Cell-free DNA methylation profile potential in the diagnosis of lung squamous cell carcinoma
Highlight box
Key findings
• Lung squamous cell carcinoma (LUSC) tumor tissue has a distinct methylation profile to peritumoral and distant normal tissues.
• Analysis of cell-free DNA (cfDNA) methylation has potential utility in diagnosing LUSC.
What is known and what is new?
• Aberrant methylation occurs very early in the process of carcinogenesis and may be a valuable marker in early cancer detection.
• This study investigated the methylation patterns of LUSC tissue, peritumoral, and distant normal tissues, and further assessed its potential in the diagnosis of LUSC.
What is the implication, and what should change now?
• We characterized a tumor-specific methylation profile and revealed the clinical utility of cfDNA methylation, which may improve the clinical diagnosis of LUSC.
Introduction
Lung cancer is a common malignancy and the leading cause of cancer-related death (1-3). About 85% of lung cancer cases are non-small cell lung cancer (NSCLC), which could be further grouped into several histological subtypes, including lung adenocarcinoma (LUAD), lung squamous cell carcinoma (LUSC), large cell carcinoma, and others. Identifying cancer driver mutations at the genomic level provides several treatment options for NSCLC, especially for LUAD (4). Patients harboring sensitizing EGFR mutations, such as EGFR exon 19 deletions and L858R mutation, may benefit from targeted therapies with several epidermal growth factor receptor (EGFR) tyrosine kinase inhibitors (TKIs) (4,5). Patients with LUSC typically harbor TP53 and CDKN2A mutations (6), but compared with LUAD, have fewer targeted treatment options (7).
Epigenetic changes, such as DNA methylation and histone modification, also play important roles in the pathogenesis and progression of different cancer types (8,9). Next-generation sequencing (NGS)-based high-throughput profiling has shown abnormal hypomethylation at the genomic level and in the promoter region of certain genes (10). Several genes, including TIMP3, TGIF, AGTR1, HOXA9, SFMTB2, MLH1, SHOX2, SFRP4, CDKN2A/P16, DAPK, and ZIC4, show higher methylation frequencies in LUSC than in LUAD (11). A recent study identified the histone H3 lysine 36 (H3K36) methyltransferase NSD3 located in the 8p11-12 amplicon as a key driver gene underlying the tumorigenesis of LUSC (7). Further investigation on methylation patterns of LUSC might provide insights into developing novel biomarkers and therapeutic targets.
The preferred material for performing molecular analysis is tumor tissue obtained during surgery or biopsy. However, tissue sampling has several limitations, including invasiveness, inaccessible lesion locations, and poor sample quality (12). In some studies, adequate tissue samples were available in only 18% of NSCLC patients (13,14). Recently, plasma emerged as a practical alternative source for tumor molecular profiling. Circulating tumor DNA (ctDNA) is released by the tumor during apoptosis or necrosis (15). The advantage of ctDNA sequencing using liquid biopsy includes a faster turnaround time, minimal invasiveness, and the ability to overcome various limitations of clinical practice due to tumor heterogeneity. This technology has been applied to monitor responses to targeted therapies, evaluate residual disease after surgery, and explore the mechanisms of drug resistance (15). A previous study has demonstrated the potential clinical utility of cfDNA methylation profiling for the sensitive detection, monitoring and molecular subtyping of patients with NSCLC (11).
Our study aimed to identify LUSC-specific methylation patterns that could potentially distinguish non-tumor from tumor tissues, thus potentially serving as blood-based methylation biomarkers for LUSC differential diagnosis. Our preliminary results might contribute to better understanding of the molecular features of LUSC at the epigenetic level. We present this article in accordance with the MDAR reporting checklist (available at https://jtd.amegroups.com/article/view/10.21037/jtd-23-1827/rc).
Methods
Patient information and study design
This study included 49 patients with LUSC who underwent pulmonary resection from June 2016 to August 2020 in the First Affiliated Hospital, Zhejiang University School of Medicine. The patient clinical data and analytical materials were collected retrospectively. For each patient, the corresponding surgically resected tumors, peritumoral (≤2 cm), and remote normal tissue samples (≥5 cm) were collected during surgery. Blood samples (10 mL) were collected at baseline or after neoadjuvant therapy, and after surgery. To identify the methylation signatures, both tissue and plasma samples were subjected to bisulfite DNA sequencing. Blood samples collected from 48 healthy volunteers without lung nodules at lose-dose computed tomography served as controls.
The study was approved by the Clinical Research Ethics Committee of the First Affiliated Hospital, Zhejiang University School of Medicine (No. 2023-0598), and was conducted in accordance with the Declaration of Helsinki (as revised in 2013). All patients provided written informed consent.
DNA isolation
Genomic DNA of tissue specimens was extracted using QIAamp DNA FFPE tissue kits (Qiagen, Hilden, Germany) from collected tumor tissues and normal tissues. The extracted DNA was then quantified using a Qubit 2.0 fluorimeter (Life Technologies, Carlsbad, CA, USA). For cell-free DNA (cfDNA) extraction, about 10 mL of peripheral blood sample was obtained and stored in Cell-Free DNA BCT tubes (Streck, La Vista, NE, USA). Within 72 hours after collection, the blood sample was centrifuged at a speed of 2,000 ×g for 10 minutes at 4 ℃; the supernatant was centrifuged at a speed of 16,000 ×g for 10 minutes at 4 ℃ and then stored at −80 ℃. cfDNA was recovered from 4 to 5 mL of plasma by using the QIAamp Circulating Nucleic Acid Kit or QIAsymphony DSP Circulating DNA Kit (Qiagen). Quantification of cfDNA was conducted with the Qubit dsDNA HS assay (Thermo Fisher Scientific, Waltham, MA, USA).
Bisulfite targeted sequencing and methylation data processing
DNA was sequenced using an enhanced linear-splinter amplification sequencing (ELSA-seq) method as described previously (16). Extracted DNA was first converted to single-strand DNA molecules with sodium bisulfite (EZ-96 DNA Methylation-Lightning MagPrep, Zymo Research, Irvine, CA, USA), which was ligated to a splinted adapter in the presence of extension primers. A uracil-tolerating DNA polymerase was used to generate whole-genome bisulfite sequencing (BS-seq) libraries. Target enrichment was completed with lung cancer-specific methylation profiling RNA baits and further quantified with real-time polymerase chain reaction (PCR; Kapa Biosystems, Wilmington, MA, USA) and sequenced on a NovaSeq 6000 (Illumina, San Diego, CA, USA) using 2×150 bp cycles.
BS-seq data were further analyzed using an optimized pipeline. Raw data were trimmed using Trimmomatic (v.0.32) and then aligned using BWA-meth (v.0.2.2). After alignment, PCR duplicates were marked with Samblaster (v.0.1.20). The low mapping quality (mapping quality <20) or improper pairing reads were cleared from further analyses with Sambamba (v.0.4.7). The overlapping reads were removed by in-house scripts to avoid the double-counting of methylation signals.
Analysis of methylation patterns
Methylation levels were translated into scores to reflect the methylation features per sample (17,18). The methylation block was defined as the genomic region between the neighboring 5'-cytosine-phosphate-guanine-3' sites (CpG) with the r2 value. All the CpGs (n=80,672) were classified into 8,312 methylation blocks. The average methylation level within each methylation block (MethylMean) was calculated using the following equation:
where l is the number of sequencing reads for the CpGs that cover the blocks; M1 and M2 are the number of sequencing reads for the methylated CpGs per block covered in the forward strand and in the reverse strand, respectively; and U1 and U2 are the number of sequencing reads for methylated CpGs per block uncovered in the forward strand and in the reverse strand, respectively.
Functional enrichment analyses
The functional annotation of blocks was revealed using gene ontology (GO), Kyoto Encyclopedia of Genes and Genomes (KEGG), and gene set enrichment analysis (GSEA). GSEA was performed on the Molecular Signatures Database (version 7.4). The KEGG terms “c2.cp.kegg.v7.4.entrez.gmt” and “c2.cp.v7.4. entrez.gmt” were applied separately. The cutoff value of the two-sided adjusted P value was set as 0.05.
Statistical analysis
Principal component analysis (PCA) was applied for subgrouping samples. The “limma” package (v.3.460) in R software (The R Foundation for Statistical Computing) was used to analyze differential methylation. Differences were calculated using the Fisher exact test for proportions of the variables across groups. For two continuous variables, Pearson correlation analysis was applied. For the DNA methylation level comparison between two groups and three groups, the Student t-test or multiple paired t-test was applied, respectively. For continuous variables between two groups and three groups, the Wilcoxon rank-sum test or analysis of variance (ANOVA) was applied, respectively. Analyses were performed in R version 3.3.3 software, with two-sided P values less than 0.05 being considered statistically significant.
Results
Demographic and clinicopathological characteristics of patients
Three types of tissue samples (surgically resected tumor, peritumoral, and tumor-distant tissue) were collected from 49 patients with LUSC. Blood samples were obtained before or during surgery. For seven patients who received neoadjuvant therapy, blood samples were obtained after its completion. All patients involved in this study generated sequencing data with sufficient quality for all four sample types and were therefore subjected to further analyses.
The demographic and clinicopathological patients’ characteristics are presented in Table 1. The study group included 47 men and 2 women. Twenty-two patients (55%) were current smokers, 12 (30%) former smokers, and 6 (15%) had no smoking history. The control group of healthy volunteers included 39 men and nine women aged 32–89 years (median 44 years) (Table S1).
Table 1
Variables | Patients (n=49) |
---|---|
Age (years), median [range] | 63 [34, 80] |
Unknown | 1 |
Sex, n (%) | |
Female | 2 (4.1) |
Male | 47 (95.9) |
Smoking, n (%) | |
Never | 6 (12.2) |
Former | 12 (24.5) |
Current | 22 (44.9) |
Unknown | 9 (18.4) |
Alcohol, n (%) | |
No | 19 (38.8) |
Yes | 21 (42.9) |
Unknown | 9 (18.4) |
Stage, n (%) | |
I | 13 (26.5) |
II | 21 (42.9) |
III | 14 (28.6) |
Unknown | 1 (2.0) |
T stage, n (%) | |
T1 | 15 (30.6) |
T2 | 17 (34.7) |
T3 | 5 (10.2) |
T4 | 4 (8.2) |
Unknown | 8 (16.3) |
N stage, n (%) | |
N0 | 25 (51.0) |
N1 | 6 (12.2) |
N2 | 9 (18.4) |
N3 | 1 (2.0) |
Unknown | 8 (16.3) |
M stage, n (%) | |
M0 | 41 (83.7) |
Unknown | 8 (16.3) |
Cancer history, n (%) | |
No | 35 (71.4) |
Yes | 4 (8.2) |
Unknown | 10 (20.4) |
Differentiation level, n (%) | |
Moderate | 15 (30.6) |
Moderate to poor | 15 (30.6) |
Poor | 10 (20.4) |
Unknown | 9 (18.4) |
Neoadjuvant therapy, n (%) | |
No | 33 (67.4) |
Yes (platinum-based) | 7 (14.3) |
Unknown | 9 (18.4) |
Methylation profile of tumor tissues
PCA analysis was performed on all blocks (n=8,312). Methylation features of the tumor tissue were distinct from the peritumoral and normal tissues, while peritumoral and normal tissues shared a similar methylation profile (Figure 1). The heterogeneous methylation profiles were demonstrated in tumor samples but not in the plasma samples (Figure 1B). A total of 871 tumor-specific blocks, including 24 hypomethylated and 847 hypermethylated blocks, were differentially methylated in tumor tissue compared to normal tissue samples (limma: |delta; MethylMean| >0.2; adjusted P value <0.05; Figure 2). The top-ranked differential blocks were associated with either lung cancer or a broad spectrum of cancers (Table 2).
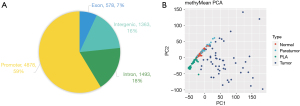
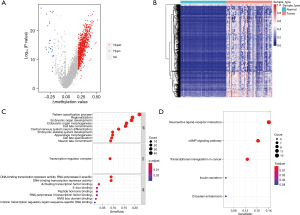
Table 2
Block | Chr | Annotation | Functions |
---|---|---|---|
br4068 | 1 | BARHL2|Promoter | Tumor specifically methylated in SCC with little or no detectable methylation seen in normal lung tissue or in blood DNA |
br5459 | 3 | GHSR|Promoter | GHSR DNA hypermethylation is a common epigenetic alteration of high diagnostic value in a broad spectrum of cancers |
br5458 | 3 | GHSR|Promoter | GHSR DNA hypermethylation is a common epigenetic alteration of high diagnostic value in a broad spectrum of cancers |
br7449 | 7 | HOXA9|Promoter, HOXA10-HOXA9|Intron | Highly prevalent DNA methylation in lung squamous and adenocarcinoma but not in normal lung tissue |
br4359 | 20 | MIR124-3|Promoter | Methylation of the miR-124 family could be used as a marker for the prognosis of tumor metastasis in NSCLC |
br1792 | 14 | OTX2|Promoter, OTX2-AS1|Promoter | DNA methylation biomarker for lung cancer |
br5093 | 2 | SIX3-AS1|Promoter, SIX3|Exon | Methylation of SIX3 promotes lung cancer proliferation and metastasis |
br1793 | 14 | OTX2|Promoter, OTX2-AS1|Promoter | DNA methylation biomarker for lung cancer |
br5122 | 2 | LINC01248|Promoter, SOX11|Promoter | Promoter methylation of SOX11 is associated with risk and development of various types of cancer |
br4358 | 20 | MIR124-3|Promoter | Methylation of the miR-124 family could be used as a marker for the prognosis of tumor metastasis in NSCLC |
Chr, chromosome; BARHL2, BarH like homeobox 2; SCC, squamous cell lung carcinoma; GHSR, growth hormone secretagogue receptor; HOXA9, homeobox A9; MIR124-3, microRNA 124-3; NSCLC, non-small cell lung cancer; OTX2, orthodenticle homeobox 2; OTX2-AS1, OTX2 antisense RNA 1; SIX3, SIX homeobox 3; SIX3-AS1, SIX3 antisense RNA 1; LINC01248, long intergenic non-protein coding RNA 1248; SOX11, SRY-box transcription factor 11.
To obtain information on the functional annotation of these blocks, both GO and KEGG enrichment analyses were performed for each block. GO analysis identified ten biological processes (BPs), one cellular component (CC), and eight molecular functions (MFs) that were significantly enriched in the hypermethylation blocks. The most significantly enriched BP terms were cell fate commitment, pattern specification process, and regionalization, all related to cell differentiation. The transcriptional factor binding was strongly implicated in enriched MF terms and was consistent with CC terms enriched in the transcription regulator complex. We also performed KEGG analysis, which revealed that neuroactive ligand-receptor interaction, cAMP signaling pathway, and transcriptional misregulation pathways were enriched in the hypermethylation blocks (Figure 2D). Together, these data suggest that hypermethylation blocks may participate in transcriptional regulation, emphasizing their importance in tumor initiation and development.
The identification of field cancerization-specific blocks
To further clarify the role of aberrant methylation in cancer development, the methylation level of each block among tumor, peritumoral, and normal tissue samples was compared using the multiple paired t-test. A total of 228 differentially methylated blocks were identified, suggesting premalignant field-related methylation patterns. The methylation levels of the 221 blocks were statistically lower in normal compared to peritumoral samples and in peritumoral compared to tumor tissue samples (Figure 3A). The methylation levels of seven blocks were significantly higher in normal compared to corresponding peritumoral samples and higher in peritumoral samples than in the tumor tissue samples (Figure 3B). The field cancerization-specific blocks did not correlate with clinical characteristics (Figure 3C).
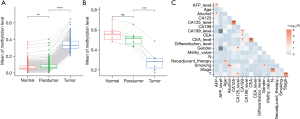
We also used the malignant contaminated ratio (MCR) (methylome-based scoring system) to compare the differences between the tumor and corresponding nontumor tissues within individual patients (19). This ratio may reflect the malignant methylation signal in the normal tissue shared by its corresponding tumor tissue. In this study, the MCR ranged from 0 to 0.2, with most samples being close to 0 (Figure S1A). The MCR in patients who underwent neoadjuvant therapy was significantly lower as compared to those who were chemotherapy-naïve (Figure S1B). Taken together, our results suggested the existence of distinct methylation profiles of tumor tissue and two types of adjacent tumor tissues.
Blood-based LUSC-specific methylation blocks differentiated LUSC from healthy controls
To identify markers for distinguishing LUSC-specific patients, methylation profiles of tumor, adjacent tissues, and healthy individuals’ blood were analyzed with capture-based targeted BS-seq. A total of 871 LUSC- and tissue-specific blocks significantly differed between tumor and matched normal tissues. We then aimed to develop a LUSC-diagnostic model based on methylation profiles to differentiate patients with LUSC from healthy individuals. Through random forest classification algorithm, 175 markers were selected between LUSC and healthy controls blood, and the methylation levels of these markers showed good methylation ranges in the plasma samples. Finally, three LUSC-specific methylation blocks (br4591, br4067, and br1095) were identified between these two methods (Figure 4A).
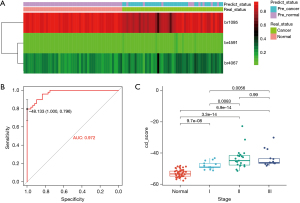
We next assessed a combined diagnostic score (cd-score) of the model for identifying patients with LUSC. We found that the cd-score could differentiate LUSC patients from healthy individuals (area under the curve =0.972; Figure 4B) and also correlated well with the tumor stage (Figure 4C). Unsupervised hierarchical clustering of the three blocks distinguished LUSC from healthy individuals with high specificity and sensitivity. The healthy control population had a significantly lower cfDNA cd-score than did patients with stage I–III LUSC, stage I patients had a significantly lower cfDNA cd-score than did stage II and III patients, whereas stage II and III patients had a similar cd-score. In addition, the cd-score preliminarily demonstrated superior sensitivity and specificity to the individual block for LUSC diagnosis (Figure S2A-S2C). Notably, there were no significant correlations among these three blocks (Figure S2D).
Molecular classification of LUSC
Unsupervised consensus clustering was employed to identify the molecular subtypes in this cohort using the blood-specific methylation blocks. According to the relative change in the area under the cumulative distribution function (CDF) curve and consensus heatmap, the optimal number of clusters was two (k=2). No appreciable increase was identified in the area under the CDF curve (Figure S3). PCA analysis indicated distinct methylation profiles between cluster 1 (C1) and cluster 2 (C2) patients (Figure 5A). The methylation profile of C1 was distinct from that of C2 and healthy controls. The cd-score of C1 was also significantly higher than that of C2 (Figure 5B). These results indicate that patients with LUSC can be classed into two subgroups with distinct methylation patterns.
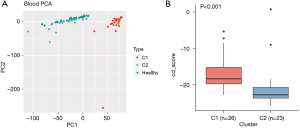
Discussion
DNA methylation occurs early in carcinogenesis in various cancer types. Its utility as a biomarker holds promise in early cancer detection and monitoring. Overall, we identified 871 LUSC-specific methylation blocks in the collected LUSC samples. Most of the blocks that have been identified may be regulated by methylation in cancer initiation or progression. For instance, both the OTX2 (20,21) and BARHL2 (20,22) genes were reported as DNA methylation markers in lung cancer, and the methylation level of the MIR124 gene was found to be increased in NSCLC (23). Further, the methylation of the HOXA9 gene has been suggested a reliable prognostic marker in NSCLC (24-26). In our study, functional enrichment analysis revealed that hypermethylation blocks are involved in transcriptional misregulation in LUSC.
The phenomenon of field cancerization involves the replacement of normal cells by cancer-primed cells without morphological change. We identified 228 field cancerization-specific blocks, which were differentially methylated in tumor tissue compared with peritumoral and normal tissues. Compared with tumor-specific blocks, the field cancerization-specific blocks may represent an earlier methylation change that occurs in tumor initiation and may serve as a more sensitive marker for early detection and LUSC management. The MCR was used to validate the results and also indicated that most of the peritumoral and normal tissue were distinct from the tumor tissue. In addition, we found that the field cancerization blocks were independent of the clinical factors, supporting potential role of methylation markers in the LUSC diagnosis.
Identifying panels for blood-based cancer diagnosis with minimal invasiveness is still an emerging field in LUSC. Wang et al. reported that gene methylation level might help to predict the survival outcomes in LUSC (27). Nunes et al. also found that methylation level assessments in cfDNA may provide a minimally invasive procedure for standard diagnostic of lung cancer (28). These findings both support the methylation level may draw some clue in LUSC diagnostic or prognostic. Therefore, we attempted to construct a diagnostic model using a three-block panel (cd-score) for blood-based liquid biopsy. The cd-score reliably discriminated patients with LUSC from healthy controls. As previous study only focused on the methylation level from promoter region (28), which may lose some information. The cd-score grouped three block which combines several CpG sites together to guarantee its stability in clinical utility. We also revealed that LUSC could be classified into two major molecular subgroups (C1 and C2). The methylation profile of the C2 subgroup was close to that of the healthy individuals. The MCR of patients who received neoadjuvant therapy was significantly lower than that of chemo-naïve patients, suggesting a methylation modifying effect of chemotherapy.
Our study has several limitations that could potentially impede the interpretation of our findings. First, it included a relatively small patient cohort from only a single institution. A well-designed validation cohort is needed to confirm the clinical utility of our cd-score. Second, we did not investigate the specific mechanism underlying the blocks utility in the model. Finally, the biological function and molecular mechanism of some genes involved in the model remain unclear. Further studies are required to consider the clinical application of our model.
Conclusions
We demonstrated the distinct tumor-specific methylation profiles in tumor-adjacent and tumor tissues, indicating the important role of DNA methylation as an early event in LUSC carcinogenesis. We also demonstrated the potential clinical utility of cfDNA methylation analysis in the diagnosis of LUSC.
Acknowledgments
We would like to thank all the participants and their families for their involvement in this study.
Funding: None.
Footnote
Reporting Checklist: The authors have completed the MDAR reporting checklist. Available at https://jtd.amegroups.com/article/view/10.21037/jtd-23-1827/rc
Data Sharing Statement: Available at https://jtd.amegroups.com/article/view/10.21037/jtd-23-1827/dss
Peer Review File: Available at https://jtd.amegroups.com/article/view/10.21037/jtd-23-1827/prf
Conflicts of Interest: All authors have completed the ICMJE uniform disclosure form (available at https://jtd.amegroups.com/article/view/10.21037/jtd-23-1827/coif). J.J. reports support from BMS, Roche, and MSD for consulting or advisory roles; support from Takeda for travel, accommodation, and expenses; and support from Pfizer, Novartis, and MSD for speakers bureau. The other authors have no conflicts of interest to declare.
Ethical Statement: The authors are accountable for all aspects of the work in ensuring that questions related to the accuracy or integrity of any part of the work are appropriately investigated and resolved. The study was approved by the Clinical Research Ethics Committee of the First Affiliated Hospital, Zhejiang University School of Medicine (No. 2023-0598), and was conducted in accordance with the Declaration of Helsinki (as revised in 2013). All patients provided written informed consent.
Open Access Statement: This is an Open Access article distributed in accordance with the Creative Commons Attribution-NonCommercial-NoDerivs 4.0 International License (CC BY-NC-ND 4.0), which permits the non-commercial replication and distribution of the article with the strict proviso that no changes or edits are made and the original work is properly cited (including links to both the formal publication through the relevant DOI and the license). See: https://creativecommons.org/licenses/by-nc-nd/4.0/.
References
- Chen W, Zheng R, Baade PD, et al. Cancer statistics in China, 2015. CA Cancer J Clin 2016;66:115-32. [Crossref] [PubMed]
- Bray F, Ferlay J, Soerjomataram I, et al. Global cancer statistics 2018: GLOBOCAN estimates of incidence and mortality worldwide for 36 cancers in 185 countries. CA Cancer J Clin 2018;68:394-424. [Crossref] [PubMed]
- Xia C, Dong X, Li H, et al. Cancer statistics in China and United States, 2022: profiles, trends, and determinants. Chin Med J (Engl) 2022;135:584-90. [Crossref] [PubMed]
- Hirsch FR, Scagliotti GV, Mulshine JL, et al. Lung cancer: current therapies and new targeted treatments. Lancet 2017;389:299-311. [Crossref] [PubMed]
- Soria JC, Ohe Y, Vansteenkiste J, et al. Osimertinib in Untreated EGFR-Mutated Advanced Non-Small-Cell Lung Cancer. N Engl J Med 2018;378:113-25. [Crossref] [PubMed]
- Herbst RS, Morgensztern D, Boshoff C. The biology and management of non-small cell lung cancer. Nature 2018;553:446-54. [Crossref] [PubMed]
- Yuan G, Flores NM, Hausmann S, et al. Elevated NSD3 histone methylation activity drives squamous cell lung cancer. Nature 2021;590:504-8. [Crossref] [PubMed]
- Normanno N, De Luca A, Bianco C, et al. Epidermal growth factor receptor (EGFR) signaling in cancer. Gene 2006;366:2-16. [Crossref] [PubMed]
- Lu Y, Chan YT, Tan HY, et al. Epigenetic regulation in human cancer: the potential role of epi-drug in cancer therapy. Mol Cancer 2020;19:79. [Crossref] [PubMed]
- Jones PA, Baylin SB. The fundamental role of epigenetic events in cancer. Nat Rev Genet 2002;3:415-28. [Crossref] [PubMed]
- Hoang PH, Landi MT. DNA Methylation in Lung Cancer: Mechanisms and Associations with Histological Subtypes, Molecular Alterations, and Major Epidemiological Factors. Cancers (Basel) 2022;14:961. [Crossref] [PubMed]
- Pisapia P, Malapelle U, Troncone G. Liquid Biopsy and Lung Cancer. Acta Cytol 2019;63:489-96. [Crossref] [PubMed]
- Aggarwal C, Thompson JC, Black TA, et al. Clinical Implications of Plasma-Based Genotyping With the Delivery of Personalized Therapy in Metastatic Non-Small Cell Lung Cancer. JAMA Oncol 2019;5:173-80. [Crossref] [PubMed]
- Leighl NB, Page RD, Raymond VM, et al. Clinical Utility of Comprehensive Cell-free DNA Analysis to Identify Genomic Biomarkers in Patients with Newly Diagnosed Metastatic Non-small Cell Lung Cancer. Clin Cancer Res 2019;25:4691-700. [Crossref] [PubMed]
- Rolfo C, Mack P, Scagliotti GV, et al. Liquid Biopsy for Advanced NSCLC: A Consensus Statement From the International Association for the Study of Lung Cancer. J Thorac Oncol 2021;16:1647-62. [Crossref] [PubMed]
- Kang G, Chen K, Yang F, et al. Monitoring of circulating tumor DNA and its aberrant methylation in the surveillance of surgical lung Cancer patients: protocol for a prospective observational study. BMC Cancer 2019;19:579. [Crossref] [PubMed]
- Liu Y, Feng Y, Hou T, et al. Investigation on the potential of circulating tumor DNA methylation patterns as prognostic biomarkers for lung squamous cell carcinoma. Transl Lung Cancer Res 2020;9:2356-66. [Crossref] [PubMed]
- Yang Y, Zheng D, Wu C, et al. Detecting Ultralow Frequency Mutation in Circulating Cell-Free DNA of Early-Stage Nonsmall Cell Lung Cancer Patients with Unique Molecular Identifiers. Small Methods 2019;3:1900206.
- Yang L, Zhang J, Yang G, et al. The prognostic value of a Methylome-based Malignancy Density Scoring System to predict recurrence risk in early-stage Lung Adenocarcinoma. Theranostics 2020;10:7635-44. [Crossref] [PubMed]
- Rauch TA, Wang Z, Wu X, et al. DNA methylation biomarkers for lung cancer. Tumour Biol 2012;33:287-96. [Crossref] [PubMed]
- Daugaard I, Dominguez D, Kjeldsen TE, et al. Identification and validation of candidate epigenetic biomarkers in lung adenocarcinoma. Sci Rep 2016;6:35807. [Crossref] [PubMed]
- Rauch TA, Zhong X, Wu X, et al. High-resolution mapping of DNA hypermethylation and hypomethylation in lung cancer. Proc Natl Acad Sci U S A 2008;105:252-7. [Crossref] [PubMed]
- Heller G, Altenberger C, Steiner I, et al. DNA methylation of microRNA-coding genes in non-small-cell lung cancer patients. J Pathol 2018;245:387-98. [Crossref] [PubMed]
- Ooki A, Maleki Z, Tsay JJ, et al. A Panel of Novel Detection and Prognostic Methylated DNA Markers in Primary Non-Small Cell Lung Cancer and Serum DNA. Clin Cancer Res 2017;23:7141-52. [Crossref] [PubMed]
- Robles AI, Arai E, Mathé EA, et al. An Integrated Prognostic Classifier for Stage I Lung Adenocarcinoma Based on mRNA, microRNA, and DNA Methylation Biomarkers. J Thorac Oncol 2015;10:1037-48. [Crossref] [PubMed]
- Wang Q, Wu L, Yu J, et al. Comparison of tumor and two types of paratumoral tissues highlighted epigenetic regulation of transcription during field cancerization in non-small cell lung cancer. BMC Med Genomics 2022;15:66. [Crossref] [PubMed]
- Wang W, Xiang M, Liu H, et al. A prognostic risk model based on DNA methylation levels of genes and lncRNAs in lung squamous cell carcinoma. PeerJ 2022;10:e13057. [Crossref] [PubMed]
- Nunes SP, Diniz F, Moreira-Barbosa C, et al. Subtyping Lung Cancer Using DNA Methylation in Liquid Biopsies. J Clin Med 2019;8:1500. [Crossref] [PubMed]