Risk factors and prediction model for thrombocytopenia following coronary artery bypass graft surgery in elderly Chinese population
Highlight box
Key findings
• A risk prediction model for thrombocytopenia after coronary artery bypass graft (CABG) was developed and validated in elderly patients using the least absolute shrinkage and selection operator regression, The model demonstrated good prediction performance in the population.
What is known and what is new?
• Preoperative baseline variables associated with thrombocytopenia included body mass index, estimated glomerular filtration rate, B-type natriuretic peptide, preoperative platelet, and use of beta-blocker.
• Intraoperative variables associated with thrombocytopenia included red blood cell (RBC) transfusion, plasma transfusion, use of intra-aortic balloon pump and cardiopulmonary bypass, reoperation for bleeding, washed RBC transfusion volume, and use of epinephrine.
What is the implication, and what should change now?
• This risk prediction model can assist clinicians in predicting the occurrence of thrombocytopenia after CABG surgery and improving patient care and surgical outcomes in elderly Chinese population.
Introduction
Coronary artery bypass graft (CABG) surgery has become a major procedure in the management of coronary heart disease (1). Thrombocytopenia after CABG is a common postoperative complication. Some studies have reported that postoperative thrombocytopenia [a minimum in-hospital platelet (PLT) value <100×109/L] is associated with acute kidney injury (AKI), stroke, and increased risk of mortality in critically ill patients after CABG surgery (2-4).
Severe thrombocytopenia is associated with higher morbidity and mortality, prolonged hospital stays, and increased medical costs (2). Various factors contribute to PLT dysfunction and reduced PLT count after CABG, such as the duration of cardiopulmonary bypass (CPB), duration of aortic clamping, and complexity of surgery, which are associated with a higher risk of postoperative thrombocytopenia (5). In addition, patient demographic characteristics, drugs, and other factors, such as age, preoperative PLT count, comorbidities (such as chronic kidney disease, liver disease, or diabetes), and the use of antiplatelet drugs, can also cause the occurrence of thrombocytopenia after CABG (6). Advanced age has been identified as a significant risk factor for thrombocytopenia, with older patients being more susceptible to thrombocytopenia following surgery. Several studies have demonstrated that older age is independently associated with lower PLT levels (7,8). In elderly patients (age ≥65 years), the incidence of thrombocytopenia is higher than that in younger patients due to age-related changes, including physiological, structural, and functional alterations (9). Medications used before and during CABG, such as aspirin and clopidogrel, might also contribute to PLT dysfunction and a decrease in PLT count (10,11). Therefore, greater attention should be paid to the prevention and management of thrombocytopenia in elderly patients undergoing CABG.
Multiple variables are associated with thrombocytopenia, making it challenging to predict thrombocytopenia. The objective of this study was to develop and validate a risk prediction model for thrombocytopenia after CABG in elderly patients. In this study, a risk prediction model was developed using preoperative and intraoperative variables to effectively identify high-risk elderly patients prone to developing thrombocytopenia and to enhance the postoperative treatment strategy for these individuals. We present this article in accordance with the TRIPOD reporting checklist (available at https://jtd.amegroups.com/article/view/10.21037/jtd-23-1396/rc).
Methods
Study population
Patients were retrospectively recruited from Beijing Anzhen Hospital between February 2019 and December 2020, based on specific inclusion and exclusion criteria. The inclusion criteria were patients aged over 65 years who had undergone CABG surgery without any concomitant surgeries. Patients with the following conditions not included in the study: (I) preoperative thrombocytopenia (PLT counts <100×109); (II) lack of baseline level of preoperative PLT; (III) preoperative severe liver or renal insufficiency [estimated glomerular filtration rate (eGFR) <30 mL/min]; (IV) missing case information; and (V) died during surgery. The study was conducted in accordance with the Declaration of Helsinki (as revised in 2013). The study was approved by the Ethics Committee of Beijing Anzhen Hospital, Capital Medical University (Approval No. 2021-069) and informed consent was taken from all the patients.
Definition of postoperative thrombocytopenia after CABG
Postoperative thrombocytopenia was defined as a postoperative PLT count <100×109/L as measured within 7 days after surgery. Severe postoperative thrombocytopenia was defined as a postoperative PLT count <50×109/L as measured within 7 days after surgery.
Statistical analysis
Statistical analyses were conducted using the R software 4.2.2. The entire study population was randomly split into the derivation set and the validation set in a 7:3 ratio. The normal distribution of continuous data was assessed using Kolmogorov-Smirnov test. Continuous data with non-normal distribution, median, and interquartile range (IQR) were reported, and the Mann-Whitney U-test was used for analysis. Categorical data were expressed as counts and percentages and analyzed using either the Chi-squared or Fisher’s exact test. A significance level of P<0.05 was considered statistically significant. Variables with more than 20% missing values were excluded from further analysis, whereas missing values for other variables were imputed using the average values or modes. In the multivariable analysis of the derivation set, all predictor variables for thrombocytopenia were included in the logistic regression model. Variable selection was performed using the least absolute shrinkage and selection operator (LASSO) regression method implemented in the “glmnet” package. Thrombocytopenia event was the dependent variable (coded as 0 for patients with thrombocytopenia and 1 for patients without thrombocytopenia). Ten-fold cross-validation was used to select the penalty term lambda (λ). The prediction performance of the fitting model was assessed using binomial deviation. Lambda+1se was considered to be the best lambda because the penalty power of lambda was too small to solve the problem of overfitting. Thus lambda.1se was chosen. Model evaluation was performed using decision curve analysis (DCA) curves, confusion matrices, and receiver operating characteristic (ROC) curves. The predictive performance of the logistic regression model for thrombocytopenia risk was analyzed separately for the derivation set and the validation set. The discrimination degree of the model was evaluated using the area under the ROC curve (AUC). Model calibration was assessed using calibration curves based on 1,000 bootstrap samplings.
Results
Patient characteristics
The cohort selection process is presented in Figure 1. A total of 1,773 patients participated in the study, with 405 cases (22.8%) classified as thrombocytopenia and 58 cases (3.3%) as severe thrombocytopenia. The patients were then randomly assigned to either the derivation set (1,242 cases) or the validation set (531 cases). Table 1 presents the baseline demographic and clinical characteristics of patients in both sets. Among the derivation set, 270 cases (21.7%) were categorized into the thrombocytopenia group, while 972 cases (78.3%) were classified into the non-thrombocytopenia group based on the postoperative thrombocytopenia definition. In the original cohort, the median age was 69.2 years (range, 65.1–87.5 years), and 68.4% of the patients were male. Hypertension (65.4%) was the most common comorbidity, followed by hyperlipidemia (53.6%) and type 2 diabetes mellitus (T2DM) (37.9%). The proportion of patients with CPB in the original cohort was 16.9%. No significant differences were observed between the two groups in terms of sex, hypertension, hyperlipidemia, prior cerebral infarction, or prior percutaneous coronary intervention (PCI), etc. (P>0.05). However, the proportion of patients with CPB and extracorporeal membrane oxygenation (ECMO) in the validation set was higher than that in the derivation set (P=0.023 and 0.030, respectively). As study groups were randomly assigned, this difference may be due to a lower incidence of events and is not thought to have affected the study results. Detailed information on other variables in the two groups is presented in Table 1.
Table 1
Variables | Total set (N=1,773) | Derivation set (N=1,242) | Validation set (N=531) | P value |
---|---|---|---|---|
Demographics | ||||
Age (years) | 69 [6] | 69 [6] | 69 [6] | 0.831 |
Male sex | 1,212 (68.4) | 852 (68.6) | 360 (67.8) | 0.739 |
Smoker† | 357 (20.1) | 253 (20.4) | 104 (19.6) | 0.706 |
Drinker‡ | 293 (16.5) | 203 (16.3) | 90 (16.9) | 0.754 |
BMI (kg/m2) | 25.3 [4.0] | 25.3 [3.9] | 25.3 [4.1] | 0.848 |
Comorbidities | ||||
Hypertension | 1,160 (65.4) | 828 (66.7) | 332 (62.5) | 0.093 |
T2DM | 672 (37.9) | 475 (38.2) | 197 (37.1) | 0.649 |
Hyperlipemia | 950 (53.6) | 673 (54.2) | 277 (52.2) | 0.434 |
Prior MI | 265 (14.9) | 175 (14.1) | 90 (16.9) | 0.122 |
Prior cerebral infarction | 197 (11.1) | 127 (10.2) | 70 (13.2) | 0.070 |
Prior PCI | 185 (10.4) | 126 (10.1) | 59 (11.1) | 0.542 |
Prior CABG | 33 (1.9) | 25 (2.0) | 8 (1.5) | 0.470 |
NYHA cardiac functional class | ||||
Class III/IV | 355 (20.0) | 239 (19.2) | 116 (21.8) | 0.210 |
Laboratory examinations at admission | ||||
eGFR (mL/min) | 77.4 [27.6] | 76.8 [27.3] | 76.1 [25.9] | 0.538 |
SCr (μmol/L) | 72.1 [22.0] | 73.4 [21.6] | 72.5 [20.7] | 0.855 |
UA (μmol/L) | 317.4 [123.1] | 327.0 [117.7] | 325.5 [121.0] | 0.378 |
BNP (pg/mL) | 185 [222] | 172 [215] | 172 [226] | 0.886 |
PLT (×109/L) | 219 [77] | 220 [75] | 214 [79] | 0.056 |
ALT (U/L) | 20 [15] | 21 [16] | 19 [15] | 0.151 |
AST (U/L) | 21 [11] | 21 [11] | 21 [10] | 0.422 |
Preoperative concomitant medication | ||||
Aspirin | 486 (27.4) | 341 (27.5) | 145 (27.3) | 0.949 |
Statin therapy | 344 (19.4) | 253 (20.4) | 91 (17.1) | 0.115 |
Contrast agent§ | 506 (28.5) | 357 (28.7) | 149 (28.1) | 0.770 |
Metformin | 195 (11.0) | 144 (11.6) | 51 (9.6) | 0.220 |
Heparin | 786 (44.3) | 543 (43.7) | 243 (45.8) | 0.428 |
Intraoperative | ||||
RBC transfusion | 415 (23.4) | 285 (22.9) | 130 (24.5) | 0.484 |
PLT transfusion | 27 (1.5) | 19 (1.5) | 8 (1.5) | 0.971 |
Plasma transfusion | 155 (8.7) | 115 (9.3) | 40 (7.5) | 0.238 |
Use of IABP | 126 (7.1) | 83 (6.7) | 43 (8.1) | 0.288 |
Use of ECMO | 5 (0.3) | 1 (0.1) | 4 (0.8) | 0.030 |
Use of CPB | 299 (16.9) | 193 (15.5) | 106 (20.0) | 0.023 |
Reoperation for bleeding | 30 (1.7) | 21 (1.7) | 9 (1.7) | 0.995 |
Use of epinephrine | 405 (22.8) | 280 (22.5) | 125 (23.5) | 0.647 |
Use of norepinephrine | 1,011 (57.0) | 721 (58.1) | 290 (54.6) | 0.180 |
Use of isoprenaline | 109 (6.1) | 83 (6.7) | 26 (4.9) | 0.151 |
Use of dopamine | 1,515 (85.4) | 1,051 (84.6) | 464 (87.4) | 0.131 |
Use of cephalosporin | 1,478 (83.4) | 1,034 (83.3) | 444 (83.6) | 0.851 |
Operation time (h) | 4 [1] | 4 [1] | 4 [1] | 0.287 |
Operation bleeding volume (×100 mL) | 8 [4] | 8 [4] | 8 [4] | 0.787 |
Operation total liquid intake (×100 mL) | 25.0 [9.5] | 25.0 [10.0] | 25.0 [9.5] | 0.692 |
Washed RBC transfusion volume (×100 mL) | 2.4 [4.0] | 2.4 [4.7] | 2.4 [3.0] | 0.271 |
Continuous data are expressed as median [interquartile range] and were calculated by Mann-Whitney U-test. Categorical data were presented as count (percentage) and were calculated by Chi-squared test. †, smoker means current smokers, excluding ex-smokers; ‡, drinker means current drinkers (men: >250 g/week; women: >100 g/week); §, contrast agent refers to the contrast agent examination within 7 days before surgery. BMI, body mass index; T2DM, diabetes mellitus type; MI, myocardial infarction; PCI, percutaneous coronary intervention; CABG, coronary artery bypass graft; NYHA, New York Heart Association; eGFR, estimated glomerular filtration rate; SCr, serum creatinine; UA, uric acid; BNP, B-type natriuretic peptide; PLT, platelet; ALT, alanine transaminase; AST, aspartate amino transferase; RBC, red blood cell; IABP, intra-aortic ballon pump; ECMO, extracorporeal membrane oxygenation; CPB, cardiopulmonary bypass.
LASSO regression analysis
Since there were many variables and relatively few cases, LASSO regression was used to extract features in patients after CABG. We utilized ten-fold cross-validation to select the penalty term. Figure 2 presents the variables obtained from the LASSO regression. Since the penalty power of lambda was too small to solve the problem of overfitting, we chose lambda.1se, which had fewer variables and was considered the best lambda, and 13 variables were selected for further logistic regression analysis. The findings indicated that risk factors associated with thrombocytopenia included preoperative baseline variables: body mass index (BMI), eGFR, B-type natriuretic peptide (BNP), preoperative PLT, and use of beta-blocker, and intraoperative variables: red blood cell (RBC) transfusion, plasma transfusion, use of intra-aortic balloon pump (IABP) and CPB, reoperation for bleeding, washed RBC transfusion volume and use of epinephrine, as shown in Table 2.
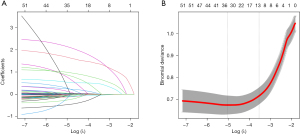
Table 2
Variables | Risk factors | Coefficient |
---|---|---|
X1 | BMI | 0.6331 |
X2 | eGFR | −0.0071 |
X3 | BNP | −0.0006 |
X4 | PLT | 0.0001 |
X5 | Beta blocker | −0.0013 |
X6 | RBC transfusion | −0.0120 |
X7 | Plasma transfusion | 0.1118 |
X8 | Use of IABP | 0.0959 |
X9 | Use of CPB | 0.2690 |
X10 | Reoperation for bleeding | 0.2610 |
X11 | Use of epinephrine | 0.0358 |
X12 | Washed RBC transfusion volume | 0.0066 |
Intercept | 0.6331 |
LASSO, least absolute shrinkage and selection operator; BMI, body mass index; eGFR, estimated glomerular filtration rate; BNP, B-type natriuretic peptide; PLT, platelet; RBC, red blood cell; IABP, intra-aortic ballon pump; CPB, cardiopulmonary bypass.
Model validation and calibration
Model validation and calibration were performed to assess the predictive performance and accuracy of the thrombocytopenia risk model. ROC curves were plotted (Figure 3). The AUC for the derivation set was 0.900 [95% confidence interval (CI): 0.880−0.920] (Figure 3A), while the AUC for the validation cohort was 0.897 (95% CI: 0.866−0.928) (Figure 3B). The confusion matrix diagrams for both sets are presented in Table 3. To evaluate the accuracy of the model, DCA was conducted and depicted in Figure 4. As shown in Table 4, the sensitivity for the model was 77.0% in the derivation set and 85.9% in the validation set. Similarly, the specificity was 88.1% in the derivation set and 79.8% in the validation set. Calibration curves were plotted to assess the agreement between the predicted and observed thrombocytopenia in the derivation set (Figure 5A) and the validation set (Figure 5B). The results demonstrated good calibration, indicating agreement between the model predictions and the actual occurrence of thrombocytopenia. Overall, these findings suggest that the model exhibits a favorable predictive performance.
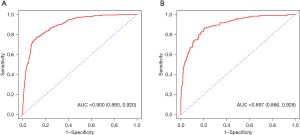
Table 3
Data set | Values | Actual value (positive) | Actual value (negative) |
---|---|---|---|
The derivation set | Predict value (positive) | 208 | 116 |
Predict value (negative) | 62 | 856 | |
The validation set | Predict value (positive) | 116 | 80 |
Predict value (negative) | 19 | 316 |
Table 4
Data set | Sensitivity (%) | Specificity (%) | NPV (%) | PPV (%) | Accuracy (%) |
---|---|---|---|---|---|
The derivation set | 77.0 | 88.1 | 93.2 | 64.2 | 85.7 |
The validation set | 85.9 | 79.8 | 94.3 | 59.2 | 81.4 |
NPV, negative predictive value; PPV, positive predictive value.
Discussion
This study mainly explored the risk factors for postoperative thrombocytopenia in elderly patients after CABG surgery, including preoperative baseline variables and intraoperative variables. A prediction model was established based on these risk factors, with good prediction performance.
PLT plays a crucial role in hemostasis, and their reduction after CABG can lead to impaired clot formation, increased bleeding complications, and potential adverse events (12,13). Inadequate clot formation may delay the healing process, increase the risk of wound infections, and potentially prolong the hospital stay (14,15). The development of thrombocytopenia after CABG is influenced by multiple factors. Although the exact mechanisms underlying thrombocytopenia after CABG are not yet fully understood, several factors have been proposed. These factors may include patient-related variables, such as age, preoperative PLT count, comorbidities (e.g., chronic kidney disease, diabetes mellitus), and medications (e.g., antiplatelet agents) (11). Surgical factors, such as the duration of CPB, aortic cross-clamp time, and complexity of the procedure can also influence PLT function and count (7).
In a retrospective study conducted by Yan et al. (7), the objective was to investigate the risk factors associated with thrombocytopenia after cardiac surgery with CPB. The analysis indicated that the following are positive predictors of thrombocytopenia: valve surgery included age >60 years, small body surface area, preoperative thrombocytopenia, and CPB time. Keles et al. (8) found that surgical procedures and the number of RBC transfusions were associated with the risk of moderate-to-severe thrombocytopenia after open-heart surgery. The risk factors identified in previous studies did not completely align with our findings. Our study specifically focused on elderly patients who underwent CABG, and it is possible that the related risk factors vary with age.
During CABG surgery, the use of CPB and activation of the inflammatory response can contribute to PLT dysfunction and decreased PLT counts (16). Studies have indicated that 30% of patients who underwent CPB develop thrombocytopenia, which is a significant predictor of morbidity and mortality after cardiac surgery (17-19). The potential mechanisms contributing to the development of thrombocytopenia include hemeprotein-induced oxidative damage, free iron-mediated toxicity, excessive oxidative stress, and endothelial dysfunction. Blood interaction with the outer surface of the CPB circuit triggers PLT activation, aggregation, and depletion (20). Additionally, the release of pro-inflammatory cytokines and activation of the clotting pathway triggered during CPB can disrupt normal PLT function, leading to PLT destruction or isolation (21). Longer CPB duration and the use of IABP have been associated with an increased risk of thrombocytopenia. Studies have reported varying degrees of PLT dysfunction and reduction associated with IABP use (22,23). These effects may be influenced by factors such as PLT activation, shear stress, PLT adhesion to the IABP surface, and altered blood flow dynamics (24). The utilization of IABP itself indicates hemodynamic instability and carries potential risk of atheroembolic during surgery, as well as additional risks to the kidney if the pump is improperly placed, thereby obstructing renal blood flow.
Old age is commonly associated with physiological changes in various organ systems, including the hematological system. The reason for this may be that bone marrow function declines with age, leading to shortened PLT turnover and lifespan in older individuals, which further contributes to PLT reduction (25,26). Moreover, older adults often have comorbidities and take multiple medications, including antiplatelet agents or anticoagulants, which can further affect PLT function and count.
Low BMI is associated with various hematological changes. Low body weight can lead to reduced PLT production in the bone marrow, resulting in lower baseline PLT counts. Additionally, deficiencies in essential nutrients and malnutrition associated with low BMI can contribute to impaired PLT function.
Moreover, individuals with low BMI often have a higher risk of comorbidities and malnutrition-related conditions (27). Chronic illnesses such as cancer or chronic kidney disease, commonly associated with low BMI, can contribute to PLT dysfunction and lower PLT counts, thereby increasing the risk of thrombocytopenia after CABG (28,29).
Studies have indicated a connection between thrombocytopenia and reduced cardiac function, which may lead to an increased incidence of postoperative thrombocytopenia (30,31). However, few studies have investigated the mechanism of postoperative thrombocytopenia caused by reduced cardiac function. One hypothesis is that thrombocytopenia could be associated with severe right heart failure and hemodynamic abnormalities associated with hepatic congestion and consequent hypersplenism (30). Preoperative elevated BNP is indicative of poor cardiac function and exhibits a positive correlation with PLT reduction. β-blocker therapy can improve left ventricular systolic and diastolic function, control heart rate, decrease cardiac afterload and preload, and serve as a protective factor for cardiac function in patients with chronic heart failure. Additionally, the use of epinephrine also reflects the level of cardiac function in patients. Our multivariate analysis showed beta-blockers were inversely associated with postoperative thrombocytopenia, while epinephrine showed a positive correlation.
Transfusion of plasma and blood cells can dilute the concentration of PLTs in the blood, leading to a relative decrease in the PLT count. This dilution effect is particularly relevant in cases of massive transfusion (32).
Heparin-induced thrombocytopenia (HIT) is a major concern in postoperative thrombocytopenia. HIT is an immune-mediated condition characterized by a drop in PLT count caused by the formation of antibodies against PLT factor 4 (PF4) complexed with heparin, with an incidence of only approximately 1–2% (33).
Our study differed from previous studies in the following aspects: (I) to our knowledge, few studies have developed a predictive model to help predict the risk of developing thrombocytopenia; (II) this study focused primarily on elderly patients aged 65 years and older who underwent CABG surgery. This population is more susceptible to thrombocytopenia due to impaired liver and kidney function; (III) to minimize the risk of overfitting, we applied LASSO regression to select variables that exhibited greater predictive value for outcomes. In this study, the incidence of thrombocytopenia after CABG was approximately 22.8%. Yan et al. reported an incidence of 10.2% (7) and Keles et al. reported 14.7% (8). These discrepancies may be attributed to differences in patient populations and thrombocytopenia definitions. Different definitions of thrombocytopenia and variations in its identification criteria can lead to inconsistent research results. Currently, there is no consistent definition for thrombocytopenia after cardiac surgery. A decrease in PLT count is common in patients after cardiac surgery, especially in elderly patients who often have lower PLT counts than normal healthy adults. The threshold for low PLT count has been inconsistent in many studies. In this study, we used a cutoff value of less than 100×109/L; patients below this value were associated with adverse events, including mortality, AKI, and extended hospital stay. Thrombocytopenia is a common postoperative clinical problem, influenced by various patient- and procedure-related factors. The utilization of a prediction model for postoperative thrombocytopenia can assist physicians in closely monitoring PLT counts of high-risk patients and administering appropriate treatment interventions, such as PLT-enhancing drugs or PLT transfusion. The aim is to minimize postoperative bleeding complications and expedite the recovery process, leading to shorter inpatient stays for patients.
Limitations
This study had several limitations. Firstly, it was a retrospective, single-center study that only included data from one center. The impact of risk factors and performance of the model may differ in centers with distinct population characteristics. Hence, external validation is imperative to ascertain its generalizability. Secondly, this study only incorporated available risk factors, while neglecting variables with too many missing values or those not considered. This omission may have influenced the prediction performance of the model. Due to the low proportion of patients who underwent CPB in the study, the predictive efficacy of this model for patients under CPB might be biased, and future studies with larger data are needed to verify and optimize the model. Thirdly, the definition of postoperative thrombocytopenia may have influenced the result. Additionally, PLT function and PLT activation markers were not evaluated in this study. Finally, the predictive ability was assessed using ROC curves in the derivation set and the validation set. Future prospective studies are needed to determine whether implementing this predictive model can effectively reduce the risk of thrombocytopenia in clinical practice.
Conclusions
The objective of this study was to develop and validate a risk prediction model for thrombocytopenia after CABG in elderly patients. LASSO regression was employed to select variables. The model demonstrated favorable predictive performance in both derivation and validation sets, which can assist clinicians in predicting the occurrence of thrombocytopenia after CABG surgery in elderly Chinese population.
Acknowledgments
The authors of this study would like to thank all the study participants.
Funding: None.
Footnote
Reporting Checklist: The authors have completed the TRIPOD reporting checklist. Available at https://jtd.amegroups.com/article/view/10.21037/jtd-23-1396/rc
Data Sharing Statement: Available at https://jtd.amegroups.com/article/view/10.21037/jtd-23-1396/dss
Peer Review File: Available at https://jtd.amegroups.com/article/view/10.21037/jtd-23-1396/prf
Conflicts of Interest: All authors have completed the ICMJE uniform disclosure form (available at https://jtd.amegroups.com/article/view/10.21037/jtd-23-1396/coif). The authors have no conflicts of interest to declare.
Ethical Statement: The authors are accountable for all aspects of the work in ensuring that questions related to the accuracy or integrity of any part of the work are appropriately investigated and resolved. The study was conducted in accordance with the Declaration of Helsinki (as revised in 2013). The study was approved by the Ethics Committee of Beijing AnZhen Hospital, Capital Medical University (Approval No. 2021-069) and informed consent was taken from all the patients.
Open Access Statement: This is an Open Access article distributed in accordance with the Creative Commons Attribution-NonCommercial-NoDerivs 4.0 International License (CC BY-NC-ND 4.0), which permits the non-commercial replication and distribution of the article with the strict proviso that no changes or edits are made and the original work is properly cited (including links to both the formal publication through the relevant DOI and the license). See: https://creativecommons.org/licenses/by-nc-nd/4.0/.
References
- Sabatine MS, Bergmark BA, Murphy SA, et al. Percutaneous coronary intervention with drug-eluting stents versus coronary artery bypass grafting in left main coronary artery disease: an individual patient data meta-analysis. Lancet 2021;398:2247-57. [Crossref] [PubMed]
- Kertai MD, Zhou S, Karhausen JA, et al. Platelet Counts, Acute Kidney Injury, and Mortality after Coronary Artery Bypass Grafting Surgery. Anesthesiology 2016;124:339-52. [Crossref] [PubMed]
- Williamson DR, Lesur O, Tétrault JP, et al. Thrombocytopenia in the critically ill: prevalence, incidence, risk factors, and clinical outcomes. Can J Anaesth 2013;60:641-51. [Crossref] [PubMed]
- Karhausen JA, Smeltz AM, Akushevich I, et al. Platelet Counts and Postoperative Stroke After Coronary Artery Bypass Grafting Surgery. Anesth Analg 2017;125:1129-39. [Crossref] [PubMed]
- Karhausen JA, Qi W, Smeltz AM, et al. Genome-Wide Association Study Links Receptor Tyrosine Kinase Inhibitor Sprouty 2 to Thrombocytopenia after Coronary Artery Bypass Surgery. Thromb Haemost 2018;118:1572-85. [Crossref] [PubMed]
- Li J, Yu D, Song Y, et al. Association Between Postoperative Thrombocytopenia and Outcomes After Coronary Artery Bypass Grafting Surgery. Front Surg 2021;8:747986. [Crossref] [PubMed]
- Yan S, Gao S, Lou S, et al. Risk Factors of Thrombocytopenia After Cardiac Surgery with Cardiopulmonary Bypass. Braz J Cardiovasc Surg 2023;38:389-97.
- Keles E, Bilen C, Aygun H, et al. Non-heparin-induced thrombocytopenia in patients after open-heart surgery. Perfusion 2023;38:781-90. [Crossref] [PubMed]
- van der Bom JG, Heckbert SR, Lumley T, et al. Platelet count and the risk for thrombosis and death in the elderly. J Thromb Haemost 2009;7:399-405. [Crossref] [PubMed]
- Verdoia M, Pergolini P, Rolla R, et al. Impact of Long-Term Dual Antiplatelet Therapy on Immature Platelet Count and Platelet Reactivity. Angiology 2018;69:490-6. [Crossref] [PubMed]
- Ibrahim H, Vapheas E, Shah B, et al. Preprocedural P2Y(12) inhibition and decrease in platelet count following transcatheter aortic valve replacement. Catheter Cardiovasc Interv 2019;94:812-7. [Crossref] [PubMed]
- Scridon A. Platelets and Their Role in Hemostasis and Thrombosis-From Physiology to Pathophysiology and Therapeutic Implications. Int J Mol Sci 2022;23:12772. [Crossref] [PubMed]
- Ho-Tin-Noé B, Boulaftali Y, Camerer E. Platelets and vascular integrity: how platelets prevent bleeding in inflammation. Blood 2018;131:277-88. [Crossref] [PubMed]
- Levoux J, Prola A, Lafuste P, et al. Platelets Facilitate the Wound-Healing Capability of Mesenchymal Stem Cells by Mitochondrial Transfer and Metabolic Reprogramming. Cell Metab 2021;33:283-299.e9. [Crossref] [PubMed]
- Golebiewska EM, Poole AW. Platelet secretion: From haemostasis to wound healing and beyond. Blood Rev 2015;29:153-62. [Crossref] [PubMed]
- Gielen CLI, Brand A, van Heerde WL, et al. Hemostatic alterations during coronary artery bypass grafting. Thromb Res 2016;140:140-6. [Crossref] [PubMed]
- Griffin BR, Bronsert M, Reece TB, et al. Thrombocytopenia After Cardiopulmonary Bypass Is Associated With Increased Morbidity and Mortality. Ann Thorac Surg 2020;110:50-7. [Crossref] [PubMed]
- Ranucci M, Baryshnikova E, Ranucci M, et al. Fibrinogen levels compensation of thrombocytopenia-induced bleeding following cardiac surgery. Int J Cardiol 2017;249:96-100. [Crossref] [PubMed]
- Agarwal S, Johnson RI, Kirmani BH. Pre- and Post-Bypass Platelet Function Testing With Multiple Electrode Aggregometry and TEG Platelet Mapping in Cardiac Surgery. J Cardiothorac Vasc Anesth 2015;29:1272-6. [Crossref] [PubMed]
- Wahba A, Black G, Koksch M, et al. Cardiopulmonary bypass leads to a preferential loss of activated platelets. A flow cytometric assay of platelet surface antigens. Eur J Cardiothorac Surg 1996;10:768-73. [Crossref] [PubMed]
- Bartoszko J, Karkouti K. Managing the coagulopathy associated with cardiopulmonary bypass. J Thromb Haemost 2021;19:617-32. [Crossref] [PubMed]
- Schiariti M, Saladini P, Cuturello D, et al. Decline in platelet count and long-term post-PCI ischemic events: implication of the intra-aortic balloon pump. Vascul Pharmacol 2014;60:25-31. [Crossref] [PubMed]
- Takano AM, Iwata H, Miyosawa K, et al. Reduced Number of Platelets During Intra-Aortic Balloon Pumping Counterpulsation Predicts Higher Cardiovascular Mortality After Device Removal in Association with Systemic Inflammation. Int Heart J 2020;61:89-95. [Crossref] [PubMed]
- Tong W, Wang JM, Li JY, et al. Incidence, predictors, and prognosis of thrombocytopenia among patients undergoing intra-aortic balloon pumping in the intensive care unit: a propensity score analysis. J Geriatr Cardiol 2021;18:123-34. [Crossref] [PubMed]
- Gibon E, Loi F, Córdova LA, et al. Aging Affects Bone Marrow Macrophage Polarization: Relevance to Bone Healing. Regen Eng Transl Med 2016;2:98-104. [Crossref] [PubMed]
- Pritz T, Lair J, Ban M, et al. Plasma cell numbers decrease in bone marrow of old patients. Eur J Immunol 2015;45:738-46. [Crossref] [PubMed]
- Romlin BS, Söderlund F, Wåhlander H, et al. Platelet count and function in paediatric cardiac surgery: a prospective observational study. Br J Anaesth 2014;113:847-54. [Crossref] [PubMed]
- Capizzi I, Teta L, Vigotti FN, et al. Weight Loss in Advanced Chronic Kidney Disease: Should We Consider Individualised, Qualitative, ad Libitum Diets? A Narrative Review and Case Study. Nutrients 2017;9:1109. [Crossref] [PubMed]
- Martin L, Senesse P, Gioulbasanis I, et al. Diagnostic criteria for the classification of cancer-associated weight loss. J Clin Oncol 2015;33:90-9. [Crossref] [PubMed]
- Mojadidi MK, Galeas JN, Goodman-Meza D, et al. Thrombocytopaenia as a Prognostic Indicator in Heart Failure with Reduced Ejection Fraction. Heart Lung Circ 2016;25:568-75. [Crossref] [PubMed]
- Chung I, Choudhury A, Lip GY. Platelet activation in acute, decompensated congestive heart failure. Thromb Res 2007;120:709-13. [Crossref] [PubMed]
- Ponschab M, Schöchl H, Gabriel C, et al. Haemostatic profile of reconstituted blood in a proposed 1:1:1 ratio of packed red blood cells, platelet concentrate and four different plasma preparations. Anaesthesia 2015;70:528-36. [Crossref] [PubMed]
- Pishko AM, Cuker A. Heparin-Induced Thrombocytopenia in Cardiac Surgery Patients. Semin Thromb Hemost 2017;43:691-8. [Crossref] [PubMed]