonia hospital admissions in Hong Kong and Taipei: A tale of two Southeast Asian cities. Environ Int 2021;156:106634. [Crossref] [PubMed]
- Wu Z, Zhang S, Tang YT, et al. Indoor environment in relation to recurrent childhood pneumonia in Southern China. Build Environ 2020;172:106727.
- Tian Y, Wu Y, Liu H, et al. The impact of ambient ozone pollution on pneumonia: A nationwide time-series analysis. Environ Int 2020;136:105498. [Crossref] [PubMed]
- Kruizinga MD, Peeters D, van Veen M, et al. The impact of lockdown on pediatric ED visits and hospital admissions during the COVID19 pandemic: a multicenter analysis and review of the literature. Eur J Pediatr 2021;180:2271-9. [Crossref] [PubMed]
- Morello F, Bima P, Ferreri E, et al. After the first wave and beyond lockdown: long-lasting changes in emergency department visit number, characteristics, diag-noses, and hospital admissions. Intern Emerg Med 2021;16:1683-90. [Crossref] [PubMed]
- Briz-Redón Á, Belenguer-Sapiña C, Serrano-Aroca Á. Changes in air pollution during COVID-19 lockdown in Spain: A multi-city study. J Environ Sci (China) 2021;101:16-26. [Crossref] [PubMed]
- Venter ZS, Aunan K, Chowdhury S, et al. COVID-19 lockdowns cause global air pollution declines. Proc Natl Acad Sci U S A 2020;117:18984-90. [Crossref] [PubMed]
- Sigala I, Giannakas T, Giannakoulis VG, et al. Effect of COVID-19-Related Lock-down οn Hospital Admissions for Asthma and COPD Exacerbations: Associations with Air Pollution and Patient Characteristics. J Pers Med 2021;11:867. [Crossref] [PubMed]
- Quintyne KI, Kelly C, Sheridan A, et al. COVID-19 transport restrictions in Ireland: impact on air quality and respiratory hospital admissions. Public Health 2021;198:156-60. [Crossref] [PubMed]
- Ambika S, Basappa U, Singh A, et al. Impact of social lockdown due to COVID-19 on environmental and health risk indices in India. Environ Res 2021;196:110932. [Crossref] [PubMed]
- Sakunkoo P, Norat T. The Situation of Air Quality in Khon Kaen Province in 2018–2019, Crisis that Creates Opportunities. KKU Journal for Public Health Research [Internet] 2021;14:1–8. Available online: https://he01.tci-thaijo.org/index.php/kkujphr/article/view/251026/169641
- Division of Epidemiology. Annual Epidemiological Surveillance Report 2019 [In-ternet]. Nonthaburi: Division of Epidemiology, Department of Disease Control; 2019. 1–802 p.
- Dominici F, McDermott A, Zeger SL, et al. On the use of generalized additive models in time-series studies of air pollution and health. Am J Epidemiol 2002;156:193-203. [Crossref] [PubMed]
- Altman DG, Bland JM. Interaction revisited: the difference between two estimates. BMJ 2003;326:219. [Crossref] [PubMed]
- Dey T, Tyagi P, Sabath MB, et al. Counterfactual time series analysis of short-term change in air pollution following the COVID-19 state of emergency in the United States. Sci Rep 2021;11:23517. [Crossref] [PubMed]
- Fu F, Purvis-Roberts KL, Williams B. Impact of the covid-19 pandemic lockdown on air pollution in 20 major cities around the world. Atmosphere (Basel) 2020;11:1189.
- Piccoli A, Agresti V, Balzarini A, et al. Modeling the effect of COVID-19 lockdown on mobility and NO2 concentration in the lombardy region. Atmosphere (Basel) 2020;11:1319.
- Aix ML, Petit P, Bicout DJ. Air pollution and health impacts during the COVID-19 lockdowns in Grenoble, France. Environ Pollut 2022;303:119134. [Crossref] [PubMed]
- Li J, Tartarini F. Changes in air quality during the COVID-19 lockdown in singapore and associations with human mobility trends. Aerosol Air Qual Res 2020;20:1748-58.
- Tobías A, Carnerero C, Reche C, et al. Changes in air quality during the lockdown in Barcelona (Spain) one month into the SARS-CoV-2 epidemic. Sci Total Environ 2020;726:138540. [Crossref] [PubMed]
- Venter ZS, Barton DN, Gundersen V, et al. Urban nature in a time of crisis: Recrea-tional use of green space increases during the COVID-19 outbreak in Oslo, Norway. Environ Res Lett 2020;15:104705.
- Huh K, Kim YE, Ji W, et al. Decrease in hospital admissions for respiratory diseases during the COVID-19 pandemic: a nationwide claims study. Thorax 2021;76:939-41. [Crossref] [PubMed]
- Bodilsen J, Nielsen PB, Søgaard M, et al. Hospital admission and mortality rates for non-covid diseases in Denmark during covid-19 pandemic: nationwide population based cohort study. BMJ 2021;373: [Crossref] [PubMed]
- Dias CF, Sarria EE, Scheffel C, et al. COVID-19 Prevention Policies Reduce Pediatric Hospital Mortality Due to Community-acquired Pneumonia. Arch Bronconeumol 2022;58:197-9. [Crossref] [PubMed]
- Tasci SS, Kavalci C, Kayipmaz AE. Relationship of Meteorological and Air Pollution Parameters with Pneumonia in Elderly Patients. Emerg Med Int 2018;2018:4183203. [Crossref] [PubMed]
- Wang X, Xu Z, Su H, et al. Ambient particulate matter (PM1, PM2.5, PM10) and childhood pneumonia: The smaller particle, the greater short-term impact?. Sci Total Environ 2021;772:145509. [Crossref] [PubMed]
- Pinkerton KE, Green FH, Saiki C, et al. Distribution of particulate matter and tissue remodeling in the human lung. Environ Health Perspect 2000;108:1063-9. [Crossref] [PubMed]
- Schulze F, Gao X, Virzonis D, et al. Air Quality Effects on Human Health and Ap-proaches for Its Assessment through Microfluidic Chips. Genes (Basel) 2017;8:244. [Crossref] [PubMed]
- Cheng CY, Cheng SY, Chen CC, et al. Ambient air pollution is associated with pe-diatric pneumonia: a time-stratified case-crossover study in an urban area. Environ Health 2019;18:77. [Crossref] [PubMed]
- Frampton MW, Boscia J, Roberts NJ Jr, et al. Nitrogen dioxide exposure: effects on airway and blood cells. Am J Physiol Lung Cell Mol Physiol 2002;282:L155-L165. [Crossref] [PubMed]
- Chauhan AJ, Johnston SL. Air pollution and infection in respiratory illness. Br Med Bull 2003;68:95-112. [Crossref] [PubMed]
- Olivieri D, Scoditti E. Impact of environmental factors on lung defences. Eur Respir Rev 2005;14:51-6.
- Bherwani H, Kumar S, Musugu K, et al. Assessment and valuation of health impacts of fine particulate matter during COVID-19 lockdown: a comprehensive study of tropical and sub tropical countries. Environ Sci Pollut Res Int 2021;28:44522-37. [Crossref] [PubMed]
- Chen G, Tao J, Wang J, et al. Reduction of air pollutants and associated mortality during and after the COVID-19 lockdown in China: Impacts and implications. Envi-ron Res 2021;200:111457. [Crossref] [PubMed]
- Hatoun J, Correa ET, Donahue SMA, et al. Social Distancing for COVID-19 and Diagnoses of Other Infectious Diseases in Children. Pediatrics 2020;146:e2020006460. [Crossref] [PubMed]
- Hsieh CC, Lin CH, Wang WYC, et al. The Outcome and Implications of Public Pre-cautionary Measures in Taiwan-Declining Respiratory Disease Cases in the COVID-19 Pandemic. Int J Environ Res Public Health 2020;17:4877. [Crossref] [PubMed]
- Ko FWS, Lau LHS, Ng SS, et al. Respiratory admissions before and during the COVID-19 pandemic with mediation analysis of air pollutants, mask-wearing and influenza rates. Respirology 2023;28:47-55. [Crossref] [PubMed]