Prediction of postoperative final degree and recurrence of pectus excavatum using machine learning algorithms
Highlight box
Key findings
• Machine learning algorithms using risk factors and basic clinical data yields good performance for prediction of final radiographical pectus index (RPI) and recurrence after bar removal (BR).
What is known and what is new?
• This is the first study in which machine learning analysis is clinically used to evaluate treatment for pectus excavatum.
• RPI values before and after minimally invasive repair of pectus excavatum (MIRPE) and age at the time of MIRPE are independent risk factors for recurrence after BR.
What is the implication, and what should change now?
• Machine learning approaches are convenient and useful for predicting outcomes of MIRPE. In addition, an approach integrating machine learning models and readily available clinical data can be used to create other models in the thoracic surgery field.
Introduction
Pectus excavatum (PE) in which the sternum and rib cage are depressed abnormally, is the most common thoracic wall deformity (1,2). Minimally invasive repair of PE (MIRPE) is a standard procedure in which metal bars are placed beneath the sternum and used to lift the depressed chest wall (3,4). Bar placement is generally maintained for 2 to 3 years to guard against re-depression of the chest wall (5,6). The optimal age for MIRPE and recurrence of PE are the most important concerns in treatment because there is a high rate of chest wall re-depression after bar removal (BR) (2,4,5,7,8). The exact mechanisms or characteristics of the chest wall re-depression remain unclear (5,9,10). Furthermore, consensus regarding the definition of recurrence, defined as significant chest wall re-depression after BR, is lacking (2,5,9,10).
In a previous study, we investigated characteristics of chest wall re-depression and risk factors of recurrence of PE after BR (9). However, accurate prediction of chest wall re-depression is crucial for preventing recurrence. The prediction modality should provide predictive numerical value as well as information about risk factors for chest wall re-depression (11-13). However, prior studies are insufficient in prediction of surgical outcomes in PE. The prediction models using machine learning for chest wall re-depression after BR are valuable for better surgical outcomes because machine learning has successfully been used to make accurate decisions and predictions using diverse and large amounts of data. The primary aims of the present study are to investigate characteristics of chest wall re-depression and to predict final degree and recurrence of PE after BR using machine learning algorithms. To the best of our knowledge, this is the first study in which machine learning analysis was clinically used in PE. We present this article in accordance with the TRIPOD reporting checklist (available at https://jtd.amegroups.com/article/view/10.21037/jtd-23-1430/rc).
Methods
All consecutive subjects who underwent both MIRPE and BR at Uijeongbu St. Mary’s Hospital from March 2012 to June 2020 were retrospectively reviewed in the present study. MIRPE was conducted according to routine procedures at our institution (3,9). Eligible criteria for subject enrollment were no reoperation due to bar migration, no accompanying thoracic deformity, and no previous thoracic procedures, and no thoracic traumas such as rib, sternal, or spinal fractures. Simple chest posteroanterior and both lateral X-rays were acquired every day during hospitalization and at every outpatient follow-up appointment. Chest computed tomography (CT) scans were routinely acquired for evaluations before MIRPE and on the third postoperative day after both MIRPE and BR. The radiographical pectus index (RPI) was designed to quantify the degree of chest wall depression because a complete series of chest CT scans for the Haller index was not available at outpatient basis during follow-ups (3,5,14). Similar to the Haller index calculated using chest CT, RPI is calculated by the ratio of the transverse length to the posteroanterior length at the most depressed point of the chest wall shown by lateral chest X-rays (Figure 1) (5,14,15). We measured the transverse and posteroanterior lengths in double-blind fashion. In the present study, recurrence of PE, or significant chest wall re-depression after BR, is defined when cases met both conditions: increased RPI value and RPI value ≥3.5 after BR. Examining the perioperative data during MIRPE, the parameters assumed to be associated with chest wall re-depression after BR were age at the time of MIRPE, sex, RPI values, pectus deformity type (symmetric or asymmetric), sternal angle of bar placement, and number of inserted bars. The sternal angle of bar placement was defined as the angle formed by the sternum and the pectus bar after MIRPE. Previous studies verified that RPI measured by chest X-ray can be used instead of the Haller index measured by chest CT to describe degree of PE (5,9,14). We collected and analyzed data for these clinical parameters during treatment of PE.
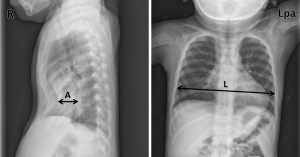
There is no consensus regarding recurrence after BR (7,9). In the present study, we dealt with this problem as follows. First, we investigated the patterns or attributes of chest wall re-depression presented by RPI after BR. Second, we investigated risk factors for recurrence according to our definition of PE recurrence and predicted postoperative final RPI and recurrence 1 year after BR using perioperative data during MIRPE. Third, to determine the optimal age at the time of MIRPE, we divided the subjects into two groups by age [<10 years (early repair group; EG) vs. ≥10 years (late repair group; LG)] when they underwent MIRPE and examined the relationship between age and recurrence.
Statistical analysis
All data are shown as mean ± standard deviation (SD). Comparisons between groups were analyzed using Student’s t-test or paired t-test. To investigate relationship between two quantitative continuous variables, we conducted Pearson correlation analysis. Chi-square tests were used to examine whether two categorical variables were independent in influencing the test statistic. Repeat measures analysis of variance test was performed to investigate changes of chest wall re-depression after BR. The binary logistic regression (LoR) test (backward, stepwise approach) was performed to investigate independent influencing factors for recurrence after BR. The Statistical Package for the Social Sciences version 22.0 (SPSS, IBM Corp, Armonk, NY, USA) was used to perform statistical evaluations. A P value <0.05 was considered statistically significant.
Machine learning analysis
Machine learning analyses were performed to predict the final postoperative RPI and recurrence after BR. The training and validation sets were used for model learning and 10-fold stratified cross-validation was used for training and validation. Performance scores of each model were calculated and evaluated based on area under the curve (AUC), accuracy, precision, F1 score, and recall. The outperformed model was selected based on AUC. Five machine learning algorithms [linear regression (LiR), neural network (NN), AdaBoost (AB), random forest (RF), and decision tree (DT)] were performed to predict the final postoperative RPI, and five machine learning algorithms [LoR, NN, Naïve Bayes (NB), RF, and support vector machine (SVM)] were used to predict recurrence. The Orange® data mining toolbox in Python (Bioinformatics Lab at University of Ljubljana, Slovenia) was used for machine learning models learning (16). This open-source platform is a machine learning and data mining suite for data analysis through Python scripting and visual programming (16). The detailed structure for each model includes LiR [regularization type: Lasso (L1), strength α=0.0001], NN [neurons in the hidden layers: 100, 50, 20; activation: rectified linear unit (ReLu); solver: Adam; regularization α=0.0001, and maximum number of iterations: 200, replicable training], AB (Bae estimator: Tree; number of estimators: 50; learning rate: 1.00000; classification algorithm: SAMME.R; regression loss function: linear), RF (number of trees: 10; replicable training; do not split subsets smaller than 5), DT (induce binary tree; minimum number of instances in leave: 2; do not split subsets smaller than 5; limit maximum tree depth: 100; and stop when majority reaches: 95%), LoR (regularization type: ridge L2; strength C =1), and SVM (cost: 1.0; regression loss epsilon: 0.10; kernel: radial basis function; optimization numerical tolerance: 0.0010; and optimization iteration limit: 100).
Ethical statement
The study conformed to the provisions of the Declaration of Helsinki (as revised in 2013). Uijeongbu St. Mary’s Hospital Ethics Committee reviewed and approved the present study (No. UC23RISI0109). The requirement for informed consent was waived because the present study was retrospective without exposure of patient information.
Results
One hundred ninety-nine patients were enrolled into the present study (Figure 2). The mean age of the study participants was 11.61±6.84 years at the age of MIRPE. The mean duration of bar placement and total observation were 28.5±5.08 and 44.1±6.63 months, respectively. One hundred fifty-seven males and 42 females were enrolled. The pectus types comprised 158 symmetric and 41 asymmetric cases. The mean Haller index and RPI before MIRPE were 4.20±1.39 and 4.10±2.00, respectively. The mean RPI before BR and 1 year after BR were 2.55±0.46 and 2.93±0.58, respectively. All subjects showed lower RPI 1 year after BR then before MIRPE. However, 26 of the 199 cases showed recurrence 1 year after BR. The overall clinical characteristics of the study subjects are shown in Table 1.
Table 1
Variables | Value |
---|---|
Age (years)† | 11.61±6.84 |
Sex | |
Male | 157 |
Female | 42 |
Morphology type | |
Symmetric | 158 |
Asymmetric | 41 |
Age group | |
EG (<10 years old) | 105 |
LG (≥10 years old) | 94 |
Recurrence | |
No | 173 |
Yes | 26 |
Initial severity of pectus excavatum | |
Haller index | 4.20±1.39 |
Radiographic pectus index | 4.10±2.00 |
Number of bars used | |
One | 128 |
Two | 69 |
Three | 2 |
Values are shown as mean ± SD or number. †, at the age of MIRPE. EG, early repair group; LG, late repair group; MIRPE, minimally invasive repair of pectus excavatum; SD, standard deviation.
Validity of RPI for degree of PE
Degree of PE is generally described by the Haller index and repair of PE is usually considered with the Haller index ≥3.25 (2,5,17). To validate usage of RPI, we compared the Haller index values and RPI before MIRPE. As in previous studies, we found no significant difference between the Haller index and RPI (P=0.431) and a significant correlation between the Haller index and RPI (P<0.001) (5,9,14).
Chest wall re-depression after BR
Serial changes of RPI were shown to describe chest wall re-depression at the time points after BR (immediate, 1 month, 6 months, and 1 year) based on age group. RPI significantly increased for the first 6 months after BR in both age groups (both P<0.001) but was maintained at 1 year after BR. RPI values of the LG were significantly higher than those in the EG for the entire period after MIRPE (P=0.041) (Tables 2,3). As in our previous study, chest wall re-depression progressed only for the first 6 months (Figure 3) (9). In addition, serial changes of RPI were investigated at subsequent time points after BR (immediate, 1 month, 6 months, and 1 year) based on recurrence. RPI values of the recurrent group (RG) were significantly higher than those of the non-recurrent group (NG) for entire period after BR (P<0.001). Interestingly, RPI of the RG continuously increased after BR while RPI of the NG did not increase after 6 months following BR (Table 4 and Figure 4). These findings suggest that the pattern of chest wall re-depression after BR can differ according to recurrence.
Table 2
Age group | Radiographic pectus index | ||
---|---|---|---|
Before MIRPE | Immediately after MIRPE | Immediate before BR | |
EG (<10 years old) | 3.90±1.15 | 2.46±0.50 | 2.36±0.34 |
LG (≥10 years old) | 4.33±2.64 | 2.69±0.31 | 2.76±0.49 |
P | 0.130 | 0.001 | <0.001 |
Values are shown as mean ± SD. MIRPE, minimally invasive repair of pectus excavatum; EG, early repair group; LG, late repair group; BR, bar removal; SD, standard deviation.
Table 3
Age group | Radiographic pectus index | F | P† | ||||
---|---|---|---|---|---|---|---|
Immediate before BR | Immediate after BR | One month after BR | Six months after BR | One year after BR | |||
EG (<10 years old) | 2.36±0.34 | 2.50±0.36 | 2.65±0.41 | 2.72±0.46 | 2.72±0.43 | 80.04 | <0.001 |
LG (≥10 years old) | 2.76±0.49 | 3.02±0.53 | 3.13±0.55 | 3.17±0.56 | 3.15±0.65 | 2.54 | 0.041 |
P | <0.001 | <0.001 | <0.001 | <0.001 | <0.001 |
Values are shown as mean ± SD. †, Pillai’s trace P value. EG, early repair group; LG, late repair group; BR, bar removal.
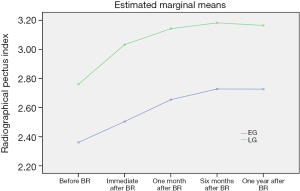
Table 4
Group | Radiographic pectus index | F | P† | ||||
---|---|---|---|---|---|---|---|
Immediate before BR | Immediate after BR | One month after BR | Six months after BR | One year after BR | |||
EG (<10 years old) | 2.45±0.35 | 2.64±0.41 | 2.75±0.41 | 2.80±0.40 | 2.77±0.37 | 221.38 | <0.001 |
LG (≥10 years old) | 3.18±0.63 | 3.50±0.52 | 3.72±0.54 | 3.82±0.62 | 4.00±0.66 | 39.68 | <0.001 |
P | <0.001 | <0.001 | <0.001 | <0.001 | <0.001 |
Values are shown as mean ± SD. †, Greenhouse-Geisser P value. EG, early repair group; LG, late repair group; BR, bar removal.
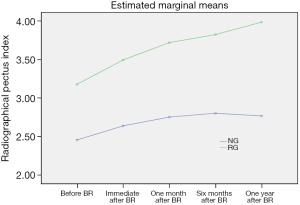
Recurrence of PE
We assume that MIRPE is best performed early, before 10 years of age. Recurrence of PE occurred in five patients in the EG and 21 cases in the LG after BR, a significant difference (P<0.001). Binary logistic regression tests were performed to investigate independent risk factors for recurrence of PE. Recurrence of PE at 1 year after BR was defined as an event and age group, sex, RPI values before and after MIRPE, pectus type (symmetric vs. asymmetric), number of bars placed, and angle of sternum were included as covariates. Accordingly, RPI values before and after MIRPE and age group were identified as independent risk factors for recurrence after BR (P<0.001, P=0.007, and P=0.001, respectively). The results of multivariate analysis of risk factors are shown in Table 5.
Table 5
Variables | Odds ratio | 95% confidence interval | P |
---|---|---|---|
LG (≥10 years old) | 11.663 | 2.747–48.682 | 0.001 |
RPI before MIRPE | 2.970 | 1.717–5.138 | <0.001 |
RPI after MIRPE | 3.894 | 1.454–10.428 | 0.007 |
LG, late repair group; RPI, radiographic pectus index; MIRPE, minimally invasive repair of pectus excavatum.
Prediction of final RPI and recurrence of PE using machine learning models
Five machine learning algorithms were performed to predict the final postoperative RPI and recurrence of PE 1 year after BR, respectively. Perioperative data on age group, sex, RPI values before and after MIRPE, pectus types (symmetric vs. asymmetric), number of bars used, and angle of sternum were included as covariates in the machine learning algorithms. The LiR model outperformed other models, with performance scores of mean squared error 0.198, root mean squared error 0.445, mean absolute error 0.336, and R2 0.415 (Table 6). In addition, age group, sex, RPI values before and after MIRPE, pectus types (symmetric vs. asymmetric), number of bars used, and angle of sternum were included as covariates for prediction of postoperative recurrence of PE. The LoR model outperformed other models for recurrence of PE, with performance scores of AUC 0.865, accuracy 0.884, F1 0.859, precision 0.865, and recall 0.884 (Table 7, Figures 5,6).
Table 6
Algorithms | MSE | RMSE | MAE | R2 |
---|---|---|---|---|
Random forest | 0.220 | 0.470 | 0.358 | 0.350 |
Neural network | 0.617 | 0.786 | 0.411 | −0.821 |
Linear regression | 0.198 | 0.445 | 0.336 | 0.415 |
AdaBoost | 0.258 | 0.508 | 0.393 | 0.238 |
Decision tree | 0.419 | 0.648 | 0.478 | −0.237 |
MSE, mean squared error; RMSE, root mean squared error; MAE, mean absolute error.
Table 7
Algorithms | AUC | Accuracy | F1 | Precision | Recall |
---|---|---|---|---|---|
Random forest | 0.738 | 0.844 | 0.826 | 0.814 | 0.844 |
Support vector machine | 0.701 | 0.874 | 0.820 | 0.890 | 0.874 |
Logistic regression | 0.865 | 0.884 | 0.859 | 0.865 | 0.884 |
Neural network | 0.834 | 0.889 | 0.871 | 0.873 | 0.889 |
Naïve Bayes | 0.847 | 0.854 | 0.863 | 0.876 | 0.854 |
AUC, area under the curve.
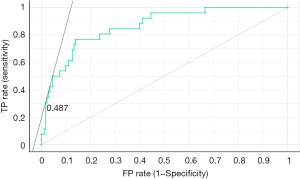
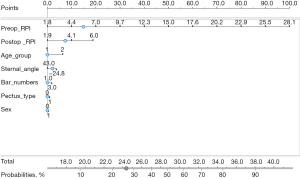
Discussion
Chest wall re-depression after BR is common and remains a concern despite otherwise generally excellent outcomes of MIRPE (5,7,9). This problem highlights several issues associated with MIRPE (4,18). The first regards questions about mechanism and characteristics of chest wall re-depression after BR. However, the mechanisms and characteristics of chest wall re-depression after BR remain unclear (5). Because chest wall re-depression is multifactorial and spatial, clinically available methods or modalities to evaluate and analyze chest wall re-depression are needed (19,20). However, comprehensive evaluations of chest wall depression are difficult (15,19,21). We examined patterns of chest wall re-depression after BR and identified risk factors for recurrence. Chest wall re-depression generally deteriorates only for the first 6 months after BR. This finding suggest that recurrence cannot be diagnosed immediately after BR, and that final diagnosis of recurrence should occur 6 months after BR. The second problem is the defining recurrence of PE, for which there is no consensus (7,9,10). We suggest a definition of recurrence of PE in the present study and create models to predict recurrence. The third problem is the question of the optimal age for MIRPE (8-10). In the present study, we found that the recurrence rate was lower in the EG than in the LG, although the change of RPI after BR showed similar patterns between groups. The lower rate of recurrence in the EG was explained by RPI values before and after MIRPE and age group, which were identified as independent risk factors of recurrence after BR. RPI values before and after MIRPE was lower in the EG than in the LG, although the degree and pattern of chest wall re-depression after BR did not differ by age. These findings are explained by the reliance of MIRPE on chest wall pliability, which may weaken with age (3,8). We conclude that early repair of PE better than late repair with respect to recurrence, and the corrective effect of MIRPE was higher in the EG than in the LG. The fourth concern is prevention of recurrence of PE. To prevent chest wall re-depression and to obtain persistent repair after BR, it is necessary to determine the details of surgical technique, to understand the mechanism of chest wall re-depression, and to investigate risk factors (4,7,9,22). The details of appropriate surgical techniques have achieved a wide consensus among surgeons even if they have minor variations (4,8,22). However, the most important criterion, the degree of repair remains undefined (19). Sufficient elevation of the depressed chest wall is essential to prevent recurrence of PE because RPI values after MIRPE is the most important factor for recurrence (5). Because patients in the LG have higher RPI than those in the EG, we suggest that depressed chest walls in the LG should be lifted more than in the EG to prevent recurrence. Risk factors for recurrence and proper techniques to prevent recurrence have been suggested in previous studies (4,9). However, the results of those studies were inconclusive, and other novel approaches to prevent recurrence are needed. The prediction of recurrence is crucial for preventing recurrence, and the prediction modality should provide information about influencing factors and predictive value for recurrence (12,16,23). Therefore, machine learning algorithms were used in the present study to estimate numerical probability values for recurrence after BR. Machine learning has successfully been used to make accurate decisions and predictions using diverse and large amounts of data (11,16). Models using machine learning algorithms can be used to predict RPI and recurrence after BR using perioperative data during MIRPE. Degree of chest wall depression immediately before and after MIRPE and basic clinical data (age, sex, number of bars used, and sternal angle) were included as variables in the machine learning algorithms (5,9). The prediction model for final RPI and recurrence after BR yielded good outcomes, which is meaningful in real clinical practice. To the best of our knowledge, this is the first study of recurrence after BR using machine learning algorithms and additional studies are required to validate our findings. The approach of integrating machine learning models and readily available clinical data can be used to develop other models for treatment of PE (11).
The present study has several limitations. First, it was a retrospectively study in a single center. The generalizability of the models to other institutions should be studied. Second, the definition of recurrence we used might affect our results. Third, measuring degree of chest wall depression by simple radiography could result in measurement errors that could be influenced by physiologic factors, especially in the asymmetric type. Fourth, the number of subjects in the dataset was relatively small, but we used strict eligibility criteria and study design to obtain data of good quality. PE cannot be simply and strictly defined into two types (symmetric vs. asymmetric) because it has many variant morphologies. In addition, RPI cannot describe the complete degree of PE. The heterogeneity in PE type and incomplete descriptions of degree reflect real-world situations, and machine learning models trained with such data may be more appropriate for clinical practice. PE occupies a minor portion of case in the thoracic surgery field, and knowledge regarding recurrence of PE is valuable because recurrence can have profound effects on patients despite its characterization as a benign condition.
Conclusions
The findings of the present study showed that machine learning algorithms considering risk factors and basic clinical data yield good performance for prediction of final RPI and recurrence after BR. Such machine learning approaches can be convenient and provide accurate decisions in management of PE. Further large-scale studies are required to validate the findings of the present study and suggest surgical details for MIRPE. In addition, an approach integrating machine learning models and readily available clinical data can be used to create other models in the thoracic surgery field.
Acknowledgments
Funding: None.
Footnote
Reporting Checklist: The authors have completed the TRIPOD reporting checklist. Available at https://jtd.amegroups.com/article/view/10.21037/jtd-23-1430/rc
Data Sharing Statement: Available at https://jtd.amegroups.com/article/view/10.21037/jtd-23-1430/dss
Peer Review File: Available at https://jtd.amegroups.com/article/view/10.21037/jtd-23-1430/prf
Conflicts of Interest: All authors have completed the ICMJE uniform disclosure form (available at https://jtd.amegroups.com/article/view/10.21037/jtd-23-1430/coif). The authors have no conflicts of interest to declare.
Ethical Statement: The authors are accountable for all aspects of the work in ensuring that questions related to the accuracy or integrity of any part of the work are appropriately investigated and resolved. The study conformed to the provisions of the Declaration of Helsinki (as revised in 2013). Uijeongbu St. Mary’s Hospital Ethics Committee reviewed and approved the present study (No. UC23RISI0109). The requirement for informed consent was waived because the present study was retrospective without exposure of patient information.
Open Access Statement: This is an Open Access article distributed in accordance with the Creative Commons Attribution-NonCommercial-NoDerivs 4.0 International License (CC BY-NC-ND 4.0), which permits the non-commercial replication and distribution of the article with the strict proviso that no changes or edits are made and the original work is properly cited (including links to both the formal publication through the relevant DOI and the license). See: https://creativecommons.org/licenses/by-nc-nd/4.0/.
References
- Kloth K, Klohs S, Bhullar J, et al. The Epidemiology behind Pectus Excavatum: Clinical Study and Review of the Literature. Eur J Pediatr Surg 2022;32:316-20. [Crossref] [PubMed]
- Janssen N, Daemen JHT, van Polen EJ, et al. Pectus Excavatum: Consensus and Controversies in Clinical Practice. Ann Thorac Surg 2023;116:191-9. [Crossref] [PubMed]
- Toci GR, Davis TA, Bigelow BF, et al. Analyzing Outcomes of Nuss and Ravitch Repair for Primary and Recurrent Pectus Excavatum in Adults. Ann Thorac Surg 2020;110:272-5. [Crossref] [PubMed]
- Haecker FM, Krebs TF, Kleitsch KU. Current Development of Minimally Invasive Repair of Pectus Excavatum (MIRPE). Children (Basel) 2022;9:478. [Crossref] [PubMed]
- Kuyama H, Uemura S, Soh H, et al. Anterior chest wall regression after Nuss bar removal in adult patients with pectus excavatum. Gen Thorac Cardiovasc Surg 2021;69:1308-12. [Crossref] [PubMed]
- Haecker FM, Hebra A, Ferro MM. Pectus bar removal - why, when, where and how. J Pediatr Surg 2021;56:540-4. [Crossref] [PubMed]
- Wang L, Bi R, Xie X, et al. A Modfied Nuss Procedure for Recurrent Pectus Excavatum of Adults. Front Surg 2021;8:814837. [Crossref] [PubMed]
- de Loos ER, Pennings AJ, van Roozendaal LM, et al. Nuss Procedure for Pectus Excavatum: A Comparison of Complications Between Young and Adult Patients. Ann Thorac Surg 2021;112:905-11. [Crossref] [PubMed]
- Cho DG, Kim JJ, Park JK, et al. Recurrence of pectus excavatum following the Nuss procedure. J Thorac Dis 2018;10:6201-10. [Crossref] [PubMed]
- Kuyama H, Uemura S, Yoshida A. Recurrence of pectus excavatum in long-term follow-up after the Nuss procedure in young children based on the radiographic Haller index. J Pediatr Surg 2020;55:2699-702. [Crossref] [PubMed]
- Daemen JHT, Heuts S, Rezazadah Ardabili A, et al. Development of Prediction Models for Cardiac Compression in Pectus Excavatum Based on Three-Dimensional Surface Images. Semin Thorac Cardiovasc Surg 2023;35:202-12. [Crossref] [PubMed]
- Tse KM, Tan LB, Lee SJ, et al. Feasibility of using computer simulation to predict the postoperative outcome of the minimally invasive Nuss procedure: Simulation prediction vs. postoperative clinical observation. J Plast Reconstr Aesthet Surg 2018;71:1496-506. [Crossref] [PubMed]
- Sakamoto Y, Yokoyama Y, Nagasao T, et al. Outcomes of the Nuss procedure for pectus excavatum in adults. J Plast Reconstr Aesthet Surg 2021;74:2279-82. [Crossref] [PubMed]
- Matsuura R, Tazuke Y, Ueno T, et al. Can plain chest X-ray replace computed tomography for the follow-up of children who have undergone the Nuss procedure? Surg Today 2020;50:1249-54. [Crossref] [PubMed]
- Silva B, Pessanha I, Correia-Pinto J, et al. Automatic Assessment of Pectus Excavatum Severity From CT Images Using Deep Learning. IEEE J Biomed Health Inform 2022;26:324-33. [Crossref] [PubMed]
- Demsar J, Curk T, Erjavec A, et al. Orange: Data Mining Toolbox in Python. J Mach Learn Res 2013;14:2349-53.
- Wei B, Gleason F. Commentary: In With The New: Three-Dimensional Surface Imaging For Pectus Excavatum. Semin Thorac Cardiovasc Surg 2022;34:1376-7. [Crossref] [PubMed]
- Abbas AE. Commentary: Funneling the funnel chest debates into appropriate management of adult pectus excavatum. JTCVS Tech 2022;12:220-1. [Crossref] [PubMed]
- Uemura S, Yoshida A, Kuyama H. Analysis of chest wall elevation after the Nuss procedure using 3D body scanning technique in patients with pectus excavatum. Pediatr Surg Int 2021;37:777-82. [Crossref] [PubMed]
- Torre M, Genova Gaia L, Calevo MG, et al. Blood metal levels after minimally invasive repair of pectus excavatum. Interact Cardiovasc Thorac Surg 2021;33:76-81. [Crossref] [PubMed]
- Coorens NA, Daemen JHT, Slump CH, et al. The Automatic Quantification of Morphological Features of Pectus Excavatum Based on Three-Dimensional Images. Semin Thorac Cardiovasc Surg 2022;34:772-81. [Crossref] [PubMed]
- Janssen N, Daemen JHT, Ashour O, et al. Nuss bar removal without straightening is a safe technique: a single center experience. J Thorac Dis 2022;14:3335-42. [Crossref] [PubMed]
- Coorens NA, Daemen JHT, Slump CH, et al. Predicting Aesthetic Outcome of the Nuss Procedure in Patients with Pectus Excavatum. Semin Thorac Cardiovasc Surg 2023;35:627-37. [Crossref] [PubMed]