Serum proteomics identifies novel diagnostic biomarkers for asthma in preschool children
Highlight box
Key findings
• An asthma diagnostic model for preschool children based on IGFALS was built and evaluated.
What is known and what is new?
• The diagnosis of childhood asthma remains challenging, and there is an urgent need to develop new diagnostic methods.
• We adopted the data-independent acquisition proteomics method to screen asthma diagnostic biomarker for children.
What is the implication, and what should change now?
• Our results provide insight into the pathophysiology of asthma. We believe that the diagnostic model will facilitate clinical decision-making regarding asthma in preschool children.
Introduction
Background
Asthma is the most common chronic disease in childhood and is characterized by airway hyperresponsiveness, reversible airway obstruction, and chronic airway inflammation (1,2). The rates of asthma morbidity and hospitalization are higher in preschool-aged children than older individuals (3). The lung function trajectory is established in childhood, and airway remodelling related to asthma develops before 3 years of age (4-7). Therefore, the preschool years are a critical time for asthma intervention (8), and accurate recognition and diagnosis of asthma in preschoolers may be helpful to understand asthma symptoms and improve treatment adherence (9).
Rationale and knowledge gap
Because asthma symptoms are recurrent and fluctuant, its diagnosis in children remains challenging, and effective stand-alone diagnostic tests are scarce (3,10,11). Diagnostic algorithms that combine the available tests, such as spirometry, bronchodilator reversibility tests, bronchial provocation tests to measure bronchial hyperresponsiveness, fractional exhaled nitric oxide (FeNO) and allergy tests, have recently been proposed by the National Institute for Health and Care Excellence and the Global Initiative for Asthma (GINA) (10-12). However, the diagnostic accuracy of these algorithms in asthma in preschool-aged children is uncertain (12-14). Therefore, better diagnostic methods for asthma are urgently required. To achieve this, new studies are required to identify biomarkers that can differentiate between asthmatic and healthy preschool-aged children, which can subsequently be incorporated into the diagnostic algorithms.
In asthma, certain proteins related to airway obstruction and inflammation are produced in tissue cells and secreted into the circulation (15). Thus, proteomics is a promising approach for the identification of potential asthma diagnostic biomarkers of asthma in preschoolers (16). Although proteomics has been used to analyze blood samples from asthmatic patients in previous studies (17-20), most of them used the data-dependent acquisition (DDA) mass spectrometry and focused on elderly children or adults. Data independent acquisition (DIA) mass spectrometry is a parallel-in-time acquisition method with better consistency and reproducibility than DDA, and is especially applicable to large sample cohort proteomics studies (21-23).
Previous studies have identified several asthma protein biomarkers. For example, Nieto-Fontarigo et al. found that serum IGFALS is a biomarker of allergic asthma in adults (20). Zamora-Mendoza et al. identified that salivary interleukin (IL)-8 and IL-10 are biomarkers of childhood asthma (24). Another study revealed that sputum LXA4 could discriminate children with severe asthma from those with intermittent asthma (25). Moreover, C7, C3, C4, α-1-antitrypsin, PDE7, arginase, UK16 binding protein, phospholipase D, and cyclooxygenase were found to differentially accumulate in the serum of patients with bronchial asthma and of healthy individuals through proteomics analysis (26). However, few studies have focused on biomarkers for asthma in preschoolers, as it is more difficult to diagnose asthma in this population.
Objective
In the present study, to obtain in-depth and reliable serum proteome data for asthma and identify potential diagnostic biomarkers of asthma in preschool children, we conducted Orbitrap-based DIA mass spectrometry serum proteomics analysis in asthmatic preschool children using the Orbitrap Exploris 480 platform. Combining bioinformatics and statistical analyses, we identified critical signalling pathways and candidate biomarker proteins related to asthma in preschool children. Finally, a preschool children asthma diagnostic model was constructed using a biomarker panel. We present this article in accordance with the STARD reporting checklist (available at https://jtd.amegroups.com/article/view/10.21037/jtd-23-974/rc).
Methods
Participants and serum sample collection
A set of 46 children were recruited from The Second Affiliated Hospital of Shaanxi University of Chinese Medicine between 2020 and 2021. The children were divided into three study cohorts: acute exacerbation of asthma [Asthma; n=17, children who were suffering from suddenly wheeze, cough, shortness of breath, chest tightness and other symptoms, being diagnosed as acute asthma exacerbations according to GINA 2019 (10)], convalescent asthma (Conva; n=19, children whose asthma symptoms disappeared with or without treatment and lung function returned to normal for more than 3 months), and healthy controls (Health; n=10). Children with a diagnosis of immune disease, chronic kidney disease, or other diseases affecting serum proteins were excluded. All clinical diagnoses followed the 2019 Global Initiative for Asthma guidelines (10). On the morning after the children were admitted to the hospital without drug treatment, blood samples (4 mL) were collected, placed at room temperature (22–25 ℃) in the dark for 1 hour, and then centrifuged at 3,000 rpm at 4 ℃ for 15 minutes to separate the serum. Finally, the serum was aliquoted and stored in a refrigerator at −80 ℃. The study was conducted in accordance with the Declaration of Helsinki (as revised in 2013). The research protocol was approved by the Ethics Committee of The Second Affiliated Hospital of Shaanxi University of Chinese Medicine (No. CYL-SQ202216). All of the participants’ guardians provided written informed consent.
Protein extraction and trypsin digestion
First, the serum samples were centrifuged at 12,000 g at 4 ℃ for 10 minutes to remove the cellular debris. Then, the supernatant was retained, and the protein concentration of the serum was determined using a bicinchoninic acid (BCA) kit (ThermoFisher Scientific, Waltham, MA, USA) according to the manufacturer’s instructions. Then, the serum protein solution was reduced with 5 mM dithiothreitol for 30 minutes at 56 ℃ and alkylated with 11 mM iodoacetamide for 15 minutes at room temperature in darkness. Trypsin was added at a ratio of 1:50 (trypsin/protein mass ratio) for the first overnight digestion and at a ratio of 1:100 for the second 4-hour digestion. The digested peptides were demineralized using C18 Ziptips (Millipore, Darmstadt, Germany), eluted with 0.1% TFA (Trifluoroacetic Acid) in 50–70% acetonitrile and then lyophilized and redissolved in 1% formic acid 5% acetonitrile. The iRT (indexed retention time) peptides (Biognosys, Schlieren, Switzerland) were spiked into the sample prior to LC-MS/MS (liquid chromatography-tandem mass spectrometry) analysis according to the manufacturer’s instructions.
High pH reversed-phase fractionation
The processed peptide solutions of subjects were equivalently pooled and further fractioned by high pH reversed-phase separation with a Dionex UHPLC (ultra performance liquid chromatography, ThermoFisher Scientific, Waltham, MA, USA) and Ethylene Bridged Hybrid C18 column (Waters) at 40 ℃ with a 0.2 mL/min flow and a 60 minutes ACN (acetonitrile) gradient (5–30%) in 5 mM ammonium formate (pH 10). Fractions were collected at 1 minute intervals and pooled into 12 fractions. Then, each fraction was lyophilized and redissolved in 1% formic acid 5% acetonitrile.
DDA LC-MS/MS analysis and spectral library generation
To generate the spectral library, DDA-MS analysis was employed and performed on an Easy-nLC 1200 UPLC (ultra performance liquid chromatography) system in tandem with an Orbitrap Exploris 480 mass spectrometer (ThermoFisher Scientific, Waltham, MA, USA). First, each peptide fraction was loaded onto the Easy-nLC 1200 UPLC system and separated within a 120-minute linear gradient from 95% solvent A (0.1% formic acid/2% acetonitrile/98% water) to 28% solvent B (0.1% formic acid/80% acetonitrile) at a flow rate of 250 nL/min at 50 ℃. The mass spectrometer was operated in the data-dependent mode. A full MS scan from 350 to 1500 m/z was acquired at high resolution R=120,000 (defined at m/z=400); MS/MS scans were performed at a resolution of 30,000 with an isolation window of 4 Da and higher energy collisional dissociation (HCD) fragmentation with collision energy (CE) of 30%±5%. Dynamic exclusion was set at 30 s.
The raw data were processed using the Pulsar search engine in Spectronaut X (Biognosys, Schlieren, Switzerland) and searched against the UniProt Homo sapiens proteome database within the default parameters to generate the spectral library. The digestion enzyme was specific trypsin enzyme with 2 missed specialized cleavages, the fixed modification was carbamidomethyl of cysteine, and the variable modification was oxidation of methionine. The iRTs were calculated from the iRT median of all DDA runs. Fragment ions for the targeted data analysis were selected from 300–1,800 m/z, minimal relative intensity was set as >5% and fragment ion number >3. The false discovery rate (FDR) was set as 1% for protein and peptide spectrum matches. Protein inference was performed using the ID Picker algorithm within the Spectronaut software.
DIA LC-MS/MS analysis
DIA-MS was performed using the same LC-MS system and the same LC linear gradient method as DDA-MS. For MS/MS acquisition, the DIA mode was set for 50 variable isolation windows according to the full width at half maximum (FWTH), and the specific window lists were constructed based on the respective DDA data of the pooled sample. The full scan was set as 1200,000 over the m/z range from 350 to 1,500, followed by DIA scans with resolution 30,000; CE: 30%±5%; AGC target: 1e6 and maximal injection time: 54 microseconds. The DIA raw files were processed with Spectronaut X (Biognosys, Schlieren, Switzerland) and the default parameters. The retention time prediction type was dynamic iRT, the correction factor was window 1, and interference correction on the MS2 level was enabled. Systematic variance was normalized by a local normalization strategy. The FDRs of peptide precursors and proteins were both estimated with the mProphet approach at a cut-off of 1%. The summed peak areas of the peptide MS2 fragment ions were calculated as the protein intensity. All results were filtered by the Q value and FDR 1% cut-off.
Bioinformatics and statistical analysis
All identified serum proteins were annotated using Gene Ontology (GO; https://geneontology.org/) and Kyoto Encyclopedia of Genes and Genomes (KEGG) databases (http://www.genome.jp/kegg/), and then the functional enrichment analysis of the differentially expressed proteins (DEPs) was performed by R package clusterProfiler with the over-representation analysis method. SIMCA 14 software (Umetrics AB, Sweden) was used for unsupervised PCA and supervised orthonormal partial least squares discriminant analysis (OPLS-DA) of the acquired proteome data. The significantly different proteins were identified using a criterion of FC >1.5 and Mann-Whitney U test P value <0.05. Heatmap analysis was performed using TBtools (https://github.com/CJ-Chen/TBtools). R (version 4.0.3) and SPSS (version 28) were used to perform functional enrichment and statistical analyses, such as the area under the curve (AUC). The significantly enriched GO functions and KEGG pathways were examined using Fisher’s exact test P value <0.05. All the P values obtained above were adjusted with FDR method by the function P.adjust in R.
Results
Clinical characteristics of the study patients
In total, 46 children were divided into three groups: acute exacerbation of asthma (Asthma; n=17), convalescent asthma (Conva; n=19), and healthy controls (Health; n=10). To identify the asthma biomarkers in preschoolers, 7, 10, and 7 preschoolers (aged <5 years) were included in the Asthma, Conva, and Health groups, respectively. The clinical characteristics of each group are displayed in Table 1 and table available at https://cdn.amegroups.cn/static/public/jtd-23-974-1.xlsx. As shown, the forced expiratory volume in one second (FEV1) of children with acute asthma was lower than that of children in Conva and Health groups. However, the FEV1/FVC (forced vital capacity) of children with acute asthma was higher than that of children in the Conva and Health groups (Table 1). The neutrophil counts were higher, and the lymphocyte count was lower, in the Asthma group compared with the Conva and Health groups (Table 1). The eosinophil count, FeNO, and immunoglobulin E (IgE) of children in the Asthma and Conva groups were significantly higher than those in the Health group (Table 1).
Table 1
Characteristics | Asthma (n=17) | Conva (n=19) | Health (n=10) | P value† |
---|---|---|---|---|
Age, years | 5.6 (2.3–12) | 4.88 (1.4–9) | 3.94 (2–6.6) | NA |
Sex (male/female) | 11/6 | 11/8 | 5/5 | NA |
Baseline treatment | ||||
ICS-LABA | 1 | 0 | 0 | NA |
ICS | 7 | 11 | 0 | NA |
OCS | 0 | 0 | 0 | NA |
Montelukast sodium | 1 | 4 | 0 | NA |
BMI (kg/m2) | 17.44 (12.94–23) | 16.51 (12.77–22.7) | 15.263 (12.5–17.1) | NA |
FEV1 (%) | 46.78 (11.6–101.8) | 64.74 (15.8–108.1) | 64.34 (31.5–133.6) | 0.3272 |
FEV1/FVC (%) | 64.43 (19.7–108.9) | 61.78 (19.5–101.4) | 59.55 (31.3–108.9) | 0.9843 |
Neutrophils (103 cells/μL) | 4.65 (0.71–10.94) | 3.55 (1.98–7.75) | 2.44 (0.78–5.95) | 0.03661 |
Lymphocytes (103 cells/μL) | 2.38 (1.09–3.65) | 3.55 (2.4–6.64) | 3.26 (0.77–5.24) | 0.0005432 |
Monocytes (103 cells/μL) | 0.63 (0.35–1.23) | 0.47 (0.26–1.2) | 0.61 (0.24–1.05) | 0.06703 |
Eosinophils (103 cells/μL) | 0.29 (0.01–0.65) | 0.33 (0.06–1.05) | 0.134 (0.04–0.3) | 0.03787 |
Basophils (103cells/μL) | 0.027 (0–0.08) | 0.028 (0.01–0.08) | 0.069 (0.01–0.44) | 0.6461 |
FeNO (ppb) | 20.06 (3.7–53) | 17.4 (2.9–63.5) | 11.02 (4.4–18.1) | 0.437 |
IgE (IU/mL) | 376.45 (3.5–1,409) | 230.49 (7.97–1,000) | 32.16 (3.5–127.02) | 0.005725 |
Data are presented as median (IQR) or number. †, Kruskal-Wallis test P value. ICS-LABA, inhaled corticosteroids-long-acting beta-agonist; ICS, inhaled corticosteroids; OCS, oral corticosteroids; BMI, body mass index; FEV1, forced expiratory volume in one second; FVC, forced vital capacity; FeNO, fractional exhaled nitric oxide; IgE, immunoglobulin E; IQR, interquartile range.
Summary of the proteomic discovery and functional alterations related to asthma
To analyze the serum proteome of asthmatic children, a total of 747 proteins were identified in 46 serum samples using DIA-MS proteome sequencing (Figure 1 and table available at https://cdn.amegroups.cn/static/public/jtd-23-974-2.xlsx). The principal component analysis (PCA) showed that the serum proteomes could discriminate between the three study groups (Figure 2A). To identify the asthma biomarkers in children, 49 significantly altered proteins [fold-change (FC) >1.5, Mann-Whitney U test P value <0.05 and FDR Q value <0.05] were identified between the Asthma and Health groups after data filtration (Figure 2B,2C; Table 2), such as IGFALS, TNXB, LCAT, MMRN1, IGFBP3, APOA1, MADCAM1, PKM, AMY2A, PZP, IGHV3-38, ABHD12B, ANTXR2, IGF1, and AGT. Meanwhile, 62 proteins were significantly differentially expressed between the Conva and Health groups (Figure 2C and table available at https://cdn.amegroups.cn/static/public/jtd-23-974-3.xlsx). Interestingly, there were 19 common DEPs between the Conva and Asthma groups compared with the Health group (Figure 2C). The cluster analysis indicated that these 19 common DEPs in the Asthma, Conva, and Health groups could be distinguished in an unsupervised clustering analysis (Figure 2D).
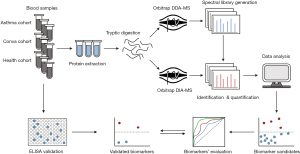
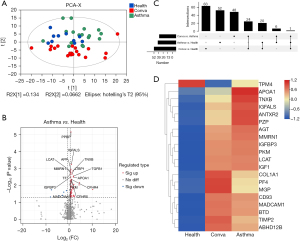
Table 2
Gene | Description | Conva/Asthma | Conva/Health | Asthma/Health | |||||
---|---|---|---|---|---|---|---|---|---|
FC | P value | FC | P value | FC | P value | ||||
MADCAM1 | Mucosal addressin cell adhesion molecule 1 | 1.09 | 7.13E–01 | 1.98 | 8.46E–03 | 1.82 | 5.33E–03 | ||
TPM4 | Tropomyosin alpha-4 chain | 0.43 | 4.72E–01 | 0.20 | 1.76E–03 | 0.46 | 2.03E–02 | ||
TNXB | Tenascin-X | 0.58 | 5.52E–01 | 1.87 | 9.07E–03 | 3.20 | 1.42E–03 | ||
IGFBP3 | Insulin-like growth factor-binding protein 3 | 0.97 | 7.07E–01 | 1.61 | 1.66E–03 | 1.66 | 3.20E–03 | ||
PKM | Pyruvate kinase | 0.96 | 7.54E–01 | 1.76 | 3.52E–02 | 1.82 | 5.58E–03 | ||
ANTXR2 | Anthrax toxin receptor | 0.66 | 6.61E–01 | 1.96 | 1.85E–02 | 2.99 | 1.51E–02 | ||
AGT | Angiotensinogen | 0.90 | 3.97E–01 | 1.75 | 1.13E–03 | 1.93 | 1.75E–02 | ||
COL1A1 | Collagen alpha-1(I) chain | 1.26 | 3.00E–01 | 1.92 | 4.08E–03 | 1.52 | 4.04E–02 | ||
APOA1 | Apolipoprotein A-I | 0.42 | 1.86E–01 | 1.54 | 3.52E–02 | 3.69 | 3.20E–03 | ||
PF4 | Platelet factor 4 | 1.60 | 1.14E–02 | 2.94 | 4.57E–06 | 1.84 | 1.75E–02 | ||
LCAT | Phosphatidylcholine-sterol acyltransferase | 0.99 | 7.78E–01 | 1.55 | 6.66E–03 | 1.57 | 1.42E–03 | ||
IGF1 | Insulin-like growth factor I | 0.95 | 8.45E–01 | 3.28 | 4.25E–03 | 3.44 | 1.66E–02 | ||
MGP | Matrix Gla protein | 1.49 | 1.65E–02 | 2.38 | 8.69E–05 | 1.60 | 2.68E–02 | ||
TIMP2 | Metalloproteinase inhibitor 2 | 1.20 | 3.50E–01 | 1.94 | 3.55E–03 | 1.62 | 2.31E–02 | ||
PZP | Pregnancy zone protein | 0.64 | 2.57E–01 | 2.11 | 4.45E–02 | 3.31 | 9.34E–03 | ||
IGFALS | Insulin-like growth factor-binding protein complex acid labile subunit | 0.80 | 1.21E–01 | 1.62 | 7.79E–03 | 2.03 | 5.74E–04 | ||
BTD | Biotinidase | 1.06 | 8.51E–01 | 1.73 | 7.79E–03 | 1.62 | 2.70E–02 | ||
MMRN1 | Multimerin-1 | 0.93 | 5.94E–01 | 1.62 | 3.96E–02 | 1.73 | 1.76E–03 | ||
ABHD12B | Protein ABHD12B | 1.27 | 1.56E–01 | 3.27 | 3.88E–04 | 2.57 | 1.29E–02 | ||
CD93 | Complement component C1q receptor | 1.08 | 9.75E–01 | 2.35 | 2.41E–02 | 2.18 | 2.03E–02 |
DEP, differentially expressed protein; FC, fold change.
GO, KEGG and Reactome enrichment analyses of the altered proteins
Based on GO annotation results (table available at https://cdn.amegroups.cn/static/public/jtd-23-974-4.xlsx), functional enrichment analysis of DEPs between the Asthma and Health groups was performed. Fisher’s exact test P value was used to determine the significance (Table S1). GO terms with a Fisher’s exact test P value <0.05 (Q value <0.05) were considered significantly enriched (Figure 3A). As shown, the DEPs were significantly enriched in immune system-related biological processes, such as the chemokine-mediated signaling pathway, positive regulation of leukocyte chemotaxis, regulation of granulocyte chemotaxis, positive regulation of leukocyte migration, positive regulation of neutrophil migration, regulation of neutrophil migration, regulation of neutrophil chemotaxis, regulation of leukocyte chemotaxis, and positive regulation of chemotaxis (Figure 3A). In terms of molecular functions, the DEPs were significantly enriched in immune-related functions, such as CXCR chemokine receptor binding, chemokine receptor binding, chemokine activity, and G-protein-coupled receptor binding (Figure 3A). The DEPs were significantly enriched in the cellular processes of sarcoplasmic reticulum, growth cone, site of polarized growth, and sarcoplasm terms (Figure 3A).
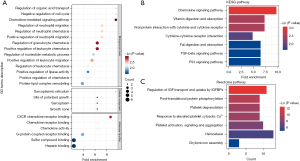
Next, KEGG pathway enrichment analysis was conducted (Tables S2). As expected, the DEPs were significantly enriched in immune-related pathways, such as the chemokine signaling pathway, viral protein interactions with cytokines and cytokine receptors, cytokine-cytokine receptor interaction, p53 signaling pathway, and TGF-beta signaling pathway. In addition, fat digestion and absorption, cholesterol metabolism, and vitamin digestion and absorption pathways were enriched (Figure 3B).
Besides, the Reactome pathway analysis were performed in the Reactome Pathway Database (https://reactome.org/) (Table S2). The regulation of insulin-like growth factor (IGF) transport and uptake by insulin-like growth factor binding proteins (IGFBPs), post-translational protein phosphorylation, platelet degranulation, response to elevated platelet cytosolic Ca2+, platelet activation, signaling and aggregation, hemostasis and chylomicron assembly reactome pathways were significantly enriched (Figure 3C).
Biomarker candidates for preschool children with asthma
A total of 50 DEPs were identified between Asthma and Health groups. Multivariate PCA revealed that the DEPs could distinguish between Asthma and Health subjects (Figure 4A and Figure S1A). To examine the potential usefulness of these proteins for distinguishing between Asthma and Health groups, OPLS-DA models were constructed (Figure 4B and Figure S1B). After 200 permutation tests, the R2 and Q2 of the OPLS-DA model of Asthma and Health groups were 0.53 and −0.45, respectively (Figure S1C), and those of the OPLS-DA model of preschool Asthma and Health groups were 0.766 and −0.491, respectively (Figure S1D), indicating that these models were well-fitted and had reliable predictive ability. The proteins MMP14, ABHD12B, PCYOX1, LTBP1, CFHR4, APOA1, IGHG4, ANG, and IGFALS significantly contributed to the discrimination between asthmatic and healthy children [predictive variable importance in projection (VIPpred) >1] and were considered biomarker candidates (Table 3 and Table S3). The relative expression levels of these candidate biomarkers are shown as boxplots in Figure 4C. Interestingly, these candidates except PCYOX1 showed significantly higher relative abundance in the Asthma cohort compared with the Health cohort. In particular, ANG, APOA1, IGFALS, and LTBP1 levels showed a gradually decreasing trend from Asthma to Conva and then to the Health cohorts (Figure 4C). Moreover, we performed a correlation analysis between these potential biomarkers and the clinical data of the patients using the spearman method (Figure S2). The results showed that APOA1 and IGFALS were significantly positively correlated with IgE, but negatively correlated with lymphocytes (Figure S2).
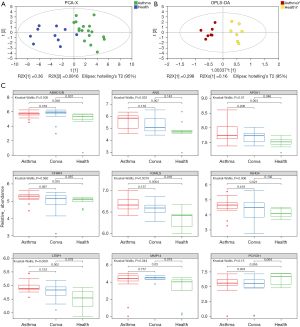
Table 3
Biomarkers | Relative abundance mean† | P value‡ | ||
---|---|---|---|---|
Health | Conva | Asthma | ||
IGFALS | 2.44E+06 | 3.96E+06 | 4.96E+06 | 5.00E–03 |
APOA1 | 3.40E+07 | 5.23E+07 | 1.26E+08 | 1.90E–03 |
LTBP1 | 4.10E+04 | 6.75E+04 | 9.73E+04 | 9.10E–02 |
ANG | 3.17E+05 | 4.57E+05 | 6.83E+05 | 2.50E–02 |
ABHD12B | 2.23E+05 | 7.29E+05 | 5.72E+05 | 5.00E–03 |
MMP14 | 1.07E+04 | 1.94E+04 | 3.60E+04 | 4.40E–02 |
†, relative abundance is LC-MS/MS intensity; ‡, P value means Kruskal Wallis test P value. LC-MS/MS, liquid chromatography-tandem mass spectrometry.
Development and evaluation of the biomarker-based diagnostic model for asthmatic preschoolers
To better present the differences in the abundance of candidate biomarkers among preschooler asthmatics, the heatmap of six candidate biomarkers was plotted using TBtools (Figure 5A). As shown, all proteins had significantly higher abundances in preschoolers with asthma than healthy preschoolers, especially ANG, APOA1, and IGFALS (Figure 5B). Next, the six proteins were subjected to receiver operating characteristic curve (ROC) analysis to evaluate their sensitivity and specificity in discriminating asthmatic individuals from healthy children (Table 4). Some candidate proteins were able to diagnose asthma with an AUC value >0.8 in all-aged children and preschool children. These proteins included IGFALS, APOA1, and LTBP1, suggesting their superior diagnostic value (Table 4). To construct an asthma diagnostic model, we performed two-logistic regression analysis and ROC analysis for the six protein biomarkers. As a result, IGFALS was proposed as a biomarker for childhood asthma (Table 4). For the diagnosis of asthma in children (preschool- and school-aged children), the AUC of the IGFALS model was 0.882 with asymptotic significance of 0.001 (Table 4). Moreover, the AUC of the IGFALS model was 0.959 (asymptotic significance: 0.004) for preschool asthma prediction (Figure 5C and Table 4). In order to verify the reliability of the biomarkers, we used ELISA (enzyme-linked immunosorbent assay) to measure the contents of IGFALS, LTBP1 and APOA1 in the validation set samples. And ROC analysis was carried out. In results, the AUC of the IGFALS model of ELISA data was 0.897 (Figure 5D), indicating that this diagnostic model based on IGFALS was useful for our cohort and may have significant diagnostic potential for the diagnosis of asthma in preschool-aged children.
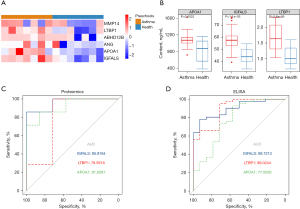
Table 4
Biomarkers | ROC (all age) | ROC (preschool) | |||||
---|---|---|---|---|---|---|---|
AUC | 95% CI | Asymptotic significance | AUC | 95% CI | Asymptotic significance | ||
IGFALS | 0.882 | 0.746–1.000 | 0.001 | 0.959 | 0.864–1.000 | 0.004 | |
APOA1 | 0.835 | 0.684–0.987 | 0.114 | 0.918 | 0.772–1.000 | 0.350 | |
LTBP1 | 0.847 | 0.676–1.000 | 0.199 | 0.796 | 0.518–1.000 | 0.108 | |
ANG | 0.794 | 0.581–1.000 | 0.496 | 0.816 | 0.533–1.000 | 0.784 | |
ABHD12B | 0.788 | 0.614–0.962 | 0.655 | 0.694 | 0.377–1.000 | 0.125 | |
MMP14 | 0.671 | 0.465–0.876 | 0.052 | 0.837 | 0.618–1.000 | 0.267 |
ROC, receiver operating characteristic curve; AUC, area under the curve; CI, confidence interval.
Discussion
Key findings
In our pilot study, we adopted the DIA-MS method to analyze the serum proteome signatures of children with acute and convalescent asthma. As a result, we identified 50 DEPs that could clearly separate asthmatic and healthy subjects. Furthermore, MMP14, ABHD12B, PCYOX1, LTBP1, CFHR4, APOA1, IGHG4, ANG, and IGFALS proteins, which made major contributions to asthma discrimination, were identified and considered candidate diagnostic biomarkers. Ultimately, a promising preschooler asthma diagnostic model based on IGFALS was constructed.
Strengths and limitations
Given that the diagnosis of asthma in children remains challenging, effective stand-alone diagnostic tests are scarce (3,10,11). We built a diagnostic model for asthma in preschoolers based on IGFALS levels. The AUCs of the IGFALS model indicated that this diagnostic model worked well and may have significant potential diagnostic value for the diagnosis of asthma in preschoolers. IGFALS may have significant clinical application applications and may facilitate clinical decision-making in preschooler asthmatic patients.
However, several limitations still exist in our current study. Firstly, the number of samples enrolled in the current study is still limited. Only a small cohort of preschool children with asthma was included in this study. Secondly, the serum samples applied in our current study were collected at the single-time point. Thirdly, our current study was a single centre research, and all samples were collected from Chinese Han population, reproducibility and accuracy in other populations require further investigation.
Comparison with similar researches
MS-based proteomic approaches are widely used for disease biomarker discovery (27-29). With regard to asthma, several serum proteomic studies have been conducted (15,20,30,31), but none have adopted the DIA-MS strategy or focused on preschool aged asthma (15,20,30,31), but none have adopted the DIA-MS strategy or focused on preschool aged asthma. In other papers, 103 (15) and 217 (20) proteins were identified in the serum by isobaric tags for relative and absolute quantitation (iTRAQ) (DDA-MS strategy) proteomics and 347 proteins were identified in plasma using a tandem mass tag (TMT) quantitative proteomics (DDA-MS strategy) (31). Here, we performed DIA-MS proteomics and obtained the largest asthma serum proteome of 747 proteins ever reported, reflecting the advantage of the DIA strategy over the DDA strategy in terms of the number of proteins identified.
Explanations of findings
Inflammation and airway remodelling, the main pathophysiological characteristics of asthma, result in immune system imbalance and airway hyperresponsiveness (1,2). Thus, asthma development is often accompanied by changes in inflammatory mediators, chemokines, and cytokines (32-34). In this study, 50 DEPs were identified between asthmatic and healthy individuals. The GO and KEGG enrichment analyses showed that these DEPs were significantly enriched in immune cell chemotaxis-related GO terms and chemokine- and cytokine-related signal pathways, consistent with the asthmatic pathological characteristics and previous results (15,20,30,35). Among these DEPs, proteins involved in leukocyte chemotaxis or migration, such as PPBP, PF4, PF4V1, and THBS1, were upregulated in asthmatic patients, implying recruitment of certain leukocytes. The asthmatic children had higher neutrophil and eosinophil counts compared with healthy children, confirming the recruitment of leukocytes. Previous studies have suggested that neutrophil counts are associated with asthma exacerbations (36,37). Moreover, chemokines are related to neutrophilic and eosinophilic inflammation in asthmatic patients (38). Our results further confirmed the abovementioned conclusions and identified several biomarkers for childhood asthma, such as MMP14, ABHD12B, LTBP1, APOA1, IGHG4, ANG, and IGFALS.
Early studies have found the dominance of the IGHG2*n allele in childhood asthma and allergy patients (39,40). Another study showed a dose-based relationship between IGHG2*n and IgE sensitization as risk factors for IgE-mediated asthma, while the opposite relationship was noted for non-IgE-mediated asthma (41). Moreover, Ooka et al. used unsupervised machine learning methods to synthesize clinical, viral, and serum proteome data to identify bronchiolitis endotypes in infants hospitalized for physician-diagnosis of bronchiolitis and found that endotype 1 infants with high proportion of IgE sensitization and rotavirus (RV) infection also had dysregulated NFκB pathways and significantly higher risks for developing asthma and endotype 2 infants with low proportion of IgE sensitization and high proportion of respiratory syncytial viral (RSV) or RV infection had dysregulated tumor necrosis factor (TNF)-mediated signalling pathway and significantly higher risks for developing asthma (42). In this study, IGHG2 and IGHG4 showed increased abundance in both cohorts with acute and convalescent asthma, but had significantly lower levels in healthy controls. These results further confirm the importance of IGHG2 and IGHG4 in asthma.
Previous results suggest that APOA1, the major structural protein of high-density lipoproteins (43), modulates airflow obstruction and airway inflammation in asthma (44,45). Recent clinical studies showed that APOA1 was significantly differentially expressed in the bronchoalveolar lavage fluid and serum of asthmatic patients (46-48), consistent with our serum proteome findings. These results revealed the importance of APOA1 in the asthmatic pathological mechanism and displayed the potential role of APOA1 as a diagnostic biomarker.
TGF-β can induce multiple cellular responses, such as differentiation, apoptosis, survival, and proliferation, and is implicated in the development of asthma (49). LTBP1, which encodes a protein that maintains TGF-β in the latent extracellular matrix (ECM)-bound form, can regulate TGF-β activity (50). Therefore, LTBP1 may play important roles in asthma development. It has been shown that LTBP1 is differentially expressed in asthma patients through transcriptome and real-time polymerase chain reaction (PCR) analysis (51,52). Our proteome analysis also found that LTBP1 was significantly accumulated in the serum of asthmatic patients, which is consistent with the previous results.
In asthma, airway angiogenesis is a prerequisite for airway remodelling (53-55). The angiogenin (ANG) protein plays a critical role in angiogenesis (56). Our proteomics analysis found that ANG is a significant DEP between asthmatic and healthy children. Several previous studies have reported that ANG was differentially accumulated between asthmatic patients and healthy controls (57-59), indicating its biomarker potential for asthma diagnosis. The matrix metalloproteinase (MMP) family was demonstrated as having a critical role in airway remodeling and could also compromise lung function under certain circumstances (60). Our results suggest that the MMP14 abundance differed between asthmatic and healthy children, consistent with a previous report (61). Meanwhile, in a previous study, TNXB was found to differently accumulate between children with and without asthma (15). There have been few reports regarding the role of ABHD12B in asthma, which requires further investigation.
IGFALS, a member of the IGF family associated with asthma pathogenesis. IGF family proteins such as IGF1 and IGF2 can promotes subepithelial fibrosis, inflammation, hyperresponsiveness, and smooth muscle cell hyperplasia in the airways (62). And the level of IGF1 could be decreased by anti-IgE antibody such as omalizumab (20). In this study, DEPs were significantly enriched the Regulation of IGFBPs pathway, and IGFALS was found highly expressed in asthma patients and being significantly positively correlated with the IgE level, suggesting that IGFALS may play a similar role as IGF1 or IGF2 in the pathogenesis of asthma, and its level could also be regulated by anti-IgE antibody. IGFALS has been reported as a serum biomarker of asthma in a previous study (15,20). We built a diagnostic model for asthma in preschoolers based on IGFALS, further indicating that IGFALS is a diagnostic biomarker for asthma.
Implications and actions needed
Future studies with a larger cohort would be required to verify the sensitivity and specificity of biomarkers such as IGFALS, LTBP1, and APOA1 in differentiating children with asthma from healthy controls. We follow up partial patients and will collect their plasma samples regularly in the next 2 years to obtain the correlation between the protein expression changes and the asthma progression. The reproducibility and accuracy of our results in other populations required further investigation.
Conclusions
In conclusion, we adopted the DIA-MS method to analyze the serum proteome signatures of children with acute and convalescent asthma. A total of 747 protein were identified in serum and 50 DEPs were found between asthmatic and healthy children. The GO and KEGG enrichment analyses showed that these DEPs were significantly enriched in immune cell chemotaxis-related GO terms and chemokine- and cytokine-related signal pathways, further confirming the central role of the inflammation-immune mechanisms in asthma pathogenesis. Several alternative asthma biomarkers, such as IMMP14, ABHD12B, LTBP1, APOA1, ANG, and IGFALS, were discovered in serum proteomes by statistical analysis. Ultimately, a preschooler asthma diagnostic model based on IGFALS was evaluated. We believe that the proteome results will provide insight into the pathophysiology of asthma, and the diagnostic model will facilitate clinical decision-making in preschooler asthmatic patients.
Acknowledgments
First, we give the sincerest thanks to all children included in this study. Next, we would like to thank LetPub (www.letpub.com) for its linguistic assistance during the preparation of this manuscript.
Funding: This research was supported by
Footnote
Reporting Checklist: The authors have completed the STARD reporting checklist. Available at https://jtd.amegroups.com/article/view/10.21037/jtd-23-974/rc
Data Sharing Statement: Available at https://jtd.amegroups.com/article/view/10.21037/jtd-23-974/dss
Peer Review File: Available at https://jtd.amegroups.com/article/view/10.21037/jtd-23-974/prf
Conflicts of Interest: All authors have completed the ICMJE uniform disclosure form (available at https://jtd.amegroups.com/article/view/10.21037/jtd-23-974/coif). The authors have no conflicts of interest to declare.
Ethical Statement: The authors are accountable for all aspects of the work in ensuring that questions related to the accuracy or integrity of any part of the work are appropriately investigated and resolved. The study was conducted in accordance with the Declaration of Helsinki (as revised in 2013). The research protocol was approved by the Ethics Committee of The Second Affiliated Hospital of Shaanxi University of Chinese Medicine (No. CYL-SQ202216). All of the participants’ guardians provided written informed consent.
Open Access Statement: This is an Open Access article distributed in accordance with the Creative Commons Attribution-NonCommercial-NoDerivs 4.0 International License (CC BY-NC-ND 4.0), which permits the non-commercial replication and distribution of the article with the strict proviso that no changes or edits are made and the original work is properly cited (including links to both the formal publication through the relevant DOI and the license). See: https://creativecommons.org/licenses/by-nc-nd/4.0/.
References
- Papi A, Brightling C, Pedersen SE, et al. Asthma. Lancet 2018;391:783-800. [Crossref] [PubMed]
- Valero A, Ribó P, Maíz L, et al. Asthma patient satisfaction with different dry powder inhalers. Expert Rev Respir Med 2019;13:133-8. [Crossref] [PubMed]
- de Jong CCM, Pedersen ESL, Mozun R, et al. Diagnosis of asthma in children: findings from the Swiss Paediatric Airway Cohort. Eur Respir J 2020;56:2000132. [Crossref] [PubMed]
- Sears MR, Greene JM, Willan AR, et al. A longitudinal, population-based, cohort study of childhood asthma followed to adulthood. N Engl J Med 2003;349:1414-22. [Crossref] [PubMed]
- McGeachie MJ, Yates KP, Zhou X, et al. Patterns of Growth and Decline in Lung Function in Persistent Childhood Asthma. N Engl J Med 2016;374:1842-52. [Crossref] [PubMed]
- Saglani S, Malmström K, Pelkonen AS, et al. Airway remodeling and inflammation in symptomatic infants with reversible airflow obstruction. Am J Respir Crit Care Med 2005;171:722-7. [Crossref] [PubMed]
- Saglani S, Payne DN, Zhu J, et al. Early detection of airway wall remodeling and eosinophilic inflammation in preschool wheezers. Am J Respir Crit Care Med 2007;176:858-64. [Crossref] [PubMed]
- Yoshihara S. Early intervention for infantile and childhood asthma. Expert Rev Clin Immunol 2010;6:247-55. [Crossref] [PubMed]
- Klok T, Kaptein AA, Duiverman E, et al. General practitioners' prescribing behaviour as a determinant of poor persistence with inhaled corticosteroids in children with respiratory symptoms: mixed methods study. BMJ Open 2013;3:e002310. [Crossref] [PubMed]
- Reddel HK, Bacharier LB, Bateman ED, et al. Global Initiative for Asthma Strategy 2021. 2022. Available online: https://www.sciencedirect.com/science/article/pii/S0300289621003471
- British Thoracic Society Scottish Intercollegiate Guidelines Network. British Guideline on the Management of Asthma. Thorax 2008;63:iv1-121. [Crossref] [PubMed]
- Asthma: diagnosis and monitoring of asthma in adults, children and young people. London: National Institute for Health and Care Excellence (NICE); 2017.
- Looijmans-van den Akker I, van Luijn K, Verheij T. Overdiagnosis of asthma in children in primary care: a retrospective analysis. Br J Gen Pract 2016;66:e152-7. [Crossref] [PubMed]
- Yang CL, Simons E, Foty RG, et al. Misdiagnosis of asthma in schoolchildren. Pediatr Pulmonol 2017;52:293-302. [Crossref] [PubMed]
- Li M, Wu M, Qin Y, et al. Differentially expressed serum proteins in children with or without asthma as determined using isobaric tags for relative and absolute quantitation proteomics. PeerJ 2020;8:e9971. [Crossref] [PubMed]
- Golebski K, Kabesch M, Melén E, et al. Childhood asthma in the new omics era: challenges and perspectives. Curr Opin Allergy Clin Immunol 2020;20:155-61. [Crossref] [PubMed]
- Rossi R, De Palma A, Benazzi L, et al. Biomarker discovery in asthma and COPD by proteomic approaches. Proteomics Clin Appl 2014;8:901-15. [Crossref] [PubMed]
- Fujii K, Nakamura H, Nishimura T. Recent mass spectrometry-based proteomics for biomarker discovery in lung cancer, COPD, and asthma. Expert Rev Proteomics 2017;14:373-86. [Crossref] [PubMed]
- Terracciano R, Pelaia G, Preianò M, et al. Asthma and COPD proteomics: current approaches and future directions. Proteomics Clin Appl 2015;9:203-20. [Crossref] [PubMed]
- Nieto-Fontarigo JJ, González-Barcala FJ, Andrade-Bulos LJ, et al. iTRAQ-based proteomic analysis reveals potential serum biomarkers of allergic and nonallergic asthma. Allergy 2020;75:3171-83. [Crossref] [PubMed]
- Bruderer R, Bernhardt OM, Gandhi T, et al. Optimization of Experimental Parameters in Data-Independent Mass Spectrometry Significantly Increases Depth and Reproducibility of Results. Mol Cell Proteomics 2017;16:2296-309. [Crossref] [PubMed]
- Bruderer R, Bernhardt OM, Gandhi T, et al. Extending the limits of quantitative proteome profiling with data-independent acquisition and application to acetaminophen-treated three-dimensional liver microtissues. Mol Cell Proteomics 2015;14:1400-10. [Crossref] [PubMed]
- Amon S, Meier-Abt F, Gillet LC, et al. Sensitive Quantitative Proteomics of Human Hematopoietic Stem and Progenitor Cells by Data-independent Acquisition Mass Spectrometry. Mol Cell Proteomics 2019;18:1454-67. [Crossref] [PubMed]
- Zamora-Mendoza BN, Espinosa-Tanguma R, Ramírez-Elías MG, et al. Surface-enhanced raman spectroscopy: A non invasive alternative procedure for early detection in childhood asthma biomarkers in saliva. Photodiagnosis Photodyn Ther 2019;27:85-91. [Crossref] [PubMed]
- Gagliardo R, Gras D, La Grutta S, et al. Airway lipoxin A4/formyl peptide receptor 2-lipoxin receptor levels in pediatric patients with severe asthma. J Allergy Clin Immunol 2016;137:1796-806. [Crossref] [PubMed]
- Xu P, Wang L, Chen D, et al. The application of proteomics in the diagnosis and treatment of bronchial asthma. Ann Transl Med 2020;8:132. [Crossref] [PubMed]
- Geyer PE, Holdt LM, Teupser D, et al. Revisiting biomarker discovery by plasma proteomics. Mol Syst Biol 2017;13:942. [Crossref] [PubMed]
- Suhre K, McCarthy MI, Schwenk JM. Genetics meets proteomics: perspectives for large population-based studies. Nat Rev Genet 2021;22:19-37. [Crossref] [PubMed]
- Khalilpour A, Kilic T, Khalilpour S, et al. Proteomic-based biomarker discovery for development of next generation diagnostics. Appl Microbiol Biotechnol 2017;101:475-91. [Crossref] [PubMed]
- Bai J, Zhong JY, Liao W, et al. iTRAQ based proteomic analysis reveals potential regulatory networks in dust mite related asthma treated with subcutaneous allergen immunotherapy. Mol Med Rep 2020;22:3607-20. [Crossref] [PubMed]
- Zhou Y, Kuai S, Pan R, et al. Quantitative proteomics profiling of plasma from children with asthma. Int Immunopharmacol 2023;119:110249. [Crossref] [PubMed]
- Hamid Q, Tulic M. Immunobiology of asthma. Annu Rev Physiol 2009;71:489-507. [Crossref] [PubMed]
- Hastie AT, Steele C, Dunaway CW, et al. Complex association patterns for inflammatory mediators in induced sputum from subjects with asthma. Clin Exp Allergy 2018;48:787-97. [Crossref] [PubMed]
- Barnes PJ. Targeting cytokines to treat asthma and chronic obstructive pulmonary disease. Nat Rev Immunol 2018;18:454-66. [Crossref] [PubMed]
- Zounemat Kermani N, Saqi M, Agapow P, et al. Type 2-low asthma phenotypes by integration of sputum transcriptomics and serum proteomics. Allergy 2021;76:380-3. [Crossref] [PubMed]
- Jatakanon A, Uasuf C, Maziak W, et al. Neutrophilic inflammation in severe persistent asthma. Am J Respir Crit Care Med 1999;160:1532-9. [Crossref] [PubMed]
- Norzila MZ, Fakes K, Henry RL, et al. Interleukin-8 secretion and neutrophil recruitment accompanies induced sputum eosinophil activation in children with acute asthma. Am J Respir Crit Care Med 2000;161:769-74. [Crossref] [PubMed]
- Kikuchi S, Kikuchi I, Takaku Y, et al. Neutrophilic inflammation and CXC chemokines in patients with refractory asthma. Int Arch Allergy Immunol 2009;149:87-93. [Crossref] [PubMed]
- Oxelius VA, Bråbäck L, Ahlstedt S, et al. Immunoglobulin constant heavy G chain genes as risk factors in childhood allergies. Clin Exp Allergy 2006;36:1616-24. [Crossref] [PubMed]
- Oxelius VA. Immunoglobulin constant heavy G subclass chain genes in asthma and allergy. Immunol Res 2008;40:179-91. [Crossref] [PubMed]
- Oxelius VA. From genotypes of immunoglobulin constant heavy G chains (Fcγ) (GM) genes (IGHG) to phenotypes in childhood asthma. Int Arch Allergy Immunol 2012;159:94-102. [Crossref] [PubMed]
- Ooka T, Raita Y, Fujiogi M, et al. Proteomics endotyping of infants with severe bronchiolitis and risk of childhood asthma. Allergy 2022;77:3350-61. [Crossref] [PubMed]
- Catapano AL, Pirillo A, Bonacina F, et al. HDL in innate and adaptive immunity. Cardiovasc Res 2014;103:372-83. [Crossref] [PubMed]
- Dai C, Yao X, Keeran KJ, et al. Apolipoprotein A-I attenuates ovalbumin-induced neutrophilic airway inflammation via a granulocyte colony-stimulating factor-dependent mechanism. Am J Respir Cell Mol Biol 2012;47:186-95. [Crossref] [PubMed]
- Yao X, Dai C, Fredriksson K, et al. 5A, an apolipoprotein A-I mimetic peptide, attenuates the induction of house dust mite-induced asthma. J Immunol 2011;186:576-83. [Crossref] [PubMed]
- Barochia AV, Kaler M, Cuento RA, et al. Serum apolipoprotein A-I and large high-density lipoprotein particles are positively correlated with FEV1 in atopic asthma. Am J Respir Crit Care Med 2015;191:990-1000. [Crossref] [PubMed]
- Ejaz S, Nasim FU, Ashraf M, et al. Serum Proteome Profiling to Identify Proteins Promoting Pathogenesis of Non-atopic Asthma. Protein Pept Lett 2018;25:933-42. [Crossref] [PubMed]
- Park SW, Lee EH, Lee EJ, et al. Apolipoprotein A1 potentiates lipoxin A4 synthesis and recovery of allergen-induced disrupted tight junctions in the airway epithelium. Clin Exp Allergy 2013;43:914-27. [Crossref] [PubMed]
- Makinde T, Murphy RF, Agrawal DK. The regulatory role of TGF-beta in airway remodeling in asthma. Immunol Cell Biol 2007;85:348-56. [Crossref] [PubMed]
- Liu G, Cooley MA, Jarnicki AG, et al. Fibulin-1c regulates transforming growth factor-β activation in pulmonary tissue fibrosis. JCI Insight 2019;5:e124529. [Crossref] [PubMed]
- Bazan-Socha S, Buregwa-Czuma S, Jakiela B, et al. Reticular Basement Membrane Thickness Is Associated with Growth- and Fibrosis-Promoting Airway Transcriptome Profile-Study in Asthma Patients. Int J Mol Sci 2021;22:998. [Crossref] [PubMed]
- Mami S, Ghaffarpour S, Faghihzadeh S, et al. Evaluation of the LTBP1 and Smad6 Genes Expression in Lung Tissue of Sulfur Mustard-exposed Individuals with Long-term Pulmonary Complications. Iran J Allergy Asthma Immunol 2019;18:473-8. [Crossref] [PubMed]
- Hoshino M, Takahashi M, Aoike N. Expression of vascular endothelial growth factor, basic fibroblast growth factor, and angiogenin immunoreactivity in asthmatic airways and its relationship to angiogenesis. J Allergy Clin Immunol 2001;107:295-301. [Crossref] [PubMed]
- Kristan SS, Malovrh MM, Silar M, et al. Airway angiogenesis in patients with rhinitis and controlled asthma. Clin Exp Allergy 2009;39:354-60. [Crossref] [PubMed]
- Simcock DE, Kanabar V, Clarke GW, et al. Induction of angiogenesis by airway smooth muscle from patients with asthma. Am J Respir Crit Care Med 2008;178:460-8. [Crossref] [PubMed]
- Cucci LM, Satriano C, Marzo T, et al. Angiogenin and Copper Crossing in Wound Healing. Int J Mol Sci 2021;22:10704. [Crossref] [PubMed]
- Ricciardolo FL, Sabatini F, Sorbello V, et al. Expression of vascular remodelling markers in relation to bradykinin receptors in asthma and COPD. Thorax 2013;68:803-11. [Crossref] [PubMed]
- Grzela K, Litwiniuk M, Krejner A, et al. Increased angiogenic factors in exhaled breath condensate of children with severe asthma - New markers of disease progression? Respir Med 2016;118:119-21. [Crossref] [PubMed]
- Abdel-Rahman AMO, El-Sahrigy SAF, Bakr SI. A comparative study of two angiogenic factors: vascular endothelial growth factor and angiogenin in induced sputum from asthmatic children in acute attack. Chest 2006;129:266-71. [Crossref] [PubMed]
- Hendrix AY, Kheradmand F. The Role of Matrix Metalloproteinases in Development, Repair, and Destruction of the Lungs. Prog Mol Biol Transl Sci 2017;148:1-29. [Crossref] [PubMed]
- Henderson N, Markwick LJ, Elshaw SR, et al. Collagen I and thrombin activate MMP-2 by MMP-14-dependent and -independent pathways: implications for airway smooth muscle migration. Am J Physiol Lung Cell Mol Physiol 2007;292:L1030-8. [Crossref] [PubMed]
- Lee H, Kim SR, Oh Y, et al. Targeting insulin-like growth factor-I and insulin-like growth factor-binding protein-3 signaling pathways. A novel therapeutic approach for asthma. Am J Respir Cell Mol Biol 2014;50:667-77. [Crossref] [PubMed]